the Creative Commons Attribution 4.0 License.
the Creative Commons Attribution 4.0 License.
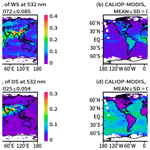
Global 3-D distribution of aerosol composition by synergistic use of CALIOP and MODIS observations
Rei Kudo
Akiko Higurashi
Eiji Oikawa
Masahiro Fujikawa
Hiroshi Ishimoto
Tomoaki Nishizawa
For the observation of the global three-dimensional distribution of aerosol composition and the evaluation of the shortwave direct radiative effect (SDRE) by aerosols, we developed a retrieval algorithm that uses observation data from the Cloud–Aerosol Lidar with Orthogonal Polarization (CALIOP) on board the Cloud–Aerosol Lidar Infrared Pathfinder Satellite Observations (CALIPSO) satellite and the Moderate Resolution Imaging Spectroradiometer (MODIS) on board Aqua. The CALIOP–MODIS retrieval optimizes the aerosol composition to both the CALIOP and MODIS observations in the daytime. Aerosols were assumed to be composed of four aerosol components: water-soluble (WS), light-absorbing (LA), dust (DS), and sea salt (SS) particles. The outputs of the CALIOP–MODIS retrieval are the vertical profiles of the extinction coefficient (αa), single-scattering albedo (ω0), asymmetry factor (g) of total aerosols (WS+LA+DS+SS), and αa of WS, LA, DS, and SS. Daytime observations of CALIOP and MODIS in 2010 were analyzed by the CALIOP–MODIS retrieval. The global means of the aerosol optical depth (τa) at 532 nm were 0.147±0.148 for total aerosols, 0.072±0.085 for WS, 0.027±0.035 for LA, 0.025±0.054 for DS, and 0.023±0.020 for SS. τa of the CALIOP–MODIS retrieval was between those of the CALIPSO and MODIS standard products and was close to the MODIS standard product. The global means of ω0 and g were 0.940±0.038 and 0.718±0.037; these values are in the range of those reported by previous studies. The horizontal distribution of each aerosol component was reasonable; for example, DS was large in desert regions, and LA was large in the major regions of biomass burning and anthropogenic aerosol emissions. The values of τa, ω0, g, and fine and coarse median radii of the CALIOP–MODIS retrieval were compared with those of the AERONET products. τa at 532 and 1064 nm of the CALIOP–MODIS retrieval agreed well with the AERONET products. The ω0, g, and fine and coarse median radii of the CALIOP–MODIS retrieval were not far from those of the AERONET products, but the variations were large, and the coefficients of determination for linear regression between them were small. In the retrieval results for 2010, the clear-sky SDRE values for total aerosols at the top and bottom of the atmosphere were and W m−2, respectively, and the impact of total aerosols on the heating rate was from 0.0 to 0.5 K d−1. These results are generally similar to those of previous studies, but the SDRE at the bottom of the atmosphere is larger than that reported previously. Consequently, comparison with previous studies showed that the CALIOP–MODIS retrieval results were reasonable with respect to aerosol composition, optical properties, and the SDRE.
- Article
(12019 KB) - Full-text XML
-
Supplement
(1006 KB) - BibTeX
- EndNote
Aerosols have significant impacts on climate change through modification of the atmospheric radiation budget by scattering and absorbing solar and terrestrial radiation (aerosol–radiation interaction) and by modifying cloud physical properties (aerosol–cloud interaction). However, large uncertainties remain in evaluations of the aerosol impact on global warming (Arias et al., 2021) because of the large spatiotemporal variations in aerosol composition and the complex physical processes of aerosol–radiation and aerosol–cloud interactions. Because the radiative forcing of almost all aerosol chemical components is negative, aerosols contribute to the suppression of global warming; however, the radiative forcing of light-absorbing aerosols such as black carbon (BC) is positive (e.g., Matsui et al., 2018). Observations of spatiotemporal variations of aerosol composition are therefore essential for a better understanding of the impacts of aerosols on climate change.
Based on recent sophisticated numerical models with aerosol modules, as well as space- and ground-based observations, the data sets of aerosol composition climatology have been developed. The Modern-Era Retrospective analysis for Research and Applications version 2 (MERRA-2; Gelaro et al., 2017), the Copernicus Atmosphere Monitoring Service Reanalysis (CAMSRA; Innes et al., 2019), and the Japanese Reanalysis for Aerosol v1.0 (JRAero; Yumimoto et al., 2017) are the reanalysis data sets created by using data assimilation schemes. The Max Planck Aerosol Climatology version 2 (MACv2; Kinne, 2019) is a climatology data set created by merging the data of the Aerosol Robotics Network (AERONET; Holben et al., 1998) and MAN (Smirnov et al., 2009) ground-based sun-photometer networks onto the ensemble mean of AeroCom models (Kinne et al., 2006). These data sets provide the global distributions of major aerosols, such as sulfate, organic carbon, BC, dust, and sea salt. The ModIs Dust AeroSol (MIDAS; Gkikas et al., 2021) data set is a global map of dust at fine resolution (0.1∘ × 0.1∘) created by the aerosol optical depth derived from the Moderate Resolution Imaging Spectroradiometer (MODIS) and the dust fraction of the MERRA-2 reanalysis. Amiridis et al. (2015) develop LIVA (Lidar climatology of Vertical Aerosol Structure for space-based lidar simulation studies), which is a three-dimensional multi-wavelength global aerosol and cloud optical data set. This data set is based on the Cloud–Aerosol Lidar with Orthogonal Polarization (CALIOP) on board the Cloud–Aerosol Lidar Infrared Pathfinder Satellite Observations (CALIPSO) satellite (Winker et al., 2010) and the ground-based European Aerosol Research Lidar Network (EARLINET; Bösenberg et al., 2003; Pappalardo et al., 2014) and AERONET.
These data sets are based on combinations of numerical models with aerosol modules, as well as space- and ground-based remote sensing products. The remote sensing of aerosols plays an important role in constructing the data sets. Several ground-based remote sensing methods to retrieve aerosol composition have been developed. Kudo et al. (2010a) estimated 10-year variations of water-soluble particles (WS), BC, dust (DS), and sea salt (SS) from the direct and diffuse solar radiation in the visible and near-infrared wavelength regions measured by two pyranometers and two pyrheliometers. Nishizawa et al. (2007, 2008, 2011, 2017) retrieved concentrations of WS, BC, DS, and SS by using conventional Mie-scattering lidar as well as high-spectral-resolution lidar or Raman lidar data from the Asian Dust and Aerosol Lidar Observation Network (AD-Net; Sugimoto et al., 2015; Shimizu et al., 2016). AERONET is an observational network of sun–sky radiometers that provides aerosol optical depth (τa), single-scattering albedo (ω0), asymmetry factor (g), phase function, and complex refractive index data products (Dubovik and King, 2000; Dubovik et al., 2006; Sinyuk et al., 2020). Schuster et al. (2005) and Dey et al. (2006) inferred BC concentrations from the AERONET-retrieved size distribution and complex refractive index. They considered internal and external mixtures of BC, sulfate, organic carbon, DS, and water. Satellite remote sensing has also been used for estimating aerosol composition and investigating global distributions. For example, Higurashi and Nakajima (2002) and Kim et al. (2007) retrieved the spatiotemporal distributions of sulfate, carbonaceous, DS, and SS aerosols from spectral information on radiances observed by satellite imagers, such as the Sea-Viewing Wide Field-of-View Sensor (SeaWIFS), MODIS, and Ozone Monitoring Instrument (OMI). The CALIOP on board the CALIPSO satellite has been utilized to classify aerosols at different altitudes (Omar et al., 2009; Winker et al., 2010). CALIOP version 4 products classify 11 aerosol types: clean marine, DS, polluted continental/smoke, clean continental, polluted DS, elevated smoke, and dusty marine for tropospheric aerosols, as well as polar stratospheric aerosol, volcanic ash, sulfate/other, and smoke for stratospheric aerosols (Kim et al., 2018). These ground- and space-based methods assume that aerosols consist of a few components with different sizes, light-absorbing features, and shapes (spherical or non-spherical), and they retrieve the aerosol composition from optical measurements made by using different wavelengths and polarization.
