the Creative Commons Attribution 4.0 License.
the Creative Commons Attribution 4.0 License.
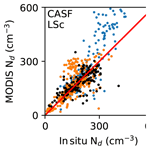
The impact of sampling strategy on the cloud droplet number concentration estimated from satellite data
Edward Gryspeerdt
Daniel T. McCoy
Ewan Crosbie
Richard H. Moore
Graeme J. Nott
David Painemal
Jennifer Small-Griswold
Armin Sorooshian
Luke Ziemba
Cloud droplet number concentration (Nd) is of central importance to observation-based estimates of aerosol indirect effects, being used to quantify both the cloud sensitivity to aerosol and the base state of the cloud. However, the derivation of Nd from satellite data depends on a number of assumptions about the cloud and the accuracy of the retrievals of the cloud properties from which it is derived, making it prone to systematic biases.
A number of sampling strategies have been proposed to address these biases by selecting the most accurate Nd retrievals in the satellite data. This work compares the impact of these strategies on the accuracy of the satellite retrieved Nd, using a selection of in situ measurements. In stratocumulus regions, the MODIS Nd retrieval is able to achieve a high precision (r2 of 0.5–0.8). This is lower in other cloud regimes but can be increased by appropriate sampling choices. Although the Nd sampling can have significant effects on the Nd climatology, it produces only a 20 % variation in the implied radiative forcing from aerosol–cloud interactions, with the choice of aerosol proxy driving the overall uncertainty. The results are summarised into recommendations for using MODIS Nd products and appropriate sampling.
- Article
(6672 KB) - Full-text XML
-
Supplement
(96 KB) - BibTeX
- EndNote
The droplet number concentration (Nd) is a key property of clouds. It is important for setting cloud and precipitation process rates (e.g. Khairoutdinov and Kogan, 2000) as well as cloud radiative properties (George and Wood, 2010; Painemal, 2018). It is closely related to the aerosol environment and the in-cloud updraught (Twomey, 1959), as well as being affected by precipitation processes (Wood, 2012) and entrainment (Baker et al., 1980). With this important role for cloud properties, Nd has been used to evaluate the performance of global climate models (Mulcahy et al., 2018; McCoy et al., 2020; Robson et al., 2020; Grosvenor and Carslaw, 2020).
Variations in Nd are also a primary method for observational characterisations of aerosol effects on clouds (e.g. Quaas et al., 2006). An increase in available cloud condensation nuclei (CCN) will typically produce an increase in Nd, which can result in changes in droplet size and cloud reflectivity (Twomey, 1974), modifications to precipitation processes (Albrecht, 1989), intensification of convection (Williams et al., 2002), and increases in evaporation and potential cloud desiccation (Wang et al., 2003; Ackerman et al., 2004). This has made aerosol relationships with Nd the target of a large number of observational studies (e.g. Quaas et al., 2006, 2008; Ghan et al., 2016; Gryspeerdt et al., 2017; McCoy et al., 2017; Hasekamp et al., 2019). With a central role in aerosol–cloud interactions, Nd relationships with other cloud properties, particularly cloud fraction (CF; Gryspeerdt et al., 2016) and liquid water path (LWP; Han et al., 2002), have also been used to quantify cloud adjustments due to aerosols.
Assessments of the effective radiative forcing due to aerosol–cloud interactions (ERFaci) rely heavily on these observation-based estimates of aerosol–cloud interactions (Boucher et al., 2013; Bellouin et al., 2020), and these estimates in turn rely on accurate observations of aerosol–Nd and Nd–cloud relationships. Reliable satellite and remotely sensed observations of Nd are therefore essential for reducing uncertainties in the anthropogenic impact on clouds and the climate.
There are a number of methods for retrieving cloud droplet size and Nd from space (Boers et al., 2006; Zeng et al., 2014; Austin and Stephens, 2001; Hu et al., 2021), but the majority of previous studies make use of the cloud droplet number calculated from a bispectral retrieval of the cloud optical depth (τc) and effective radius (re; Nakajima and King, 1990), assuming an adiabatic cloud (Boers et al., 2006; Quaas et al., 2006). Previous studies in stratocumulus regions have found a good agreement between the satellite and in situ Nd (Painemal and Zuidema, 2011; Kang et al., 2021), but this retrieval depends on assumptions with varying applicability (Grosvenor et al., 2018b). To improve our knowledge of Nd across the globe, a number of sampling strategies have been applied in recent work to select more reliable retrievals (Quaas et al., 2006; Grosvenor et al., 2018b; Bennartz and Rausch, 2017; Zhu et al., 2018), based on the characteristics of the retrieval and the observed liquid clouds.
Each of these sampling strategies is based on an understanding of cloud physics and the character and reliability of satellite retrievals such that it is not immediately clear which is most suitable for selecting valid Nd retrievals. In addition, as Nd products are used for a variety of different tasks, different sampling methods may be more appropriate for each. Removing low-optical-depth clouds may limit the Nd retrieval to more accurate cases but may produce a biased climatology and estimates of the ERFaci by neglecting a large fraction of the cloud population (Leahy et al., 2012). This work examines these sampling strategies and how the choices made impact the accuracy of the Nd retrieval when compared to in situ data, the representativeness of the Nd climatology and the impact of these choices on the implied aerosol–cloud radiative forcing.
2.1 Nd from satellite
Nd is rarely retrieved directly but is estimated from the cloud optical depth (τc) and effective radius (re). Assuming an adiabatic cloud (no precipitation or mixing with its environment), Nd is derived from the retrieved properties (τc, re) following Brenguier et al. (2000), Quaas et al. (2006) and Boers et al. (2006):
where the density of water ρw and the scattering efficiency Q (equal to 2) are assumed constant. , where rv is the droplet mean volume radius, depends on the droplet size spectrum. Although k has been observed to vary in in situ studies (Martin et al., 1994) and it may vary under particularly extreme aerosol environments (Noone et al., 2000), this work uses a constant value of 0.8, following Painemal and Zuidema (2011) and Grosvenor and Wood (2014).
The condensation rate cad is a function of temperature and pressure. Assuming a saturated adiabatic lapse rate, the pressure dependence is weak, but a temperature change from 270 to 300 K can double the condensation rate and hence Nd. To account for this variation, Nd is calculated using the linear Nd temperature correction from Gryspeerdt et al. (2016), using the cloud top temperature (a suitable assumption if the cloud layers are thin; Grosvenor and Wood, 2014).
The sub-adiabatic factor (fad) in Eq. (1) represents the reduction in the condensation rate due to mixing with sub-saturated environmental air. However, a full accounting for sub-adiabaticity also requires a potential change in the droplet size distribution (except under extreme inhomogeneous mixing), which modifies the k parameter. Previous work has suggested that there might be a cancellation between these two effects (Painemal and Zuidema, 2011). Observational studies have found a range of values for the adiabatic factor from 0.63 (Merk et al., 2015), 0.74 (Kang et al., 2021), 0.8 (Braun et al., 2018), 0.88 (Painemal et al., 2017) and 0.9 (Painemal and Zuidema, 2013). In this work a constant factor of 0.8 is used, noting that this may be responsible for an offset in the retrieved Nd.
2.2 Satellite sampling
Two of the major uncertainties in the Nd retrieval are the cloud adiabaticity assumption and the accuracy of the cloud retrievals used to derive Nd. This work examines sampling strategies to minimise these uncertainties in the MODIS collection 6.1 cloud optical properties retrieval (MOD06_L2) dataset for both Aqua and Terra (Platnick et al., 2017). This is a bispectral retrieval (Nakajima and King, 1990), with known uncertainties in broken-cloud situations and where there are large variations in the effective radius (Zhang and Platnick, 2011). The Nd sampling methods in this work (Table 1) aim to reduce these uncertainties through sampling retrievals with higher confidence.
Only liquid water clouds can be considered here, so our analysis is restricted to cases with a valid optical properties retrieval and a retrieved liquid water phase. As a baseline strategy, this sampling method is referred to as “All” throughout this work. Unless otherwise noted, the re and τc values come from the standard MODIS 2.1 µm retrieval.
With a high uncertainty in re retrievals at a low τc and a degeneracy in the retrievals for a low re (where multiple τc and re combinations have the same reflected radiances), Quaas et al. (2006) suggested the exclusion of cases with a τc or re less than 4 (or 4 µm) when calculating Nd. This sampling is hence called “Q06”.
Maddux et al. (2010) and Grosvenor and Wood (2014) demonstrated the uncertainties at high solar zenith and satellite viewing angles, where cloud 3D effects and multiple scattering generate uncertainties, in both re and τc. The non-linear nature of these retrievals can also bias retrievals in broken-cloud and inhomogeneous scenes (Zhang and Platnick, 2011). Recognising these issues, Grosvenor et al. (2018b) make several recommendations to avoid these problematic retrievals. Following these, cases with a solar zenith angle >65∘, a satellite viewing zenith angle >55∘ and a cloud mask SPI (sub-pixel inhomogeneity index, the standard deviation relative to the mean of the 250 m radiances; Liang et al., 2009) >30 % are excluded. To select more homogeneous cloud cases, pixels with a 5 km cloud fraction less than 0.9 are also excluded. This is in addition to the Q06 sampling. This sampling strategy is named “G18”.
These sampling strategies focus primarily on the properties of the retrievals. However, the cloud adiabaticity plays an important role in the Nd retrieval. The final two methods make attempts to address this. Bennartz and Rausch (2017) propose a method for locating adiabatic pixels by comparing re at different wavelengths. The re value retrieved using the 3.7 µm band is typically located closer to the cloud top than the 2.1 and 1.6 µm re retrievals, due to the wavelength dependence of water absorption (Platnick, 2000). For an adiabatic cloud, re at 3.7 µm is therefore expected to be larger than the shorter wavelengths (and re at 2.1 µm > re at 1.6 µm), although other factors including retrieval biases can also impact these relationships (Zhang and Platnick, 2011). Only pixels satisfying these inequalities (known as re stacking) are included in this sampling method. As it is applied on top of G18, it is more stringent than the sampling proposed in Bennartz and Rausch (2017) but is named “BR17” due to the importance of the re-stacking criterion.
Finally, Zhu et al. (2018) suggest that the adiabatic fraction can be maximised by only using data from cloud “cores” – the 10 % highest τc values in 100 km × 100 km regions. This is applied on top of the G18 sampling and called “Z18”. As with BR17, this is more stringent than Zhu et al. (2018), due to the additional filters inherited from G18.
