the Creative Commons Attribution 4.0 License.
the Creative Commons Attribution 4.0 License.
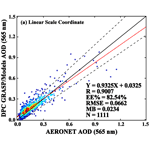
Performance evaluation for retrieving aerosol optical depth from the Directional Polarimetric Camera (DPC) based on the GRASP algorithm
Shikuan Jin
Yingying Ma
Cheng Chen
Oleg Dubovik
Jin Hong
Boming Liu
Wei Gong
Aerosol spatial distribution obtained from satellite sensors is critical for understanding regional aerosol environments, anthropogenic aerosol emissions, and global climate change. The Directional Polarimetric Camera (DPC) is the first generation of multi-angle polarized sensor developed by China. It is on-board the GaoFen-5 satellite, running in 705 km sun-synchronous orbit with a 13:30 LT (local time) ascending node. The sensor has three polarized channels at 490, 670, and 865 nm and ∼ 9 viewing angles, mainly used for observing aerosols. The spatial resolution is ∼ 3.3 km at nadir, and global coverage is ∼ 2 d. In this study, the performance of aerosol optical depth (AOD) retrievals from the DPC/GaoFen-5 using the Generalized Retrieval of Atmosphere and Surface Properties (GRASP) algorithm were evaluated on a global basis for the first time. The results showed that the DPC GRASP/Model scheme, which used several forms of aerosol-type mixing, achieved good performance. By comparing with Aerosol Robotic Network (AERONET) observations, the correlation coefficient (R), root-mean-square error (RMSE), and expected error (EE%, ± ()) were 0.9007 %, 0.0662 %, and 82.54 %, respectively. The scattering angle, number of averaged pixels, length of time steps, and radiative and polarized fitting residuals showed impacts on the results of AOD retrieval in the DPC GRASP/Model scheme. From the most AERONET sites, the R and EE% were larger than ∼ 0.9 % and ∼ 80%. Compared with Moderate resolution Imaging Spectroradiometer (MODIS) products, the spatial and temporal variations of aerosol could be caught by the DPC with the GRASP/Model scheme, showing a good performance. However, values of AOD were also underestimated by DPC, probably due to an overly strict cloud mask. The above findings validated the ability of the DPC sensor to monitor aerosols. It should contribute to the development of aerosol parameter retrieval from multi-angle polarized sensors in the future.
- Article
(7720 KB) - Full-text XML
- BibTeX
- EndNote
Aerosol is one of the most important components in the atmosphere. It influences the global radiation budget balance and climate directly by scattering and absorbing incoming solar radiation and indirectly by changing cloud microphysical properties (Albrecht, 1989; Rosenfeld et al., 2008). Due to their different emission sources and relatively transitory life cycle in the atmosphere, aerosol particles show large spatiotemporal variability and are difficult to describe uniformly on a global scale (Eck et al., 2010; Jin et al., 2019; Ma et al., 2021). This property can further affect atmospheric motions and the hydrological cycle and probably contributes to regional extreme weather events (Guo et al., 2016; Li et al., 2016; Nakajima et al., 2007; Shi et al., 2021). Therefore, the development of aerosol measurement technologies has received widespread attention in recent decades.
Satellite observation is the main approach to monitor and quantify aerosol distributions at a global scale (Kaufman et al., 1997). Traditional satellite technology relies on prior assumptions about the properties of the surface and atmosphere because the prerequisite for successful retrieval of aerosol is that the aerosol signal should be isolated from the remainder of the signal received by satellite, which includes the combined effect from molecule, aerosol, cloud, and the underlying surface (Lenoble et al., 2013). For instance, the appropriate spatial resolution helps when observing aerosol through clear holes in otherwise cloudy skies (Jin et al., 2021). The choice of spectral channel and bandwidth can avoid impact by gas absorption in narrow spectral bands known as atmosphere window regions. More importantly, the spectral channel should be set in a carefully selected band to avoid introducing uncertainty from underlying surface features, such as vegetation, bright desert, and ocean colour (Hsu et al., 2004; McCormick et al., 1979; Rao et al., 1989). Based on these principles, a series of aerosol products from different sensors have been released, and they greatly promote the developments of studies in aerosol-related fields, including aerosol climate effect, interaction of aerosol and cloud, air quality and public health, and global climate modelling (Gao et al., 2017; Liu et al., 2022; Sayer et al., 2013; Tegen and Lacis, 1996; Zhang et al., 2021).
With the progress of satellite technology, sensors with broader spectral range, multiple angles, and polarization observations have been applied to aerosol observations (Dubovik et al., 2019). POLDER-3 is the third sensor in the Polarization and Directionality of the Earth's Reflectance series, carried on the Polarization and Anisotropy of Reflectances for Atmospheric Science coupled with Observations from a Lidar (PARASOL) satellite, which was launched on 18 December 2004 as part of the A-Train constellation (Tanré et al., 2011). This instrument views (±51∘ along track and ±43∘ across track) Earth from ∼ 14 different angles by using a set of wide-field telecentric optics and a rotating filter wheel in nine spectral channels from 443 to 1020 nm (Deschamps et al., 1994). Among them, three channels at 490, 670, and 865 nm have polarization observation capabilities. POLDER-3 provides the longest multi-angle polarimetric observation record of the Earth atmosphere system in space to date, and the PARASOL mission was terminated in December 2013 due to limited on-board fuel. The Directional Polarimetric Camera (DPC) is the first Chinese multi-angle polarized Earth observation satellite sensor, on-board the fifth satellite (GaoFen-5) of the Chinese high-resolution Earth observation program (Li et al., 2018). It was launched successfully on 9 May 2018, with the goal of measuring aerosol parameters and providing information for the assessment of urban air pollution. The design of DPC is similar to the POLDER-3. It is equipped with five non-polarized bands at 443, 565, 763,765, and 910 nm and three polarized bands at 490, 670, and 865 nm, with a relatively high spatial resolution of 3.3 km, that can observe Earth from ∼ 9 different angles. Therefore, the DPC occupies an important position in the development of polarization instruments in China and is expected to provide beneficial information for atmospheric aerosol monitoring and satellite payload research.
The multi-angle polarized sensor can provide many more observations for the same pixel in an aerosol parameter retrieval. Compared to traditional spectral measurement, the multi-angle can help constrain bidirectional reflection functions, reducing uncertainty from the surface (Diner et al., 1998), while the polarized signal is mainly from atmospheric aerosol and sensitive to particle microphysical properties (Mishchenko and Travis, 1997). Generally, the polarized signal can be considered an independent source of information. A well-known advantage is that the polarized light from the surface accounts for a small part of the total polarized light compared with that from the particles and is mostly wavelength independent. In the algorithms for POLDER, the polarized signals at 670 and 865 nm are used for deriving the best aerosol model over the ocean and retrieving aerosol optical depth (AOD) over land due to the sensitivity to fine particles (Deuzé et al., 2001; Ge et al., 2020; Kacenelenbogen et al., 2006; Nadal and Bréon, 1999). In addition, the existence of the cloudbow effect in the polarized signal can also be used to recognize cloud mask and detect cloud structure (Breon and Colzy, 1999; Breon and Goloub, 1998; Li et al., 2021).
However, the algorithms that retrieve aerosol parameters from only one or two polarized channels struggle to obtain complex aerosol optical and microphysical parameters, such as aerosol size distribution and absorbing and scattering properties. To solve this problem, the Generalized Retrieval of Atmosphere and Surface Properties (GRASP) algorithm was developed, which provides a statistically optimized strategy that allows all aerosol-related measurement data from multi-angle polarized sensors to participate in the retrieval (Dubovik et al., 2014). It points out that the measured redundancy provided by multi-angle polarized sensor is considered to be positive and useful, especially when the number of observations is larger than the unknowns (Dubovik et al., 2011). At present, the GRASP algorithm has been successfully applied to a variety of sensors, including POLDER, lidar, and sun photometers, to retrieve complex aerosol parameters (Chen et al., 2020; Li et al., 2019; Lopatin et al., 2021). In this study, we retrieved AOD from DPC observations by using the GRASP algorithm and evaluated possible error-influencing factors. At the same time, by comparing Moderate resolution Imaging Spectroradiometer (MODIS) and Aerosol Robotic Network (AERONET) observations, the aerosol monitoring performance of DPC were verified in different spatial scales and timescales. This will partially lay the foundation for the retrieval of aerosol parameters from multi-angle polarized sensors in the future of China.