The abovementioned remote sensing methods retrieve aerosol data obtained by a single instrument. Recently, synergistic remote sensing methods using active and passive sensors have been developed. Passive sensors such as spectral radiometers and polarimeters provide the columnar properties of aerosols, whereas aerosol vertical profiles are obtained by active sensing by lidar. The LIRIC (Chaikovsky et al., 2016) and GARRLiC (Lopatin et al., 2013) algorithms retrieve the vertical profiles of aerosol physical and optical properties from lidar and AERONET sun–sky radiometer observations. SKYLIDAR (Kudo et al., 2016) estimates aerosol vertical profiles from AD-Net lidar and SKYNET sky radiometer observations (Nakajima et al., 2020). Xu et al. (2021) have retrieved aerosol physical and optical properties as well as ocean parameters such as chlorophyll a concentration and surface wind speed from lidar and polarimetric observations over the ocean obtained during the ORACLES field campaign (Redemann et al., 2021).
To observe the global three-dimensional distribution of the aerosol composition, we have developed a new aerosol composition retrieval method that uses the CALIOP and MODIS observations. The CALIOP–MODIS retrieval optimizes the aerosol composition to both the CALIOP and MODIS observations in the daytime. The columnar properties of aerosols are available from the MODIS multi-wavelength information, and τa is retrieved accurately (e.g., Shi et al., 2019), but aerosol vertical profiles cannot be obtained, and strong surface reflection (e.g., snow, desert) makes the retrieval difficult (Hsu et al., 2013). CALIOP observations exclude the data in the layers contaminated by surface reflection and provide information on the vertical profiles of aerosol optical properties and particle shapes (spherical or non-spherical), but only limited wavelength information. Additionally, CALIOP does not detect the tenuous layers in the daytime due to the low signal-to-noise ratio. This results in the underestimation of τa (Omar et al., 2013; Kim et al., 2018). The synergistic use of both instruments decreases the influences of the surface reflection and provides more accurate columnar properties and vertical profiles of aerosols. Furthermore, the particle size information is obtained from the combined spectral information of the CALIOP and MODIS observations (Kaufman et al., 2003).
In previous remote sensing methods of aerosol compositions, there are two approaches for assuming aerosol components. One is the CALIOP-type categorization, such as clean marine, polluted continental, and smoke. These types are based on the aerosol characteristics observed in typical scenes. The other is a similar categorization to the numerical models, i.e., sulfate, organic carbon, BC, DS, and SS. We adopted the latter approach because the external mixing of these components is applicable to various scenes, and the τa and extinction coefficient (αa) of each component are suited for the comparison with the numerical models and the data assimilation. In this study, aerosols are assumed to consist of four components with different sizes, light-absorbing features, particle mixtures, and shapes. We defined these components as WS, light-absorbing particles (LA), DS, and SS. WS is defined by an external mixture of sulfate and organic carbon, for example, because both the sulfate and organic carbon are fine and less light-absorbing particles, and it is difficult to estimate sulfate and organic carbon separately from the MODIS and CALIOP measurements. LA is defined by an internal mixture of WS and BC. The details of the assumed aerosols are described in Sect. 3. In this study, the global three-dimensional distributions of these components were estimated from the CALIOP–MODIS retrieval.
The aerosol-induced effects on the radiation field are denoted as aerosol radiative effects and are evaluated by the anomalies with respect to a reference state (Korras-Carrat et al., 2021). The clear-sky shortwave direct radiative effects (SDREs) are defined as the anomalies from the shortwave radiation field without aerosols. The SDREs have been investigated based on the numerical models, as well as satellite and ground-based measurements. A number of measurement-based approaches estimate the SDRE at the top of the atmosphere (TOA) to be W m−2 over the ocean and W m−2 over land (Yu et al., 2006). Since the aerosol vertical profile affects the SDRE at TOA, the aerosol vertical profiles derived from the CALIOP have been considered in the evaluation of the SDREs (e.g., Oikawa et al., 2018). Furthermore, the impacts of aerosols on the atmospheric heating rate are estimated using the aerosol vertical profiles (Korras-Carraca et al., 2019). These studies estimate the SDREs for total aerosols. In this study, the clear-sky SDREs at the top and bottom of the atmosphere and the impacts on the heating rate for each aerosol component are estimated based on the CALIOP–MODIS retrievals.
This article is organized as follows. The CALIOP and MODIS observation data used for the retrievals are described in Sect. 2. The retrieval algorithm and the SDRE calculation method are described in Sect. 3. The uncertainties in the retrieval results are evaluated by using simulated CALIOP and MODIS observation data in Sect. 4. The global three-dimensional distribution of aerosol compositions and the shortwave direct radiative forcing in 2010 are analyzed in Sect. 5. All of the results are summarized in Sect. 6.
2.1 Input of the CALIOP–MODIS retrievals
The CALIOP–MODIS retrieval is applied to only the clear-sky (cloud-free) data from the CALIOP and MODIS observations. We made a clear-sky match-up data set of CALIOP attenuated backscatter coefficients, MODIS radiances, surface albedo, and meteorological data acquired along the orbital track of A-train satellites, which includes the CALIPSO and Aqua satellites. The CALIOP data comprise the attenuated backscatter coefficients (β) at 532 and 1064 nm and the total (or volume) depolarization ratio (δ) at 532 nm in the CALIPSO lidar level 1B version 4 data product (Getzewich et al., 2018; Kar et al., 2018; Vaughan et al., 2019). The horizontal resolution of the original β data set is 333 m; the vertical resolution is 30 m for β at 532 nm and 60 m for β at 1064 nm. Since the resolutions are different for the measurements, we created a clear-sky data set with a horizontal resolution of 1 km and vertical resolutions of 120 m from −0.5 to 20.2 km altitudes and 180 m from 20.2 to 30.1 km altitudes by using the following procedure. Firstly, we collected the clear-sky CALIOP observations discriminated as clear air, tropospheric aerosol, and stratospheric aerosol by the vertical feature mask (VFM) product of CALIPSO lidar level 2 version 4 (Kim et al., 2018; Liu et al., 2019). The VFM product describes layer classification information and provides a cloud–aerosol discrimination (CAD) score, which is the confidence level for cloud–aerosol classification. CAD can range from −100 to +100, where positive (negative) values indicate clouds (aerosols). A higher absolute value indicates greater confidence in the classification result. In this study, we used aerosol–cloud classification results with a CAD score greater than 70 for quality assurance (Liu et al., 2009). Secondly, the clear-sky CALIOP observations at our defined horizontal and vertical coordinates were obtained by a running mean with a horizontal window of 10 km and vertical windows of 120 and 180 m. The signal noises of the CALIOP observations are reduced by the running mean.