The application of BR17 and Z18 on top of G18 (different from the original papers) is due to the different aims of these sampling strategies (Table 1). G18 focusses on the identification of uncertain retrievals, while BR17 and Z18 make statements about cloud adiabaticity. Both BR17 and Z18 benefit from the sampling in G18, and applying them on top of G18 makes it easier to assess the impact of the adiabaticity statements in these sampling strategies.
These sampling strategies are all applied at 1 km resolution (pixel level). These retrievals are aggregated to daily means at a 1∘ × 1∘ resolution for aerosol susceptibility calculations.
2.3 Aircraft data selection
To assess these sampling methods, satellite retrievals are compared to aircraft measurements of Nd. A selection of aircraft data is used to provide a variety of different cloud and meteorological conditions, including marine stratocumulus (a key region for the radiative forcing from aerosol–cloud interactions), mid-latitude storm tracks and the Southern Ocean (Fig. 1).
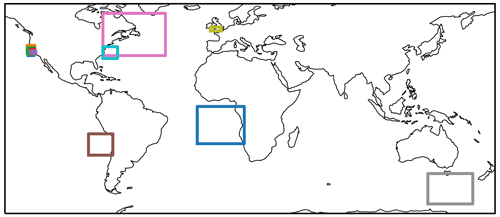
Figure 1Locations of the campaigns used in this work. Colours are shown in Fig. 2.
Stratocumulus data come from the CIRPAS (Center for Interdisciplinary Remotely Piloted Aircraft Studies) Twin Otter data in Sorooshian et al. (2018), including data from the E-PEACE (Eastern Pacific Emitted Aerosol Cloud Experiment), FASE (Fog and Stratocumulus Evolution Experiment), MACAWS (Marine Aerosol Cloud and Wildfire Study), MASE1 and MASE2 (Marine Stratus/Stratocumulus Experiment) campaigns. These campaigns took place over the northeastern Pacific near the coast (Fig. 1). These campaigns had a consistent use of the CASF (the forward-scattering component of the cloud, aerosol and precipitation spectrometer) and a large number of intersections with the MODIS instrument. For these campaigns the liquid water content (LWC) comes from the PVM-100A probe on the Twin Otter. Data from the NCAR (National Center for Atmospheric Research) C-130 during VOCALS-REx (Variability of the American Monsoon Systems (VAMOS) Ocean-Cloud-Atmosphere-Lands Study – Regional Experiment; Wood et al., 2011) provide measurements of a different stratocumulus region. The C-130 used a cloud droplet probe (CDP) to measure the droplet size spectrum. Data from the phase Doppler interferometer (PDI) on board the P-3 during ORACLES (ObseRvations of Aerosols above CLouds and their intEractionS; Redemann et al., 2021) are used to provide Nd measurements of the Namibian stratocumulus deck. Only data from 2016 and 2018 are used, due to issues with the PDI in 2017.
Four other flight campaigns are used to investigate the Nd retrieval in a broader range of clouds, often in more challenging conditions. Data for North Atlantic boundary layer clouds come from the North Atlantic Aerosol and Marine Ecosystem (NAAMES) campaign (Behrenfeld et al., 2019). A CDP was used to measure the droplet size distribution during a 3-year period (2015–2017). Data from ACTIVATE (Aerosol Cloud meTeorology Interactions oVer the western ATlantic Experiment; Sorooshian et al., 2019) include Nd data from a CDP during 2020, aimed primarily at shallow liquid clouds (cumulus and winter postfrontal stratocumulus) off the eastern coast of the USA. SOCRATES (Southern Ocean Clouds, Radiation, Aerosol Transport Experimental Study; McFarquhar et al., 2021), aimed at Southern Ocean clouds, provides CDP observations of Nd in a challenging, often mixed-phase environment. Finally, COPE (Convective Precipitation Experiment Leon et al., 2016) used a CDP to measure Nd in convective environments. For the COPE campaign, LWC data come from the Johnson Williams instrument; for all other campaigns using a CDP, the LWC is calculated from the CDP size distribution.
For each flight campaign, 1 Hz data are used. For the CDP instruments, the total particle number (2–50 µm) is used. For the campaigns using CASF and PDI data, bins are selected (with a linear interpolation for partial bins) to produce an Nd representative of the range 2–30 µm (the exact values have little effect on the results presented in this work). A correction for advection between the satellite and aircraft measurement times is applied, along with a parallax correction based on the aircraft height.
2.4 In situ data sampling
As the aim of this work is to evaluate the satellite sampling strategies and products, extensive filtering on the aircraft data is not performed, relying on the satellite to select cases where there are valid Nd retrievals (as is required for a global product). In particular, no attempt is made to select the Nd value at the cloud top. While the Nd retrieval uses cloud top re, it is based on the assumption that Nd is constant throughout the cloud depth. This assumption is valid on average for VOCALS-REx (Painemal and Zuidema, 2011), SOCRATES (Kang et al., 2021) and NAAMES (Painemal et al., 2021) but may not be for a non-adiabatic cloud. A satellite retrieval has to be able to identify these situations.
The LWC–Nd relationship in Fig. 2 shows a very strong relationship at low LWC values, likely due to inhomogeneous mixing reducing Nd and LWC at cloud edges (Baker et al., 1980). To ensure that the in situ Nd measurements are representative of the whole cloud, rather than a mixing region close to a cloud edge, a uniform minimum LWC of 0.1 g m−3 is used, discarding aircraft Nd measurements below this when comparing to the satellite retrievals.
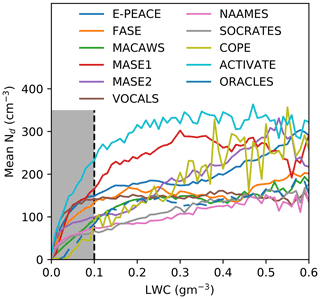
Figure 2Average aircraft Nd as a function of LWC. Aircraft data with an LWC less than 0.1 g m−3 are excluded from this analysis.
The aircraft data are aggregated and compared to MODIS data at a pixel level (1 km × 1 km at nadir). For each MODIS pixel, all the 1 Hz aircraft data within that pixel (that satisfy the sampling criteria) are averaged together. A pixel must have more than two aircraft points (2 s) of data to be included in this analysis. To minimise errors from cloud motion and cloud development, a co-incidence time between the satellite and aircraft data of less than 15 min is required.
2.5 Aerosol data
Assessing the impact of Nd sampling techniques implied radiative forcing from aerosol–cloud interactions (RFaci); the susceptibility of Nd to aerosol (β) variations is calculated (Feingold, 2003):
where A is an aerosol proxy. Three aerosol proxies are used in this work, with all β values calculated at 1∘ × 1∘ resolution. The aerosol optical depth (AOD) is a simple proxy used in previous work (e.g. Quaas et al., 2008), but that underestimates the aerosol impact on clouds (Gryspeerdt et al., 2017). The aerosol index (AI), defined as the AOD multiplied by the Ångström exponent (Nakajima et al., 2001), is able to diagnose the RFaci to within 20 %, provided accurate retrievals of AI and Nd (Gryspeerdt et al., 2017). Following Hasekamp et al. (2019), AI retrievals less than 0.1 are discarded due to their high uncertainty. Both AOD and AI are from the daily mean MODIS collection 6.1 1∘ × 1∘ product (MYD08_D3). The AOD is the combined Dark Target (Levy et al., 2013) and Deep Blue (Sayer et al., 2014) product, while the AI is calculated from the AOD–Ångström exponent joint histograms over ocean only. Reanalysis aerosol products are also a potential aerosol proxy, correlating well to Nd in a variety of environments (McCoy et al., 2017). The MERRA-2 (Modern-Era Retrospective analysis for Research and Applications) 900 hPa SO4 concentration is also used as an aerosol proxy, as in McCoy et al. (2017).
To estimate the contribution of sensitivity variations to the implied RFaci, the RFaci is calculated as
where F↓ is the CERES downwelling flux; fc is the MODIS liquid cloud fraction; and αc is the cloud albedo, derived from the MODIS cloud optical depth. These estimates are calculated at a 1∘ × 1∘ resolution.
3.1 Satellite and in situ comparison (pixel level)
Given the large number of assumptions and uncertainties in the Nd retrieval, the agreement between MODIS and in situ Nd is surprisingly good (Fig. 3). Coefficients of determination (r2 – the square of the Pearson product-moment correlation coefficient) for the stratocumulus campaigns are in the range 0.5 to 0.8 (Table 2). For the more challenging situations, the coefficient of determination is lower (in the range 0.25 to 0.5) but still shows skill at retrieving Nd.
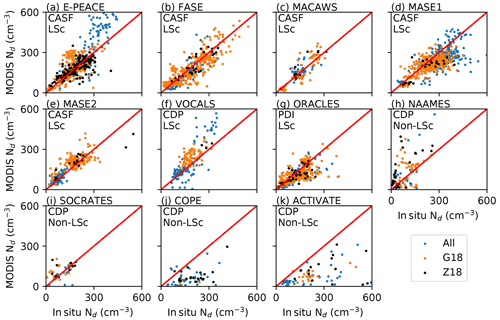
Figure 3Comparison between MODIS and in situ Nd at a pixel level, for aircraft data within 15 min of a MODIS (Aqua or Terra) overpass. Blue is data that do not satisfy G18; orange dots are G18 sampling; and green are Z18. The instrument used in each campaign and the main cloud type (LSc – liquid stratocumulus) is shown in the top left of each subplot.
Even for the least stringent filtering (All), r2 values remain high for the stratocumulus campaigns (as in Painemal and Zuidema, 2011). This agreement holds even for some of the large Nd values (500 cm−3) seen in E-PEACE (Fig. 3a) and FASE (Fig. 3b), even though these pixels are removed by the G18 and Z18 sampling strategies as potentially biased.
The retrievals for most of the stratocumulus campaigns have high r2 values (Table 2) and close alignment to the 1:1 line (Fig. 3). However, in some of the more challenging situations, particularly NAAMES and SOCRATES, MODIS can overestimate the in situ values (Fig. 3h–k), sometimes by more than 100 cm−3. Even the more stringent sampling strategies of G18 and Z18 are unable to identify these pixels as biased, suggesting that further filtering techniques are be required to provide accurate Nd values under these circumstances.