2.1 DPC data
The DPC is a multi-angle polarized sensor carried on the GF-5 satellite, which was launched in 9 May 2018. This sensor completes a scan of the entire Earth's surface about every 2 d from a sun-synchronous orbit and provides a swath of 1850 km with a spatial resolution of 3.3 km (Li et al., 2018). The DPC contains eight bands from 443 to 910 nm with a bandwidth of 10–40 nm that can observe Earth from ∼ 9 different angles at ∼ 13:30 LT (local time). Along with channels for water vapour (910 nm) and pressure (oxygen A band: 763 and 765 nm), five bands (443, 490, 565, 670, and 865 nm) are designed to measure aerosols (Li et al., 2018). The polarimetric capability at 490, 670, and 865 nm is realized by a polarized filter wheel (0, 60, and 120∘) and a step motor (Hagolle et al., 1999). The laboratory calibration uncertainties are 5 % for normalized radiation and 0.02 for degree of linear polarization (DOLP) (Li et al., 2021). An in-flight calibration study showed that the radiometric calibration error increased to ∼ 9 % at 865 nm and that the polarimetric calibration error increases to ∼ 0.04 at 490 and 670 nm after launch by applying Rayleigh and glint scenes, respectively, over ocean (Qie et al., 2021). Degradation of instrument performance over time may result in higher negative radiometric shift (Zhu et al., 2022). Thus, additional correction coefficients were also applied in this study to correct the image of the DPC observations from March to April 2020. The processing of DPC data is described in Sect. 3.2 in detail.
2.2 MODIS aerosol products
The Moderate resolution Imaging Spectroradiometer (MODIS) has been in service for over 2 decades, providing valuable Earth observations. The MODIS Level 2 C6.1 aerosol product (MxD04) is generated by using the Dark Target (DT) algorithm and the Deep Blue (DB) algorithm (Hsu et al., 2013; Levy et al., 2013). It provides multi-wavelength AOD data from each individual image with spatial resolutions of 3 and 10 km. The MODIS level 2 C6 aerosol product (MCD19A2) calculates aerosol parameters by using the Multi-Angle Implementation of Atmospheric Correction (MAIAC) algorithm from the continuous scenes of two satellites (Terra and Aqua) and considers temporal and spatial correlation of aerosols, with a spatial resolution of 1 km (Lyapustin et al., 2018). DT algorithm provides retrievals over ocean and land except for bright surfaces (such as desert dust), while the DB algorithm is only applied over land, and the MAIAC algorithm is used over land and part of the surrounding ocean. These MODIS aerosol products have been rigorously tested and verified and are widely used in aerosol-related studies (Che et al., 2019; Sayer et al., 2014; Zhdanova et al., 2020). In this study, the corrected AOD (quality flag = 3) on land and average AOD (quality flag = 1, 2, 3) on the ocean are selected from the DT products. The best estimated AOD (quality flag = 2, 3) is selected in the DB products. The best quality AOD (QA AOD = 0000) is selected in the MAIAC products.
2.3 AERONET observations
The Aerosol Robotic Network (AERONET) is a federation of ground-based remote sensing aerosol networks, established and expanded by various institutions from different countries (Holben et al., 1998). It has contributed continuous and long-term aerosol optical, microphysical, and radiative properties for more than 25 years in major ecosystems and human activity areas around the world. The AOD data used for validation were acquired from 178 AERONET sites with level 2.0 AOD products, which are cloud-screened and quality-controlled products. The uncertainties of AOD are less than 0.02 (Eck et al., 1999). In order to match the AERONET data to the satellite observations, a common approach is followed to average satellite data within ±30 min and a circle of 0.25∘ (∼ 25 km) radius centred at the selected site (Sayer et al., 2013). The relationship between multi-wavelength AOD proposed by Ångstrom (1964) was applied to calculate the AOD at corresponding wavelength of satellite bands from AERONET data.
3.1 Introduction of GRASP algorithm
GRASP is an open-source software package (https://www.grasp-open.com/, last access: 15 March 2020) for calculating and retrieving various optical and microphysical properties of aerosol and surface from observations of different remote sensing instruments, such as satellite, lidar, radiometer, and radiosonde (Dubovik et al., 2021). It was originally designed to solve the problem of aerosol retrieval from the PARASOL observations (Dubovik et al., 2014), but it has now become a scientifically rigorous and versatile algorithm based on generalization principles that works with diverse remote sensing applications in the community after continuous development (Dubovik et al., 2021). The GRASP algorithm contains two pivotal and independent modules. One is used to calculate the scattering, absorption, and extinction of light between different media from the physical level, simulating theoretical observational radiation signal, called the “Forward Model”. It allows for definition of various complex aerosol (size distribution, refractive index, sphere fraction, etc.) and surface properties (Bidirectional Reflectance/Polarization Distribution Function, BR/PDF, etc.) in the construction of the modelled reflectance. Therefore, it is possible to transform from optical observations to aerosol microphysical properties and estimate the surface parameters (Dubovik et al., 2011). The other module can be thought of as general mathematical operations without any particularly physical nature, called “Numerical Inversion”. It follows the statistically optimized strategy to fit observations under the fundamental frameworks of the maximum likelihood method and multi-term least-squares method (Dubovik and King, 2000). GRASP also realizes multiple-pixel retrieval, which constrains the variability of aerosol and surface optical properties in fitting process by an extra prior knowledge. Due to the consideration of the surrounding pixel information, the multi-pixel retrieval is more stable, and more importantly it can make up for the lack of aerosol reflection information in some cases, such as conditions that the signal from aerosol is much less than that from the surface (Dubovik et al., 2011). Based on the above advantages, the GRASP supports input measurements and parameters from different sources and levels, such as normalized and polarized radiance, vertical extinction and backscatter profile, and optical depth. This avoids the problem that the most popular look-up-table-based methods are difficult to apply to each other due to the limitations in different sensor channels and characteristics.
3.2 Pre-processing of DPC data
In order to partially offset the signal attenuation due to instrument ageing, before the pre-processing and retrieval. the radiance signals from the DPC were transferred and corrected to normalized radiative and polarized reflectance at top of the atmosphere, as described in Eq. (1).
The represents the radiative and polarized radiances received by the DPC in the form of the first three parts of the Stokes vector. and are the two additional correction coefficients. For I, this is applied following the results of Zhu et al. (2022), which depends on the view zenith angle (θ), and it is calculated based on Rayleigh scenes over the sea surface. For polarimetric signals, the additional correction coefficients can be referred to Qie et al. (2021). The E0 is the standard solar radiation flux, and is the corrected normalized signals at the top of the atmosphere of DPC.
In successful AOD retrieval, one of the key processes is to screen appropriate pixels. Cloudy pixels are the main factor impacting aerosol retrieval because they will block the signal from aerosol due to high reflectance, large coverage, and relatively high vertical position. Even very thin cirrus clouds and missed cloud edges can cause an positive error of ∼ 13 % in the visible channel (Koren et al., 2007). To remove cloud pixels in DPC images, we used several universal methods by considering cloud-sensitive characteristics in radiative and polarized bands:
-
The first step is to filter the image with a 3×3 sliding window at the blue (490 nm) and red (670 nm) bands for land and sea surfaces, respectively (Remer et al., 2012). If the standard deviation of a window is greater than 0.0025, then the centre pixel will be marked as a cloud pixel and removed (Martins et al., 2002). This method was initially applied to the MODIS image by considering the spatial variability of aerosol and cloud pixels. In addition, a threshold of > 0.4 in the green (565 nm) band is also used to detect cloud pixels after the filter process, in accordance with the DT algorithm. This threshold is to exclude very uniformly distributed cloud pixels in the central area of thick clouds, and some snow pixels and glint area will also be excluded at the same time.
-
In second step, a whiteness test was applied by using reflectance in visible bands. It uses the characteristic that clouds are white in the visible band, considering that pixel with the absolute value of relative deviations greater than 0.7 is cloud, as described in Eqs. (2)–(3).
Band1, Band2, and Band3 are reflectance in red, green, and blue bands received by satellite at top of the atmosphere, respectively. Corresponding to the DPC, they are 490, 565, and 670 nm, respectively. In the absence of infrared and thermal infrared information, it can supplementally remove any pixels that have flat reflectance, similar to some operators using reflectance ratio to detect clouds. This method was proposed by Gomez-Chova et al. (2007) for a Medium Resolution Imaging Spectrometer (MERIS) multispectral image, and it has also been considered in the well-known Fmask algorithm (Zhu and Woodcock, 2012).
