the Creative Commons Attribution 4.0 License.
the Creative Commons Attribution 4.0 License.
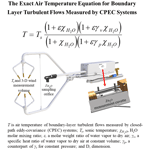
Air temperature equation derived from sonic temperature and water vapor mixing ratio for turbulent airflow sampled through closed-path eddy-covariance flux systems
Xinhua Zhou
Eugene S. Takle
Xiaojie Zhen
Andrew E. Suyker
Tala Awada
Jane Okalebo
Jiaojun Zhu
Air temperature (T) plays a fundamental role in many aspects of the flux exchanges between the atmosphere and ecosystems. Additionally, knowing where (in relation to other essential measurements) and at what frequency T must be measured is critical to accurately describing such exchanges. In closed-path eddy-covariance (CPEC) flux systems, T can be computed from the sonic temperature (Ts) and water vapor mixing ratio that are measured by the fast-response sensors of a three-dimensional sonic anemometer and infrared CO2–H2O analyzer, respectively. T is then computed by use of either , where q is specific humidity, or , where e is water vapor pressure and P is atmospheric pressure. Converting q and into the same water vapor mixing ratio analytically reveals the difference between these two equations. This difference in a CPEC system could reach ±0.18 K, bringing an uncertainty into the accuracy of T from both equations and raising the question of which equation is better. To clarify the uncertainty and to answer this question, the derivation of T equations in terms of Ts and H2O-related variables is thoroughly studied. The two equations above were developed with approximations; therefore, neither of their accuracies was evaluated, nor was the question answered. Based on first principles, this study derives the T equation in terms of Ts and the water vapor molar mixing ratio () without any assumption and approximation. Thus, this equation inherently lacks error, and the accuracy in T from this equation (equation-computed T) depends solely on the measurement accuracies of Ts and . Based on current specifications for Ts and in the CPEC300 series, and given their maximized measurement uncertainties, the accuracy in equation-computed T is specified within ±1.01 K. This accuracy uncertainty is propagated mainly (±1.00 K) from the uncertainty in Ts measurements and a little (±0.02 K) from the uncertainty in measurements. An improvement in measurement technologies, particularly for Ts, would be a key to narrowing this accuracy range. Under normal sensor and weather conditions, the specified accuracy range is overestimated, and actual accuracy is better. Equation-computed T has a frequency response equivalent to high-frequency Ts and is insensitive to solar contamination during measurements. Synchronized at a temporal scale of the measurement frequency and matched at a spatial scale of measurement volume with all aerodynamic and thermodynamic variables, this T has advanced merits in boundary-layer meteorology and applied meteorology.
- Article
(806 KB) - Full-text XML
- BibTeX
- EndNote
The equation of state, P=ρRT, is a fundamental equation for describing all atmospheric flows, where P is atmospheric pressure, ρ is moist-air density, R is the gas constant for moist air, and T is air temperature (Wallace and Hobbs, 2006). In boundary-layer flow, where turbulence is nearly always present, accurate representation of the “state” of the atmosphere at any given “point” and time requires consistent representation of spatial and temporal scales for all thermodynamic factors of P, ρ, and T (Panofsky and Dutton, 1984). Additionally, for observing fluxes describing exchanges of quantities, such as heat and moisture between the Earth and the atmosphere, it is critical to know all three-dimensional (3-D) components of wind speed at the same location and temporal scale as the thermodynamic variables (Laubach and McNaughton, 1998).
In a closed-path eddy-covariance (CPEC) system, the 3-D wind components and sonic temperature (Ts) are measured by a 3-D sonic anemometer in the sonic measurement volume near which air is sampled through the orifice of an infrared H2O–CO2 analyzer (hereafter referred to as the infrared analyzer) into its closed-path H2O–CO2 measurement cuvette, where air moisture is measured by the analyzer (Fig. 1). The flow pressure inside the cuvette (Pc) and the differential (ΔP) between Pc and ambient flow pressure in the sampling location are also measured (Campbell Scientific Inc., 2018c). Atmospheric P in the sampling volume, therefore, is a sum of Pc and ΔP. Pc, along with the internal T, is further used for infrared measurements of air moisture (i.e., ρw, H2O density) to calculate the water mixing ratio (χw) inside the cuvette that is also equal to χw in the CPEC measurement volume, including sonic measurement volume and the air sampling location. Finally, the Ts and χw from the CPEC measurement volume, after spatial and temporal synchronization (Horst and Lenschow, 2009), are used to calculate the T inside this volume. Two optional equations (Schotanus et al., 1983; Kaimal and Gaynor, 1991; see Sect. 2, Background), which need rigorous evaluation, are available for this T calculation. In summary, the boundary-layer flow measured by a CPEC system has all variables quantified with consistent representation of spatial and temporal scales for moist turbulence thermodynamics (i.e., state) if the following are available: 3-D wind; P measured differentially; T from an equation; and ρ from P, T, and χw.
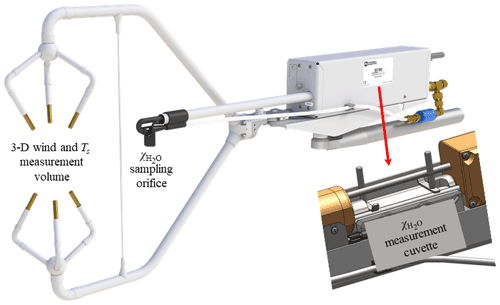
Figure 1Measurement volume for three-dimensional (3-D) wind and sonic temperature (Ts), sampling orifice for the H2O molar mixing ratio (), and measurement cuvette for in the CPEC300 series (Campbell Scientific Inc., UT, USA).
In this paper, the authors (1) derive a T equation in terms of Ts and χw based on first principles as an alternative to the commonly used equations that are based on approximations, (2) estimate and verify the accuracy of the first-principles T, (3) assess the expected advantages of the first-principles T as a high-frequency signal insensitive to solar contamination suffered by conventional T sensor measurements (Lin et al., 2001; Blonquist and Bugbee, 2018), and (4) brief the potential applications of the derived T equation in flux measurements. We first provide a summary of the moist turbulence thermodynamics of the boundary-layer flows measured by CPEC flux systems.
A CPEC system is commonly used to measure boundary-layer flows for the CO2, H2O, heat, and momentum fluxes between ecosystems and the atmosphere. Such a system is equipped with a 3-D sonic anemometer to measure the speed of sound in three dimensions in the central open space of the instrument (hereafter referred to as open space), from which can be calculated Ts and 3-D components of wind with a fast response. Integrated with this sonic anemometer, a fast-response infrared analyzer concurrently measures CO2 and H2O in its cuvette (closed space) of infrared measurements, through which air is sampled under pump pressure while being heated (Fig. 1). The analyzer outputs the CO2 mixing ratio (i.e., , where is CO2 density and ρd is dry-air density) and χw (i.e., ). Together, these instruments provide high-frequency (e.g., 10 Hz) measurements from which the fluxes are computed (Aubinet et al., 2012) at a point represented by the sampling space of the CPEC system.
These basic high-frequency measurements of 3-D wind speed, Ts, χw, and provide observations from which mean and fluctuation properties of air, such as ρd, ρ, ρw, and , and, hence, fluxes can be determined. For instance, water vapor flux is calculated from , where w is the vertical velocity of air, and the prime indicates the fluctuation of the variable away from its mean as indicated by the overbar (e.g., ). Given the measurements of χw and P from CPEC systems and based on the gas laws (Wallace and Hobbs, 2006), ρd is derived from
where Rd is the gas constant for dry air and Rv is the gas constant for water vapor. In turn, ρw is equal to ρdχw and ρ is a sum of ρd and ρw. All mentioned physical properties can be derived if T in Eq. (1) for ρd is acquired.
Additionally, equations for ecosystem exchange and flux require (Gu et al., 2012) and (Foken et al., 2012). Furthermore, due to accuracy limitations in measurements of w from a modern sonic anemometer, the dry-air flux of must be derived from (Webb et al., 1980; Lee and Massman, 2011). Because of its role in flux measurements, a high-frequency representation of ρd is needed. To acquire such a ρd from Eq. (1) for advanced applications, high-frequency T in temporal synchronization with χw and P is needed.
In a modern CPEC system, P is measured using a fast-response barometer suitable for measurements at a high frequency (e.g., 10 Hz; Campbell Scientific Inc., 2018a), and, as discussed above, χw is a high-frequency signal from a fast-response infrared analyzer (e.g., commonly up to 20 Hz). If T is measured using a slow-response sensor, the three independent variables in Eq. (1) do not have equivalent synchronicity in their frequency response. In terms of frequency response, cannot be correctly acquired. derived based on Eq. (1) also has uncertainty, although it can be approximated from either of the two following equations:
and
Equation (2) is mathematically valid in averaging rules (Stull, 1988), but the response of the system to T is slower than to χw and even P, while Eq. (3) is invalid under averaging rules, although its three overbar independent variables can be evaluated over an average interval. Consequently, neither nor can be evaluated strictly in theory.
Measurements of T at a high frequency (similarly to those at a low frequency) are contaminated by solar radiation, even under shields (Lin et al., 2001) and when aspirated (Campbell Scientific Inc., 2010; R.M. Young Company, 2004; Apogee Instruments Inc., 2013; Blonquist and Bugbee, 2018). Although a naturally ventilated or fan-aspirated radiation shield could ensure the accuracy of a conventional (i.e., slow-response) thermometer often within ±0.2 K at 0 ∘C (Harrison and Burt, 2021) to satisfy the standard for conventional T measurement as required by the World Meteorological Organization (WMO, 2018), the aspiration shield method cannot acquire T at a high frequency due to the disturbance of an aspiration fan and the blockage of a shield to natural turbulent flows. Additionally, fine wires have limited applicability for long-term measurements in rugged field conditions typically encountered in ecosystem monitoring.
To avoid the issues above in use of either slow- or fast-response T sensors under field conditions, deriving T from Ts and χw (Schotanus et al., 1983; Kaimal and Gaynor, 1991) is an advantageous alternative to the applications of T in CPEC measurements and is a significant technology for instrumentation to pursue. In a CPEC system, Ts is measured at a high frequency (e.g., 10 Hz) using a fast-response sonic anemometer to detect the speed of sound in the open space (Munger et al., 2012), provided there is no evidence of contamination by solar radiation. It is a high-frequency signal. χw is measured at the same frequency as for Ts using an infrared analyzer equivalent to the sonic anemometer with a high-frequency response time (Ma et al., 2017). χw reported from a CPEC system is converted from water vapor molar density measured inside the closed-space cuvette, whose internal pressure and internal temperature are more stable than P and T in the open space and can be more accurately measured. Because of this, solar warming and radiation cooling of the cuvette is irrelevant, as long as water molar density, pressure, and temperature inside the closed-space cuvette are more accurately measured. Therefore, it could be reasonably expected that T calculated from Ts and χw in a CPEC system should be a high-frequency signal insensitive to solar radiation.