All the sampling strategies fail to accurately characterise Nd from COPE. Convective clouds are a uniquely challenging environment for the Nd retrieval, with strong mixing limiting potential adiabatic locations (Eytan et al., 2021). Not only does this limit the applicability of Eq. (1), but the extremely heterogeneous clouds also limit the accuracy of the MODIS retrievals (Zhang and Platnick, 2011), and large variations in Nd increase representation errors for the aircraft data. The comparisons with ACTIVATE are slightly better, especially for the more restrictive sampling strategies. Even so, MODIS typically produces underestimates of Nd when compared to the in situ data. This is expected in broken-cloud and inhomogeneous scenes, which lead to overestimates in re (Zhang and Platnick, 2011) and corresponding underestimates in Nd.
Table 2Coefficient of determination (r2) for MODIS in situ comparisons for the 2.1 µm retrieval. “–” indicates too few points to calculate a correlation. The “Average” row is the average r2 across the campaigns, and the “All” row is the r2 value for all the valid data points across all campaigns (with 5 % and 95 % bounds). The bottom rows show the root mean squared deviation (RMSD), the RMSD normalised by the mean Nd and the mean bias (MODIS–in situ) across all the campaigns. Numbers of data points for each campaign are shown in Fig. 6.
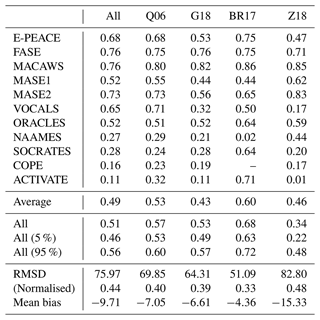
Considering all the available pixel-level matches between MODIS and the in situ data, BR17 produces the strongest overall correlation, with an r2 value of 0.68 and a low mean bias (defined as MODIS Nd minus in situ Nd) of −4.36 (Table 2). The bias is negative for all sampling strategies (a MODIS underestimate), likely due to overestimates in the effective radius (Zhang and Platnick, 2011). Both Q06 and G18 are improvements on using all data, with only a 10 % and 25 % reduction in the data volume respectively. In comparison, BR17 discards almost 63 % of available liquid cloud pixels. Interestingly, while Z18 often produces high correlations to the in situ data, the overall r2 (0.34) and bias (−15.33) values are lower than any other sampling strategy. This is partly due to it preferentially selecting sub-adiabatic convective retrievals in the more convective campaigns (e.g. COPE), as it selects the highest optical-depth cases. Although the correlation in a single campaign can be high, the bias varies between campaigns and so produces a worse correlation overall.
3.2 Other sampling choices
3.2.1 Should I use a minimum cloud fraction?
The G18 strategy introduces filtering by the 5 km CF, ensuring the retrieval is more than 2 km from a cloud edge. While this reduces the impact of cloud inhomogeneities, some studies have required a high 1∘ × 1∘ liquid CF to further reduce the impact of this uncertainty (e.g. Grosvenor et al., 2018a). This can remove broken-cloud scenes where retrieval uncertainties can be higher.
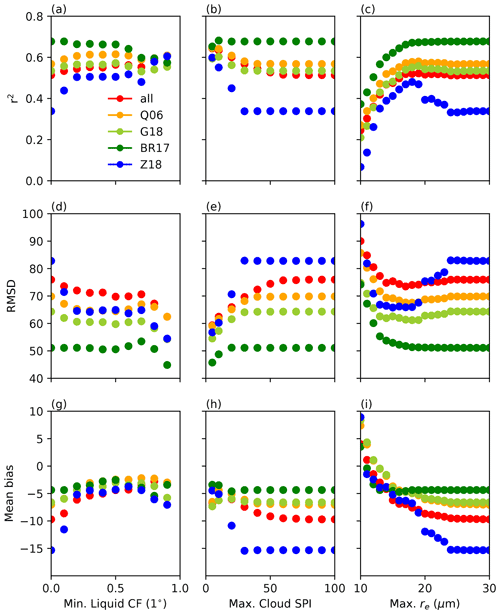
Figure 4The impact of filtering by (a) large-scale liquid cloud fraction, (b) pixel-level cloud SPI and (c) the maximum permitted re on the total r2 for each sampling strategy. (d, e, f) As (a), (b) and (c) but for the root mean squared deviation. (f, g, h) As (a), (b) and (c) but for the mean bias.
Specifying a minimum large-scale liquid CF has a relatively small impact on r2 (Fig. 4a), with a gradual increase in the total r2 as the minimum cloud fraction increases for the majority of sampling strategies. There is a corresponding decrease in the data volume; only around 50 % of investigated pixels have a total liquid CF > 90 %, but it would improve the accuracy of the remaining retrievals if that was the only consideration.
The Z18 sampling shows a slightly larger increase in r2 as the minimum CF increases, becoming the highest-accuracy strategy for a high liquid CF (Fig. 4a). This is likely due to the cloud core assumption of Z18 being most valid for closed-cell stratocumulus cases. This suggests that while the Z18 sampling might be less suited to broken-cloud cases, it could be preferred in environments of a high liquid CF.
Similar effects are seen in the RMSD, where there is a small decrease in RMSD as the minimum cloud fraction increases. There is a slight decrease in the mean bias as the minimum liquid cloud fraction increases such that all the sampling strategies have a very similar mean bias for cases of a high liquid CF.
3.2.2 Which SPI threshold should I use?
G18 also introduces a cloud mask SPI threshold, which aims to exclude pixels with sub-pixel variation in cloud properties. Gryspeerdt et al. (2019) used a maximum value of 30 %, finding that further limiting this value made little difference to their results. However, for the pixel-level MODIS–in situ comparison (Fig. 3), limiting the SPI further produces a measurable increase in the accuracy of the MODIS Nd retrieval (Fig. 4b), particularly for the Z18 sampling strategy. This limitation also decreases the RMSD (Fig. 4e) and mean bias (Fig. 4h).
Using a maximum SPI of 5 % reduces the available data with the All strategy by 45 %. This is only a 29 % reduction for the Z18 strategy (where SPI is already limited to a maximum of 30 %; Table 1). If a higher accuracy is required, a lower SPI limit can help achieve this. A very strict SPI limit significantly reduces the accuracy difference between the sampling strategies and may be a more data-efficient way to achieve accuracy levels close to BR17 than re stacking (Fig. 4b).
3.2.3 Should I use a maximum re?
A large cloud top re has been proposed as an indicator of warm rain (Rosenfeld and Gutman, 1994). As a precipitating cloud is non-adiabatic, this creates a systematic bias as a function of re. Restricting the Nd calculation to a maximum re might potentially increase the overall accuracy of the sampled Nd.
For all the sampling strategies, setting a very low maximum re (<15 µm) results in a reduction in the accuracy of the Nd retrieval by removing most of the data being studied (Fig. 4c, f). A very high maximum re recovers the values from Table 2. For Z18, there is an increase in accuracy between these two limits, with a maximum correlation between the MODIS and in situ Nd for a maximum re of around 15 µm. This may be due to Z18 targeting retrievals in cloud cores where precipitation is more likely. In these situations, removing precipitating cases would have the biggest effect on the accuracy of the Nd retrieval. Further accuracy improvements may be found from using a more sophisticated precipitation threshold, such as H3 / Nd, where H is the cloud depth (vanZanten et al., 2005). In contrast, a maximum re has no impact on the BR17 filtering, as the re stacking is already designed to filter out precipitating cases.
The impact of a maximum re on the mean bias (Fig. 4i) shows some similar properties, with little change at very large values for re. For the all data and Z18 strategies, there is a significant improvement in the mean bias limiting retrievals to a maximum re of less than 20 µm. This may be related to the focus on cloud cores in Z18 (which are more likely to be precipitating). Very stringent re filtering shifts the bias positively for all sampling strategies, due to the reduction in cases of high re that produce potential Nd underestimates. However, the exact correction for Nd varies depending on the cloud field, making this an unreliable method for correcting Nd biases.
3.2.4 Which wavelength should I use?
The standard MODIS re retrieval uses the 2.1 µm band. In broken-cloud and inhomogeneous conditions, the 3.7 µm re retrieval is expected to produce more accurate re retrievals (Zhang and Platnick, 2011; Painemal and Zuidema, 2013). For ideal clouds, the 3.7 µm retrieval retrieves re closer to the cloud top and the 1.6 µm retrieval deeper into the cloud. With potential compensating errors, it is not clear which wavelength retrieval gives the best Nd.
Table 3The impact of re wavelength on the total r2. The second set of values is for only the non-stratocumulus campaigns.
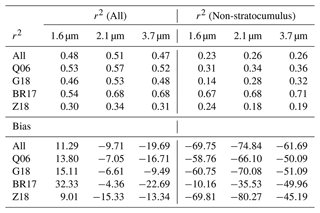
The agreement between the MODIS and in situ Nd values depends on the absorbing wavelength used in the joint τc–re retrieval (Table 3). When considering all the data together, the 2.1 µm retrieval has a higher r2 for all the sampling strategies (with similar performance for the 1.6 µm and 3.7 µm retrievals), other than BR17 (where the effective radius stacking criterion imposes a strict relationship between re at different wavelengths). The 2.1 µm retrieval is also the least biased against the in situ data, typically having an underestimate of less than 10 cm−3, while the 1.6 µm overestimates Nd, and the 3.7 µm retrieval underestimates Nd by a similar amount.
Considering all the campaigns together hides the behaviour in more challenging situations. In non-stratocumulus situations, the 1.6 µm Nd retrieval does not perform as well as the standard (2.1 µm) retrieval, whereas the 3.7 µm retrieval performs slightly better than the standard (Table 3, right three columns). The variation in non-stratocumulus campaigns is consistent with inhomogeneity generated biases in re retrievals, where the 3.7 µm retrieval performs better in broken-cloud environments (Zhang and Platnick, 2011).
The biases in these more challenging conditions are larger and universally negative (due to the re overestimate in broken-cloud conditions). The 2.1 µm retrieval shows the largest mean bias under these conditions, with the 3.7 µm retrieval having the smallest bias and the 1.6 µm retrieval being in between. For the BR17 strategy, the 1.6 µm retrieval has the smallest bias, due to the re stacking criterion. With a higher r2 and a lower mean bias, the 3.7 µm retrieval could be preferred in these broken-cloud conditions.
3.3 Should I correct for penetration depth biases?
The derivation of Eq. (1) assumes re is from the cloud top, but satellite retrievals provide re at a distance below the cloud top, based on the photon penetration depth (Platnick, 2000). This low bias in re is hypothesised to lead to a high bias in Nd, particularly for thin clouds (Grosvenor et al., 2018a).