-
The third step used polarized bands to remove cloud pixels, following the fact that cloud drops can show a relatively strong polarized reflectance by multiple scattering (cloudbow effect) under a specific observation geometry. This feature has been used to a generate cloud mask product for both POLDER and DPC sensors (Breon and Colzy, 1999; Li et al., 2021). When the scattering angle (SCA) is between 127 and 157∘, pixels with corrected polarized radiation at 865 nm larger than 0.03 and 0.05 for ocean and surface, respectively, are defined as cloud (Li et al., 2021). The relatively large SCA range is for a strict screening, given that the main peak of the polarized reflectance by cloud water droplets is ∼ 142∘ (Goloub and Deuze, 1994). In addition, any obvious noise is also removed in this step, such as the case of DOLP > 1.
3.3 Construction of a multi-pixel retrieval unit
Next, we will explain the necessary operations and settings of parameters to apply the GRASP algorithm to DPC data in detail. The GRASP algorithm can use the temporal and spatial continuity of pixels and allow a group of pixels to be inverted at the same time. The multi-pixel retrieval unit for DPC in the study is shown in Fig. 1. Each small cube represents a pixel in geographic grids with a spatial resolution of 0.1∘ ×0.1 ∘ (average of 3×3 DPC pixels). This is in accordance with the MODIS 04_L2 product (∼ 10 km). The projection is determined by the DPC data. Each pixel is guaranteed to have at least three different observation angles. The size of the retrieval unit can be arbitrarily selected but is limited by the hardware memory. Different colours show the percentage of land or sea and usually do not change with time. They need to be clearly defined in GRASP to select different surface reflectance models. Cloud and no-data pixels need to be removed before the retrieval because the cloud flag setting has not been implemented in the current version of code. Finally, this retrieval unit was processed using the GRASP algorithm to derive the AOD distributions.
3.4 Settings of retrieval parameters
The settings of initial value and spatial–temporal constraint can significantly impact results of the statistically optimized strategy in the GRASP algorithm (Dubovik et al., 2011). The GRASP allows several strategies to fit observations. For instance, the GRASP software gives two retrieval schemes for POLDER observations. The configurations of the two schemes differ only in settings of aerosol size distribution in the forward model. One fits the aerosol size distribution with 16 triangle bins from the range of 0.05 to 15.0 µm, while the other uses five lognormal bins at 0.1, 0.1732, 0.3, 1.0, and 2.9 µm based on pre-calculated optimized kernels of the POLDER-3. The scheme with five lognormal bins increases the speed by ∼ 9 times (2.5GHz CPU) without any graphical acceleration compared to the scheme with 16 triangle bins, and it has been used to generate the operational PARASOL/GRASP aerosol products (Chen et al., 2020). In addition, there is a scheme that is being tested called “GRASP/Model”. The GRASP/Model approach assumes an external mixture of several aerosol types with fixed optical parameters, which is a faster and more stable AOD retrieval, especially when aerosol loading is low (Chen et al., 2020).
A tolerable absolute error in radiative transfer calculations is set to 0.0005, and multiple scattering effects have been considered. The number of atmospheric layers is set to 10 with an exponential distribution. The input data of the GRASP algorithm were the normalized radiative measurements at 443, 490, 565, and 670 nm and the DOLP values of 490 and 670 nm. The initial guess of aerosol and surface properties are default in the GRASP software. They are applied to calculate AOD at a global scale. The Ross–Li model (Li and Strahler, 1992) and the Cox–Munk model (Cox and Munk, 1954) were used for modelling radiative (non-polarized) reflectance over land and ocean, respectively, while the surface polarized reflectance followed the method of Nadal and Bréon (1999). More details are documented in Dubovik et al. (2011). Among them, the complex refractive index and surface properties are generally allowed to be fit as wavelength-dependent parameters in iterations. All constraints on values are given a default sizeable range, such as the first parameter in the Ross–Li model being allowed to vary from 0.001 to 1.100. By using light-scattering calculations (Dubovik et al., 2006), all aerosol microphysical parameters are converted into optical parameters to participate in radiative simulation. Spatial and temporal constraints of variabilities of aerosol and surface properties are realized using the Lagrange multiplier method. More details can be referred to Dubovik et al. (2021). In this study, the GRASP/Model scheme was used to retrieve AOD from DPC. All calculations of the GRASP relied on the supercomputing system in the Supercomputing Center of Wuhan University.
4.1 Validation of DPC/GRASP with AERONET
As shown in Fig. 2, the AERONET observations were used as the references to estimate the performance of AOD retrieval from DPC images based on the GRASP algorithm. Linear regression, correlation coefficient (R), root-mean-square error (RMSE), mean bias (MB), percentage falling into expected error (EE%, .± ()), and matching number (N) were also calculated. Among these measurements, the EE% is selected in accordance with the MODIS error envelop, and the ideal EE% is ∼ 68 % under assumption of normal distribution within a sigma confidence interval. Overall, the DPC GRASP/Model AOD matches the AERONET observations with an R of 0.8511, an MB of 0.0256, and an RMSE of 0.0842, showing good performance without any quality control. Nearly 80 % of the GRASP/Model AOD retrievals fall within the EE% bounds, revealing that the error envelopment of DPC is probably narrower than that of MODIS. The slope of linear regression was 0.8686, which is less than 1. This means that the DPC/GRASP probably underestimates the AOD under heavy aerosol loading. More details are presented in Fig. 2c. It is found the lower slope of linear regression is mainly controlled by several points that have larger AOD (> 0.8). By contrast, the retrieval is stable when AOD is less than 0.8 .
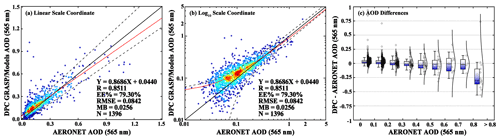
Figure 2(a) Density scatterplot of AOD retrievals from DPC with the GRASP/Model scheme versus the AERONET observations with a linear coordinate system. (b) The density scatterplot with a logarithmic coordinate system. The solid black line is the one to one value and the dashed black lines show the ranges of expected error. The solid red lines represent the linear regression line. (c) Box plots show changes in differences between DPC GRASP/Model and AERONET with AOD increasing. Diamonds and curves represent distributions of samples and normal distribution fitting lines, respectively.
In order to further study the retrieval performance of GRASP/Model and control the quality of the retrieval result from DPC data, we calculated the dependences of absolute MB with retrieval residuals, time steps (serial length), and average pixel (involved in retrieval) number in retrieval units and observation geometry, as shown in Fig. 3. The retrieval absolute MB showed an obvious increase when the SCA is larger than 150∘. Critical observation conditions, such as pixels at the edge of the image, will probably result to a larger error in both the satellite sensor and forward model. By contrast, using a different viewing angle number (3–11) has relatively little impact on the retrieval results, with the absolute MB per bin ranging between 0.0296 and 0.0595. With an increase in time step, the absolute MB showed a slightly decreasing trend, from 0.0543 to 0.0561. The same phenomenon was also found in the Fig. 3d. The absolute MB decreased from 0.0691 to 0.0435, with the number of averaged pixels increasing. This indicated that the fitting scheme for using the external mixing of different aerosol types in the GRASP/Model scheme showed positive dependence on the length of time steps and number of pixels. In addition, the spatial–temporal constraints in the retrieval are also affected by Lagrange multipliers, which can be customized in the configuration file.
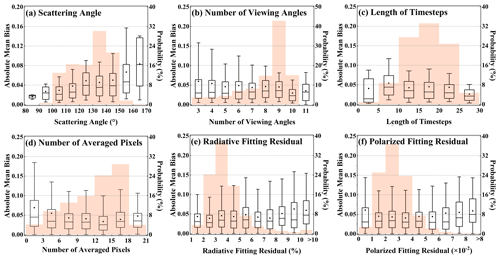
Figure 3Influencing factors of AOD retrieval performance of DPC based on the GRASP/Model scheme: (a) SCA, (b) number of viewing angles, (c) length of time steps, (d) number of averaged pixels, (e) non-polarized fitting residual, and (f) polarized fitting residual. Orange shadows in the background represent the probability distribution of the samples.