We used Aqua MODIS Level 1B Calibrated Radiances (MYD02SSH, Collection 6.0) in bands 1 (620–670 nm) and 2 (841–876 nm) with along- and across-track resolutions of 5 km. To exclude cloud-contaminated observations, we used the Level 2 Cloud Mask Product (MYD35_L2, Collection 6.0; Ackerman et al., 2015). We used the black- and white-sky albedo of MCD43C3 Collection 6.0 (Schaaf et al., 2002; Wang et al., 2018) for the land surface reflection in the forward calculation of MODIS observations (in the section “Forward model of MODIS observations”). The clear-sky radiances and albedos at the nearest pixel within a 10 km range from the near–nadir measurements (∼3∘ off nadir) of CALIOP were selected for retrieval.
As ancillary data for the forward calculations of CALIOP and MODIS observations, we used pressure, temperature, relative humidity, ozone concentration, and ocean surface wind speed from the MERRA-2 reanalysis data product (Gelaro et al., 2017). The ocean surface wind speed was used in calculating the ocean surface reflection in the forward model of the MODIS observations.
2.2 Data for comparison of retrieval results
The results of the CALIOP–MODIS retrievals in 2010 are compared with the CALIPSO and MODIS standard products and AERONET products in Sect. 5. The CALIPSO standard product comprises the monthly means of τa and αa in the cloud-free daytime data set of the CALIPSO lidar level 3 tropospheric aerosol product version 4 (Tackett et al., 2018), which has longitudinal, latitudinal, and vertical resolutions of 5∘, 2∘, and 60 m, respectively. The MODIS standard product comprises the monthly means of τa in the MYD08_M3 Collection 6.1 Aqua Atmosphere Monthly Global Product (Platnick et al., 2015), with longitudinal and latitudinal resolutions of 1∘. The annual means were calculated from the monthly means. The AERONET products comprise τa, ω0, g, and fine- and coarse-mode radii in the level 2 almucantar retrievals of the version 3 inversion data product (Giles et al., 2019; Sinyuk et al., 2020).
3.1 Retrieval methods
3.1.1 Retrieval procedure
Figure 1 is a flow diagram of the retrieval procedures. The vertical profiles of the dry volume concentrations (Vdry) of WS, LA, DS, and SS, as well as the columnar values of the dry median radii (rm,dry) of the fine (WS and LA) and coarse (DS) particles are optimized to each CALIOP and MODIS data pair. Vdry is defined as the volume of aerosols at a relative humidity of 0 % per unit atmospheric volume, and rm,dry is defined as the median radius of aerosols at a relative humidity of 0 %. rm,dry of SS is given by a parameterization that uses the ocean surface wind speed (Erickson and Duce, 1988). Only the vertical layers discriminated as aerosols in the VFM data are targeted for retrieval, and the CALIOP–MODIS retrieval is conducted for only clear-sky data in the daytime. If clouds are detected in the VFM data, the CALIOP–MODIS retrieval is not conducted.
Inversion is conducted by the optimal estimation technique developed by Kudo et al. (2016). The state vector is optimized simultaneously to the measurements and a priori constraints by minimizing the following objective function:
where x is the state vector to be optimized and is comprised of Vdry for WS, LA, DS, and SS, as well as rm,dry for the fine (WS and LA) and coarse (DS) particles; the vector yobs represents the CALIOP and MODIS measurements; the vector y(x) represents the calculations by the forward models corresponding to yobs; W2 is the covariance matrix of y; the vector ya(x) gives the a priori constraints for x; and is an associated covariance matrix. The forward calculations of the optical properties from Vdry and rm,dry for WS, LA, DS, and SS are described in the section “Forward model of aerosol physical and optical properties”. The forward models of the CALIOP and MODIS observations from the aerosol optical properties are described in the sections “Forward model of CALIOP observations” and “Forward model of MODIS observations”. The details of the CALIOP–MODIS retrieval and the a priori constraints are described in Sect. 3.1.3. The minimization of f(x) is conducted by using an iterative algorithm, with logarithmic transformation applied to x and y for stable and fast convergence of the iteration. Because the CALIOP measurements can have negative values caused by large signal noise, CALIOP measurements were transformed by , where ymin is a possible minimum value of y. The best solution of x, which minimizes f(x), is searched for by the iteration of in ln (x) space, where the vector d is determined by the Gauss–Newton method, and the scalar α is determined by a line search with the Armijo rule. The convergence criterion for the iteration is that the difference between f(xk) and f(xk+1) is smaller than the given threshold two consecutive times.
3.1.2 Forward models
Forward model of aerosol physical and optical properties
We assumed that the aerosols consisted of four components: WS, LA, DS, and SS. Their physical and optical properties at relative humidities of 0 % and 80 % are summarized in Table 1. WS and LA are small particles with small g. DS and SS are large particles with large g. LA and DS are light-absorbing particles and have small ω0. WS and SS have large ω0.
Table 1Physical and optical properties of the four aerosol components at relative humidities of 0 % and 80 % (0 % 80 %).
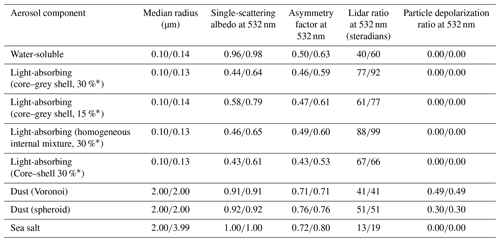
* Volume fraction of black carbon in a particle.
WS was assumed to be a mixture of sulfates, nitrates, and organic and water-soluble substances (Hess et al., 1998). Their shape was assumed to be spherical, and their refractive index was defined from the OPAC database (Hess et al., 1998). We considered WS to grow hygroscopically and used the dependencies of particle size and refractive index on relative humidity given in the OPAC database.
BC particles are emitted into the atmosphere by incomplete combustion of fossil fuels, biomass, and biofuels. The freshly emitted BC particles are generally externally mixed with the other particles and are in a hydrophobic state (Weingartner et al., 1997). These particles are gradually internally mixed by aging processes (condensation, coagulation, and/or photochemical oxidation process) in the atmosphere and become hydrophilic by coating with water-soluble compounds (Oshima et al., 2009). We defined LA as an internal mixture of BC and WS, and we introduced the core–grey shell (CGS) model (Kahnert et al., 2013). The CGS model has a spherical shape with a BC core and a shell consisting of a homogeneous mixture of WS and BC. The optical properties of CGS model are better representations of a realistic encapsulated aggregate model than the internally homogeneous mixture model obtained by using the Maxwell Garnett mixing rule (MG; Maxwell Garnett, 1904) and the core–shell (CS) model. The optical properties (αa, ω0, g, and lidar ratio – Sa) of CGS have values between those of the CS and MG models (Table 1). Kahnert et al. (2013) defined a CGS model as a mixture of BC and sulfate, but we used WS instead of sulfate in our definition. The details of the application of the CGS model are described in Appendix A. The refractive index of BC was defined from the measurements of Chang and Charalampopoulos (1990). The hygroscopic growth of LA particles was considered because the WS particles mixed in the shell are hydrophilic. We used the dependencies of the volume and refractive index of WS on the relative humidity in the OPAC database for the shell of LA particles. In general, the volume fraction of BC in an internally mixed particle changes spatiotemporally due to the different emission sources and the aging processes (e.g., Moteki et al., 2007), but it is difficult to optimize the BC volume fraction in the CALIOP–MODIS retrieval. Therefore, we fixed the BC volume fraction at 30 % of the total (BC+WS) volume, which is within the range of values observed by the A-FORCE aircraft campaign in East Asia (Matsui et al., 2013). Because there are large uncertainties in the particle models and the BC volume fraction, we conducted sensitivity tests using the different particle models (CGS, CS, and MG) and BC volume fractions (15 % and 30 %) (Sect. 5).