Applying the Grosvenor et al. (2018a) correction for penetration depth results in a reduced high Nd bias at high Nd for the VOCALS and E-PEACE campaigns (not shown). For the other campaigns, there is either little change or a decrease in Nd retrieval accuracy. This may be due to compensating biases in the Nd retrieval and the Q06 sampling removing cases with low optical depths where this penetration depth bias is strongest. Although this correction is not applied in this work, as the quality of Nd retrievals improves, the penetration depth bias may play a more important role in the overall Nd error budget.
3.4 Satellite and in situ comparison (1∘ × 1∘)
Many studies using data derived from the MODIS Nd do so at 1∘ × 1∘ resolution. Although in situ data have difficulty representing such a large region, it is instructive to make a simple comparison between MODIS and in situ data at this resolution (see also McCoy et al., 2020). It is not possible to collect aircraft data to perfectly characterise an entire grid box this size. To increase the representation of the data for each grid box, 300 s of in-cloud aircraft data and more than 2000 MODIS pixels are required for each 1∘ × 1∘ grid box. Only 200 MODIS pixels are required for the Z18 mask, as it makes an explicit aim to select fewer but more representative MODIS pixels. While there is not an explicit selection for specific campaigns, these representation criteria implicitly bias the results in Fig. 5 towards the liquid stratocumulus campaigns.
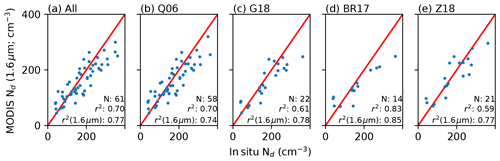
Figure 5Comparison of 1∘ × 1∘ mean in situ and MODIS Nd. Requires at least 300 in situ measurements and 2000 valid MODIS retrievals in a grid box. Each scatterplot also shows the number of points and the r2 value, along with the r2 value for the 1.6 µm retrieval in the bottom right. The 1.6 µm retrieval is used, as it offers the best correlation to in situ Nd at 1∘ × 1∘.
The correlations between the in situ and MODIS data are high (Fig. 5), with r2 values above 0.7 even when considering all available liquid pixels. This is considerably higher than the pixel-level correlations in Table 2. The correlations increase for the more restrictive sampling methods, although there is a corresponding decrease in the number of valid grid boxes. The r2 value reaches 0.8 for BR17, increasing still further when using the 1.6 µm retrieval (Fig. 5). Although the strategy requiring a large coverage of MODIS and in situ data biases this comparison toward high CF values, stratocumulus regimes where the Nd assumptions are more likely to hold, this comparison gives increasing confidence that the MODIS Nd retrieval is capable of accurately retrieving Nd at a pixel level and over larger regions.
4.1 Representing the Nd climatology
A key requirement for an Nd retrieval is the ability to represent the Nd climatology, especially if it is being used to constrain model simulations (Mulcahy et al., 2018; McCoy et al., 2020). While BR17 has the lowest mean bias (Table 2), this is only for the pixels that satisfy the sampling strategy. This may not be a good representation of the overall Nd climatology. This is already conceptually difficult, as a model maintains Nd even in situations with a very low LWC where a satellite or aircraft is unable to measure Nd, requiring the use of a satellite simulator.
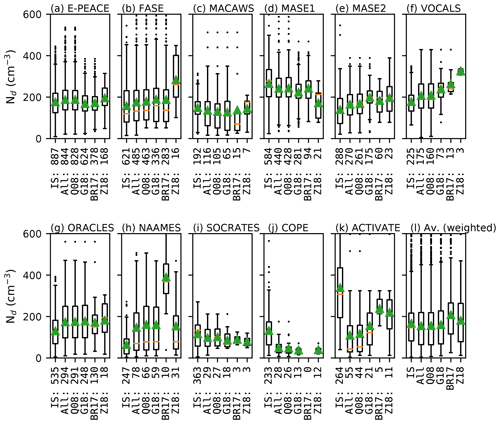
Figure 6Comparison between the MODIS and in situ Nd distributions for each campaign. In each subplot, the in situ distribution is the left-most boxplot, composed of all the valid in situ data points with a coincident (within 30 min) satellite view (from either Aqua or Terra), independent of whether there is a valid retrieval. Green triangles are the mean, and orange lines are the median. The other boxes in each subplot are the distributions of valid satellite Nd retrievals for each sampling strategy that are coincident with aircraft measurements. The number of Nd data points for each boxplot is given below the x axis; (l) is the average of all campaigns (equally weighted).
Figure 6 shows how well each of the satellite sampling strategies represents the climatology of in situ Nd data for all the potential locations in each campaign. For each sampling strategy, the number of remaining data points is shown along the x axis.
In general, the satellite sampling strategies all do a good job representing the climatology, particularly in stratocumulus regions (as expected following their agreement in this regime; see Fig. 7). However, for NAAMES, both BR17 and Z18 appear to slightly overestimate the mean Nd for the campaign. This may also be the case for ACTIVATE, but the low number of intersections limits our ability to draw strong conclusions. The overestimate in NAAMES appears to be due to the sampling method keeping pixels where MODIS overestimates Nd whilst discarding cases with better agreement but a lower MODIS Nd (Fig. 3g).
The distribution for the complete dataset is dominated by the stratocumulus campaigns, particularly E-PEACE. The similarity of Nd from the different sampling strategies (Fig. 7) means that there is relatively little variation between the regimes, although BR17 and Z18 (and to a lesser extent G18) have a narrower Nd range compared to the in situ data. Weighting each campaign equally (Fig. 6l) shows that, for these campaigns, BR17 has the tendency to remove the lowest Nd values (giving it a slightly high bias) and Z18 tends to remove the highest.
For representing the climatology, this suggests that G18 may be a better choice, particularly outside of stratocumulus regimes. However, the small number of satellite–aircraft comparisons in these cases limits current confidence in the accuracy of the satellite Nd climatology outside stratocumulus.
4.2 Satellite climatologies
The different sampling strategies for the MODIS Nd produce broadly similar Nd climatological patterns (Fig. 7), with higher Nd values over land and in coastal regions and lower values over the remote ocean. While some previous studies have removed data over land, it is kept here, as Nd information over land is used for observation-based estimates of the RFaci.
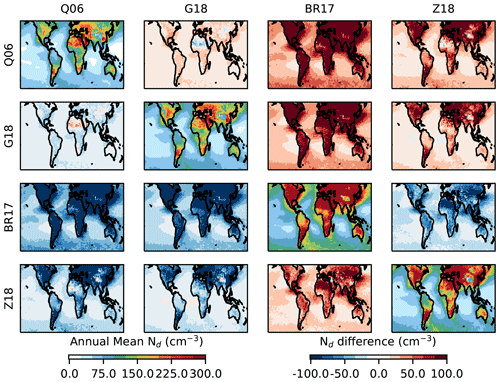
Figure 7MODIS Nd climatology (2011–2020) for different sampling strategy. The diagonal is the annual mean Nd for each strategy, while the off-diagonal plots show the difference (e.g. the top right is Z18−Q06).
The mean Nd and land ocean contrast differ significantly between sampling methods. While Q06 and G18 have similar global patterns, the G18 mean is typically higher than Q06, with this increase being slightly larger over land than ocean (Fig. 7). BR17 produces a significantly larger Nd across most of the globe (particularly over land) than either Q06 or G18. Similar to BR17, the Z18 enhancement over land is also large (although smaller than BR17), but there is a smaller overall enhancement over ocean.
The difference between the sampling strategies is much smaller in stratocumulus regions, where the CF is larger. In these regions, clouds are much more likely to be adiabatic (and so more likely to satisfy the BR17 re stacking criterion). This means that even sampling methods that do not apply this criterion directly will satisfy it most of the time, leading to the small difference in mean Nd between the sampling methods (consistent with the results in Fig. 4a). Over ocean, there is a significant difference in the mean Nd along the eastern coasts of North America and Asia, where the liquid CF is lower and retrievals are more challenging.
4.3 Data coverage
The similarity between the climatologies derived from the different sampling methods hide the very different data coverage (Fig. 8). With a relatively relaxed sampling criterion, Q06 has an Nd retrieval in the majority of available MODIS grid boxes. This is larger than the liquid cloud fraction, as only a single valid Nd pixel is required to count a 1∘ × 1∘) grid box as “retrieved”. Only regions with large ice-cloud coverage (the warm pool and over land) have a significantly lower fraction of retrievals.
With much more stringent filtering, G18 provides an Nd retrieval on only around 30 % of days, climbing to around 50 % of days in stratocumulus regions. While many of the G18 sampling conditions are based on geometric properties, these also rely on the cloud SPI, which is typically lower in stratocumulus regions (as they are more homogeneous). This inhomogeneity criterion also contributes to the significantly reduced retrieval fraction over land.
As an even more stringent sampling strategy, BR17 has valid retrievals on an even lower fraction of days. While similar to G18 in the middle of the stratocumulus decks, the requirement for stacked re retrievals limits the retrievals primarily to these regions, with very few retrieved points away from stratocumulus decks. Z18 has a pattern similar to G18. As it selects just the highest 10 % of τc within each 100 km region, it can return a retrieval on any day in a grid box where G18 has more than 10 valid retrievals, with around 25 % of days having a valid Nd retrieval.
4.4 Aerosol–cloud sensitivities and RFaci
Another major use for Nd is calculating aerosol–cloud sensitivities, either for use as an emergent constraint (Quaas et al., 2009) or for making direct estimates of the RFaci and ERFaci (e.g. Quaas et al., 2008).
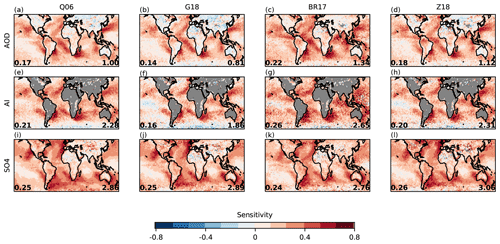
Figure 9Maps of the sensitivity of Nd to a selection of aerosol proxies (βN). Each plot shows the global mean βN in the lower left and the ratio of the implied RFaci to that calculated using Q06 Nd and AOD (a) in the lower right.
As shown in Fig. 9, the sensitivity (as defined in Eq. 2) is largely unaffected by the choice of Nd sampling strategy. The biggest difference appears over land, where BR17 produces a more positive sensitivity when compared to other methods.