The fitting residual is an important factor for estimating the quality of retrieval in GRASP. It was found that the absolute MB showed a slight increase (from 0.0397 to 0.0596) when the radiative fitting residuals were larger than 8 %. The absolute MB had a trend to decrease first and then increase, with an increase in the polarized fitting residuals. Given that the DPC designed uncertainty is about 5 % for radiometric measurements and 0.02 for DOLP, the relatively large absolute MB (0.069) at 0.01 of the polarized fitting residuals is probably caused by the noise. To summarize, the SCA, number of averaged pixels, and fitting residuals showed the impacts on DPC GRASP/Model AOD retrieval in this test. A retrieval is considered low quality if any of the following conditions are met: (1) pixels with SCA > 150, (2) number of averaged pixels < 4, (3) length of time steps < 5, (4) non-polarized fitting residual >8 %, and (5) polarized fitting residual >0.06.
Figure 4a–b shows the scatterplots and density distributions of DPC/GRASP AOD versus the AERONET observations after quality control. About 20 % of the points were removed. It was found that the performance of AOD retrieval from DPC images showed an enhancement. For the DPC GRASP/Model scheme, the R increased from 0.8511 to 0.9001, the EE% increased from 79.30% to 82.54%, the RMSE decreased from 0.0842 to 0.0662, and the MB decreased from 0.0256 to 0.0234. Figure 4c displays the changes in differences between DPC and AERONET AOD. The underestimations when AOD > 0.8 were not found to be restrained by the quality control. A possible reason is that an overly restrictive cloud mask can remove aerosol pixels during heavy pollution. In addition, the negative drift after the launch of the DPC may also be the reason if it is not fully corrected.
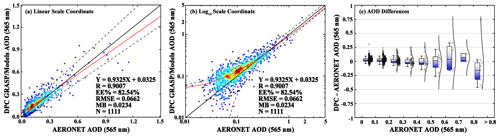
Figure 4(a) Density scatterplot of AOD retrievals from DPC with the GRASP/Model scheme versus the AERONET observations with a logarithmic coordinate system after quality control. (b) The density scatterplot with a logarithmic coordinate system. The solid black lines are diagonal and the dashed black lines show the ranges of expected error. The solid red lines represent the linear regression line. (c) Box plots show changes in differences between the DPC GRASP/Model scheme and AERONET with AOD increasing. Diamonds and curves represent the distributions of samples and normal distribution fitting lines, respectively.
4.2 Evaluation of DPC AOD performance at a spatial scale
The DPC AOD retrieved by the GRASP/Model scheme were compared with AERONET observations at each individual site to show a worldwide retrieval result as Fig. 5. The R, RMSE, MB, and EE% were calculated and displayed for sites where the matching number of pixels was larger than 5. In addition to the observation performance of the DPC itself, spatial variations in performances of AOD retrieval greatly depend on settings of initial parameter and constraint in the GRASP, whether or not they are in line with the local aerosol and surface environments. Results showed that the GRASP/Model scheme achieved great performance in different regions. The high values of R (> 0.8) were found in most regions, while the lower values (∼ 0.6) were mainly observed in North America. The values of RMSE at most sites were less than 0.08. These suggest that most values of AOD retrieval matched the AERONET AOD very well. At several sites, such as Asia and Africa, the RMSE was larger than 0.2, revealing that the AOD has a relatively large deviation calculated from DPC images based on current parameter setting with the GRASP algorithm in the regions. From the MB of Fig. 5c, the values of AOD were overestimated (∼ 0.04) in most areas. By contrast, the underestimations were found in high aerosol loading regions, such as South Asia and North Africa, and MB values were between −0.02 and −0.10, in accordance with the large underestimation when AOD > 0.8, as mentioned above. The EE% showed that over 80 % of AOD retrieved at sites can fall within the expected error range. It is worth noting that the parameterization in the GRASP/Model scheme is a globally consistent configuration in this study and does not consider the characteristics between different regions. This means that it is possible to achieve better results in local regions by adjusting different parameterizations.
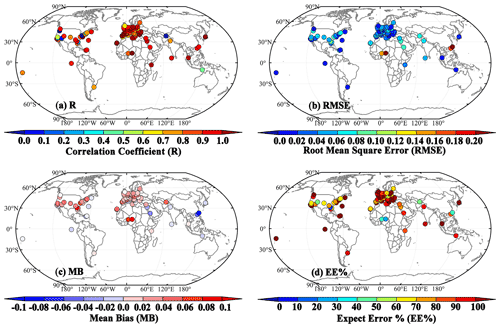
Figure 5Spatial distributions of (a) R, (b) RMSE, (c) MB, and (d) EE% calculated from the DPC GRASP/Model scheme compared with AERONET observations. Only sites with more than five matching points are included.
To further estimate the performance of DPC/GRASP AOD, two regions were selected as cases, as shown in Fig. 6. The MODIS MAIAC, DT, and DB aerosol products were used as comparisons. It was noted that the DB algorithm was only executed over land in the C6.1 MODIS DB aerosol products. It was found that the spatial coverage of GRASP/Models AOD from DPC over land was slightly lower than the MAIAC MODIS aerosol products. In addition to the narrower field of view and longer re-visit cycle of DPC (MODIS operated on two satellites: Terra and Aqua), the cloud mask method probably also misclassified the cloud-free pixels in heavy aerosol loading conditions. This also partially resulted in the underestimation of DPC AOD because the heavy aerosol loading pixels are removed. Nevertheless, DPC still properly captures the spatial distribution of AOD. The highest AOD values (> 1.0) in the southern part of China (mainly Guangdong and Guangxi) were caught by the current retrieval strategy. This is in accordance with the three MODIS products. By contrast, the AOD found in the North China Plain and central China by the DPC GRASP/Model scheme (∼ 0.5) were a little bit lower than MAIAC and DT products (∼ 0.6). However, the DT aerosol products showed higher AOD in this region (close to ∼ 1.0). This phenomenon is caused by unsuitable aerosol models, which further results in a persistent overestimation in the DT algorithm (Che et al., 2019). Using the additional radiometric and polarimetric correction, the DPC GRASP/Model scheme showed good performance over both land and ocean. The high values of AOD in the South China Sea and estuaries of the Yangtze River can be clearly captured. To summarize, the DPC showed the spatial ability of AOD retrieval based on the GRASP algorithm in the Chinese region, and similar results have also been reported recently by using the GRASP component module (Li et al., 2022).
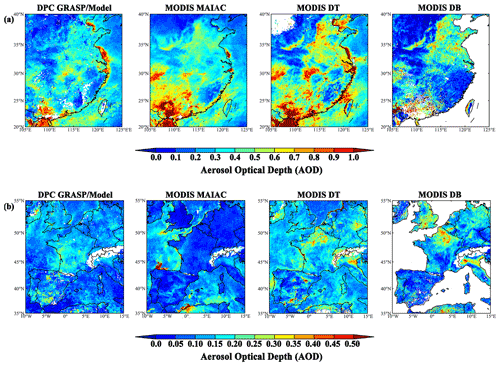
Figure 6Spatial distribution of AOD from the DPC GRASP/Model scheme compared with MODIS MAIAC, DT, and DB aerosol products in March 2020: (a) eastern and southern China with its adjacent sea areas, (b) areas of western Europe including the Atlantic Ocean and the Mediterranean Sea. The DPC AOD is at 565 nm, and the MODIS AOD is set at 550 nm. Publisher's note: Please note that the above figure contains disputed territories
Another case was selected in Western Europe where the air is clean and aerosol loading is low (<0.2) most of the year. As shown in Fig. 6b, different satellites and aerosol retrieval methods showed slightly different distributions of AOD. In addition to the different transit times between DPC and MODIS, this phenomenon is also probably because the aerosol signal is difficult to separate from the remainder of signal under low aerosol loading conditions and thus results in relative larger uncertainties of retrieval. From the AOD maps of DPC GRASP/Model values, relatively high values of AOD (∼ 0.25) were found in central France, southern Spain, and southern England. MODIS MAIAC showed lower AOD (∼ 0.1) over the mainland and two points of high AOD (∼ 0.5) northern coastal areas of Spain and Algeria. By contrast, the distributions of AOD calculated by the DT and DB algorithms were also different from those calculated by the DPC GRASP/Model scheme and MAIAC. The high AOD (∼ 0.4) region appeared in northern France, Italy, and southern England. Compared with single pixel-based retrieval algorithm (such as DT and DB), GRASP and MAIAC considered more temporal and spatial information from aerosol and surface parameters and benefit from the consistency of all assumptions (regarding aerosol and a priori constrains). The DPC GRASP exhibits minimal land–sea contrast. All of them have been proven to have good performance for AOD retrievals (Chen et al., 2020; Lyapustin et al., 2018; Ou et al., 2021; Sayer et al., 2014).