The first of two equations commonly used to compute T from Ts and air-moisture-related variables is given by Schotanus et al. (1983) as
where q is specific humidity, defined as a ratio of water vapor to moist-air density. The second equation is given by Kaimal and Gaynor (1991) as
where e is water vapor pressure. Rearranging these two equations gives T in terms of Ts and χw. Expressing q in terms of ρd and ρw, Eq. (4) becomes
and expressing e and P using the equation of state, Eq. (5) becomes
The χw-related terms in the denominator inside parentheses in both equations above clearly reveal that T values from the same Ts and χw using the two commonly used Eqs. (4) and (5) will not be the same. The absolute difference in the values (ΔTe, i.e., the difference in T between Eqs. 4 and 5) can be analytically expressed as
Given that, in a CPEC system, the sonic anemometer has an operational range in Ts of −30 to 57 ∘C (Campbell Scientific Inc., 2018b) and an infrared analyzer has a measurement range in χw of 0 to 0.045 kgH2O kg−1 (Campbell Scientific Inc., 2018a), ΔTe ranges up to 0.177 K, which brings an uncertainty in the accuracy of T calculated from either Eq. (4) or Eq. (5) and raises the question of which equation is better.
Reviewing the sources of Eq. (4) (Schotanus et al., 1983; Swiatek, 2009; van Dijk, 2002) and Eq. (5) (Ishii, 1935; Barrett and Suomi, 1949; Kaimal and Businger, 1963; Kaimal and Gaynor, 1991), it was found that approximation procedures were used in the derivation of both equations, but the approach to the derivation of Eq. (4) (Appendix A) is different from that of Eq. (5) (Appendix B). These different approaches create a disparity between the two commonly used equations as shown in Eq. (8), and the approximation procedures lead to the controversy as to which equation is more accurate. The controversy can be avoided if the T equation in terms of Ts and χw can be derived from the Ts equation and first-principles equations, if possible without an approximation and verified against precision measurements of T with minimized solar contamination.
As discussed above, a sonic anemometer measures the speed of sound (c) concurrently with measurement of the 3-D wind speed (Munger et al., 2012). The speed of sound in the homogeneous atmospheric boundary layer is defined by Barrett and Suomi (1949) as
where γ is the ratio of moist-air specific heat at constant pressure (Cp) to moist-air specific heat at constant volume (Cv). Substitution of the equation of state into Eq. (9) gives T as a function of c:
This equation reveals the opportunity to use measured c for the T calculation; however, both γ and R depend on air humidity, which is unmeasurable by sonic anemometry itself; Eq. (10) is, therefore, not applicable for T calculations inside a sonic anemometer. Alternatively, γ is replaced with its counterpart for dry air (γd, 1.4003, i.e., the ratio of dry-air specific heat at constant pressure (Cpd, 1004 J K−1 kg−1) to dry-air specific heat at constant volume (Cvd, 717 J K−1 kg−1)) and R is replaced with its counterpart for dry air (Rd, 287.06 J K−1 kg−1, i.e., the gas constant for dry air). Both replacements make the right side of Eq. (10) become , which is no longer a measure of T. However, γd and Rd are close to their respective values of γ and R in magnitude, and, after the replacements, the right side of Eq. (10) is defined as sonic temperature (Ts), given by (Campbell Scientific Inc., 2018b)
Comparing this equation to Eq. (10), given c, if air is dry, T must be equal to Ts; therefore, the authors state that “sonic temperature of moist air is the temperature that its dry-air component reaches when moist air has the same enthalpy.” Since both γd and Rd are constants and c is measured by a sonic anemometer and corrected for the crosswind effect inside the sonic anemometer based on its 3-D wind measurements (Liu et al., 2001; Zhou et al., 2018), Eq. (11) is used inside the operating system (OS) of modern sonic anemometers to report Ts instead of T.
Equations (9) to (11) provide a theoretical basis of first principles to derive the relationship of T to Ts and χw. In Eq. (9), γ and ρ vary with air humidity and P is related to ρ as described by the equation of state. Consequently, the derivation of T from Ts and χw for CPEC systems needs to address the relationship of γ, ρ, and P to air humidity in terms of χw.
3.1 Relationship of γ to χw
For moist air, the ratio of specific heat at constant pressure to specific heat at constant volume is
where Cp varies with air moisture between Cpd and Cpw (water vapor specific heat at constant pressure, 1952 J kg−1 K−1). It is the arithmetical average of Cpd and Cpw weighted by the dry air mass and water vapor mass, respectively, given by (Stull, 1988; Swiatek, 2009)
Based on the same rationale, Cv is
where Cvw is the specific heat of water vapor at constant volume (1463 J kg−1 K−1). Substituting Eqs. (13) and (14) into Eq. (12) generates
3.2 Relationship of to χw
Atmospheric P is the sum of Pd and e. Similarly, ρ is the sum of ρd and ρw. Using the equation of state, the ratio of P to ρ can be expressed as
In this equation, the ratio of Rv to Rd is given by
where R∗ is the universal gas constant, Mw is the molecular mass of water vapor (18.0153 kg kmol−1), and Md is the molecular mass of dry air (28.9645 kg kmol−1). The ratio of Mw to Md is 0.622, conventionally denoted by ε. Substituting Eq. (17), after its denominator is represented by ε, into Eq. (16) leads to
3.3 Relationship of Ts to T and χw
Substituting Eqs. (15) and (18) into Eq. (9), c2 is expressed in terms of T and χw along with atmospheric physics constants:
Further, substituting c2 into Eq. (11) generates
This Eq. (20) now expresses Ts in terms of the T of interest to this study, χw measured in CPEC systems, and atmospheric physics constants (i.e., ε, Cpw, Cpd, Cvw, and Cvd).
3.4 Air temperature equation
Rearranging the terms in Eq. (20) results in
This equation shows that T is a function of Ts and χw that are measured at a high frequency in a CPEC system by a sonic anemometer and an infrared analyzer.
A CPEC system outputs the water vapor molar mixing ratio (Campbell Scientific Inc., 2018a) commonly used in the community of eddy-covariance fluxes (AmeriFlux, 2018). The relation of water vapor mass to the molar mixing ratio ( in molH2O mol−1) is given by
Substituting this relation into Eq. (21) and denoting with γv = 2.04045 and with γp=1.94422, Eq. (21) is expressed as
This is the air temperature equation in terms of Ts and for use in CPEC systems. It is derived from a theoretical basis of first principles (i.e., Eqs. 9 to 11). In its derivation, except for the use of the equation of state and Dalton's law, no other assumptions or approximations are used. Therefore, Eq. (23) is an exact equation of T in terms of Ts and for the turbulent airflow sampled through a CPEC system and thus avoids the controversy in the use of Eqs. (4) and (5) arising from approximations, as shown in Appendices A and B. Therefore, T computed from this equation (hereafter referred to as equation-computed T) should be accurate, as long as the values of Ts and are exact.
For this study, however, Ts and are measured by the CPEC systems deployed in the field under changing weather conditions through four seasons. Their measured values must include measurement uncertainty in Ts, denoted by ΔTs, and in as well, denoted by . The uncertainties, ΔTs and/or , unavoidably propagate to create uncertainty in equation-computed T, denoted by ΔT, which makes an exact T impossible. In numerical analysis (Burden and Faires, 1993) or in statistics (Snedecor and Cochran, 1989), any applicable equation requires the specification of an uncertainty term. Therefore, the equations for T should include a specification of their respective uncertainty expressed as the bounds (i.e., the maximum and minimum limits) specifying the range of the equation-computed T that need to be known for any application. According to the definition of accuracy that was advanced by the International Organization for Standardization (2012), this uncertainty range is equivalent to the “accuracy” of the range contributed by both systematic errors (trueness) and random variability (precision). Apparently, ΔTs is the accuracy of Ts measurements and is the accuracy of measurements. Both should be evaluated from their respective measurement uncertainties. The accuracy of equation-computed T is ΔT. It should be specified through its relationship to ΔTs and .
3.5 Relationship of ΔT to ΔTs and
As measurement accuracies, ΔTs and can be reasonably considered small increments in a calculus sense. As such, depending on both small increments, ΔT is the total differential of T with respect to Ts and , given by
The two partial derivatives on the right side of this equation can be derived from Eq. (23). Substituting the two partial derivatives into this equation leads to
This equation indicates that in dry air when T=Ts, ΔT is equal to ΔTs if is measured accurately (i.e., while 0). However, air in the atmospheric boundary layer where CPEC systems are used is always moist. Given this equation, ΔT at Ts and can be evaluated by using ΔTs and , both of which are related to the measurement specifications of sonic anemometers for Ts (Campbell Scientific Inc., 2018b) and of infrared analyzers for (Campbell Scientific Inc., 2018a). Sonic anemometers and infrared analyzers with different models and brands have different specifications from their manufacturers. The manufacturer of the anemometer we studied (Fig. 1) employs carbon fiber with minimized thermo-expansion and thermo-contraction for sonic strut stability (via personal communication with CSAT structural designer Antoine Rousseau, 2021); structural design with optimized sonic volume for less aerodynamic disturbance (Fig. 1); and advanced proprietary sonic firmware for more accurate measurements (Zhou et al., 2018), which reduces the variability in Ts by several kelvins compared to what has been reported for sonics from other models (Mauder and Zeeman, 2018). Any combination of sonic and infrared instruments has a combination of ΔTs and , which are specified by their manufacturers. In turn, from Eq. (25), the combination generates ΔT of equation-computed T for the corresponding combination of the sonic and infrared instruments with given models and brands. Therefore, Eqs. (23) and (25) are applicable to any CPEC system beyond the brand of our study (Fig. 1). The applicability of Eq. (23) for any sonic or infrared instrument can be assessed based on ΔT against the required T accuracy for a specific application.
On the right side of Eq. (25), the first term with ΔTs can be expressed as (i.e., uncertainty portion of ΔT due to ΔTs) and the second term with can be expressed as (i.e., uncertainty portion of ΔT due to ). Using and , this equation can be simplified as
Assessment of the accuracy of equation-computed T is to evaluate and correspondingly from ΔTs and .
The CPEC system for this study is CPEC310 (Campbell Scientific Inc., UT, USA), whose major components are a CSAT3A sonic anemometer (updated version in 2016) for a fast response to 3-D wind and Ts and an EC155 infrared analyzer for a fast response to H2O along with CO2 (Burgon et al., 2015; Ma et al., 2017). The system operates in a T range of −30 to 50 ∘C and measures in a range up to 79 mmolH2O mol−1 (i.e., 37 ∘C dew point temperature at 86 kPa in manufacturer environment); therefore, the accuracy of equation-computed T, depending on ΔTs and , should be defined and estimated in a domain over both ranges.