The variations in sensitivity and its spatial pattern produce around a 20 % variation in the implied RFaci (Fig. 9, lower-right corners), with larger RFaci values implied when using the BR17 and Z18 strategies. The smaller impact of Nd uncertainties on the RFaci (compared to aerosol uncertainties) is expected, as Nd is the independent variable in the βN calculation. As such, the correlation between satellite Nd and true Nd does not strongly affect the value of βN inferred from linear regression for reasonable sample sizes (e.g. larger than a few dozen).
For a simple linear regression calculation, only deviations from a linear relationship between the observed and actual Nd affect the calculated βN. Biases in Nd that scale with true Nd do not affect inferred βN because of the power law relationship assumed in the regression. Examining the correspondence between aircraft Nd and satellite Nd in Figs. 3 and 5 supports a linear relationship with zero intercept, even in cases where they do not fall along the 1:1 line. Thus the Nd calculation methods examined here appear to be all be of sufficient accuracy to produce accurate estimates of βN. However, bi-variate methods for calculating βN (e.g. Pitkänen et al., 2016) are more sensitive to the estimates of uncertainty in the Nd retrieval and would have a different error profile. In addition, as Nd is the independent variable in many calculations of cloud adjustments, the uncertainty here still has a critical role to play in the calculation of the ERFaci.
Figure 9 demonstrates that although the aerosol proxy is still the major source of uncertainty in observation-based estimates of the RFaci and ERFaci, the Nd sampling strategy is a non-negligible source of uncertainty because it affects the aerosol proxy data considered and thus sampled deviations between aerosol proxy and actual CCN. It is not clear which of these sampling strategies provides the best estimate of the RFaci. Although BR17 is the most accurate at a pixel level (Table 2), it is based on a subset of cases which may not be representative of the overall climatology (Fig. 6). Further studies will be necessary to understand the impact of this potential selection bias.
Nd is an important property of clouds, both for assessing cloud models and for constraining aerosol–cloud interactions. However, its retrieval is based on a number of assumptions of varying validity. In addition, it is derived from retrievals of τc and re (Eq. 1) that are themselves uncertain, inheriting potential biases from these retrievals. In recent years, a number of sampling strategies have been suggested (Table 1) to select cases where the assumptions are more likely to be valid and the retrievals less likely to be biased. This work investigates these assumptions and their impact on the implied radiative forcing.
At a pixel level (1 km), the satellite Nd (from MODIS) and in situ Nd are well correlated (Fig. 3). This is especially true in stratocumulus regimes (r2 in the range 0.5 to 0.8, Table 2), where high-cloud fractions and adiabatic clouds are more common. Even in more challenging cumulus and convective situations, the MODIS Nd retrieval can provide useful information about Nd, although correlations are significantly lower. These correlations are lower than previous studies (Painemal and Zuidema, 2011; Kang et al., 2021), but the demands placed on the retrieval in this work are much tougher, requiring the satellite sampling strategy to identify accurate retrievals, with no additional data from in situ measurements.
The different sampling strategies have varying strengths and weaknesses. BR17 has the strongest correlation to in situ Nd across a range of aircraft campaigns but has the lowest coverage of any of the strategies investigated (Fig. 8). While Z18 has a lower accuracy than other strategies, it has a higher correlation to in situ Nd in locations of a high CF. It is important to note that the BR17 and Z18 strategies in this work are applied on top of G18 (Table 1), differing from their original application in Bennartz and Rausch (2017) and Zhu et al. (2018). BR17 and Z18 both benefit from the identification of uncertain retrievals provided by G18.
The RMSD, normalised by the mean Nd for each of the sampling strategies, is around 30 %–50 % (Table 2). This is significantly smaller than the 78 % uncertainty calculated in Grosvenor et al. (2018b), partly due to the focus more on stratocumulus cases in this work and partly due to the success of the sampling strategies in identifying and excluding biased Nd retrievals.
Potential improvements to the sampling strategies are demonstrated (Fig. 4), leading to a number of recommendations for the use of MODIS-derived Nd products in the future.
-
A high correlation between MODIS and in situ Nd is achieved even with minimal filtering. This can represent the variability in Nd better than the more selective sampling methods (Fig. 6).
-
BR17 appears to have the best correlation with aircraft data across a wide variety of conditions (Table 2) but may be biased high in broken-cloud conditions (Fig. 6).
-
Z18 has a lower skill for low-cloud fractions, but the accuracy increases for high-cloud fractions (likely due to the validity of the assumptions used; Fig. 4).
-
The 3.7 µm retrieval is a better match to in situ data in non-stratocumulus cases, consistent with studies looking at the effective radius retrieval. There may be a small advantage to using the 1.6 µm retrieval for 1∘ × 1∘ averages (Fig. 5). This may be due to cloud top entrainment effects, but given the known uncertainties in the 1.6 µm re retrieval (Zhang and Platnick, 2011), confidence in this result is low, and users should be cautious if they intend to employ the 1.6 µm Nd retrieval.
-
Across the campaigns, G18 has the closest match to the climatology (Fig. 6). However, the difference between sampling strategies in stratocumulus regions is small, and the lack of satellite–in situ coincidences in non-stratocumulus regimes reduces confidence in this result in these locations.
The correlation between in situ and satellite Nd increases further when considering 1∘ × 1∘ averages, with r2 values of almost 0.9 for the BR17 sampling strategy (Fig. 5). However, the uncertainty in these correlations remains high due to the small number of data points and the high representation errors for aircraft measurements of a 1∘ × 1∘ region.
Even with the different climatologies produced by the sampling strategies (Fig. 7), the susceptibility of Nd to aerosol proxies remains remarkably similar (Fig. 9). The similarity is closest in stratocumulus regions, resulting in Nd sampling generating only a 20 % variation in the implied forcing. The impact of the aerosol proxy on the estimated RFaci remains the largest uncertainty, although Nd sampling produces an uncertainty of around 20 %.
The apparent close agreement between MODIS and in situ Nd masks a number of uncertainties. While Nd measurements in stratocumulus regions agree well, there is significant diversity in the Nd estimates in non-stratocumulus cases. While these are less important for the RFaci (Gryspeerdt and Stier, 2012), they may be critical for the forcing from cloud adjustments (e.g. Koren et al., 2014), and observations of Nd in these regions are essential for constraining the magnitude of these adjustments (Gryspeerdt et al., 2016). Additionally, biases in Nd may be correlated to biases in other cloud properties (such as the LWP). Understanding and reducing these systematic biases is beyond the scope of this work but vital to make progress in observationally constraining aerosol–cloud interactions.
While significant uncertainties remain, this work has demonstrated that the MODIS Nd retrieval has skill in retrieving Nd in a variety of different cloud regimes. There is a close match between not only in situ and satellite data at a pixel level but also the in situ and satellite Nd climatologies, with a sufficient accuracy for addressing a wide range of questions in cloud and aerosol–cloud physics at the global scale.
The Nd data created for this work are available at the Centre for Environmental Data Analysis at https://doi.org/10.5285/864a46cc65054008857ee5bb772a2a2b (Gryspeerdt et al., 2022). Data from the Twin Otter campaigns are available at https://figshare.com/articles/dataset/A_Multi-Year_Data_Set_on_Aerosol-Cloud-Precipitation-Meteorology_Interactions_for_Marine_Stratocumulus_Clouds/5099983 (last access: 21 June 2022; Sorooshian et al., 2022). ACTIVATE and NAAMES data are available from the Langley Atmospheric Research Center https://www-air.larc.nasa.gov/missions.htm (last access: 21 June 2022; NASA, 2022). VOCALS and SOCRATES data are available from the University Corporation for Atmospheric Research (UCAR) at https://www.eol.ucar.edu/all-field-projects-and-deployments (last access: 21 June 2022; EOL, 2022). COPE data are available from the National Centre for Atmospheric Science (NCAS) British Atmospheric Data Centre (BADC) at http://catalogue.ceda.ac.uk/uuid/8440933238f72f27762005c33d2aa278 (last access: 21 June 2022; CEDA Archive, 2022).
The supplement related to this article is available online at: https://doi.org/10.5194/amt-15-3875-2022-supplement.
EG and DTM designed the study, EG performed the analysis, all the authors assisted in the interpretation of the results and commented on the manuscript.
The contact author has declared that neither they nor their co-authors have any competing interests.
Publisher’s note: Copernicus Publications remains neutral with regard to jurisdictional claims in published maps and institutional affiliations.
The authors thank the VOCALS and SOCRATES teams for their work collecting the data used in this work. Daniel T. McCoy acknowledges the support of the University of Wyoming. The authors would also like to thank the two referees, whose comments helped significantly improve the paper.
This research has been supported by the Royal Society (grant no. URF/R1/191602), the Office of Naval Research (grant nos. N00014-20-1-2385, N00014-04-1-0118, N00014-10-1-0200, N00014-11-1-0783, N00014-10-1-0811, N00014-16-1-2567 and N00014-04-1-0018), and the Earth Sciences Division of the National Aeronautics and Space Administration (grant nos. 80NSSC19K0442, 80NSSC21K2014).
This paper was edited by Hang Su and reviewed by two anonymous referees.