Figure 7 shows density distributions for differences between DPC and MODIS products in ranges of AOD ≤ 0.2, 0.2 < AOD ≤ 0.7, and AOD > 0.7. Corresponding to the Fig. 6, this is used to complement quantitative evaluations for the two regions. A common pattern is seen in all sub-plots, namely that the differences were nearly normally distributed and centred on the 0 under low aerosol loading conditions (AOD ≤ 0.2). With increasing AOD (AOD > 0.7), the differences showed an increasing negative bias, with the peak value varying from −0.5 to −1.0. The DPC GRASP/Model scheme underestimated AOD under heavy aerosol loading conditions, similar to the comparisons with AERONET. In follow-up studies, a more detailed investigation of this problem is required.
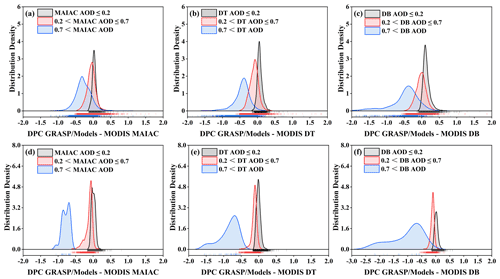
Figure 7Distribution density of AOD differences between the DPC GRASP/Model scheme and MODIS DT, DB, and MAIAC products for (a–c) eastern and southern China with its adjacent sea areas and (d–e) areas of western Europe including the Atlantic Ocean and the Mediterranean Sea. It is noted that the MODIS DB product only releases terrestrial AOD data.
4.3 Comparison of DPC AOD with MODIS products at a temporal scale
In this section, time series of AOD were evaluated against MODIS aerosol products based on the observations of AERONET site. The time series of daily RMSE is calculated for the global AERONET data set, as shown in Fig. 8. Lower values of RMSE mean smaller actual errors, indicating a good match with the AERONET. The AERONET stations had relatively continuous observations during the study period to avoid errors relating to how global validation statistics shift with the spatial distribution of observations (Gupta et al., 2018). As shown in Fig. 8, it was found that the time series of AOD from the DPC GRASP/Model scheme had a matched well with the AERONET AOD. The values of RMSE were ∼ 0.06 and stable before 87th day. The reason for the relatively large RMSE (∼ 0.12) around the 90th day is presumed to be heavy aerosol loading conditions, as the DPC GRASP/Model scheme would underestimate AOD in that kind of situation. The similar temporary rapid increases in RMSE were also found in MODIS products, such as the 80th day of the DT, the 85th day of the DB, and 98th day of the MAIAC. This reflects the time instability of algorithms. In addition, the lowest daily averaged RMSE was found in DPC GRASP/Model scheme, with a value of 0.0663, and then MODIS DT (0.0863) and MODIS DB (0.0913). The low RMSE of DPC may be due to it ignoring some high-value AODs. It is worth noting that the same parameter scheme (including start points and constraints) was applied globally in the GRASP/Model scheme. Therefore, the difference in aerosol optical properties and spatial–temporal heterogeneity in different regions ma not y be considered appropriately. The optimization of the region is expected to improve the retrieval effect, and further evaluation also requires the use of longer sequences of DPC data in the future.
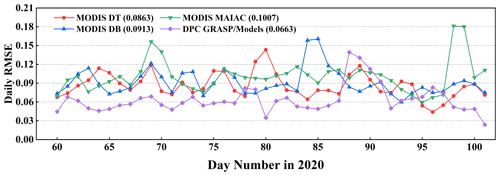
Figure 8Time series of daily RMSE for the selected AERONET stations during March and April of 2020. The numbers in brackets are averaged values of daily RMSE. The positions of AERONET stations are the same with Fig. 5.
Figure 9 shows three cases at different underlying surfaces to display the time series of AOD retrieved from the DPC GRASP/Model scheme on the basis of AERONET observations. The DT AOD was also compared as a reference due to its stable performance. It was found that the behaviour of AOD from DPC/GRASP and MODIS DT was generally consistent with AERONET at the three sites. From the scatterplots, the values of R were 0.947 and 0.949, 0.943 and 0.959, and 0.967 and 0.859 for MODIS DT and DPC GRASP/Models AOD at Raciborz, Magurele_Inoe, and FZJ-JOYCE, respectively. The GRASP/Model AOD values retrieved from DPC were slightly higher than the AERONET values at the FZJ-JOYCE site, resulting in a relatively low R. Nevertheless, in general, DPC/GRASP has a good ability to capture the temporal variation of aerosols.
DPC/GaoFen-5 is the first multi-angle polarized sensor launched by China and thus it occupies an important position in the development of satellite sensors. In this study, AOD was retrieved from the DPC images by using the GRASP algorithm and compared with AERONET and MODIS observations. The main purpose was to evaluate the performance of the DPC to monitor global aerosols.
On a global basis, a uniform parameterization scheme, which defined the variation ranges and start values of the optical and microphysical properties (realized by aerosol type) of the aerosol, was applied in the “Model” module of GRASP. Validations against AERONET showed that the R and EE% of the DPC GRASP/Model scheme were 0.8511 % and 79.30 %, respectively, in the first attempt. The SCA, number of averaged pixels in retrieval units, and fitting residual showed an impact on the results of AOD. A larger number of pixels in retrieval units and a smaller fitting residual can help improve the quality of retrieval. By quality control (i.e. removing pixels with SCA > 150, number of averaged pixels <4, length of time steps <5, non-polarized fitting residual > 8 %, polarized fitting residual > 0.06), the R and EE% of DPC GRASP/Model AOD improve to 0.9007 % and 82.54 %, respectively. The corresponding MB and RMSE decreased from 0.0256 and 0.0842 to 0.0234 and 0.0662, respectively. This indicated that DPC has a good ability to detect aerosols under this scheme.
At a spatial scale, the R and EE% of the GRASP/Model scheme were larger than 0.9 % and 80 %, respectively, at most AERONET sites. Large RMSE and low EE% were found in heavy aerosol loading conditions such as Asia and Africa. When the actual AOD was large, the retrieval bias of AOD from satellite observations would be amplified as reflected in RMSE and EE% to some extent. When compared to MODIS aerosol products, the AOD from the DPC GRASP/Model scheme showed good consistency in China, with all heavy aerosol loading regions being detected. However, the values of AOD were underestimated by DPC, probably due to overstrict cloud masking. Evaluation of the time series AOD showed the performance of the DPC GRASP/Model scheme was similar to the MODIS DT and better than the MODIS DB and MAIAC products. Therefore, to summarize, the DPC could capture spatial and temporal variations in aerosols. The study improves our understanding of DPC and finds a solution for retrieving AOD based on GRASP algorithm. The continuous development of polarized multi-angle sensors plays an important role in aerosol monitoring in the future.
The AERONET Level 2.0 AOD dataset can be downloaded from https://aeronet.gsfc.nasa.gov/ (last access: 15 March 2022; Holben et al., 2006) and a request to download the GRASP model can be made at https://www.grasp-open.com/ (last access: 15 March 2022; Dubovik et al., 2022). The MODIS Dark Target and Deep Blue aerosol products can be accessed and downloaded at https://ladsweb.modaps.eosdis.nasa.gov/ (last access: 15 March 2022).
The study was completed with cooperation between all authors. SJ and YM designed the study topic, and SJ conducted the experiment and prepared the manuscript. OD and CC advised the analysis, revised the manuscript, and helped to run the GRASP model. JH provided the general guidance of DPC/Gaofen-5 data. YM, BL, and WG provided funding and gave validation of study results.
At least one of the (co-)authors is a member of the editorial board of Atmospheric Measurement Techniques. The peer-review process was guided by an independent editor, and the authors also have no other competing interests to declare.
Publisher's note: Copernicus Publications remains neutral with regard to jurisdictional claims in published maps and institutional affiliations.
We are grateful to the Moderate Resolution Imaging Spectroradiometer (MODIS) team, the Aerosol Robotic Network (AERONET) organization and the GaoFen-5 Directional Polarimetric Camera (DPC) development team for freely distributing their aerosol products and measurements. The numerical calculations in this paper have been done on the supercomputing system in the Supercomputing Center of Wuhan University. Finally, we would also like to thank all reviewers for their constructive and valuable comments.
This research has been supported by the National Key Research and Development Program of China (grant no. 2018YFB0504500) and the National Natural Science Foundation of China (grant nos. 41875038, 42071348, and 42001291).
This paper was edited by Linlu Mei and reviewed by Stefan Kinne and two anonymous referees.