4.1 ΔTs (measurement accuracy in Ts)
As is true for other sonic anemometers (e.g., Gill Instruments, 2004), the CSAT3A has not been assigned a Ts measurement performance (Campbell Scientific Inc., 2018b) because the theories and methodologies of how to specify this performance, to the best of our knowledge, have not been clearly defined. The performance of the CSAT series for Ts is best near the production temperature at around 20 ∘C and drifts a little away from this temperature. Within the operational range of a CPEC system in ambient air temperature, the updated version of CSAT3A has an overall uncertainty of ±1.00 ∘C (i.e., |ΔTs|<1.00 K, via personal communication with CSAT authority Larry Jacobsen through email in 2017 and in person in 2018).
4.2 (measurement accuracy in )
The accuracy in H2O measurements from infrared analyzers depends upon analyzer measurement performance. This performance is specified using four component uncertainties: (1) precision variability (), (2) maximum zero drift range with ambient air temperature (dwz), (3) maximum gain drift with ambient air temperature (±, where is the gain drift percentage), and (4) cross-sensitivity to CO2 (sc) (LI-COR Biosciences, 2016; Campbell Scientific Inc., 2018c). Zhou et al. (2021) composited the four component uncertainties as an accuracy model formulated as the H2O accuracy equation for CPEC systems applied in ecosystems, given by
where Tc is the ambient air temperature at which an infrared analyzer was calibrated by the manufacturer to fit its working equation or zeroed/spanned by a user in the field to adjust the zero/gain drift; subscripts rh and rl indicate the range of the highest and lowest values, respectively; and Trh and Trl are the highest and lowest T, respectively, over the operational range in T of CPEC systems. Given the infrared analyzer specifications – , sc, dwz, , Trl, and Trh – this equation can be used to estimate in Eq. (25) and eventually in Eq. (26) over the domain of T and .
4.3 ΔT (accuracy of equation-computed T)
The accuracy of equation-computed T can be evaluated using ΔTs and (Eq. 25), varying with T, Ts, and . Both T and Ts reflect air temperature, being associated with each other through (Eq. 23). Given , T can be calculated from Ts, and vice versa; therefore, for the figure presentations in this study, it is sufficient to use either T or Ts, instead of both, to show ΔT with air temperature. Considering T to be of interest to this study, T will be used. As such, ΔT can be analyzed over a domain of T and within the operational range in T of CPEC systems from −30 to 50 ∘C across the analyzer measurement range of from 0 to 0.079 molH2O mol−1.
To visualize the relationship of ΔT with T and , ΔT is presented better as the ordinate along T and as the abscissa associated with . However, due to the positive dependence of air water vapor saturation on T (Wallace and Hobbs, 2006), has a range that is wider at a higher T and narrower at a lower T. To present ΔT over the same measure of air moisture, even at different T values, the saturation water vapor pressure is used to scale air moisture to 0, 20, 40, 60, 80, and 100 (i.e., RH, relative humidity in %). For each scaled RH value, can be calculated at different T and P values (Appendix C) for use in Eq. (25). In this way, over the range of T, the trend of ΔT due to each measurement uncertainty source can be shown along the curves with equal RH as the measure of air moisture (Fig. 2).
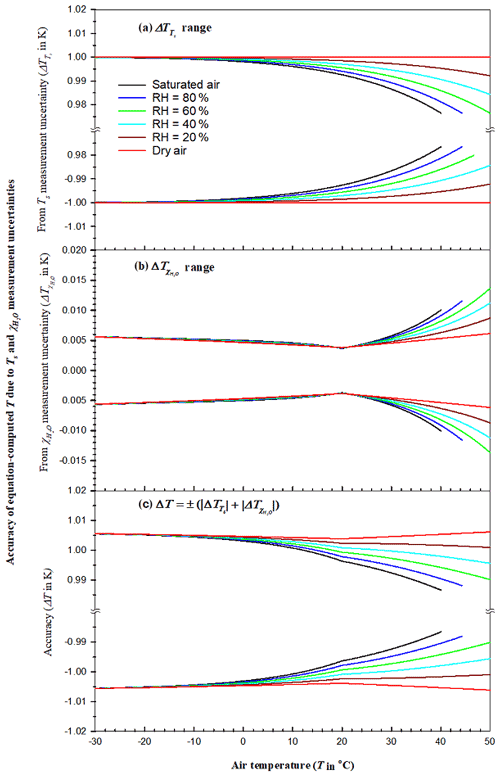
Figure 2Accuracy of air temperature computed from Eq. (23) (equation-computed T) over the measurement range of the H2O molar mixing ratio () within the operational range of T for the CPEC300 series (Campbell Scientific Inc., UT, USA): (a) accuracy component of equation-computed T due to sonic temperature (Ts) measurement uncertainty, (b) accuracy component of equation-computed T due to measurement uncertainty, and (c) overall accuracy of equation-computed T.
4.3.1 (uncertainty portion of ΔT due to ΔTs)
Given 1.00 K and Ts from the algorithm in Appendix C, in Eq. (26) was calculated over the domain of T and (Fig. 2a). Over the whole T range, the limits range ±1.00 K, becoming a little narrower with increasing due to a decrease, at the same Ts, in the magnitude of in Eq. (25). The narrowest limits of , in an absolute value, vary < 0.01 K over the range of T below 20 ∘C, although > 0.01 K but < 0.03 K above 20 ∘C.
4.3.2 (uncertainty portion of ΔT due to )
Given from the algorithm in Appendix C and from Eq. (27), was calculated over the domain of T and (Fig. 2b). The parameters in Eq. (27) are given through the specifications of the CPEC300 series (Campbell Scientific Inc., 2018a, c; is 6.0 × 10−6 molH2O mol−1, where mol is a unit (moles) for dry air; dwz, ±5.0 × 10−5 molH2O mol−1; , 0.30 %; sc, ±5.0 × 10−8 molH2O mol−1 (µmolCO2 mol; Tc, 20 ∘C as normal temperature – Wright et al., 2003; Trl, −30 ∘C; Trh, 50 ∘C).
As shown in Fig. 2b, tends to be smallest at T=Tc. However, away from Tc, its range nonlinearly becomes wider, very gradually widening below Tc but widening more abruptly above it because, as temperature increases, at the same RH increases exponentially (Eqs. C1 and C5 in Appendix C), while increases linearly with in Eq. (27). This nonlinear range can be summarized as ±0.01 K below 30 ∘C and ±0.02 K above 30 ∘C. Compared to , is much smaller by 2 orders of magnitude. is a larger component in ΔT.
4.3.3 ΔT (combined uncertainty as the accuracy in equation-computed T)
Equation (26) is used to determine the maximum combined uncertainty in equation-computed T for the same RH grade in Fig. 2 by adding together the same sign (i.e., ±) curve data of in Fig. 2a and in Fig. 2b. ΔT ranges at different RH grades are shown in Fig. 2c. Figure 2c specifies the accuracy of equation-computed T at 101.325 kPa (i.e., normal atmospheric pressure as used by Wright et al., 2003) over the measurement range to be within ±1.01 K. This accuracy for high-frequency T is currently the best in turbulent flux measurement because ±1.00 K is the best in terms of the accuracy of Ts from the individual sonic anemometers which are widely used for sensible heat flux in almost all CPEC systems.
4.4 Accuracy of equation-computed T from CPEC field measurements
Equation (23) is derived particularly for CPEC systems in which Ts and are measured neither at the same volume nor at the same time. Both variables are measured separately using a sonic anemometer and an infrared analyzer in a spatial separation between the Ts measurement center and the measurement cuvette (e.g., Fig. 1), along with a temporal lag in the measurement of relative to Ts due to the transport time and phase shift (Ibrom et al., 2007) of turbulent airflows sampled for through the sampling orifice to the measurement cuvette (Fig. 3).
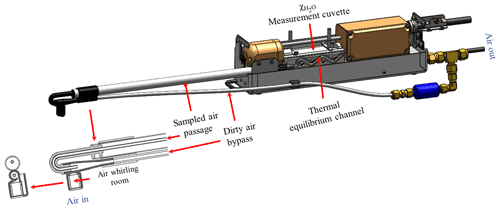
Figure 3Vortex intake system for airflow through its individual compartments: air whirling room (2.200 mL), sampled air passage (1.889 mL), thermal equilibrium channel (0.587 mL), and measurement cuvette (5.887 mL). The internal space of all compartments adds up to a total volume of 10.563 mL.
Fortunately, the spatial separation scale is tens of centimeters, and the temporal lag scale is tens of milliseconds. In eddy-covariance flux measurements, such a separation misses some covariance signals at a higher frequency, which is correctable (Moore, 1986), and such a lag diminishes the covariance correlation, which is recoverable (Ibrom et al., 2007). How such a separation along with the lag influences the accuracy of Eq. (23), as shown in Fig. 2, needs testing against precision measurements of air temperature. The two advantages of the equation-computed T discussed in the Introduction, namely the fast response to high-frequency signals and the insensitivity to solar contamination in measurements, were studied and assessed during testing when a CPEC system was set up in the Campbell Scientific instrument test field (41.8∘ N, 111.9∘ W; 1360 m a.s.l.; UT, USA).
5.1 Field test station
A CPEC310 system was set as the core of the station in 2018. Beyond its major components briefly described in Sect. 4, the system also included a barometer (model MPXAZ6115A, Freescale Semiconductor, TX, USA) for flow pressure; pump module (SN 1001) for air sampling; valve module (SN 1003) to control flows for auto-zero/span CO2 and H2O; scrub module (SN 1002) to generate zero air (i.e., without CO2 and H2O) for the auto-zero procedure; CO2 cylinder for CO2 span; and EC100 electronic module (SN 1002, OS Rev 07.01) to control and measure a CSAT3A, EC155, and barometer. In turn, the EC100 was connected to and instructed by a central CR6 datalogger (SN 2981, OS 04) for sensor measurements, data processing, and data output. In addition to receiving the data output from the EC100, the CR6 also controlled the pump, valve, and scrub modules and measured other micrometeorological sensors in support of this study.
The micrometeorological sensors included an LI-200 pyranometer (SN 18854, LI-COR Biosciences, NE, USA) to monitor incoming solar radiation, a precision platinum resistance temperature detector (RTD, model 41342, SN TS25360) inside a fan-aspirated radiation shield (model 43502, R.M. Young Company, MI, USA) to more accurately measure the T considered with minimized solar contamination due to higher fan-aspiration efficiency, and an HMP155A temperature and humidity sensor (SN 1073, Vaisala Corporation, Helsinki, Finland) inside a 14-plate wind-aspirated radiation shield (model 41005) to measure the T under conditions of potentially significant solar contamination during the day due to low wind-aspiration efficiency. The sensing centers of all sensors related to Ts, T, and RH were set at a height of 2.57 m above ground level. The land surface was covered by natural prairie with a grass height of 5 to 35 cm.