Ackerman, A. S., Kirkpatrick, M. P., Stevens, D. E., and Toon, O. B.: The impact of humidity above stratiform clouds on indirect aerosol climate forcing, Nature, 432, 1014, https://doi.org/10.1038/nature03174, 2004. a
Albrecht, B. A.: Aerosols, Cloud Microphysics, and Fractional Cloudiness, Science, 245, 1227–1230, https://doi.org/10.1126/science.245.4923.1227, 1989. a
Austin, R. T. and Stephens, G. L.: Retrieval of stratus cloud microphysical parameters using millimeter-wave radar and visible optical depth in preparation for CloudSat: 1. Algorithm formulation, J. Geophys. Res., 106, 28233–28242, https://doi.org/10.1029/2000JD000293, 2001. a
Baker, M. B., Corbin, R. G., and Latham, J.: The influence of entrainment on the evolution of cloud droplet spectra: I. A model of inhomogeneous mixing, Q. J. Roy. Meteor. Soc., 106, 581–598, https://doi.org/10.1002/qj.49710644914, 1980. a, b
Behrenfeld, M. J., Moore, R. H., Hostetler, C. A., Graff, J., Gaube, P., Russell, L. M., Chen, G., Doney, S. C., Giovannoni, S., Liu, H., Proctor, C., Bolaños, L. M., Baetge, N., Davie-Martin, C., Westberry, T. K., Bates, T. S., Bell, T. G., Bidle, K. D., Boss, E. S., Brooks, S. D., Cairns, B., Carlson, C., Halsey, K., Harvey, E. L., Hu, C., Karp-Boss, L., Kleb, M., Menden-Deuer, S., Morison, F., Quinn, P. K., Scarino, A. J., Anderson, B., Chowdhary, J., Crosbie, E., Ferrare, R., Hair, J. W., Hu, Y., Janz, S., Redemann, J., Saltzman, E., Shook, M., Siegel, D. A., Wisthaler, A., Martin, M. Y., and Ziemba, L.: The North Atlantic Aerosol and Marine Ecosystem Study (NAAMES): Science Motive and Mission Overview, Front. Marine Sci., 6, 122, https://doi.org/10.3389/fmars.2019.00122, 2019. a
Bellouin, N., Quaas, J., Gryspeerdt, E., Kinne, S., Stier, P., Watson‐Parris, D., Boucher, O., Carslaw, K., Christensen, M., Daniau, A., Dufresne, J., Feingold, G., Fiedler, S., Forster, P., Gettelman, A., Haywood, J., Lohmann, U., Malavelle, F., Mauritsen, T., McCoy, D., Myhre, G., Mülmenstädt, J., Neubauer, D., Possner, A., Rugenstein, M., Sato, Y., Schulz, M., Schwartz, S., Sourdeval, O., Storelvmo, T., Toll, V., Winker, D., and Stevens, B.: Bounding global aerosol radiative forcing of climate change, Rev. Geophys., 17, e2019RG000660, https://doi.org/10.1029/2019RG000660, 2020. a
Bennartz, R. and Rausch, J.: Global and regional estimates of warm cloud droplet number concentration based on 13 years of AQUA-MODIS observations, Atmos. Chem. Phys., 17, 9815–9836, https://doi.org/10.5194/acp-17-9815-2017, 2017. a, b, c, d
Boers, R., Acarreta, J. R., and Gras, J. L.: Satellite monitoring of the first indirect aerosol effect: Retrieval of the droplet concentration of water clouds, J. Geophys. Res., 111, D22208, https://doi.org/10.1029/2005JD006838, 2006. a, b, c
Boucher, O., Randall, D. A., Artaxo, P., Bretherton, C., Feingold, G., Forster, P. M., Kerminen, V.-M., Kondo, Y., Liao, H., Lohmann, U., Rasch, P., Satheesh, S. K., Sherwood, S., Stevens, B., and Zhang, X. Y.: Clouds and Aerosols, Cambridge University Press, https://doi.org/10.1017/CBO9781107415324.016, 2013. a
Braun, R. A., Dadashazar, H., MacDonald, A. B., Crosbie, E., Jonsson, H. H., Woods, R. K., Flagan, R. C., Seinfeld, J. H., and Sorooshian, A.: Cloud Adiabaticity and Its Relationship to Marine Stratocumulus Characteristics Over the Northeast Pacific Ocean, J. Geophys. Res., 123, 24, https://doi.org/10.1029/2018JD029287, 2018. a
Brenguier, J.-L., Pawlowska, H., Schüller, L., Preusker, R., Fischer, J., and Fouquart, Y.: Radiative Properties of Boundary Layer Clouds: Droplet Effective Radius versus Number Concentration., J. Atmos. Sci., 57, 803, https://doi.org/10.1175/1520-0469(2000)057<0803:RPOBLC>2.0.CO;2, 2000. a
CEDA Archive: MICROphysicS of COnvective PrEcipitation (MICROSCOPE) project: In-situ airborne atmospheric and ground-based radar measurements, CEDA Archive [data set], http://catalogue.ceda.ac.uk/uuid/8440933238f72f27762005c33d2aa278, last access: 21 June 2022. a
EOL: All Field Projects and Deployments, EOL [data set], https://www.eol.ucar.edu/all-field-projects-and-deployments, last access: 21 June 2022. a
Eytan, E., Koren, I., Altaratz, O., Pinsky, M., and Khain, A.: Revisiting adiabatic fraction estimations in cumulus clouds: high-resolution simulations with a passive tracer, Atmos. Chem. Phys., 21, 16203–16217, https://doi.org/10.5194/acp-21-16203-2021, 2021. a
Feingold, G.: First measurements of the Twomey indirect effect using ground-based remote sensors, Geophys. Res. Lett., 30, 1287, https://doi.org/10.1029/2002GL016633, 2003. a
George, R. C. and Wood, R.: Subseasonal variability of low cloud radiative properties over the southeast Pacific Ocean, Atmos. Chem. Phys., 10, 4047–4063, https://doi.org/10.5194/acp-10-4047-2010, 2010. a
Ghan, S., Wang, M., Zhang, S., Ferrachat, S., Gettelman, A., Griesfeller, J., Kipling, Z., Lohmann, U., Morrison, H., Neubauer, D., Partridge, D. G., Stier, P., Takemura, T., Wang, H., and Zhang, K.: Challenges in constraining anthropogenic aerosol effects on cloud radiative forcing using present-day spatiotemporal variability, P. Natl. Acad. Sci. USA, 113, 5804–5811, https://doi.org/10.1073/pnas.1514036113, 2016. a
Grosvenor, D. P. and Carslaw, K. S.: The decomposition of cloud–aerosol forcing in the UK Earth System Model (UKESM1), Atmos. Chem. Phys., 20, 15681–15724, https://doi.org/10.5194/acp-20-15681-2020, 2020. a
Grosvenor, D. P. and Wood, R.: The effect of solar zenith angle on MODIS cloud optical and microphysical retrievals within marine liquid water clouds, Atmos. Chem. Phys., 14, 7291–7321, https://doi.org/10.5194/acp-14-7291-2014, 2014. a, b, c
Grosvenor, D. P., Sourdeval, O., and Wood, R.: Parameterizing cloud top effective radii from satellite retrieved values, accounting for vertical photon transport: quantification and correction of the resulting bias in droplet concentration and liquid water path retrievals, Atmos. Meas. Tech., 11, 4273–4289, https://doi.org/10.5194/amt-11-4273-2018, 2018a. a, b, c
Grosvenor, D. P., Sourdeval, O., Zuidema, P., Ackerman, A., Alexandrov, M. D., Bennartz, R., Boers, R., Cairns, B., Chiu, J. C., Christensen, M., Deneke, H., Diamond, M., Feingold, G., Fridlind, A., Hünerbein, A., Knist, C., Kollias, P., Marshak, A., McCoy, D., Merk, D., Painemal, D., Rausch, J., Rosenfeld, D., Russchenberg, H., Seifert, P., Sinclair, K., Stier, P., van Diedenhoven, B., Wendisch, M., Werner, F., Wood, R., Zhang, Z., and Quaas, J.: Remote Sensing of Droplet Number Concentration in Warm Clouds: A Review of the Current State of Knowledge and Perspectives, Rev. Geophys., 56, 409–453, https://doi.org/10.1029/2017RG000593, 2018b. a, b, c, d
Gryspeerdt, E. and Stier, P.: Regime-based analysis of aerosol-cloud interactions, Geophys. Res. Lett., 39, 21802, https://doi.org/10.1029/2012GL053221, 2012. a
Gryspeerdt, E., Quaas, J., and Bellouin, N.: Constraining the aerosol influence on cloud fraction, J. Geophys. Res., 121, 3566–3583, https://doi.org/10.1002/2015JD023744, 2016. a, b, c
Gryspeerdt, E., Quaas, J., Ferrachat, S., Gettelman, A., Ghan, S., Lohmann, U., Morrison, H., Neubauer, D., Partridge, D. G., Stier, P., Takemura, T., Wang, H., Wang, M., and Zhang, K.: Constraining the instantaneous aerosol influence on cloud albedo, P. Natl. Acad. Sci. USA, 114, 4899–4904, https://doi.org/10.1073/pnas.1617765114, 2017. a, b, c
Gryspeerdt, E., Goren, T., Sourdeval, O., Quaas, J., Mülmenstädt, J., Dipu, S., Unglaub, C., Gettelman, A., and Christensen, M.: Constraining the aerosol influence on cloud liquid water path, Atmos. Chem. Phys., 19, 5331–5347, https://doi.org/10.5194/acp-19-5331-2019, 2019. a
Gryspeerdt, E., McCoy, D., Crosbie, E., Moore, R. H., Nott, G. J., Painemal, D., Small-Griswold, J., Sorooshian, A., and Ziemba, L.: Cloud droplet number concentration, calculated from the MODIS (Moderate resolution imaging spectroradiometer) cloud optical properties retrieval and gridded using different sampling strategies, CEDA Archive [data set], https://doi.org/10.5285/864a46cc65054008857ee5bb772a2a2b, 2022. a
Han, Q., Rossow, W. B., Zeng, J., and Welch, R.: Three Different Behaviors of Liquid Water Path of Water Clouds in Aerosol–Cloud Interactions, J. Atmos. Sci., 59, 726–735, https://doi.org/10.1175/1520-0469(2002)059<0726:TDBOLW>2.0.CO;2, 2002. a
Hasekamp, O. P., Gryspeerdt, E., and Quaas, J.: Analysis of polarimetric satellite measurements suggests stronger cooling due to aerosol-cloud interactions, Nat. Commun., 10, 5405, https://doi.org/10.1038/s41467-019-13372-2, 2019. a, b