Albrecht, B. A.: AEROSOLS, CLOUD MICROPHYSICS, AND FRACTIONAL CLOUDINESS, Science, 245, 1227–1230, https://doi.org/10.1126/science.245.4923.1227, 1989.
Ångstrom, A.: The Parameter of Atmospheric Turbidity, Tellus, 16, 64–75, https://doi.org/10.3402/tellusa.v16i1.8885, 1964.
Breon, F. M. and Colzy, S.: Cloud detection from the spaceborne POLDER instrument and validation against surface synoptic observations, J. Appl. Meteorol., 38, 777–785, https://doi.org/10.1175/1520-0450(1999)038<0777:cdftsp>2.0.co;2, 1999.
Breon, F. M. and Goloub, P.: Cloud droplet effective radius from spaceborne polarization measurements, Geophys. Res. Lett., 25, 1879–1882, https://doi.org/10.1029/98gl01221, 1998.
Che, H., Yang, L., Liu, C., Xia, X., Wang, Y., Wang, H., Wang, H., Lu, X., and Zhang, X.: Long-term validation of MODIS C6 and C6.1 Dark Target aerosol products over China using CARSNET and AERONET, Chemosphere, 236, 124268, https://doi.org/10.1016/j.chemosphere.2019.06.238, 2019.
Chen, C., Dubovik, O., Fuertes, D., Litvinov, P., Lapyonok, T., Lopatin, A., Ducos, F., Derimian, Y., Herman, M., Tanré, D., Remer, L. A., Lyapustin, A., Sayer, A. M., Levy, R. C., Hsu, N. C., Descloitres, J., Li, L., Torres, B., Karol, Y., Herrera, M., Herreras, M., Aspetsberger, M., Wanzenboeck, M., Bindreiter, L., Marth, D., Hangler, A., and Federspiel, C.: Validation of GRASP algorithm product from POLDER/PARASOL data and assessment of multi-angular polarimetry potential for aerosol monitoring, Earth Syst. Sci. Data, 12, 3573–3620, https://doi.org/10.5194/essd-12-3573-2020, 2020.
Cox, C. and Munk, W.: Measurement Of The Roughness Of The Sea Surface From Photographs Of The Suns Glitter, J. Opt. Soc. America, 44, 838–850, https://doi.org/10.1364/JOSA.44.000838, 1954.
Deschamps, P., Breon, F., Leroy, M., Podaire, A., Bricaud, A., Buriez, J., and Seze, G.: The POLDER mission: instrument characteristics and scientific objectives, IEEE Trans. Geosci. Remote Sens., 32, 598–615, https://doi.org/10.1109/36.297978, 1994.
Deuzé, J. L., Bréon, F. M., Devaux, C., Goloub, P., Herman, M., Lafrance, B., Maignan, F., Marchand, A., Nadal, F., Perry, G., and Tanré, D.: Remote sensing of aerosols over land surfaces from POLDER-ADEOS-1 polarized measurements, J. Geophys. Res.-Atmos., 106, 4913–4926, https://doi.org/10.1029/2000jd900364, 2001.
Diner, D. J., Beckert, J. C., Reilly, T. H., Bruegge, C. J., Conel, J. E., Kahn, R. A., Martonchik, J. V., Ackerman, T. P., Davies, R., Gerstl, S. A. W., Gordon, H. R., Muller, J. P., Myneni, R. B., Sellers, P. J., Pinty, B., and Verstraete, M. M.: Multi-angle Imaging SpectroRadiometer (MISR) – Instrument description and experiment overview, IEEE Trans. Geosci. Remote Sens., 36, 1072–1087, https://doi.org/10.1109/36.700992, 1998.
Dubovik, O. and King, M. D.: A flexible inversion algorithm for retrieval of aerosol optical properties from Sun and sky radiance measurements, J. Geophys. Res.-Atmos., 105, 20673–20696, https://doi.org/10.1029/2000JD900282, 2000.
Dubovik, O., Sinyuk, A., Lapyonok, T., Holben, B.N., Mishchenko, M., Yang, P., Eck, T.F., Volten, H., Munoz, O., Veihelmann, B., van der Zande, W. J., Leon, J. F., Sorokin, M., and Slutsker, I.: Application of spheroid models to account for aerosol particle nonsphericity in remote sensing of desert dust, J. Geophys. Res.-Atmos., 111, D11208, https://doi.org/10.1029/2005jd006619, 2006.
Dubovik, O., Herman, M., Holdak, A., Lapyonok, T., Tanré, D., Deuzé, J. L., Ducos, F., Sinyuk, A., and Lopatin, A.: Statistically optimized inversion algorithm for enhanced retrieval of aerosol properties from spectral multi-angle polarimetric satellite observations, Atmos. Meas. Tech., 4, 975–1018, https://doi.org/10.5194/amt-4-975-2011, 2011.
Dubovik, O., Lapyonok, T., Litvinov, P., Herman, M., Fuertes, D., Ducos, F., Lopatin, A., Chaikovsky, A., Torres, B., Derimian, Y., Huang, X., Aspetsberger, M., and Federspiel, C.: GRASP: a versatile algorithm for characterizing the atmosphere, SPIE Newsroom, https://doi.org/10.1117/2.1201408.005558, 2014.
Dubovik, O., Li, Z., Mishchenko, M.I., Tanré, D., Karol, Y., Bojkov, B., Cairns, B., Diner, D. J., Espinosa, W. R., Goloub, P., Gu, X., Hasekamp, O., Hong, J., Hou, W., Knobelspiesse, K.D., Landgraf, J., Li, L., Litvinov, P., Liu, Y., Lopatin, A., Marbach, T., Maring, H., Martins, V., Meijer, Y., Milinevsky, G., Mukai, S., Parol, F., Qiao, Y., Remer, L., Rietjens, J., Sano, I., Stammes, P., Stamnes, S., Sun, X., Tabary, P., Travis, L. D., Waquet, F., Xu, F., Yan, C., and Yin, D.: Polarimetric remote sensing of atmospheric aerosols: Instruments, methodologies, results, and perspectives, J. Quant. Spectrosc. Ra. Transf., 224, 474–511, https://doi.org/10.1016/j.jqsrt.2018.11.024, 2019.
Dubovik, O., Fuertes, D., Litvinov, P., Lopatin, A., Lapyonok, T., Doubovik, I., Xu, F., Ducos, F., Chen, C., Torres, B., Derimian, Y., Li, L., Herreras-Giralda, M., Herrera, M., Karol, Y., Matar, C., Schuster, G. L., Espinosa, R., Puthukkudy, A., Li, Z., Fischer, J., Preusker, R., Cuesta, J., Kreuter, A., Cede, A., Aspetsberger, M., Marth, D., Bindreiter, L., Hangler, A., Lanzinger, V., Holter, C., and Federspiel, C.: A Comprehensive Description of Multi-Term LSM for Applying Multiple a Priori Constraints in Problems of Atmospheric Remote Sensing: GRASP Algorithm, Concept, and Applications, Front. Remote Sens., 2, 706851, https://doi.org/10.3389/frsen.2021.706851, 2021.
Dubovik, O., Ducos, F., and Fuertes, D.: The GRASP Package, An overview, GRASP open [data set], https://www.grasp-open.com/, last access: 15 March, 2022.
Eck, T. F., Holben, B. N., Reid, J. S., Dubovik, O., Smirnov, A., O'Neill, N. T., Slutsker, I., and Kinne, S.: Wavelength dependence of the optical depth of biomass burning, urban, and desert dust aerosols, J. Geophys. Res.-Atmos., 104, 31333–31349, https://doi.org/10.1029/1999jd900923, 1999.
Eck, T. F., Holben, B. N., Sinyuk, A., Pinker, R. T., Goloub, P., Chen, H., Chatenet, B., Li, Z., Singh, R. P., Tripathi, S. N., Reid, J. S., Giles, D. M., Dubovik, O., O'Neill, N. T., Smirnov, A., Wang, P., and Xia, X.: Climatological aspects of the optical properties of fine/coarse mode aerosol mixtures, J. Geophys. Res., 115, D19205, https://doi.org/10.1029/2010jd014002, 2010.
Gao, J., Woodward, A., Vardoulakis, S., Kovats, S., Wilkinson, P., Li, L., Xu, L., Li, J., Yang, J., Li, J., Cao, L., Liu, X., Wu, H., and Liu, Q.: Haze, public health and mitigation measures in China: A review of the current evidence for further policy response, Sci. Total Environ., 578, 148–157, https://doi.org/10.1016/j.scitotenv.2016.10.231, 2017.