The Voronoi particle model (Ishimoto et al., 2010) was used for DS in this study. Based on electron microscope observations, the shape of the Voronoi particle model was created by a spatial Poisson–Voronoi tessellation. As an optional model, the spheroid particle model of Dubovik et al. (2006) was also introduced in the retrieval. The particle depolarization ratio (δa) of a spheroid particle is less than that of a Voronoi particle (Table 1). We therefore conducted a sensitivity study of the two particle models (Sect. 5). The refractive index of DS was obtained from the database of Aoki et al. (2005); this database was created from in situ measurements of dust samples in the Taklimakan Desert, China.
SS particles were assumed to be spherical, and the refractive index in the OPAC database was used. Hygroscopic growth of SS was also considered, and the particle size and refractive index were changed depending on the relative humidity. In retrievals over the ocean, four components (WS, LA, DS, and SS) were considered, but SS was ignored in retrievals over land.
Each component was assumed to have a lognormal size distribution, and hygroscopic growth was considered by including a growth factor as follows:
where r is radius, V is total volume, rm is median radius, σ is the standard deviation, RH is relative humidity, and GF is the growth factor. The standard deviation is fixed at 0.45 for WS and LA and at 0.8 for DS and SS. These values are slightly larger than those of AERONET retrievals in worldwide locations (Dubovik et al., 2002). rm,dry values of fine (WS and LA) and coarse (DS) particles were parameters to be optimized. Here, rm,dry of WS and LA was assumed to be the same. rm,dry of SS was determined by the following relationship between the ocean surface wind speed and the mass-mean radius for a relative humidity of 80 % (Erickson and Duce, 1988):
where mmr is the mass-mean radius and u is the ocean surface wind speed. The mass-mean radius is defined as the ratio of the fourth moment of the radius with respect to the number size distribution to the third moment (Lewis and Schwartz, 2004). rm,dry was calculated from mmr by using the lognormal size distribution obtained by Eq. (2). The growth factors (GFs) for WS, the LA shell, and SS were obtained from the OPAC database.
To reduce the computational time, we constructed lookup tables of αa, ω0, and the phase matrix for each model using the abovementioned particle models and size distributions. The inputs of the lookup tables were Vdry and rm,dry of WS, LA, DS, and SS, as well as relative humidity. The outputs were αa, ω0, the phase matrix, and the size distribution of each component at the input relative humidity. Finally, αa, ω0, the phase matrix, g, Sp, δp, and the size distribution of total aerosols (WS+LA+DS+SS) were calculated according to the external mixture. These optical properties are used in the forward models of CALIOP and MODIS observations.
Forward model of CALIOP observations
We constructed a forward model to calculate β at 532 and 1064 nm and δ at 532 nm from the vertical profiles of αa, Sa, and δa by using the following lidar equations:
where βco and βcr are co- and cross-polarization components of β; λ is wavelength; z is altitude; αm, Sm, and δm are the extinction coefficient, lidar ratio, and depolarization ratio of molecular scattering; and TOA is the top of the atmosphere.
Forward model of MODIS observations
The band 1 and 2 radiances corresponding to the MODIS observations were calculated by the PSTAR vector radiative transfer model (Ota et al., 2010). The inputs of the forward model were the vertical profiles of αa, ω0, and phase matrix calculated by the forward model of the aerosol optical properties. The surface reflection over the ocean was calculated from the surface wind speed by using the physical model of Nakajima and Tanaka (1983). The surface reflection over the land was assumed to be Lambert reflectance, and the actual albedo calculated from the black- and white-sky albedo of MODIS land surface products (Sect. 2.3) was used. The actual albedo from the black- and white-sky albedo was calculated by the method of Schaaf et al. (2002). Absorption of H2, O3, CO2, O2, O3, and NO gases was considered in the radiative transfer calculation. The absorption coefficient was calculated by the correlated-k distribution method (Sekiguchi and Nakajima, 2008).
For rapid calculation, the response functions of bands 1 and 2 were divided into three sub-bands. The atmospheric vertical layers were assumed to consist of five vertical layers: 0–1, 1–3, 3–6, 6–10, and 10–120 km above the surface. The influence of these assumptions was evaluated by referring to radiances simulated with the 10 sub-bands and 271 vertical layers. The properties of the aerosols, surfaces, and solar zenith angles used in the simulations were the same as those used in the simulations described in Sect. 4. The relative error of the radiances was less than 1 % for bands 1 and 2.
3.1.3 CALIOP–MODIS retrieval
The vertical profiles of Vdry of WS, LA, DS, and SS, as well as the columnar values of rm,dry of fine (WS and LA) and coarse (DS) particles were optimized to each CALIOP and MODIS data pair. rm,dry of SS was given by the parameterization using the ocean surface wind speed. The vertical profiles of rm,dry were not considered in this study.
DS and SS are coarse particles, and they are more sensitive to β at 1064 nm compared with the fine particles of WS and LA. Because only DS was assumed to be non-spherical, Vdry of DS and SS could be estimated from β at 1064 nm and δ at 532 nm. Vdry of WS and LA could not be independently retrieved from only β at 532 nm. Therefore, we introduced a priori constraints for WS and LA, as described later. The retrieval of the median radius from the satellite measurements is highly challenging, but Kaufman et al. (2003) have shown that the effective radius can be estimated from the wavelength dependencies of β measurements at 532 and 1064 nm, as well as the radiance measurements at the near-infrared wavelength. We conducted a similar sensitivity study to that conducted by Kaufman et al. (2003). The scattering intensity is defined as
where θ is the scattering angle, λ is wavelength, P is the normalized phase function, and τsca is the scattering coefficient. In the calculations of the phase function and scattering coefficient, a lognormal size distribution with a standard deviation of 0.4 and the refractive index of DS were used. We calculated the scattering intensities for different wavelengths, scattering angles, median radii, and particle shapes. Figure 2 shows the ratios of the scattering intensities. The scattering intensity at the scattering angle of 180∘ (Fig. 2a) represents lidar measurements, and the other angles (Fig. 2b, c, and d) represent MODIS measurements. For spherical and spheroidal particles, the scattering intensity ratios increase with an increase in the median radius within the ranges of 0.05–0.2 and 0.5–2.0 µm. The scattering intensity ratios for Voronoi particles increase with an increase in the radius over the entire radius range. These relationships indicate that the median radii of fine and coarse particles can be estimated from the spectral information of CALIOP and MODIS measurements.