Hu, Y., Lu, X., Zhai, P.-W., Hostetler, C. A., Hair, J. W., Cairns, B., Sun, W., Stamnes, S., Omar, A., Baize, R., Videen, G., Mace, J., McCoy, D. T., McCoy, I. L., and Wood, R.: Liquid Phase Cloud Microphysical Property Estimates From CALIPSO Measurements, Front. Rem. Sens., 2, 724615, https://doi.org/10.3389/frsen.2021.724615, 2021. a
Kang, L., Marchand, R., and Smith, W.: Evaluation of MODIS and Himawari‐8 Low Clouds Retrievals Over the Southern Ocean With In Situ Measurements From the SOCRATES Campaign, Earth Space Sci., 8, e2020EA001397, https://doi.org/10.1029/2020EA001397, 2021. a, b, c, d
Khairoutdinov, M. and Kogan, Y.: A New Cloud Physics Parameterization in a Large-Eddy Simulation Model of Marine Stratocumulus, Mon. Weather Rev., 128, 229–243, https://doi.org/10.1175/1520-0493(2000)128<0229:ANCPPI>2.0.CO;2, 2000. a
Koren, I., Dagan, G., and Altaratz, O.: From aerosol-limited to invigoration of warm convective clouds, Science, 344, 1143–1146, https://doi.org/10.1126/science.1252595, 2014. a
Leahy, L. V., Wood, R., Charlson, R. J., Hostetler, C. A., Rogers, R. R., Vaughan, M. A., and Winker, D. M.: On the nature and extent of optically thin marine low clouds, J. Geophys. Res., 117, D22201, https://doi.org/10.1029/2012JD017929, 2012. a
Leon, D., French, J. R., Lasher-Trapp, S., Blyth, A. M., Abel, S. J., Ballard, S., Barrett, A., Bennett, L. J., Bower, K., Brooks, B., Brown, P., Charlton-Perez, C., Choularton, T., Clark, P., Collier, C., Crosier, J., Cui, Z., Dey, S., Dufton, D., Eagle, C., Flynn, M. J., Gallagher, M., Halliwell, C., Hanley, K., Hawkness-Smith, L., Huang, Y., Kelly, G., Kitchen, M., Korolev, A., Lean, H., Liu, Z., Marsham, J., Moser, D., Nicol, J., Norton, E. G., Plummer, D., Price, J., Ricketts, H., Roberts, N., Rosenberg, P. D., Simonin, D., Taylor, J. W., Warren, R., Williams, P. I., and Young, G.: The Convective Precipitation Experiment (COPE): Investigating the Origins of Heavy Precipitation in the Southwestern United Kingdom, B. Am. Meteorol. Soc., 97, 1003–1020, https://doi.org/10.1175/BAMS-D-14-00157.1, 2016. a
Levy, R. C., Mattoo, S., Munchak, L. A., Remer, L. A., Sayer, A. M., Patadia, F., and Hsu, N. C.: The Collection 6 MODIS aerosol products over land and ocean, Atmos. Meas. Tech., 6, 2989–3034, https://doi.org/10.5194/amt-6-2989-2013, 2013. a
Liang, L., Di Girolamo, L., and Platnick, S.: View-angle consistency in reflectance, optical thickness and spherical albedo of marine water-clouds over the northeastern Pacific through MISR-MODIS fusion, Geophys. Res. Lett., 36, L09811, https://doi.org/10.1029/2008GL037124, 2009. a
Maddux, B. C., Ackerman, S. A., and Platnick, S.: Viewing Geometry Dependencies in MODIS Cloud Products, J. Atmos. Ocean. Technol., 27, 1519–1528, https://doi.org/10.1175/2010JTECHA1432.1, 2010. a
Martin, G. M., Johnson, D. W., and Spice, A.: The Measurement and Parameterization of Effective Radius of Droplets in Warm Stratocumulus Clouds., J. Atmos. Sci., 51, 1823, https://doi.org/10.1175/1520-0469(1994)051<1823:TMAPOE>2.0.CO;2, 1994. a
McCoy, D. T., Bender, F. A.-M., Mohrmann, J. K. C., Hartmann, D. L., Wood, R., and Grosvenor, D. P.: The global aerosol-cloud first indirect effect estimated using MODIS, MERRA, and AeroCom, J. Geophys. Res., 122, 1779–1796, https://doi.org/10.1002/2016JD026141, 2017. a, b, c
McCoy, I. L., McCoy, D. T., Wood, R., Regayre, L., Watson-Parris, D., Grosvenor, D. P., Mulcahy, J. P., Hu, Y., Bender, F. A.-M., Field, P. R., Carslaw, K. S., and Gordon, H.: The hemispheric contrast in cloud microphysical properties constrains aerosol forcing, P. Natl. Acad. Sci. USA, 117, 18998–19006, https://doi.org/10.1073/pnas.1922502117, 2020. a, b, c
McFarquhar, G. M., Bretherton, C. S., Marchand, R., Protat, A., DeMott, P. J., Alexander, S. P., Roberts, G. C., Twohy, C. H., Toohey, D., Siems, S., Huang, Y., Wood, R., Rauber, R. M., Lasher-Trapp, S., Jensen, J., Stith, J. L., Mace, J., Um, J., Järvinen, E., Schnaiter, M., Gettelman, A., Sanchez, K. J., McCluskey, C. S., Russell, L. M., McCoy, I. L., Atlas, R. L., Bardeen, C. G., Moore, K. A., Hill, T. C. J., Humphries, R. S., Keywood, M. D., Ristovski, Z., Cravigan, L., Schofield, R., Fairall, C., Mallet, M. D., Kreidenweis, S. M., Rainwater, B., D’Alessandro, J., Wang, Y., Wu, W., Saliba, G., Levin, E. J. T., Ding, S., Lang, F., Truong, S. C. H., Wolff, C., Haggerty, J., Harvey, M. J., Klekociuk, A. R., and McDonald, A.: Observations of Clouds, Aerosols, Precipitation, and Surface Radiation over the Southern Ocean: An Overview of CAPRICORN, MARCUS, MICRE, and SOCRATES, B. Am. Meteorol. Soc., 102, E894–E928, https://doi.org/10.1175/BAMS-D-20-0132.1, 2021. a
Merk, D., Deneke, H., Pospichal, B., and Seifert, P.: Investigation of the adiabatic assumption for estimating cloud micro- and macrophysical properties from satellite and ground observations, Atmos. Chem. Phys., 16, 933–952, https://doi.org/10.5194/acp-16-933-2016, 2016. a
Mulcahy, J. P., Jones, C., Sellar, A., Johnson, B., Boutle, I. A., Jones, A., Andrews, T., Rumbold, S. T., Mollard, J., Bellouin, N., Johnson, C. E., Williams, K. D., Grosvenor, D. P., and McCoy, D. T.: Improved Aerosol Processes and Effective Radiative Forcing in HadGEM3 and UKESM1, J. Adv. Model. Earth Sy., 10, 2786–2805, https://doi.org/10.1029/2018MS001464, 2018. a, b
Nakajima, T. and King, M. D.: Determination of the Optical Thickness and Effective Particle Radius of Clouds from Reflected Solar Radiation Measurements. Part I: Theory, J. Atmos. Sci., 47, 1878–1893, https://doi.org/10.1175/1520-0469(1990)047<1878:DOTOTA>2.0.CO;2, 1990. a, b
Nakajima, T., Higurashi, A., Kawamoto, K., and Penner, J. E.: A possible correlation between satellite-derived cloud and aerosol microphysical parameters, Geophys. Res. Lett., 28, 1171, https://doi.org/10.1029/2000GL012186, 2001. a
NASA: Airborne Science Data for Atmospheric Composition, NASA [data set], https://www-air.larc.nasa.gov/missions.htm, last access: 21 June 2022. a
Noone, K. J., Johnson, D. W., Taylor, J. P., Ferek, R. J., Garrett, T., Hobbs, P. V., Durkee, P. A., Nielsen, K., Öström, E., O'Dowd, C., Smith, M. H., Russell, L. M., Flagan, R. C., Seinfeld, J. H., de, B. L., van, G. R. E., Hudson, J. G., Brooks, I., Gasparovic, R. F., and Pockalny, R. A.: A Case Study of Ship Track Formation in a Polluted Marine Boundary Layer, J. Atmos. Sci., 57, 2748, https://doi.org/10.1175/1520-0469(2000)057<2748:ACSOST>2.0.CO;2, 2000. a
Painemal, D.: Global Estimates of Changes in Shortwave Low-Cloud Albedo and Fluxes Due to Variations in Cloud Droplet Number Concentration Derived From CERES-MODIS Satellite Sensors, Geophys. Res. Lett., 45, 9288–9296, https://doi.org/10.1029/2018GL078880, 2018. a
Painemal, D. and Zuidema, P.: Assessment of MODIS cloud effective radius and optical thickness retrievals over the Southeast Pacific with VOCALS-REx in situ measurements, J. Geophys. Res., 116, D24206, https://doi.org/10.1029/2011JD016155, 2011. a, b, c, d, e, f
Painemal, D. and Zuidema, P.: The first aerosol indirect effect quantified through airborne remote sensing during VOCALS-REx, Atmos. Chem. Phys., 13, 917–931, https://doi.org/10.5194/acp-13-917-2013, 2013. a, b
Painemal, D., Xu, K.-M., Palikonda, R., and Minnis, P.: Entrainment rate diurnal cycle in marine stratiform clouds estimated from geostationary satellite retrievals and a meteorological forecast model, Geophys. Res. Lett., 44, 7482–7489, https://doi.org/10.1002/2017GL074481, 2017. a
Painemal, D., Spangenberg, D., Smith Jr., W. L., Minnis, P., Cairns, B., Moore, R. H., Crosbie, E., Robinson, C., Thornhill, K. L., Winstead, E. L., and Ziemba, L.: Evaluation of satellite retrievals of liquid clouds from the GOES-13 imager and MODIS over the midlatitude North Atlantic during the NAAMES campaign, Atmos. Meas. Tech., 14, 6633–6646, https://doi.org/10.5194/amt-14-6633-2021, 2021. a
Pitkänen, M. R. A., Mikkonen, S., Lehtinen, K. E. J., Lipponen, A., and Arola, A.: Artificial bias typically neglected in comparisons of uncertain atmospheric data, Geophys. Res. Lett., 43, 10003–10011, https://doi.org/10.1002/2016GL070852, 2016. a
Platnick, S.: Vertical photon transport in cloud remote sensing problems, J. Geophys. Res., 105, 22919, https://doi.org/10.1029/2000JD900333, 2000. a, b
Platnick, S., Meyer, K. G., King, M. D., Wind, G., Amarasinghe, N., Marchant, B., Arnold, G. T., Zhang, Z., Hubanks, P. A., Holz, R. E., Yang, P., Ridgway, W. L., and Riedi, J.: The MODIS Cloud Optical and Microphysical Products: Collection 6 Updates and Examples From Terra and Aqua, IEEE T. Geosci. Remote, 55, 502–525, https://doi.org/10.1109/TGRS.2016.2610522, 2017. a