Ge, B., Mei, X., Li, Z., Hou, W., Xie, Y., Zhang, Y., Xu, H., Li, K., and Wei, Y.: An improved algorithm for retrieving high resolution fine-mode aerosol based on polarized satellite data: Application and validation for POLDER-3, Remote Sens. Environ., 247, 111894, https://doi.org/10.1016/j.rse.2020.111894, 2020.
Goloub, P. and Deuze, J. L.: Analysis of the POLDER polarization measurements performed over cloud covers, IEEE Trans. Geosci. Remote Sens., 32, 78–88, https://doi.org/10.1109/36.285191, 1994.
Gomez-Chova, L., Camps-Valls, G., Calpe-Maravilla, J., Guanter, L., and Moreno, J.: Cloud-screening algorithm for ENVISAT/MERIS multispectral images, IEEE Trans. Geosci. Remote Sens., 45, 4105–4118, https://doi.org/10.1109/tgrs.2007.905312, 2007.
Guo, J., Deng, M., Lee, S. S., Wang, F., Li, Z., Zhai, P., Liu, H., Lv, W., Yao, W., and Li, X.: Delaying precipitation and lightning by air pollution over the Pearl River Delta. Part I: Observational analyses, J. Geophys. Res.-Atmos., 121, 6472–6488, https://doi.org/10.1002/2015jd023257, 2016.
Gupta, P., Remer, L. A., Levy, R. C., and Mattoo, S.: Validation of MODIS 3 km land aerosol optical depth from NASA's EOS Terra and Aqua missions, Atmos. Meas. Tech., 11, 3145–3159, https://doi.org/10.5194/amt-11-3145-2018, 2018.
Hagolle, O., Goloub, P., Deschamps, P.-Y., Cosnefroy, H., Briottet, X., Bailleul, T., Nicolas, J.-M., Parol, F., Lafrance, B., and Herman, M.: Results of POLDER in-flight calibration, IEEE Trans. Geosci. Remote Sens., 37, 1550–1566, https://doi.org/10.1109/36.763266, 1999.
Holben, B. N., Eck, T. F., Slutsker, I., Tanre, D., Buis, J. P., Setzer, A., Vermote, E., Reagan, J. A., Kaufman, Y. J., Nakajima, T., Lavenu, F., Jankowiak, I., and Smirnov, A.: AERONET – A federated instrument network and data archive for aerosol characterization, Remote Sens. Environ., 66, 1–16, https://doi.org/10.1016/s0034-4257(98)00031-5, 1998.
Holben, B., Eck, T., Slutsker, I., Smirnov, A., Sinyuk, A., Schafer, J., Giles, D., and Dubovik, O.: AERONET's version 2.0 quality assurance criteria, Remote Sens. Atmos. Clouds [data set], 6408, 64080Q, https://doi.org/10.1117/12.706524, 2006.
Hsu, N. C., Tsay, S. C., King, M. D., and Herman, J. R.: Aerosol properties over bright-reflecting source regions, IEEE Trans. Geosci. Remote Sens., 42, 557–569, https://doi.org/10.1109/TGRS.2004.824067, 2004.
Hsu, N. C., Jeong, M. J., Bettenhausen, C., Sayer, A. M., Hansell, R., Seftor, C. S., Huang, J., and Tsay, S. C.: Enhanced Deep Blue aerosol retrieval algorithm: The second generation, J. Geophys. Res.-Atmos., 118, 9296–9315, https://doi.org/10.1002/jgrd.50712, 2013.
Jin, S., Ma, Y., Zhang, M., Gong, W., Dubovik, O., Liu, B., Shi, Y., and Yang, C.: Retrieval of 500 m Aerosol Optical Depths from MODIS Measurements over Urban Surfaces under Heavy Aerosol Loading Conditions in Winter, Remote Sens., 11, 2218, https://doi.org/10.3390/rs11192218, 2019.
Jin, S., Zhang, M., Ma, Y., Gong, W., Chen, C., Yang, L., Hu, X., Liu, B., Chen, N., Du, B., and Shi, Y.: Adapting the Dark Target Algorithm to Advanced MERSI Sensor on the FengYun-3-D Satellite: Retrieval and Validation of Aerosol Optical Depth Over Land, IEEE Trans. Geosci. Remote Sens., 59, 8781–8797, https://doi.org/10.1109/TGRS.2020.3021021, 2021.
Kacenelenbogen, M., Léon, J.-F., Chiapello, I., and Tanré, D.: Characterization of aerosol pollution events in France using ground-based and POLDER-2 satellite data, Atmos. Chem. Phys., 6, 4843–4849, https://doi.org/10.5194/acp-6-4843-2006, 2006.
Kaufman, Y. J., Tanré, D., Gordon, H. R., Nakajima, T., Lenoble, J., Frouin, R., Grassl, H., Herman, B. M., King, M. D., and Teillet, P. M.: Passive remote sensing of tropospheric aerosol and atmospheric correction for the aerosol effect, J. Geophys. Res.-Atmos., 102, 16815–16830, https://doi.org/10.1029/97jd01496, 1997.
Koren, I., Remer, L. A., Kaufman, Y. J., Rudich, Y., and Martins, J. V.: On the twilight zone between clouds and aerosols, Geophys. Res. Lett., 34, L08805, https://doi.org/10.1029/2007gl029253, 2007.
Lenoble, J., Remer, L., and Tanre, D.: Aerosol Remote Sensing, Springer-Verlag Berlin, Heidelberg, https://doi.org/10.1007/978-3-642-17725-5, 2013.
Levy, R. C., Mattoo, S., Munchak, L. A., Remer, L. A., Sayer, A. M., Patadia, F., and Hsu, N. C.: The Collection 6 MODIS aerosol products over land and ocean, Atmos. Meas. Tech., 6, 2989–3034, https://doi.org/10.5194/amt-6-2989-2013, 2013.
Levy, R. and Hsu, C.: MODIS Atmosphere L2 Aerosol Product, NASA MODIS Adaptive Processing System, Goddard Space Flight Center, LAADS DAAC [data set], https://ladsweb.modaps.eosdis.nasa.gov/ (last access: 15 March 2022), 2015.
Li, J. H., Ma, J. J., Li, C., Wang, Y. Y., Li, Z. Q., and Hong, J.: Multi-information collaborative cloud identification algorithm in Gaofen-5 Directional Polarimetric Camera imagery, J. Quant. Spectrosc. Ra. Transf., 261, 107439, https://doi.org/10.1016/j.jqsrt.2020.107439, 2021.
Li, L., Dubovik, O., Derimian, Y., Schuster, G. L., Lapyonok, T., Litvinov, P., Ducos, F., Fuertes, D., Chen, C., Li, Z., Lopatin, A., Torres, B., and Che, H.: Retrieval of aerosol components directly from satellite and ground-based measurements, Atmos. Chem. Phys., 19, 13409–13443, https://doi.org/10.5194/acp-19-13409-2019, 2019.
Li, L., Che, H., Zhang, X., Chen, C., Chen, X., Gui, K., Liang, Y., Wang, F., Derimian, Y., Fuertes, D., Dubovik, O., Zheng, Y., Zhang, L., Guo, B., Wang, Y., and Zhang, X.: A satellite-measured view of aerosol component content and optical property in a haze-polluted case over North China Plain, Atmos. Res., 266, 105958, https://doi.org/10.1016/j.atmosres.2021.105958, 2022.
Li, X. and Strahler, A. H.: Geometric-optical bidirectional reflectance modeling of the discrete crown vegetation canopy: effect of crown shape and mutual shadowing, IEEE Trans. Geosci. Remote Sens., 30, 276–292, https://doi.org/10.1109/36.134078, 1992.
Li, Z. Q., Lau, W. K. M., Ramanathan, V., Wu, G., Ding, Y., Manoj, M. G., Liu, J., Qian, Y., Li, J., Zhou, T., Fan, J., Rosenfeld, D., Ming, Y., Wang, Y., Huang, J., Wang, B., Xu, X., Lee, S. S., Cribb, M., Zhang, F., Yang, X., Zhao, C., Takemura, T., Wang, K., Xia, X., Yin, Y., Zhang, H., Guo, J., Zhai, P. M., Sugimoto, N., Babu, S. S., and Brasseur, G.P.: Aerosol and monsoon climate interactions over Asia, Rev. Geophys., 54, 866–929, https://doi.org/10.1002/2015rg000500, 2016.