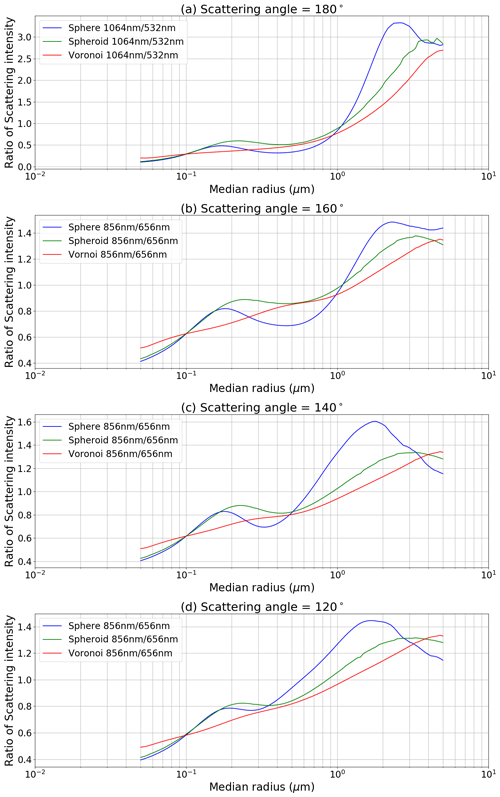
Figure 2Relation between median radius and the ratio of scattering intensity at different wavelengths for (a) CALIOP and (b, c, d) MODIS observations. Blue, green, and red indicate sphere, spheroid, and Voronoi particle models, respectively.
The CALIOP–MODIS retrieval procedure is diagrammed in Fig. 1, and the objective function is given by Eq. (1). The state vector x consists of the vertical profiles of Vdry of WS, LA, DS, and SS, as well as rm,dry of fine (WS and LA) and coarse (DS) particles. rm,dry of WS and LA were assumed to be the same. rm,dry of SS was given by the parameterization using ocean surface wind speed. The measurement vector yobs was β at 532 and 1064 nm, δ at 532 nm, and the band 1 and 2 MODIS radiances. The forward calculation y(x) was processed by the forward models of the CALIOP (section “Forward model of CALIOP observations”) and MODIS (section “Forward model of MODIS observations”) observations. The covariance matrix W2 was assumed to be diagonal, and the diagonal element of matrix W was obtained from the measurement accuracy. The measurement accuracy of β at 532 nm of CALIOP version 3 was estimated by comparison with airborne high-spectral-resolution lidar (HSRL) data (Rogers et al., 2011). The mean difference was 2.9 %, and the standard deviation was 20 % in the daytime. The bias of β at 532 nm of CALIOP version 4 was smaller than that of CALIOP version 3 (Getzewich et al., 2018), and our data set was smoothed by calculating the running mean (Sect. 2.1); thus, the accuracy of β at 532 nm was assumed to be 15 %. The measurement accuracies of β at 1064 nm and δ at 532 nm were assumed to be 20 % and 50 %, respectively. Because we could not find previous reports of the measurement accuracies of β at 1064 nm and δ at 532 nm when we started this study, we used values greater than the standard deviations for some scenes as the measurement accuracies. We defined the diagonal elements of W for the band 1 and 2 radiances of MODIS by using the following equation:
where τa at 532 nm is obtained from the result of the CALIOP retrieval (Fujikawa et al., 2020), and the slope α and intercept β values were calculated from the equation as well as two ordered pairs of x and y: and (0.5,0.1). We assumed that W for the radiances depended on τa and that its range was from 0.1 to 1.0. When τa is small, the upward radiance at the top of the atmosphere is significantly affected by the surface reflectance. However, we used the Lambert surface reflectance in the forward model of MODIS observations, and the surface albedo was obtained from the ancillary data. Therefore, when τa was small, we decreased the relative contribution of the MODIS measurements to the objective function by W (Eq. 9).
The retrieval of the vertical profiles of Vdry is significantly affected by lidar signal noise. Smoothness of the vertical profiles of Vdry of WS, LA, DS, and SS was assumed, and an a priori smoothness constraint was introduced by using the second derivatives for the vertical profiles of Vdry:
where zi is altitude. The vertical variation of Vdry was limited by minimizing Eq. (10). The covariance matrix in Eq. (1) was assumed to be a diagonal matrix, and the values of the diagonal elements used for the smoothness constraints were 0.2.
It is difficult to retrieve Vdry of WS and LA independently from only β at 532 nm. Therefore, we introduced two a priori constraints. First, the similarity of the vertical profiles of WS and LA was introduced. If the emission source of LA is the same as that of WS, for example, as with biomass burning emissions, the vertical profile of LA would be similar to that of WS near the emission source. We assumed that the vertical profile shape of LA was similar to that of WS, and the vertical profiles of LA and WS were constrained by
where is Vdry of LA and WS at altitude zi. The vertical changes in Vdry of WS and LA approach the same values when Eq. (11) is minimized. The second constraint was the inequality of τa of LA and WS. In the AERONET product at worldwide locations, ω0 ranges from 0.8 to 1.0 (Dubovik et al., 2002). ω0 is about 0.96 for WS and about 0.44 for LA (Table 1), and ω0 for an external mixture of WS and LA is calculated by . Thus, τa of WS must be greater than that of LA. Therefore, we introduced the following log barrier function as a constraint:
where represents τa of LA and WS at 532 nm. When τa,LA approaches τa,WS, Eq. (12) approaches infinity, and the objective function (Eq. 1) also becomes infinity. The similarity and inequality constraints limited the retrieval range of LA and prevented abnormal solutions. The diagonal elements of Wa were assumed to be 1.0 for both the similarity and inequality constraints.
In addition to the abovementioned a priori constraints, we applied an a priori constraint to rm,dry of fine (WS and LA) and coarse (DS) particles. The spectral dependencies of the CALIOP and MODIS measurements have information on the particle radius. However, the large noise in the CALIOP measurements affects the spectral dependencies of the CALIOP measurements, and errors in the given surface reflectance affect the forward calculation of the MODIS measurements. To avoid abnormal solutions, we therefore constrained rm,dry by Eq. (13):
where is rm,dry of fine and coarse particles, and is the a priori value. We assumed that was 0.1 µm for fine particles and 2.0 µm for coarse particles. The diagonal element Wa for the constraint of rm,dry was assumed to be 0.2 for fine particles and 0.3 for coarse particles.
The minimization of the objective function was based on the Gauss–Newton method (Sect. 3.1.1). This method requires the numerical derivatives of y(x), where the vector x consists of the vertical profiles of the four aerosol components as well as the fine and coarse median radii, and the number of elements is of the order of 10 to 100. The forward calculation of the MODIS observations by PSTAR is time-consuming. For more rapid calculation, we therefore approximated the numerical derivatives of the radiances at bands 1 and 2 for Vdry of WS, LA, DS, and SS. First, the numerical derivative was calculated from the monochromatic radiative transfer calculation at the center wavelengths of bands 1 and 2. Because logarithmic transformation was applied to x and y(x) and the best solution of x was searched for in log (x) space, the numerical derivative was defined as
is a relative value, and the radiances at bands 1 and 2 have no strong line absorptions. The monochromatic radiative transfer calculation for the numerical derivative is thus a good approximation. Second, the dependency of the numerical derivatives on Vdry was investigated. Figure 3 shows an example of the approximated and reference numerical derivatives for the radiances at bands 1 and 2. The vertical profiles of WS, LA, DS, and SS used in the calculation of the numerical derivatives are shown in the first column of Fig. 3. τa at 532 nm used in the calculation was 0.3. The surface was the ocean, and the wind speed was 15 m s−1. The solar zenith angle was 40∘. The reference numerical derivatives in the second column of Fig. 3 were calculated using the non-approximated forward model described in the section “Forward model of MODIS observations”. The numerical derivatives mainly depend on Vdry (the third column of Fig. 3). The altitude dependency is shown in the fourth column of Fig. 3. The altitude dependency of LA, in particular, cannot be ignored. Using these relations, we approximated the numerical derivatives by using the following procedure.
- 1.