Quaas, J., Boucher, O., and Lohmann, U.: Constraining the total aerosol indirect effect in the LMDZ and ECHAM4 GCMs using MODIS satellite data, Atmos. Chem. Phys., 6, 947–955, https://doi.org/10.5194/acp-6-947-2006, 2006. a, b, c, d, e, f
Quaas, J., Boucher, O., Bellouin, N., and Kinne, S.: Satellite-based estimate of the direct and indirect aerosol climate forcing, J. Geophys. Res., 113, 05204, https://doi.org/10.1029/2007JD008962, 2008. a, b, c
Quaas, J., Ming, Y., Menon, S., Takemura, T., Wang, M., Penner, J. E., Gettelman, A., Lohmann, U., Bellouin, N., Boucher, O., Sayer, A. M., Thomas, G. E., McComiskey, A., Feingold, G., Hoose, C., Kristjánsson, J. E., Liu, X., Balkanski, Y., Donner, L. J., Ginoux, P. A., Stier, P., Grandey, B., Feichter, J., Sednev, I., Bauer, S. E., Koch, D., Grainger, R. G., Kirkevåg, A., Iversen, T., Seland, Ø., Easter, R., Ghan, S. J., Rasch, P. J., Morrison, H., Lamarque, J.-F., Iacono, M. J., Kinne, S., and Schulz, M.: Aerosol indirect effects – general circulation model intercomparison and evaluation with satellite data, Atmos. Chem. Phys., 9, 8697–8717, https://doi.org/10.5194/acp-9-8697-2009, 2009. a
Redemann, J., Wood, R., Zuidema, P., Doherty, S. J., Luna, B., LeBlanc, S. E., Diamond, M. S., Shinozuka, Y., Chang, I. Y., Ueyama, R., Pfister, L., Ryoo, J.-M., Dobracki, A. N., da Silva, A. M., Longo, K. M., Kacenelenbogen, M. S., Flynn, C. J., Pistone, K., Knox, N. M., Piketh, S. J., Haywood, J. M., Formenti, P., Mallet, M., Stier, P., Ackerman, A. S., Bauer, S. E., Fridlind, A. M., Carmichael, G. R., Saide, P. E., Ferrada, G. A., Howell, S. G., Freitag, S., Cairns, B., Holben, B. N., Knobelspiesse, K. D., Tanelli, S., L'Ecuyer, T. S., Dzambo, A. M., Sy, O. O., McFarquhar, G. M., Poellot, M. R., Gupta, S., O'Brien, J. R., Nenes, A., Kacarab, M., Wong, J. P. S., Small-Griswold, J. D., Thornhill, K. L., Noone, D., Podolske, J. R., Schmidt, K. S., Pilewskie, P., Chen, H., Cochrane, S. P., Sedlacek, A. J., Lang, T. J., Stith, E., Segal-Rozenhaimer, M., Ferrare, R. A., Burton, S. P., Hostetler, C. A., Diner, D. J., Seidel, F. C., Platnick, S. E., Myers, J. S., Meyer, K. G., Spangenberg, D. A., Maring, H., and Gao, L.: An overview of the ORACLES (ObseRvations of Aerosols above CLouds and their intEractionS) project: aerosol–cloud–radiation interactions in the southeast Atlantic basin, Atmos. Chem. Phys., 21, 1507–1563, https://doi.org/10.5194/acp-21-1507-2021, 2021. a
Robson, J., Aksenov, Y., Bracegirdle, T. J., Dimdore‐Miles, O., Griffiths, P. T., Grosvenor, D. P., Hodson, D. L. R., Keeble, J., MacIntosh, C., Megann, A., Osprey, S., Povey, A. C., Schröder, D., Yang, M., Archibald, A. T., Carslaw, K. S., Gray, L., Jones, C., Kerridge, B., Knappett, D., Kuhlbrodt, T., Russo, M., Sellar, A., Siddans, R., Sinha, B., Sutton, R., Walton, J., and Wilcox, L. J.: The Evaluation of the North Atlantic Climate System in UKESM1 Historical Simulations for CMIP6, J. Adv. Model. Earth Sy., 12, e2020MS002126, https://doi.org/10.1029/2020MS002126, 2020. a
Rosenfeld, D. and Gutman, G.: Retrieving microphysical properties near the tops of potential rain clouds by multispectral analysis of AVHRR data, Atmos. Res., 34, 259–283, https://doi.org/10.1016/0169-8095(94)90096-5, 1994. a
Sayer, A. M., Munchak, L. A., Hsu, N. C., Levy, R. C., Bettenhausen, C., and Jeong, M.-J.: MODIS Collection 6 aerosol products: Comparison between Aqua's e-Deep Blue, Dark Target, and “merged” data sets, and usage recommendations, J. Geophys. Res., 119, 13,965–13,989, https://doi.org/10.1002/2014JD022453, 2014. a
Sorooshian, A., MacDonald, A. B., Dadashazar, H., Bates, K. H., Coggon, M. M., Craven, J. S., Crosbie, E., Hersey, S. P., Hodas, N., Lin, J. J., Negrón Marty, A., Maudlin, L. C., Metcalf, A. R., Murphy, S. M., Padró, L. T., Prabhakar, G., Rissman, T. A., Shingler, T., Varutbangkul, V., Wang, Z., Woods, R. K., Chuang, P. Y., Nenes, A., Jonsson, H. H., Flagan, R. C., and Seinfeld, J. H.: A multi-year data set on aerosol-cloud-precipitation-meteorology interactions for marine stratocumulus clouds, Sci. Data, 5, 180026, https://doi.org/10.1038/sdata.2018.26, 2018. a
Sorooshian, A., Anderson, B., Bauer, S. E., Braun, R. A., Cairns, B., Crosbie, E., Dadashazar, H., Diskin, G., Ferrare, R., Flagan, R. C., Hair, J., Hostetler, C., Jonsson, H. H., Kleb, M. M., Liu, H., MacDonald, A. B., McComiskey, A., Moore, R., Painemal, D., Russell, L. M., Seinfeld, J. H., Shook, M., Smith, W. L., Thornhill, K., Tselioudis, G., Wang, H., Zeng, X., Zhang, B., Ziemba, L., and Zuidema, P.: Aerosol–Cloud–Meteorology Interaction Airborne Field Investigations: Using Lessons Learned from the U.S. West Coast in the Design of ACTIVATE off the U.S. East Coast, B. Am. Meteorol. Soc., 100, 1511–1528, https://doi.org/10.1175/BAMS-D-18-0100.1, 2019. a
Sorooshian, A., MacDonald, A. B., Dadashazar, H., Bates, K. H., Coggon, M. M., Craven, J. S., Crosbie, E., Edwards, E.-L., Hersey, S. P., Hodas, N., Lin, J. J., Mardi, A. H., Marty, A. N., Maudlin, L. C., Metcalf, A. R., Murphy, S. M., Padro, L. T., Prabhakar, G., Rissman, T. A., Schlosser, J., Shingler, T., Varutbangkul, V., Wang, Z., Woods, R. K., Chuang, P. Y., Nenes, A., Jonsson, H. H., Flagan, R. C., Seinfeld, J. H., and Stahl, C.: A Multi-Year Data Set on Aerosol-Cloud-Precipitation-Meteorology Interactions for Marine Stratocumulus Clouds, figshare [code], https://figshare.com/articles/dataset/A_Multi-Year_Data_Set_on_Aerosol-Cloud-Precipitation-Meteorology_Interactions_for_Marine_Stratocumulus_Clouds/5099983, last access: 21 June, 2022. a
Twomey, S.: The nuclei of natural cloud formation part II: The supersaturation in natural clouds and the variation of cloud droplet concentration, Geofis. Pura Appl., 43, 243–249, https://doi.org/10.1007/BF01993560, 1959. a
Twomey, S.: Pollution and the planetary albedo, Atmos. Environ., 8, 1251–1256, https://doi.org/10.1016/0004-6981(74)90004-3, 1974. a
vanZanten, M. C., Stevens, B., Vali, G., and Lenschow, D. H.: Observations of the Structure of Heavily Precipitating Marine Stratocumulus, J. Atmos. Sci., 62, 4327–4342, https://doi.org/10.1175/JAS3611.1, 2005. a
Wang, S., Wang, Q., and Feingold, G.: Turbulence, Condensation, and Liquid Water Transport in Numerically Simulated Nonprecipitating Stratocumulus Clouds, J. Atmos. Sci., 60, 262–278, https://doi.org/10.1175/1520-0469(2003)060<0262:TCALWT>2.0.CO;2, 2003. a
Williams, E., Rosenfeld, D., Madden, N., Gerlach, J., Gears, N., Atkinson, L., Dunnemann, N., Frostrom, G., Antonio, M., Biazon, B., Camargo, R., Franca, H., Gomes, A., Lima, M., Machado, R., Manhaes, S., Nachtigall, L., Piva, H., Quintiliano, W., Machado, L., Artaxo, P., Roberts, G., Renno, N., Blakeslee, R., Bailey, J., Boccippio, D., Betts, A., Wolff, D., Roy, B., Halverson, J., Rickenbach, T., Fuentes, J., and Avelino, E.: Contrasting convective regimes over the Amazon: Implications for cloud electrification, J. Geophys. Res., 107, 8082, https://doi.org/10.1029/2001JD000380, 2002. a
Wood, R.: Stratocumulus Clouds, Mon. Weather Rev., 140, 2373–2423, https://doi.org/10.1175/MWR-D-11-00121.1, 2012. a
Wood, R., Mechoso, C. R., Bretherton, C. S., Weller, R. A., Huebert, B., Straneo, F., Albrecht, B. A., Coe, H., Allen, G., Vaughan, G., Daum, P., Fairall, C., Chand, D., Gallardo Klenner, L., Garreaud, R., Grados, C., Covert, D. S., Bates, T. S., Krejci, R., Russell, L. M., de Szoeke, S., Brewer, A., Yuter, S. E., Springston, S. R., Chaigneau, A., Toniazzo, T., Minnis, P., Palikonda, R., Abel, S. J., Brown, W. O. J., Williams, S., Fochesatto, J., Brioude, J., and Bower, K. N.: The VAMOS Ocean-Cloud-Atmosphere-Land Study Regional Experiment (VOCALS-REx): goals, platforms, and field operations, Atmos. Chem. Phys., 11, 627–654, https://doi.org/10.5194/acp-11-627-2011, 2011. a
Zeng, S., Riedi, J., Trepte, C. R., Winker, D. M., and Hu, Y.-X.: Study of global cloud droplet number concentration with A-Train satellites, Atmos. Chem. Phys., 14, 7125–7134, https://doi.org/10.5194/acp-14-7125-2014, 2014. a
Zhang, Z. and Platnick, S.: An assessment of differences between cloud effective particle radius retrievals for marine water clouds from three MODIS spectral bands, J. Geophys. Res., 116, 20215, https://doi.org/10.1029/2011JD016216, 2011. a, b, c, d, e, f, g, h, i
Zhu, Y., Rosenfeld, D., and Li, Z.: Under What Conditions Can We Trust Retrieved Cloud Drop Concentrations in Broken Marine Stratocumulus?, J. Geophys. Res., 123, 8754–8767, https://doi.org/10.1029/2017JD028083, 2018. a, b, c, d