A CR6, supported by EasyFlux DL CR6CP (revised version for this study, Campbell Scientific Inc., UT, USA), controlled and sampled the EC100 at 20 Hz. For spectral analysis, the EC100 filtered the data of Ts and for anti-aliasing using a finite-impulse-response filter with a 0-to-10 Hz (Nyquist folding frequency) passing band (Saramäki, 1993). The EC155 was zeroed for CO2–H2O and spanned for CO2 automatically every other day and spanned for H2O monthly using an LI-610 Portable Dew Point Generator (LI-COR Biosciences, NE, USA). The LI-200, RTD, and HMP155A were sampled at 1 Hz because of their slow response and the fact that only their measurement means were of interest to this study.
The purpose of this station was to measure the eddy-covariance fluxes to determine turbulent transfers in the boundary-layer flows. The air temperature equation (i.e., Eq. 23) was developed for the T of the turbulent airflows sampled through the CPEC systems. Therefore, this equation can be tested based on how the CPEC310 measures the boundary-layer flows related to turbulent transfer.
5.2 Turbulent transfer and CPEC310 measurement
In atmospheric boundary-layer flows, air constituents along with heat and momentum (i.e., air properties) are transferred dominantly by individual turbulent flow eddies with various sizes (Kaimal and Finnigan, 1994). Any air property is considered to be more homogenous inside each smaller eddy and more heterogenous among larger eddies (Stull, 1988). Due to this heterogeneity, an eddy in motion among others transfers air properties to its surroundings. Therefore, to measure the transfer in amount and direction, a CPEC system was designed to capture Ts, , and 3-D flow speeds from individual eddies. Ideal measurements, although impossible, would be fast enough to capture all eddies with different sizes through the measurement volume and sampling orifice of the CPEC system (Fig. 1). To capture more eddies of as many sizes as possible, the CPEC measurements were set at a high frequency (20 Hz in this study) because, given 3-D speeds, the smaller the eddy, the shorter time the said eddy takes to pass the sensor measurement volume. Ideally, each measurement captures an individual eddy for all variables of interest so that the measured values are representative of this eddy. So, for instance, in our effort to compute T from a pair of Ts and values, the pair simultaneously measured from the same eddy could better reflect its T at the measurement time; however, in a CPEC system, Ts and are measured with separation in both space (Fig. 1) and time (Fig. 3).
If an eddy passing the sonic anemometer is significantly larger than the dimension of separation between the Ts measurement volume and the sampling orifice (Fig. 1), the eddy is instantaneously measured for its 3-D wind and Ts in the volume while also sampled in the orifice for measurements. However, if the eddy is smaller and flows along the alignment of separation, the sampling takes place either a little earlier or a little later than the measurement (e.g., earlier if Ts is measured later, and vice versa). However, depending on its size, an eddy flowing beyond the alignment from other directions, although measured by the sonic anemometer, may be missed by the sampling orifice passed by other eddies and, in other cases, although sampled by the orifice, may be missed by the measurement of the sonic anemometer.
Additionally, the airflow sampled for measurements is not measured at its sampling time on the sampling orifice but instead is measured, in lag, inside the measurement cuvette (Fig. 3). The lag depends on the time needed for the sampled flow to travel through the CPEC sampling system (Fig. 3). Therefore, for the computation of is better synchronized and matched with Ts as if they were simultaneously measured from the same eddy.
5.3 Temporal synchronization and spatial match for Ts with
In the CPEC310 system, a pair of Ts and values that were received by the CR6 from the EC100 in one data record (i.e., data row) were synchronously measured, through the Synchronous Device for Measurement communication protocol (Campbell Scientific Inc., 2018c), in the Ts measurement volume and measurement cuvette (Fig. 1). Accordingly, within one data row of time series received by the CR6, was sampled earlier than Ts was measured. As discussed above, Ts and in the same row, although measured at the same time, might not be measured from the same eddy. If so, the measurement from the same eddy of this Ts might occur in another data row, and vice versa. In any case, a logical procedure for a synchronized match is first to pair Ts with programmatically in the CR6, as the former was measured at the same time as the latter was sampled.
5.3.1 Synchronize Ts measured to sampled at the same time
Among the rows in time series received by the CR6, any two consecutive rows were measured sequentially at a fixed time interval (i.e., measurement interval). Accordingly, anemometer data in any data row can be synchronized with analyzer data in a later row from the eddy sampled by the analyzer sampling orifice at the measurement time of the sonic anemometer. How many rows later depends on the measurement interval and the time length of the analyzer sample from its sampling orifice to the measurement cuvette. The measurement interval commonly is 50 or 100 ms for a 20 or 10 Hz measurement frequency, respectively. The time length is determined by the internal space volume of the sampling system (Fig. 3) and the flow rate of sampled air driven by a diaphragm pump (Campbell Scientific Inc., 2018a).
As shown in Fig. 3, the total internal space is 10.563 mL. The rate of sampled air through the sampling system nominally is 6.0 L min−1, at which the sampled air takes 106 ms to travel from the analyzer sampling orifice to the cuvette exhaust outlet (Fig. 3). Given that the internal optical volume inside the cuvette is 5.887 mL, the air in the cuvette was sampled during a period of 47 to 106 ms earlier. Accordingly, anemometer data in a current row of time series should be synchronized with analyzer data in the next row for 10 Hz data and, for 20 Hz data, in the row after that. After synchronization, the CR6 stores anemometer and analyzer data in a synchronized matrix (variables unrelated to this study were omitted) as a time series:
where u and v are horizontal wind speeds orthogonal to each other; w is vertical wind speed; ds and dg are diagnosis codes for the sonic anemometer and infrared analyzer, respectively; s is the analyzer signal strength for H2O; t is time, and its subscript i is its index; and the difference between ti and ti+1 is a measurement interval ( − ti). In any row of the matrix (28) (e.g., the ith row), ti for anemometer data is the measurement time plus instrument lag, and ti for analyzer data is the sampling time plus the same lag. The instrument lag is defined as the number of measurement intervals used for data processing inside the EC100 after the measurement and subsequent data communication to the CR6. Regardless of instrument lag, Ts and in each row of the synchronization matrix were temporally synchronized as measured and sampled at the same time.
5.3.2 Match Ts measured to sampled from the same eddy
As discussed in Sect. 5.2, at either the Ts measurement or the sampling time, if an eddy is large enough to enclose both the Ts measurement volume and the sampling orifice (Fig. 1), Ts and in the same row of the synchronization matrix (28) belong to the same eddy; otherwise, they belong to different eddies. For any eddy size, it would be ideal if Ts could be spatially matched with as a pair for the same eddy; however, this match would not be possible for all Ts values simply because, in some cases, an eddy measured by the sonic anemometer might never be sampled by the sampling orifice, and vice versa (see Sect. 5.2). Realistically, Ts may be matched with overall with the most likelihood to as many pairs as possible for a period (e.g., an averaging interval).
The match will eventually lag either Ts or , relatively, in the synchronization matrix (28). The lag can be counted as an integer number (ls, where subscript s indicates the spatial separation causing lag) in measurement intervals, where ls is positive if an eddy flowed through the Ts measurement volume earlier, negative if it flowed through later, or zero if it flowed through the sampling orifice at the same time. This number is estimated through the covariance maximization (Irwin, 1979; Moncrieff et al., 1997; Ibrom et al., 2007; Rebmann et al., 2012). According to ls over an averaging interval, the data columns of the infrared analyzer over an averaging interval in the synchronization matrix (28) can be moved together up ls rows as positive, down ls rows as negative, or nowhere as zero to form a matched matrix:
For details on how to find ls, see EasyFlux DL CR6CP on https://www.campbellsci.com (last access: 11 December 2021). In the matched matrix (29), over an averaging interval, a pair of Ts and values in the same row can be assumed to be matched as if they were measured and sampled from the same eddy.
Using Eq. (23), the air temperature can now be computed using
where subscript ls for t indicates that spatially lagged is used for computation of T. In verification of the accuracy of equation-computed T and in assessments of its expected advantages of high-frequency signals insensitive to solar contamination in measurements, could minimize the uncertainties due to the spatial separation in measurements of Ts and between the Ts measurement volume and the sampling orifice (Fig. 1).
6.1 Verification of the accuracy of equation-computed T
The accuracy of equation-computed T was theoretically specified by Eqs. (25) to (27) and was estimated in Fig. 2c. This accuracy specifies the range of equation-computed T minus true T (i.e., ΔT). However, true T was not available in the field, but, as usual, precision measurements could be considered benchmarks to represent true T. In this study, T measured by the RTD inside a fan-aspirated radiation shield (TRTD) was the benchmark to compute ΔT (i.e., equation-computed T minus TRTD). If almost all ΔT values fall within the accuracy-specified range over a measurement domain of T and , the accuracy is correctly defined, and the equation-computed T is accurate as specified.
To verify that the accuracy over the domain is as large as possible, ΔT values in the coldest (January) and hottest (July) months were used as shown in Fig. 4 (−21 ∘C < T < 35.5 ∘C, and up to 20.78 mmolH2O mol−1 in a 30 min mean over both months). Out of 2976 ΔT values from both months, 44 values fell out of the specified accuracy range but were near the range line within 0.30 K. The ΔT values were 0.549 ± 0.281 K in January and 0.436 ± 0.290 K in July. Although these values were almost all positively away from the zero line due to either overestimation for Ts by the sonic anemometer within ±1.00 K accuracy or underestimation for TRTD by the RTD within ±0.20 K accuracy, the ranges are significantly narrower than the specified accuracy range of equation-computed T (Figs. 2c and 4).
It is common for sonic anemometers to have a systematic error in Ts of ±0.5 ∘C or a little greater, which is the reason that the Ts accuracy is specified by Larry Jacobsen (anemometer authority) to be ±1.0 ∘C for the updated CSAT3A. The fixed deviation in measurements of sonic path lengths is asserted as a source of bias in Ts (Zhou et al., 2018). This bias brings an error to equation-computed T. If the T equation were not exact as in Eqs. (4) and (5), there would be an additional equation error. In our study effort, this bias from fixed deviation is possibly around 0.5 ∘C. With this bias, the equation-computed T is still accurate, as specified by Eqs. (25) to (27), and even better.