For each aerosol component, 10th, 30th, and 80th percentiles of Vdry are selected. When the number of aerosol layers is low, 25th and 75th percentiles of Vdry are selected.
- 2.
The numerical derivatives for the selected Vdry are calculated for each aerosol component.
- 3.
The following equation is fit to the results of Eq. (2),
where z is altitude. The coefficients, a1, a2, and a3 are determined by the fitting.
- 4.
The numerical derivatives at all altitudes for each aerosol component are calculated by Eq. (15).
Figure 3 shows that the approximated numerical derivatives agree well with the reference values. However, the numerical derivatives of WS and SS near the surface have a unique behavior (see the second and fourth columns of Fig. 3), and our method could not approximate these. At present, we are unable to determine the cause of this unique behavior.
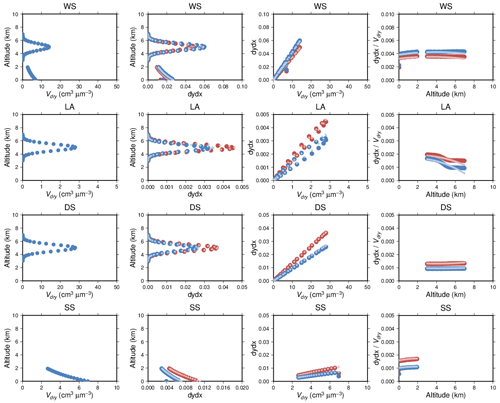
Figure 3Approximation of the numerical derivatives of MODIS radiances for Vdry of WS (first row), LA (second row), DS (third row), and SS (fourth row). The first column shows vertical profiles of Vdry, the second column shows vertical profiles of the numerical derivatives (dydx), the third column shows the dependency of dydx on Vdry, and the fourth column shows the dependency of on altitude. Blue and red indicate dydx at MODIS bands 1 and 2, respectively. Dark and light colors indicate the reference values and the approximated calculations, respectively.
The objective function was minimized by the method described in Sect. 3.1.1 using the approximated numerical derivatives. The outputs of the CALIOP–MODIS retrieval were the vertical profiles of Vdry and αa of WS, LA, DS, and SS, as well as the vertical profiles of αa, ω0, g, along with the size distribution of total aerosols at the ambient relative humidity. Even though we introduced some approximations for more rapid calculation, the CALIOP–MODIS retrieval is still time-consuming. Therefore, the CALIOP–MODIS retrieval was conducted every 5 km along the track of the CALIPSO satellite's orbit.
3.2 Clear-sky shortwave direct radiative effect
Aerosols directly affect the radiation field within the Earth–atmosphere system by the scattering and absorption of radiation. The aerosol-induced direct radiative effect is evaluated by the anomalies with respect to a reference state (Korras-Carraca et al., 2021). In this study, the clear-sky shortwave direct radiative effect (SDRE) was defined as the anomalies from the shortwave radiation field without aerosols and was calculated by the following procedure. We prepared a module to calculate the aerosol optical properties (τa, ω0, phase matrix) at any wavelength in the solar wavelength region from the retrieved Vdry and rm,dry of WS, LA, DS, and SS, as well as relative humidity by the forward model described in the section “Forward model of aerosol physical and optical properties”. The aerosol optical properties from 300 to 3000 nm were calculated by this module, and the clear-sky SDRE of aerosols was calculated by our developed radiative transfer model (Asano and Shiobara, 1989; Nishizawa et al., 2004; Kudo et al., 2011). The solar spectrum from 300 to 3000 nm was divided into 54 intervals. Gaseous absorption by H2O, CO2, O2, and O3 was calculated by the correlated-k distribution method. We calculated the SDRE of total aerosols (WS+LA+DS+SS) and of each component (WS, LA, DS, and SS) at the top of the atmosphere (TOA) and the bottom of the atmosphere (BOA) as follows:
where Fwith is the net flux density with the aerosol (total or each component), and Fwithout is the net flux density without the aerosol (total or each component). Furthermore, we calculated the impact of aerosols on the shortwave heating rate as
where HR is the heating rate in units of Kelvin per day (K d−1), and z is altitude.
4.1 Configuration of the simulation
The uncertainties of the CALIOP–MODIS retrieval products were evaluated by applying the CALIOP–MODIS retrieval to the synthetic data of the CALIOP and MODIS observations. The synthetic data for 16 patterns of aerosol compositions (Table 2, Fig. 4) and for different values of τa, land and ocean surfaces, and solar zenith angles were created by the simulations using the forward models in Sect. 3. The transport of WS, LA, and DS in the free atmosphere was considered in the biomass burning and dust cases (Table 2). The vertical profiles for the transported aerosols were assumed to have a normal distribution (Fig. 4). The boundary layer height was 2 km, and αa in the boundary layer decreased linearly with increasing altitude (Fig. 4). rm,dry values of 0.07, 0.1, and 0.15 µm were used for WS and LA, and values of 1.0, 2.0, and 4.0 µm were used for DS (Table 2). For τa at 532 nm, values of 0.05, 0.1, 0.3, 0.5, 0.7, and 1.0 were used. Three land surface types were considered, and as surface albedo at bands 1 and 2, values of 0.05 and 0.50 for grass, 0.35 and 0.41 for desert, and 0.96 and 0.88 for snow, respectively, were used. These values were taken from the ECOSTRESS Spectral Library database (https://speclib.jpl.nasa.gov/, last access: 27 August 2022). For the ocean surface, surface wind speeds of 5, 15, and 25 m s−1 were used. Solar zenith angles of 0, 20, 40, and 60∘ were used. Random errors were added to the simulated CALIOP and MODIS observations and to the simulated surface albedo and surface wind speed data. The random errors for the CALIOP observations were less than ±15 % for β at 532 nm, ±20 % for β at 1064 nm, and ±50 % for δ at 532 nm. The random errors for the MODIS observations were less than ±5 % for the radiances at bands 1 and 2. The random error added to the surface albedo was less than ±0.10; this value is greater than the root mean square errors of the MOD43 albedo products: 0.07 for snow–ice surface (Stroeve et al., 2005, 2013; Williamson et al., 2016) and 0.03 for agriculture, grassland, and forest (Wang et al., 2014). The random errors of surface wind speed over the ocean were considered to be less than ±5 m s−1; this error is slightly larger than the root mean square errors obtained by comparing the reanalysis data with ship measurements: 2.7 to 4.10 m s−1 for the National Centers for Environmental Prediction–Department of Energy reanalysis and from 1.67 to 2.77 m s−1 for the European Centre for Medium-Range Weather Forecasts Interim Re-Analysis (Li et al., 2013). Using the above conditions, the simulations of CALIOP and MODIS observations were conducted by the forward models described in the sections “Forward model of CALIOP observations” and “Forward model of MODIS observations”. A total of 1152 simulations were conducted.
Table 2Aerosol components and median radius (Vdry) values used in the simulations of CALIOP and MODIS observations.