Li, Z., Hou, W., Hong, J., Zheng, F., Luo, D., Wang, J., Gu, X., and Qiao, Y.: Directional Polarimetric Camera (DPC): Monitoring aerosol spectral optical properties over land from satellite observation, J. Quant. Spectrosc. Ra. Transf., 218, 21–37, https://doi.org/10.1016/j.jqsrt.2018.07.003, 2018.
Liu, B., Ma, X., Ma, Y., Li, H., Jin, S., Fan, R., and Gong, W.: The relationship between atmospheric boundary layer and temperature inversion layer and their aerosol capture capabilities, Atmos. Res., 271, 106121, https://doi.org/10.1016/j.atmosres.2022.106121, 2022.
Lopatin, A., Dubovik, O., Fuertes, D., Stenchikov, G., Lapyonok, T., Veselovskii, I., Wienhold, F. G., Shevchenko, I., Hu, Q., and Parajuli, S.: Synergy processing of diverse ground-based remote sensing and in situ data using the GRASP algorithm: applications to radiometer, lidar and radiosonde observations, Atmos. Meas. Tech., 14, 2575–2614, https://doi.org/10.5194/amt-14-2575-2021, 2021.
Lyapustin, A., Wang, Y., Korkin, S., and Huang, D.: MODIS Collection 6 MAIAC algorithm, Atmos. Meas. Tech., 11, 5741–5765, https://doi.org/10.5194/amt-11-5741-2018, 2018.
Ma, Y., Zhu, Y., Liu, B., Li, H., Jin, S., Zhang, Y., Fan, R., and Gong, W.: Estimation of the vertical distribution of particle matter (PM2.5) concentration and its transport flux from lidar measurements based on machine learning algorithms, Atmos. Chem. Phys., 21, 17003–17016, https://doi.org/10.5194/acp-21-17003-2021, 2021.
Martins, J. V., Tanré, D., Remer, L., Kaufman, Y., Mattoo, S., and Levy, R.: MODIS Cloud screening for remote sensing of aerosols over oceans using spatial variability, Geophys. Res. Lett., 29, MOD4, https://doi.org/10.1029/2001GL013252, 2002.
McCormick, M. P., Hamill, P., Pepin, T. J., Chu, W. P., Swissler, T. J., and McMaster, L. R.: SATELLITE STUDIES OF THE STRATOSPHERIC AEROSOL, B. Am. Meteorol. Soc., 60, 1038–1046, https://doi.org/10.1175/1520-0477(1979)060<1038:ssotsa>2.0.co;2, 1979.
Mishchenko, M. I. and Travis, L. D.: Satellite retrieval of aerosol properties over the ocean using polarization as well as intensity of reflected sunlight, J. Geophys. Res.-Atmos., 102, 16989–17013, https://doi.org/10.1029/96JD02425, 1997.
Nadal, F. and Bréon, F. M.: Parameterization of Surface Polarized Reflectance Derived from POLDER Spaceborne Measurements, IEEE Trans. Geosci. Remote Sens., 37, 1709–1718, https://doi.org/10.1109/36.763292, 1999.
Nakajima, T., Yoon, S. C., Ramanathan, V., Shi, G. Y., Takemura, T., Higurashi, A., Takamura, T., Aoki, K., Sohn, B. J., Kim, S. W., Tsuruta, H., Sugimoto, N., Shimizu, A., Tanimoto, H., Sawa, Y., Lin, N. H., Lee, C. T., Goto, D., and Schutgens, N.: Overview of the Atmospheric Brown Cloud East Asian Regional Experiment 2005 and a study of the aerosol direct radiative forcing in east Asia, J. Geophys. Res.-Atmos., 112, D24S91, https://doi.org/10.1029/2007jd009009, 2007.
Ou, Y., Li, L., Ying, Z., Dubovik, O., Derimian, Y., Chen, C., Fuertes, D., Xie, Y., Lopatin, A., Ducos, F., and Peng, Z.: Spatio-Temporal Variability of Aerosol Components, Their Optical and Microphysical Properties over North China during Winter Haze in 2012, as Derived from POLDER/PARASOL Satellite Observations, Remote Sens., 13, 2682, https://doi.org/10.3390/rs13142682, 2021.
Qie, L., Li, Z., Zhu, S., Xu, H., Xie, Y., Qiao, R., Hong, J., and Tu, B.: In-flight radiometric and polarimetric calibration of the Directional Polarimetric Camera onboard the GaoFen-5 satellite over the ocean, Appl. Opt., 60, 7186–7199, https://doi.org/10.1364/AO.422980, 2021.
Rao, C. R. N., Stowe, L. L., and McClain, E. P.: REMOTE-SENSING OF AEROSOLS OVER THE OCEANS USING AVHRR DATA THEORY, PRACTICE AND APPLICATIONS, Int. J. Remote Sens., 10, 743–749, https://doi.org/10.1080/01431168908903915, 1989.
Remer, L. A., Mattoo, S., Levy, R. C., Heidinger, A., Pierce, R. B., and Chin, M.: Retrieving aerosol in a cloudy environment: aerosol product availability as a function of spatial resolution, Atmos. Meas. Tech., 5, 1823–1840, https://doi.org/10.5194/amt-5-1823-2012, 2012.
Rosenfeld, D., Lohmann, U., Raga, G. B., O'Dowd, C. D., Kulmala, M., Fuzzi, S., Reissell, A., and Andreae, M. O.: Flood or drought: How do aerosols affect precipitation?, Science, 321, 1309–1313, https://doi.org/10.1126/science.1160606, 2008.
Sayer, A. M., Hsu, N. C., Bettenhausen, C., and Jeong, M. J.: Validation and uncertainty estimates for MODIS Collection 6 “Deep Blue” aerosol data, J. Geophys. Res.-Atmos., 118, 7864–7872, https://doi.org/10.1002/jgrd.50600, 2013.
Sayer, A. M., Munchak, L. A., Hsu, N. C., Levy, R. C., Bettenhausen, C., and Jeong, M. J.: MODIS Collection 6 aerosol products: Comparison between Aqua's e-Deep Blue, Dark Target, and “merged” data sets, and usage recommendations, J. Geophys. Res.-Atmos., 119, 13965–13989, https://doi.org/10.1002/2014jd022453, 2014.
Shi, T., Han, G., Ma, X., Gong, W., Chen, W., Liu, J., Zhang, X., Pei, Z., Gou, H., and Bu, L.: Quantifying CO2 Uptakes Over Oceans Using LIDAR: A Tentative Experiment in Bohai Bay, Geophys. Res. Lett., 48, e2020GL091160, https://doi.org/10.1029/2020GL091160, 2021.
Tanré, D., Bréon, F. M., Deuzé, J. L., Dubovik, O., Ducos, F., François, P., Goloub, P., Herman, M., Lifermann, A., and Waquet, F.: Remote sensing of aerosols by using polarized, directional and spectral measurements within the A-Train: the PARASOL mission, Atmos. Meas. Tech., 4, 1383–1395, https://doi.org/10.5194/amt-4-1383-2011, 2011.
Tegen, I. and Lacis, A. A.: Modeling of particle size distribution and its influence on the radiative properties of mineral dust aerosol, J. Geophys. Res.-Atmos., 101, 19237–19244, https://doi.org/10.1029/95JD03610, 1996.
Zhang, M., Jin, S., Ma, Y., Fan, R., Wang, L., Gong, W., and Liu, B.: Haze events at different levels in winters: A comprehensive study of meteorological factors, Aerosol characteristics and direct radiative forcing in megacities of north and central China, Atmos. Environ., 245, 118056, https://doi.org/10.1016/j.atmosenv.2020.118056, 2021.
Zhdanova, E. Y., Chubarova, N. Y., and Lyapustin, A. I.: Assessment of urban aerosol pollution over the Moscow megacity by the MAIAC aerosol product, Atmos. Meas. Tech., 13, 877–891, https://doi.org/10.5194/amt-13-877-2020, 2020.
Zhu, S., Li, Z., Qie, L., Xu, H., Ge, B., Xie, Y., Qiao, R., Xie, Y., Hong, J., Meng, B., Tu, B., and Chen, F.: In-Flight Relative Radiometric Calibration of a Wide Field of View Directional Polarimetric Camera Based on the Rayleigh Scattering over Ocean, Remote Sens., 14, 1211, https://doi.org/10.3390/rs14051211, 2022.
Zhu, Z. and Woodcock, C. E.: Object-based cloud and cloud shadow detection in Landsat imagery, Remote Sens. Environ., 118, 83–94, https://doi.org/10.1016/j.rse.2011.10.028, 2012.