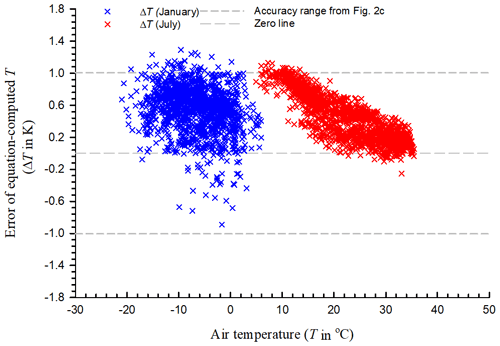
Figure 4The error in equation-computed T in the coldest (January) and hottest (July) months of 2019 in Logan, UT, USA. ΔT is equation-computed T minus RTD-measured T, where RTD denotes a precision platinum resistance temperature detector inside a fan-aspirated radiation shield. ΔT is 0.549 ± 0.281 K in January and 0.436 ± 0.290 K in July. See Fig. 2c for the accuracy range.
6.2 Assessments of the advantages of equation-computed T
As previously discussed, the data stream of equation-computed T consists of high-frequency signals insensitive to solar contamination in measurements. Its frequency response can be assessed against known high-frequency signals of Ts, and the insensitivity can be assessed by analyzing the equation-computed, RTD-measured, and sensor-measured T, where the sensor is an HMP155A inside a wind-aspirated radiation shield.
6.2.1 Frequency response
The matched matrix (29) and Eq. (30) were used to compute (i.e., equation-computed T). Paired power spectra of equation-computed T and Ts are compared in Fig. 5 for three individual 2 h periods of atmospheric stratifications, including unstable ( is −0.313 to −2.999, where z is a dynamic height of measurement minus displacement height and L is the Monin–Obukhov length), near-neutral ( is −0.029 to +0.003), and stable ( is +0.166 to +0.600). Slower response of equation-computed T than Ts at a higher frequency (e.g., > 5 Hz) was expected because equation-computed T is derived from two variables (Ts and ) measured in a spatial separation, which attenuates the frequency response of correlation of two measured variables (Laubach and McNaughton, 1998), and from a CPEC system has a slower response than Ts in terms of frequency (Ibrom et al., 2007). However, the expected slower response was not found in this study. In unstable and stable atmospheric stratifications (Fig. 5a and c), each pair of power spectra almost overlaps. Although they do not overlap in the near-neutral atmospheric stratification (Fig. 5b), the pairs follow the same trend slightly above or below one another. In the higher-frequency band of 1 to 10 Hz in Fig. 5a and b, equation-computed T has a little more power than Ts. The three pairs of power spectra in Fig. 5 indicate that equation-computed T has a frequency response equivalent to Ts up to 10 Hz, with a 20 Hz measurement rate considered to be a high frequency. The equivalent response might be accounted for by a dominant role of Ts in the magnitude of equation-computed T.
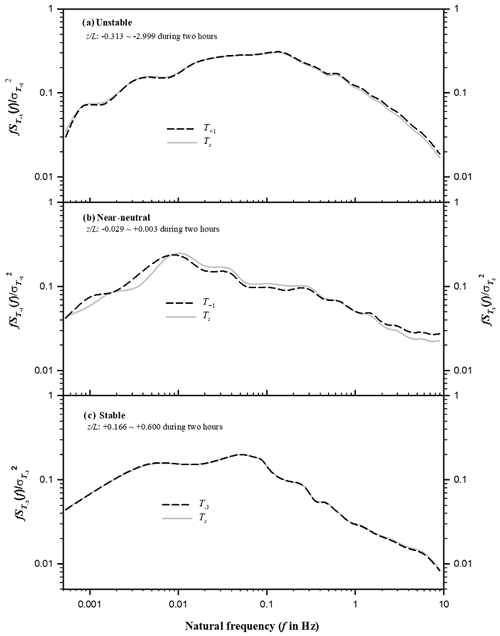
Figure 5Paired comparisons of power spectra for equation-computed air temperature (T) and sonic temperature (Ts) at each of three atmospheric stratifications: unstable (a), near-neutral (b), and stable (c). T+1 and T−3 are equation-computed T from Ts and the H2O mixing ratio of air sampled by the CPEC system through its sampling orifice in +1 lag (50 ms behind) and in −3 lags (150 ms ahead) of Ts measurement; z is the dynamic height of measurement minus the displacement height; L is the Monin–Obukhov length; , , and are the power spectra of Ts, T+1, and T−3 at f; and , , and represent the variance of Ts, T+1, and T−3.
6.2.2 Insensitivity to solar contamination in measurements
The data of equation-computed, sensor-measured, and RTD-measured T in July, during which incoming solar radiation (Rs) at the site was strongest in a yearly cycle, are used to assess the insensitivity of equation-computed T. From the data, ΔT is considered to be an error in equation-computed T. The error in sensor-measured T can be defined as sensor-measured T minus RTD-measured T, denoted by ΔTm. From Fig. 6, ΔT (0.690 ± 0.191 K) is > ΔTm (0.037 ± 0.199 K) when Rs < 50 W m−2 at lower radiation. However, ΔT (0.234 ± 0.172 K) is < ΔTm (0.438 ± 0.207 K) when Rs>50 W m−2 at higher radiation. This difference between ΔT and ΔTm shows a different effect of Rs on equation-computed and sensor-measured T.
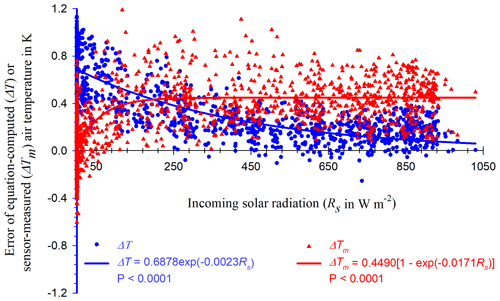
Figure 6Errors in equation-computed and sensor-measured air temperature (T) with incoming solar radiation. ΔT is equation-computed T minus RTD-measured T, where RTD denotes a precision platinum resistance temperature detector inside a fan-aspirated radiation shield. ΔTm is sensor-measured T minus RTD-measured T, where the sensor is an HMP155A air temperature and humidity probe inside a wind-aspirated radiation shield.
As shown in Fig. 6, ΔTm increases sharply with increasing Rs for Rs<250 W m−2, beyond which it asymptotically approaches 0.40 K. In the range of lower Rs, atmospheric stratification was likely stable (Kaimal and Finnigan, 1994), under which the heat exchange by wind was ineffective between the wind-aspirated radiation shield and boundary-layer flows. In this case, sensor-measured T was expected to increase with Rs increase (Lin et al., 2001; Blonquist and Bugbee, 2018). Along with Rs increase, the atmospheric boundary layer develops from stable to neutral or unstable conditions (Kaimal and Finnigan, 1994). During the stability change, the exchange becomes increasingly more effective, offsetting the further heating from Rs increase on the wind-aspirated radiation shield as indicated by the red asymptote portion in Fig. 6. Compared to the ΔTm mean (0.037 K) while Rs<50 W m−2, the magnitude of the asymptote above the mean is the overestimation of sensor-measured T due to solar contamination.
However, ΔT decreases asymptotically from about 0.70 K toward zero with the increase in Rs from 50 to 250 W m−2 and beyond, with a more gradual rate of change than ΔTm at the lower radiation range. Lower Rs (e.g., < 250 W m−2) concurrently occurs with lower T, higher RH, and/or unfavorable weather to Ts measurements. Under lower T (e.g., below 20 ∘C of normal CSAT3A manufacturing conditions), the sonic path lengths of CSAT3A (Fig. 1) must become, due to thermo-contraction of sonic anemometer structure, shorter than those at 20 ∘C. As a result, the sonic anemometer could overestimate the speed of sound (Zhou et al., 2018) and, hence, Ts for equation-computed T, resulting in greater ΔT with lower Rs. Under higher RH conditions, dew may form on the sensing surface of the six CSAT3A sonic transducers (Fig. 1). The dew, along with unfavorable weather, could contaminate the Ts measurements, resulting in ΔT greater in magnitude. Higher Rs (e.g., > 250 W m−2) concurrently occurs with weather favorable to Ts measurements, which is the reason that ΔT slightly decreases rather than increases with Rs when Rs > 250 W m−2.
Again from Fig. 6, the data pattern of ΔT>ΔTm in the lower Rs range and ΔT<ΔTm in the higher Rs range shows that equation-computed T is not as sensitive to Rs as sensor-measured T. The decreasing trend of ΔT with Rs increase shows the insensitivity of equation-computed T to Rs. Although the purpose of this study is not particularly to eliminate solar radiation contamination, equation-computed T is indeed less contaminated by solar radiation, as shown in Fig. 6.
7.1 Actual accuracy
The range of ΔT curves for each RH level in Fig. 2 is the maximum at that level because the data were evaluated using the maximized measurement uncertainties from all sources. Accordingly, in field applications under weather favorable to Ts measurements, the range of actual accuracy in equation-computed T can be reasonably inferred to be narrower. In our study case as shown in Figs. 4 and 6, the variability in ΔT was narrower than the accuracy range as specified in Fig. 2. In other words, the actual accuracy is better.
However, under weather conditions unfavorable to Ts measurements, such as dew, rain, snow, or dust storms, the accuracy of Ts measurements cannot be easily evaluated. Ts measurements also possibly have a systematic error due to the fixed deviation in the measurements of sonic path lengths for sonic anemometers, although the error should be within the accuracy specified in Fig. 2. A measurement can also be erroneous if the infrared analyzer is not periodically zeroed and spanned for its measurement environment. Therefore, if Ts is measured under unfavorable weather conditions and the sonic anemometer produces a systematic Ts error and if the infrared analyzer is not zeroed and spanned as instructed in its manual, then the accuracy of equation-computed T would be unpredictable. Normally, the actual accuracy is better than that specified in Fig. 2. Additionally, with the improvement in measurement accuracies of sonic anemometers (e.g., weather-condition-regulated, heated, 3-D sonic anemometers; Mahan et al., 2021) and infrared analyzers, this accuracy of equation-computed T would gradually become better.
For this study, filtering out the Ts data in the periods of unfavorable weather could narrow the error range of equation-computed T. The unfavorable weather was suspected of contributing to the stated error. However, although filtering out unfavorable weather cases could create a lower error estimate, most field experiments include periods when weather increases a Ts error, so including a weather contribution to error would prevent overstating instrument accuracy under typical (unfiltered) applications. Therefore, both Ts and data in this study were not programmatically or manually filtered based on weather.
7.2 Spatial separation of Ts and in measurements
In this study, T was successfully computed from Ts and as a high-frequency signal (Fig. 5) with expected accuracy as tested in Figs. 2, 4, and 6, where both were measured separately from two sensors in a spatial separation. Some open-path eddy-covariance (OPEC) flux systems (e.g., CSAT3A + EC150 and CSAT3B + LI-7500) measure Ts and ρw also using two sensors in a spatial separation. To OPEC systems, although the air temperature equation (Eq. 23) is not applicable, the algorithms developed in Sect. 5.3 to temporally synchronize and spatially match Ts with for computation of T are applicable for computation of T from Ts and ρw along with P in such OPEC systems (Swiatek, 2018).