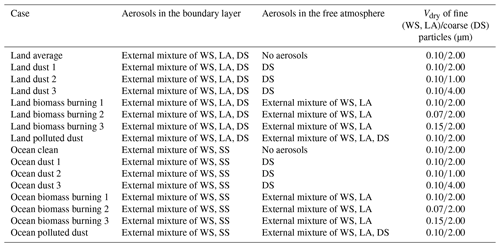
4.2 Uncertainties in the retrieval products
The retrievals of the columnar properties; τa, ω0, and g of total aerosols; τa of WS, LA, DS, and SS; and rm,dry of fine (WS and LA) and coarse (DS) particles are compared with the simulation results in Fig. 5. The plots of τa in Fig. 5a are aligned vertically in the lines because we controlled the total volume of aerosols by giving τa at 532 nm in the simulations. Overall, the retrieval results are scattered near the one-to-one line. τa retrievals at 532 and 1064 nm are estimated particularly well. τa of WS, DS, and SS also agree with the simulated values. However, τa of LA is overestimated, and ω0 at 532 nm is underestimated because of the overestimation of τa of LA. g of the CALIOP–MODIS retrieval agrees with the simulated values. rm,dry of fine (WS and LA) and coarse (DS) particles agrees well with the simulations. Figure 6 shows box-and-whisker plots of the differences between the retrievals and simulations for different values of the simulated τa at 532 nm. All of the differences except for τa of LA and ω0 decreased with an increase in the simulated τa, particularly in the cases with τa greater than 0.3. ω0 is underestimated over the entire range of simulated τa, and τa of LA is overestimated. Table 3 summarizes the means and standard deviations of the differences between the retrievals and simulations separately for the land and ocean surface results. In general, the small value of the ocean surface albedo is an ideal situation for the satellite remote sensing of aerosols. However, the retrieval results for τa of WS over the ocean are worse than those over the land because SS is taken into account, in addition to WS, LA, and DS, in the ocean surface cases. In the simulations, the random errors are added to the ocean surface wind speed. Since rm,dry of SS is determined by the given ocean surface wind speed and is not optimized in the CALIOP–MODIS retrieval, the random errors cause the difference of rm,dry of SS between the simulation and retrieval. The difference affects τa of SS. Since both WS and SS are less light-absorbing particles, τa of WS is overestimated (underestimated) when τa of SS is underestimated (overestimated). This opposite sign is seen in the ocean cases in Table 3.
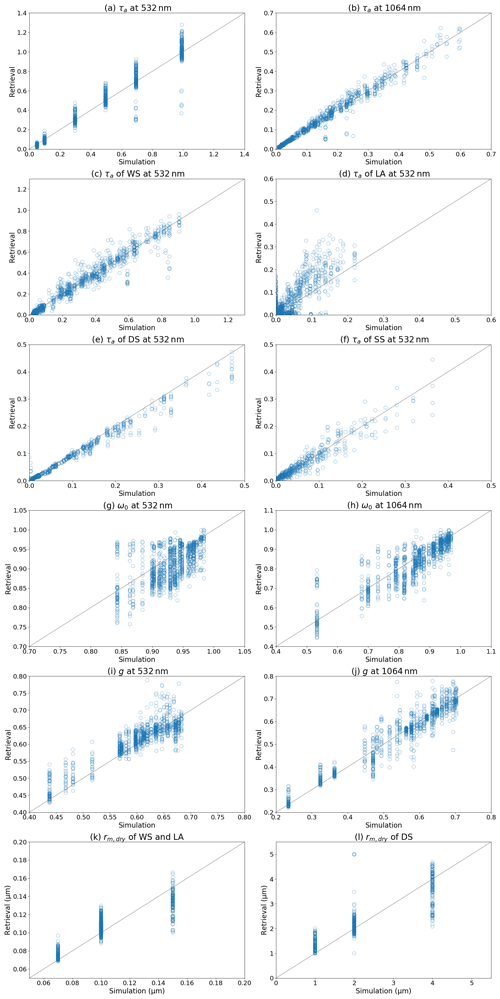
Figure 5Scatter plots of simulated and retrieved columnar properties: τa at (a) 532 nm and (b) 1064 nm; τa at 532 nm of (c) WS, (d) LA, (e) DS, and (f) SS; ω0 at (g) 532 nm and (h) 1064 nm; g at (i) 532 nm and (j) 1064 nm; rm,dry of (k) fine (WS and LA) and (l) coarse (DS) particles.
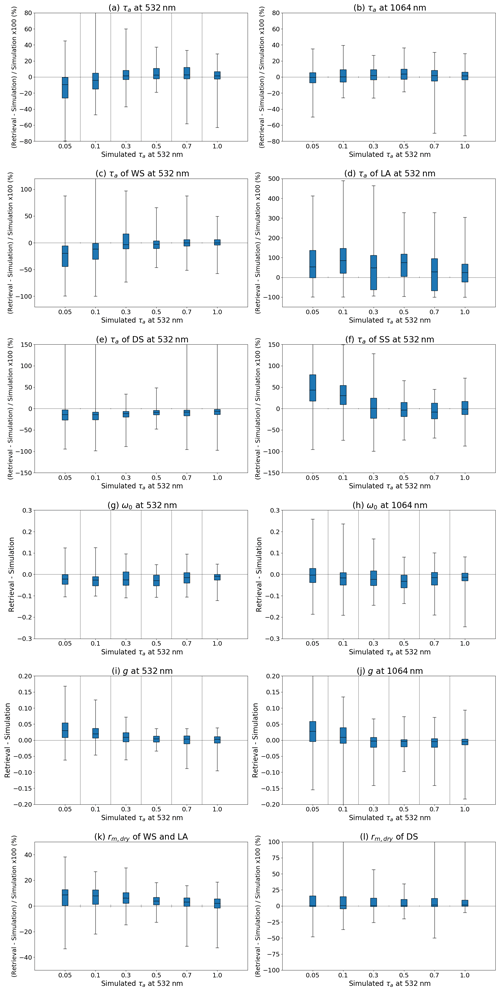
Figure 6Box-and-whisker plots for relative or absolute differences of columnar properties between retrievals and simulations. The box extends from the first quartile to the third quartile of the data, with a line at the median. The whiskers extend from the box to 1.5× interquartile range. The column properties are τa at (a) 532 nm and (b) 1064 nm; τa at 532 nm of (c) WS, (d) LA, (e) DS, and (f) SS; ω0 at (g) 532 nm and (h) 1064 nm; g at (i) 532 nm and (j) 1064 nm; and rm,dry of (k) fine (WS and LA) and (l) coarse (DS) particles.
Table 3Means and standard deviations of differences of columnar properties between retrievals and simulations.
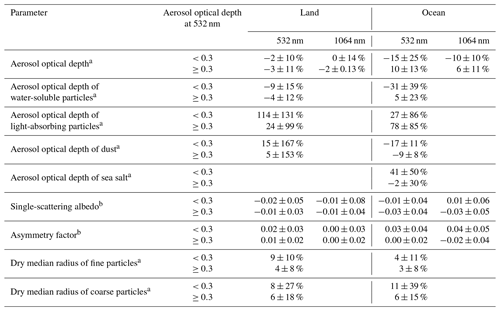
a Differences are calculated by . b Differences are calculated by (retrieval−simulation).
Figure 7 shows the relative differences in αa for WS, LA, DS, and SS between the retrievals and simulations. The relative differences in αa for WS, LA, and DS are very large at altitudes from 3 to 5 km and from 6 to 7 km because αa is very small near the bottom and top edges of the vertical distribution of transported aerosols (see Fig. 4). The relative difference in αa for WS ranges from −30 % to 10 %, and it tends to be underestimated at all altitudes except for the bottom and top edges of the transported aerosol layer. The median value of the relative differences is close to 0 %. The relative difference in αa for LA tends to be overestimated and ranges from −100 % to 200 %. The median value in the boundary layer is close to 0 %, but the variances are large. αa of DS tends to be underestimated; the relative difference ranges from −50 % to 0 %. The relative difference in αa for SS tends to be overestimated; the relative error is from −40 % to 40 %. Table 4 shows the means and standard deviations of these relative differences and the differences for αa, ω0, and g of total aerosols. αa of LA over the land was overestimated, and this was compensated for by the underestimating αa of WS and DS. Hereby, the relative difference of αa for total aerosols was small at about −4 %. In the ocean cases, αa of LA and SS was overestimated, and this was compensated for by underestimating αa of WS and DS. Similar to the results for the columnar properties, the results of αa for the ocean are worse than those over the land.