In Sect. 5.3, programming and computing are needed to pair Ts measured to sampled at the same time into the synchronization matrix (28) as the first step and from the same eddy into matched matrix (29) as the second step. The second requires complicated programming and much computing. To test the necessity of this step in specific cases, using Eq. (30), T0i was computed from a row of the synchronization matrix, and was computed from this matrix by lagging columns up ls rows if ls>0 and down rows if ls<0, where ls is 1 and 5. From the data of this study, individual values were different for different subscript ls values, but their means for subscript i over an averaging interval are the same to at least the fourth digit after the decimal place. Further, the power spectrum of T0i time series was compared to those of time series, where ls≠0. Any pair of power spectra from the same period overlaps exactly (figures omitted). Therefore, the second step of lag maximization to match Ts measured to sampled from the same eddy is not needed if only the hourly mean and power spectrum of equation-computed T are of interest to computations, for both CPEC and OPEC systems.
7.3 Applications
The air temperature equation (Eq. 23) is derived from first principles without any assumption and approximation. It is an exact equation from which T can be computed in CPEC systems as a high-frequency signal insensitive to solar radiation. These merits, in additional to its consistent representation of spatial measurement and temporal synchronization scales with other thermodynamic variables for boundary-layer turbulent flows, will be particularly needed for advanced applications. The EasyFlux series is one of the two most popular field eddy-covariance flux software packages used in the world, the other being EddyPro (LI-COR Biosciences, 2015). Currently, it has used equation-computed T for ρd in Eq. (1), sensible heat flux (H), and RH as a high-frequency signal in CPEC systems (Campbell Scientific Inc., 2018a).
7.3.1 Dry-air density
As a high-frequency signal insensitive to solar radiation, equation-computed T is more applicable than sensor-measured T for calculations of and for advanced applications (Gu et al., 2012; Foken et al., 2012). In practice, equation-computed T can surely be used for and under normal weather conditions while the sonic anemometer and infrared analyzer are normally running, which can be judged by their diagnosis codes (Campbell Scientific Inc., 2018a). Under a weather condition unfavorable to Ts measurements, such as dew, rain, snow, and/or ice, equation-computed T from weather-condition-regulated, heated, 3-D sonic anemometers (Mahan et al., 2021) and infrared analyzers could be an alternative.
Currently, in CO2, H2O, and trace gas flux measurements, for flux calculations is estimated from T and RH along with P. T and RH are measured mostly by a slow-response T–RH probe without fan aspiration (e.g., HMP155A; Zhu et al., 2021). As shown in Fig. 6, equation-computed T is better than probe-measured T. The air moisture measured by an infrared analyzer in CPEC systems must be more accurate (Eq. 27 and Fig. 2b) than probe-measured air moisture. The better equation-computed T along with more accurate air moisture has no reason not to improve the estimation for .
7.3.2 Sensible heat flux estimated from a CPEC system
Currently, beyond the EasyFlux DL CR6CP series, H is derived from with a humidity correction (van Dijk, 2002). The correction equations were derived by Schotanus et al. (1983) and van Dijk (2002) in two ways, but both were derived with the approximation from Eq. (4) (see Appendix A). Using the exact equation from this study, theoretically H can be more accurately estimated directly from , where T is the equation-computed air temperature, although more studies and tests for this potential application are needed. Without our exact T equation, in any flux software, either Eq. (4) or Eq. (5) must be used for H computation. Both equations are approximate (see Appendices A and B). Compared to either, our exact equation must be an improvement on the mathematical representation of H. If the equation for sensible heat flux is approximate, then even a perfect measurement gives only an approximate value for the flux.
7.3.3 RH as a high-frequency signal
Conventionally, RH is measured using a T–RH probe, which is unable to track the high-frequency fluctuations in RH. In a CPEC system, equation-computed T, analyzer-measured , and transducer-measured P are able to catch the fluctuations in these variables at a high frequency, from which RH can be computed (Sonntag, 1990; also see Appendix C). This method should provide high-frequency RH, although verification for a frequency response is needed. Currently, the applications of high-frequency properties in this RH are unknown in a CPEC system. Regardless, equation-computed T provides a potential opportunity to acquire the high-frequency RH for its application in the future.
In a CPEC flux system, the air temperature (T) of boundary-layer flows through the space of sonic anemometer measurement and infrared analyzer sampling (Fig. 1) is desired for a high frequency (e.g., 10 Hz) with consistent representation of spatial and temporal scales for moist turbulence thermodynamics characterized by three-dimensional wind from the sonic anemometer and H2O–CO2 and atmospheric pressure from the infrared analyzer. High-frequency T in the space can be measured using fine-wire thermocouples, but this kind of thermocouple for such an application is not durable under adverse climate conditions, being easily contaminated by solar radiation (Campbell, 1969). Nevertheless, the measurements of sonic temperature (Ts) and H2O inside a CPEC system are high-frequency signals. Therefore, high-frequency T can be reasonably expected when computed from Ts and H2O-related variables. For this expectation, two equations (i.e., Eqs. 4 and 5) are currently available. In both equations, converting H2O-related variables into H2O mixing ratios analytically reveals the difference between the two equations. This difference in CPEC systems reaches ±0.18 K, bringing an uncertainty into the accuracy of T from either equation and raising the question of which equation is better. To clarify the uncertainty and answer this question, the air temperature equations in terms of Ts and H2O-related variables are thoroughly reviewed (Sects. 2 and 3, Appendices A and B). The two currently used equations (i.e., Eqs. 4 and 5) were developed and completed with approximations (Appendices A and B). Because of the approximations, neither of their accuracies was evaluated, nor was the question answered.
Using the first-principles equations, the air temperature equation in terms of Ts and (H2O molar mixing ratio) is derived without any assumption and approximation (Eq. 23); therefore, the equation derived in this study does not itself have any error, and, as such, the accuracy in equation-computed T depends solely on the measurement accuracies of Ts and . Based on the specifications for Ts and in the CPEC300 series, the accuracy of equation-computed T over the Ts and measurement ranges can be specified within ±1.01 K (Fig. 2). This accuracy range is propagated mainly (±1.00 K) from the uncertainty in Ts measurements (Fig. 2a) and a little (±0.02 K) from the uncertainty in measurements (Fig. 2b).
Under normal sensor and weather conditions, the specified accuracy is verified based on field data as valid, and actual accuracy is better (Figs. 4 and 6). Field data demonstrate that equation-computed T values under unstable, near-neutral, and stable atmospheric stratifications all have frequency responses equivalent to high-frequency Ts up to 10 Hz at a 20 Hz measurement rate (Fig. 5), being insensitive to solar contamination in measurements (Fig. 6).
The current applications of equation-computed T in a CPEC system are to calculate dry-air density (ρd) for the estimations of CO2 flux (, where is the CO2 mixing ratio, w is vertical velocity of air, the prime indicates the fluctuation of the variable away from its mean, and the overbar implies the mean), H2O flux (), and other fluxes. Combined with measurements of , 3-D wind speeds, and P, the equation-computed T can be applied to the estimation of and if needed (Gu et al., 2012; Foken et al., 2012), to the computation of high-frequency RH (Sonntag, 1990), and to the derivation of sensible heat flux (H) avoiding the humidity correction as needed for H indirectly from Ts (Schotanus et al., 1983; van Dijk, 2002).
In a CPEC flux system, although Ts and are measured using two spatially separated sensors of a sonic anemometer and infrared analyzer, T was successfully computed from both measured variables as a high-frequency signal (Fig. 5) with an expected accuracy (Figs. 2 and 4). Some open-path eddy-covariance (OPEC) flux systems measure Ts and water vapor density (ρw) also using two sensors in a similar way. The algorithms developed in Sect. 5.3 to temporally synchronize and spatially match Ts with for computation of T are applicable to such OPEC systems to compute T from Ts and ρw along with P. This T would be a better option than sensor-measured T in the systems for the correction of the spectroscopic effect in measuring CO2 fluctuations at high frequencies (Helbig et al., 2016; Wang et al., 2016). With the improvements in measurement technologies for Ts and , particularly for Ts, the T from our developed equation will become increasingly more accurate. Having its accuracy combined with its high frequency, this T with consistent representation of all other thermodynamic variables for moist air at the spatial and temporal scales in CPEC measurements has its advanced merits in boundary-layer meteorology and applied meteorology.
The sonic temperature (Ts) reported by a three-dimensional sonic anemometer is internally calculated from its measurements of the speed of sound in moist air (c) after the crosswind correction (Zhou et al., 2018), using
where subscript d indicates dry air, γd is the specific heat ratio of dry air between constant pressure and constant volume, and Rd is the gas constant for dry air (Campbell Scientific Inc., 2018b). The speed of sound in the atmospheric boundary layer as in a homogeneous gaseous medium is well defined in acoustics (Barrett and Suomi, 1949), given by
where γ is the counterpart of γd for moist air, P is atmospheric pressure, and ρ is moist-air density. These variables are related to air temperature and air specific humidity (q, i.e., the mass ratio of water vapor to moist air).
A1 Moist-air density (ρ)
Moist-air density is the sum of dry-air and water vapor densities. Based on the ideal gas law (Wallace and Hobbs, 2006), dry-air density (ρd) is given by
where e is water vapor pressure, and the water vapor density (ρw) is given by
where Rv is the gas constant for water vapor. Therefore, moist-air density in Eq. (A2) can be expressed as
Because (i.e., 0.622, the molar mass ratio between water vapor and dry air), this equation can be rearranged as
Using Eqs. (A4) and (A6), the air specific humidity can be expressed as
Because , q can be approximated as
Substituting this relation into Eq. (A6) generates
A2 Specific heat ratio of moist air (γ)
The specific heat ratio of moist air is determined by two moist-air properties: (1) the specific heat at constant pressure (Cp) and (2) specific heat at constant volume (Cv). Cp varies with the air moisture content between the specific heat of dry air at constant pressure (Cpd) and the specific heat of water vapor at constant pressure (Cpw). It must be the average of Cpd and Cpw that is arithmetically weighted by the dry air mass and water vapor mass, respectively, given by (Stull, 1988)
Cv can be similarly determined:
where Cvd is the specific heat of dry air at constant volume and Cvw is the specific heat of water vapor at constant volume. Denoting as γd, Eqs. (A10) and (A11) are used to express γ as
A3 Relation of sonic temperature to air temperature
Substituting Eqs. (A9) and (A12) into Eq. (A2) leads to
Using this equation to replace c2 in Eq. (A1), Ts is expressed as
Given Cpw=1952, Cpd=1004, Cvw=1463, and Cvd=717 J K−1 kg−1 (Wallace and Hobbs, 2006), this equation becomes
Expression of the last two parenthesized terms on the right side of this equation separately as Taylor series of q (Burden and Faires, 1993) by dropping, due to q≪1, the second-or-higher-order terms related to q leads to
On the right side of this equation, the three parenthesized terms can be expanded into a polynomial of q of the third order. Also due to q≪1 in this polynomial, the terms of q of the second or third order can be dropped. Further arithmetical manipulations result in
This is Eq. (4) in a different form. In its derivations from Eqs. (A1) and (A2), three approximation procedures were used from Eqs. (A7) to (A8), (A15) to (A16), and (A16) to (A17). The three approximations must bring unspecified errors into the derived equation.
Equation (5) was sourced from Ishii (1935) in which the speed of sound in moist air (c) was expressed in his Eq. (1) as
where all variables in this equation are for moist air, γ is the specific heat ratio of moist air between constant pressure and constant volume, P is moist-air pressure, ρ is moist-air density, α is the moist-air expansion coefficient, and β is the moist-air pressure coefficient. Accordingly, the speed of sound in dry air (cd) is given by
where subscript d indicates dry air in which γd, Pd, ρd, αd, and βd are the counterparts of γ, P, ρ, α, and β in moist air. Equations (B1) and (B2) can be combined as
Experimentally by Ishii (1935), each term inside the three pairs of parentheses in this equation was linearly related to the ratio of water vapor pressure (e) to dry-air pressure (Pd). Substituting the relationship into Eq. (B3) leads to
The three parenthesized terms in this equation sequentially correspond to the three parenthesized terms in Eq. (B3). Dividing γdRd, where Rd is the gas constant for dry air, over both sides of Eq. (B4) and referencing Eq. (11), sonic temperature (Ts) is expressed in terms of air temperature (T), e, and Pd as
Using the relationship of Pd=P–e, this equation can be manipulated as
Dropping the second-order terms due to in boundary-layer flows, this equation becomes
Expanding the second parenthesized term into Taylor series and, also due to , dropping the terms related to of an order of 2 or higher, this equation becomes
Further expanding the two parenthesized terms on the right side of this equation and dropping the second-order term of led to
This is Eq. (5) in a different form. From the experimental source of Eq. (B4), it was derived using three approximations from Eqs. (B4) to (B7), (B7) to (B8), and (B8) to (B9). The approximations and therefore combined uncertainty in T bring unspecified errors into Eq. (5) (i.e., Eq. B9) as an equation error.
For a given air temperature (T in ∘C) and atmospheric pressure (P in kPa), air has a limited capacity to hold water vapor (Wallace and Hobbs, 2006). This limited capacity is described in terms of saturation water vapor pressure (es in kPa) for moist air, given through the Clausius–Clapeyron equation (Sonntag, 1990):
where f(P) is an enhancement factor for moist air, being a function of atmospheric pressure: . At relative humidity (RH in %), the water vapor pressure (eRH(T,P) in kPa) is
Given the mole numbers of H2O (nRH) and dry air (nd) at RH, the H2O molar mixing ratio at RH ( is
where R∗ is the universal gas constant and Pd is dry-air pressure in kilopascals. Using this equation and the relation
can be expressed as
Using Eq. (23), this along with T can be used to calculate sonic temperature (Ts in K) at RH, given by
where ε=0.622 (Eq. 17), γv=2.04045, and γp=1.94422 (Eq. 23). Through Eqs. (C1) and (C2), Eqs. (C5) and (C6) express and , respectively, in terms of T, RH, and P. and can be used to replace (H2O molar mixing ratio) and Ts in Eq. (25). After replacements, Eq. (25) can be used to evaluate the uncertainty, due to Ts and measurement accuracy uncertainties, in air temperature computed from Eq. (23) for different RH values over a T range.
Code is available at https://www.campbellsci.com/downloads?b=5 (last access: 18 December 2021, Campbell Scientific Inc., 2018a).
Data are available at https://datadryad.org/stash/share/ZiwOBaIBtu85UQ2kFye2LCtkzgp6l_UFg7dMeFi52ww (Zhou and Gao, 2021) via the following files: Data_Fig2a.xlsx, Data_Fig2b.xlsx, Data_Fig2c.xlsx, Data_Fig4.xlsx, and Data_Fig6.xlsx.
XinZ and TG developed the manuscript; ET substantially structured and revised the manuscript; XiaoZ analyzed time series data; AS, TA, and JO made comments on the manuscript; and JZ led the team.
Xinhua Zhou is affiliated with Campbell Scientific Inc., whose products were used in this research. The authors have no other competing interests to declare.
Publisher’s note: Copernicus Publications remains neutral with regard to jurisdictional claims in published maps and institutional affiliations.
The authors thank the anonymous reviewers for their professional review, understanding of our study topic, and constructive comments on the manuscript for significant improvement; Brittney Smart for her professional and dedicated proofreading; Rex Burgon for his advice about the technical design of a CPEC sampling system; and Edward Swiatek for his installing of the CPEC system in the Campbell Scientific instrument test field.
This research has been supported by the Bureau of Development and Planning, Chinese Academy of Sciences (grant no. XDA19030204); Research and Development, Campbell Scientific Inc. (project no. 14433); the Bureau of International Co-operation, Chinese Academy of Sciences (grant no. 2020VBA0007); Chinese Academy of Sciences President's International Fellowship Initiative, Chinese Academy of Sciences (grant no. 2020VBA0007); and Long-Term Agroecosystem Research, USDA (award no. 58-3042-9-014).
This paper was edited by Keding Lu and reviewed by two anonymous referees.
AmeriFlux: Data Variables, Lawrence Berkeley National Laboratory, 1–12, available at: http://ameriflux.lbl.gov/data/aboutdata/data-variables/ (last access: 11 December 2021), 2018.
Apogee Instruments Inc.: Owner's Manual: Aspirated Radiation Shield (model: TS-100), Logan, UT, USA, 19 pp., 2013.
Aubinet, M., Vesala, T., and Papale, D. (Eds.): Eddy Covariance: A Practice Guide to Measurement and Data Analysis, Springer, NY, USA, 438 pp., https://doi.org/10.1007/978-94-007-2351-1, 2012.
Barrett, E. W. and Suomi, V. E.: Preliminary report on temperature measurement by sonic means, J. Atmos. Sci., 6, 273–276, https://doi.org/10.1175/1520-0469(1949)006<0273:PROTMB>2.0.CO;2, 1949.
Blonquist, J. M. and Bugbee, B.: Air temperature, in: Agroclimatology: Linking Agriculture to Climate, Agronomy Monographs, edited by: Hatfield, J., Sivakumar, M., and Prueger, J., American Society of Agronomy, Crop Science Society of America, and Soil Science Society of America, Inc., Madison, WI, USA, https//doi.org/10.2134/agronmonogr60.2016.0012, 2018.
Burden, R. L. and Faires, J. D.: Numerical Analysis, 5th Edn., PWS Publishing Company, Boston, MA, USA, 768 pp., 1993.
Burgon Jr., R. P., Sargent, S., Zha, T., and Jia, X.: Field performance verification of carbon dioxide, water, and nitrous oxide closed-path eddy covariance systems with vortex intakes, in: AGU Fall Meeting Abstracts, San Francisco, CA, USA, 14–18 December 2015, B33C-0669, 2015.
Campbell, G. S.: Measurement of air temperature fluctuations with thermocouples, Atmospheric Sciences Laboratory, White Sands Missile Range, NM, USA, ECOM-5273, 17 pp., 1969.
Campbell Scientific Inc.: Model ASPTC Aspirated Shield with Fine Wire Thermocouple, Revision 6/10, Logan, UT, USA, 8 pp., 2010.
Campbell Scientific Inc.: CPEC300/306/310 Closed-Path Eddy-Covariance Systems, Revision 10/18, Logan, UT, USA, 8 pp., 2018a.
Campbell Scientific Inc.: CSAT3B Three-Dimensional Sonic Anemometer, Revision 3/18, Logan, UT, USA, 58 pp., 2018b.
Campbell Scientific Inc.: EC155 Closed-Path Gas Analyzer, Revision 7/18, Logan, UT, USA, 5–7, 2018c.
Foken, T., Aubinet, M., and Leuning, R.: The eddy covariance method, in: Eddy Covariance: A Practical Guide to Measurement and Data Analysis, edited by: Aubinet, M., Vesala, T., and Papale, D., Springer Netherlands, Dordrecht, 1–19, https://doi.org/10.1007/978-94-007-2351-1_1, 2012.
Gill Instruments: Horizontally Symmetrical Research Ultrasonic Anemometer: User Manual, document number: 1199-PS-0003, Issue 08, Hampshire, UK, 70 pp., 2004.
Gu, L., Massman, W. J., Leuning, R., Pallardy, S. G., Meyers, T., Hanson, P. J., Riggs, J. S., Hosman, K. P., and Yang, B.: The fundamental equation of eddy covariance and its application in flux measurements, Agr. Forest Meteorol., 152, 135–148, https://doi.org/10.1016/j.agrformet.2011.09.014, 2012.
Harrison, R. G. and Burt, S. D.: Quantifying uncertainties in climate data: measurement limitations of naturally ventilated thermometer screens, Environ. Res. Commun., 3, 1–10, https://doi.org/10.1088/2515-7620/ac0d0b, 2021.
Helbig, M., Wischnewski, K., Gosselin, G. H., Biraud, S. C., Bogoev, I., Chan, W. S., Euskirchen, E. S., Glenn, A. J., Marsh, P. M., Quinton, W. L., and Sonnentag, O.: Addressing a systematic bias in carbon dioxide flux measurements with the EC150 and the IRGASON open-path gas analyzers, Agr. Forest Meteorol., 228–229, 349–359, https://doi.org/10.1016/j.agrformet.2016.07.018, 2016.
Horst, T. W. and Lenschow, D. H.: Attenuation of scalar fluxes measured with spatially-displaced sensors, Bound.-Lay. Meteorol., 130, 275–300, https://doi.org/10.1007/s10546-008-9348-0, 2009.
Ibrom, A., Dellwik, E., Flyvbjerg, H., Jensen, N. O., and Pilegaard, K.: Strong low-pass filtering effects on water vapour flux measurements with closed-path eddy correlation systems, Agr. Forest Meteorol., 147, 140–156, https://doi.org/10.1016/j.agrformet.2007.07.007, 2007.
International Organization for Standardization: Accuracy (trueness and precision) of measurement methods and results – Part 1: General principles and definitions, ISO 5725-1, 1994 (reviewed in 2012), Geneva, Switzerland, 17 pp., 2012.
Irwin, H. P. A. H.: Cross-spectra of turbulence velocities in isotropic turbulence, Bound.-Lay. Meteorol., 16, 237–243, https://doi.org/10.1007/BF03335368, 1979.
Ishii, C.: Supersonic velocity in gases: especially in dry and humid air, Scientific Papers of the Institute of Physical and Chemical Research, Institute of Physical and Chemical Research, Tokyo, Japan, 26, 201–207, 1935.
Kaimal, J. C. and Businger, J. A.: A continuous wave sonic anemometer-thermometer, J. Appl. Meteorol., 2, 156–164, https://doi.org/10.1175/1520-0450(1963)0022.0.CO;2, 1963.
Kaimal, J. C. and Finnigan, J. J. (Eds.): Atmospheric Boundary Layer Flows: Their Structure and Measurement, Oxford University Press, Oxford, 289 pp., 1994.
Kaimal, J. C. and Gaynor, J. E.: Another look at sonic thermometry, Bound.-Lay. Meteorol., 56, 401–410, https://doi.org/10.1007/BF00119215, 1991.
Laubach, J. and McNaughton, K. G.: A spectrum-independent procedure for correcting eddy fluxes measured with separated sensors, Bound.-Lay. Meteorol., 89, 445–467, https://doi.org/10.1023/A:1001759903058, 1998.
Lee, X. and Massman, W. J.: A perspective on thirty years of the Webb, Pearman, and Leuning density corrections, Bound.-Lay. Meteorol., 139, 37–59, https://doi.org/10.1007/s10546-010-9575-z, 2011.
LI-COR Biosciences: EddyPro® Eddy Covariance Software: Instruction Manual, Lincoln, NE, USA, 1-1–10-6, 2015.
LI-COR Biosciences: LI-7500RS Open Path Gas Analyzer: Instruction Manual, Lincoln, NE, USA, 4-1–11 and 8-1–9, 2016.
Lin, X., Hubbard, K. G., Walter-Shea, E. A., Brandle, J. R., and Meyer, G. E.: Some perspectives on recent in situ air temperature observations: modeling the microclimate inside the radiation shields, J. Atmos. Ocean. Tech., 18, 1470–1484, https://doi.org/10.1175/1520-0426(2001)018<1470:SPORIS>2.0.CO;2, 2001.
Liu, H., Peters, G., and Foken, T.: New equations for sonic temperature variance and buoyancy heat flux with an omnidirectional sonic anemometer, Bound.-Lay. Meteorol., 100, 459–468, https://doi.org/10.1023/A:1019207031397, 2001.
Ma, J., Zha, T., Jia, X., Sargent, S., Burgon, R., Bourque, C. P.-A., Zhou, X., Liu, P., Bai, Y., and Wu, Y.: An eddy-covariance system with an innovative vortex intake for measuring carbon dioxide and water fluxes of ecosystems, Atmos. Meas. Tech., 10, 1259–1267, https://doi.org/10.5194/amt-10-1259-2017, 2017.
Mahan, H., Gao, T., Li, X., Forbush, T., Payne, K., Yang, Q., Li, Y., Zhou, H., Wu, S., Zheng, N., and Zhou, X.: Weather-condition-regulated, heated 3-D sonic anemometers (CSAT3AH and CSAT3BH): Working rationale, operation algorithm, and performance assessment, EGU General Assembly 2021, online, 19–30 Apr 2021, EGU21-13671, https://doi.org/10.5194/egusphere-egu21-13671, 2021.
Mauder, M. and Zeeman, M. J.: Field intercomparison of prevailing sonic anemometers, Atmos. Meas. Tech., 11, 249–263, https://doi.org/10.5194/amt-11-249-2018, 2018.
Moncrieff, J. B., Massheder, J. M., de Bruin, H., Elbers, J., Friborg, T., Heusinkveld, B., Kabat, P., Scott, S., Soegaard, H., and Verhoef, A.: A system to measure surface fluxes of momentum, sensible heat, water vapour and carbon dioxide, J. Hydrol., 188–189, 589–611, https://doi.org/10.1016/S0022-1694(96)03194-0, 1997.
Moore, C. J.: Frequency response corrections for eddy correlation systems, Bound.-Lay. Meteorol., 37, 17–35, https//doi.org/10.1007/BF00122754, 1986.
Munger, J. W., Loescher, H. W., and Luo, H.: Measurement, tower, and site design considerations, in: Eddy Covariance: A Practical Guide to Measurement and Data Analysis, edited by: Aubinet, M., Vesala, T., and Papale, D., Springer Netherlands, Dordrecht, 21–58, https://doi.org/10.1007/978-94-007-2351-1_2, 2012.
Panofsky, H. A. and Dutton, J. A. (Eds.): Atmospheric Turbulence: Model and Methods for Engineering Applications, John Wiley & Sons, NY, USA, 397 pp., 1984.
Rebmann, C., Kolle, O., Heinesch, B., Queck, R., Ibrom, A., and Aubinet, M.: Data acquisition and flux calculations, in: Eddy Covariance: A Practical Guide to Measurement and Data Analysis, edited by: Aubinet, M., Vesala, T., and Papale, D., Springer Netherlands, Dordrecht, 59–83, https://doi.org/10.1007/978-94-007-2351-1_3, 2012.
R.M. Young Company: Compact Aspirated Radiation Shield: Model 43502, Traverse City, MI, USA, 1–4, 2004.
Saramäki, T.: Finite impulse response filter design, in: Handbook for Digital Signal Processing, edited by: Mitra, K. S. and Kaiser, J. F., John Wiley & Sons, Inc., NY, USA, 155–277, 1993.
Schotanus, P., Nieuwstadt, F. T. M., and de Bruin, H. A. R.: Temperature measurement with a sonic anemometer and its application to heat and moisture fluxes, Bound.-Lay. Meteorol., 26, 81–93, https//doi.org/10.1007/BF00164332, 1983.
Snedecor, G. W. and Cochran, W. G. (Eds.): Statistical Methods, 8th Edn., Iowa State University Press, Ames, IA, USA, 502 pp., 1989.
Sonntag, D.: Important new values of the physical constants of 1986, vapour pressure formulations based on the ITS-90, and psychrometer formulae, Z. Meteorol., 40, 340–344, 1990.
Stull, R. B. (Ed.): An Introduction to Boundary Layer Meteorology, Kluwer Academic Publisher, Dordrecht, 35–437, 1988.
Swiatek, E.: Derivation of the Calculated Sensible Heat Flux (Hc) from the Sonic Sensible Heat Flux (Hs) and Latent Heat Flux (LE), Campbell Scientific Inc., Logan, UT, USA, 1–2, 2009.
Swiatek, E: Derivation of Temperature (Tc) from the Sonic Virtual Temperature (Ts), Vapor Density (ρv)/Vapor Pressure (e) and Pressure (P), Campbell Scientific Inc., Logan, UT, USA, 1–5, 2018.
van Dijk, A.: The Principles of Surface Flux Physics, Department of Meteorology and Air Quality, Agriculture University Wageningen, 40–41, 2002.
Wallace, J. M. and Hobbs, P. V. (Eds.): Atmospheric Science: An Introductory Survey, Academic Press, London, 350 pp., 2006.
Wang, W., Xu, J., Gao, Y., Bogoev, I., and Lee, X.: Performance evaluation of an integrated open-path eddy covariance system in a cold desert environment, J. Atmos. Ocean. Tech., 33, 274–283, https//doi.org/10.1175/JTECH-D-15-0149.1, 2016.
Webb, E. K., Pearman, G. I., and Leuning, R.: Correction of flux measurements for density effects due to heat and water vapour transfer, Q. J. Roy. Meteor. Soc., 106, 85–100, https//doi.org/10.1002/qj.49710644707, 1980.
WMO: Guide to Instruments and Methods of Observation, WMO-No. 8, Volume I – Measurement of Meteorological Variables, World Meteorological Organization, Geneva, 548 pp., 2018.
Wright, J. D., Johnson, A. N., and Moldover, M. R.: Design and uncertainty for a PVTt gas flow standard, J. Res. Natl. Inst. Stan., 108, 21–47, https//doi.org/10.6028/jres.108.00, 2003.
Zhou, X. and Gao, T.: Dataset for: Air temperature equation derived from sonic temperature and water vapor mixing ratio for turbulent air flow sampled through closed-path eddy-covariance flux systems, Dryad [data set], availale at: https://datadryad.org/stash/share/ZiwOBaIBtu85UQ2kFye2LCtkzgp6l_UFg7dMeFi52ww, last access: 21 December 2021.
Zhou, X., Gao, T., Pang, Y., Manhan, H., Li, X., Zheng, N., Suyker, A. E., Awada, T., and Zhu, J.: Based on atmospheric physics and ecological principle to assess the accuracies of field measurements from infrared gas analyzers in closed-path eddy-covariance systems, Earth Space Sci., 8, e2021EA001763, https://doi.org/10.1029/2021EA001763, 2021.
Zhou, X., Yang, Q., Zhen, X., Li, Y., Hao, G., Shen, H., Gao, T., Sun, Y., and Zheng, N.: Recovery of the three-dimensional wind and sonic temperature data from a physically deformed sonic anemometer, Atmos. Meas. Tech., 11, 5981–6002, https://doi.org/10.5194/amt-11-5981-2018, 2018.
Zhu, J., Gao, T., Yu, L., Yu, F., Yang, K., Lu, D., Yan, Q., Sun, Y., Liu, L., Xu, S., Zhang, J., Zheng, X., Song, L., and Zhou, X.: Functions and applications of Multi-Tower Platform of Qingyuan Forest Ecosystem Research Station of Chinese Academy of Sciences (Qingyuan Ker Towers), Bulletin of Chinese Academy of Sciences, 3, 351–361, https//doi.org/10.16418/jissn.1000-3045.20210304002, 2021.
- Abstract
- Introduction
- Background
- Theory
- Accuracy
- Materials and methods
- Results
- Discussion
- Concluding remarks
- Appendix A: Derivation of Eq. (4)
- Appendix B: Derivation of Eq. (5)
- Appendix C: Water vapor mixing ratio and sonic temperature from relative humidity, air temperature, and atmospheric pressure
- Code availability
- Data availability
- Author contributions
- Competing interests
- Disclaimer
- Acknowledgements
- Financial support
- Review statement
- References
- Abstract
- Introduction
- Background
- Theory
- Accuracy
- Materials and methods
- Results
- Discussion
- Concluding remarks
- Appendix A: Derivation of Eq. (4)
- Appendix B: Derivation of Eq. (5)
- Appendix C: Water vapor mixing ratio and sonic temperature from relative humidity, air temperature, and atmospheric pressure
- Code availability
- Data availability
- Author contributions
- Competing interests
- Disclaimer
- Acknowledgements
- Financial support
- Review statement
- References