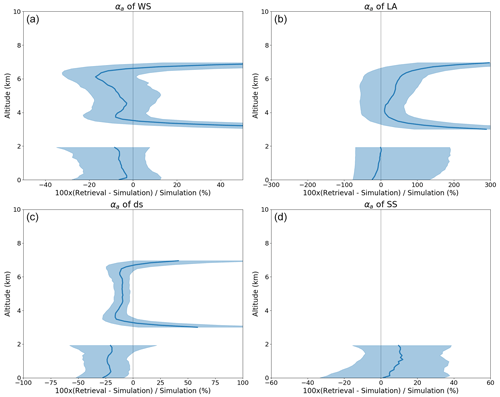
Figure 7Relative differences of αa at 532 nm for (a) WS, (b) LA, (c) DS, and (d) SS between retrievals and simulations. The shading indicates the areas between the first and third quartiles of the data, and the thick lines indicate median values.
Table 4Means and standard deviations of differences of vertically resolved properties between retrievals and simulations.
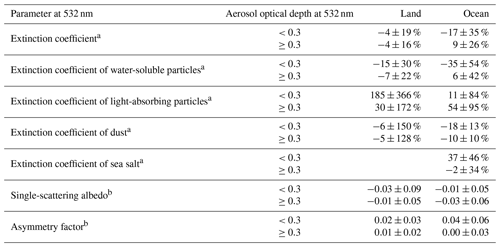
a Differences are calculated by . b Differences are calculated by (retrieval−simulation).
Overall, the uncertainties in the retrieval results over land are smaller than those over the ocean. The retrieval results become better in the larger τa cases. The CALIOP–MODIS retrievals tend to overestimate the amount of LA, and ω0 is underestimated. The retrieval of rm,dry is a challenging problem, but rm,dry of fine (WS and LA) and coarse (DS) particles is estimated well.
5.1 Global 3-D distribution
The annual means of τa and αa in the CALIOP–MODIS retrievals for 2010 are compared with the CALIPSO and MODIS standard products in Fig. 8. The grid resolutions are 5∘ latitude by 2∘ longitude for the CALIOP–MODIS retrieval and the CALIPSO standard product and 1∘ latitude by 1∘ longitude for the MODIS standard product. Note that the MODIS standard product is at 550 nm, but the difference of τa between 532 and 550 nm is small. The horizontal distributions of τa are similar in all results. Large τa values are distributed in the middle of the Atlantic Ocean as well as in Africa and western, southern, and eastern Asia. The global mean ± standard deviation of τa was 0.113±0.161 for the CALIPSO standard product, 0.147±0.148 for the CALIOP–MODIS retrieval, and 0.164±0.145 for the MODIS standard product. Thus, the global mean of the CALIOP–MODIS retrieval was between those of the CALIPSO and MODIS standard products and was close to that of the MODIS standard product. Compared with τa of the AERONET, the CALIPSO version 4 product has a negative bias of (Kim et al., 2018), and τa of the merged data set of the dark target (DT) and deep blue (DB) algorithms in the Aqua MODIS Collection 6.1 product has a small positive bias of 0.004 (Shi et al., 2019). Considering that the CALIOP–MODIS retrieval method used both CALIOP and MODIS observations, the retrieval result is reasonable and is better than the CALIPSO standard product.
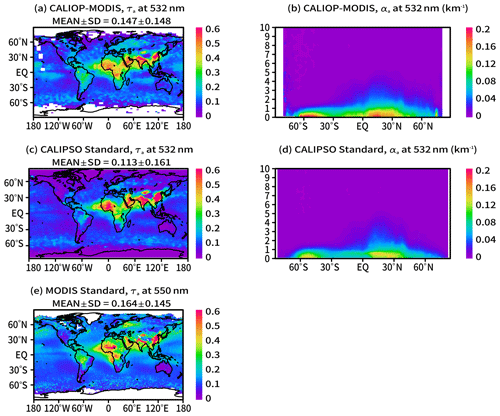
Figure 8Annual means of τa and αa in 2010. The left column shows horizontal distributions of τa, and the right column shows zonal means of αa for the (a, b) CALIOP–MODIS retrieval, (c, d) CALIPSO standard product, and (e) MODIS standard product. At the top of the left panels, MEAN ± SD indicates the global mean and its standard deviation.
The zonal means of αa in all results showed similar distributions to the CALIPSO standard product. αa was large at latitudes from 60 to 40∘ S and from 0 to 30∘ N. The top altitude of the vertical distribution was about 5 km at latitudes from 0 to 30∘ N. In the CALIOP–MODIS retrieval, slightly large αa was observed at altitudes from 0 to 9 km and latitudes from 70 to 80∘ S, and a peak of αa was seen at altitudes from 0 to 1 km and latitudes around 70∘ N. These unnaturally large values in the polar regions may be attributable to cloud contamination. Additionally, since the CALIOP–MODIS retrieval is applied to observation data over the ice surface, it is possible that the high albedo of the ice surface results in the unnatural αa.
We further compared the regional distributions of τa with the CALIOP and MODIS standard products. In North America, South America, and Europe, the CALIOP–MODIS retrieval is close to the MODIS standard product. In Africa, the CALIOP–MODIS retrieval is between the MODIS and CALIOP standard products, but the CALIOP standard product is largest in western Africa, and the CALIOP–MODIS retrieval was smallest in the three products. Additionally, the famous dust source, the Bodélé depression located northeast of Lake Chad in central Africa (Koren et al., 2006), can be clear in the MODIS standard product but cannot be detected in the other two products. The local dust source of the Bodélé depression did not appear in the CALIOP–MODIS retrieval even though the MODIS measurements are utilized. This detection failure of the local dust source may be attributed to the sparse observations of the CALIOP in the longitude direction. In western, southern, and eastern Asia, the CALIOP standard product is larger than the MODIS product, and the CALIOP–MODIS retrieval is between the two standard products. In Australia, the CALIOP–MODIS retrieval was largest. The values of τa in the three products are different by region. Kim et al. (2018) also show the different positive and negative biases by region in comparisons of the CALIOP and MODIS products. The comparisons of τa of the Aqua MODIS Collection 6.1 products with the AERONET products also show that the bias sign is different for the regions and the DT and DB algorithms (Sayer et al., 2019; Shi et al., 2019; Wei et al., 2020; Huang et al., 2020; Eibedingil et al., 2021; Sharma et al., 2021). Further comparisons of the CALIOP–MODIS retrieval with the L2 products of CALIOP and MODIS at the regional scale are necessary in the future.
Figure 9 shows the horizontal distributions of ω0 and g of the CALIOP–MODIS retrieval. The global means of ω0 and g were about 0.940±0.038 and 0.718±0.037. Previous studies have shown that the global mean ω0 is from 0.89 to 0.953 (Korras-Carraca et al., 2019; Kinne, 2019), and the global mean g is 0.702 (Kinne, 2019). Our results are thus consistent with these previous studies. ω0 over land was from 0.8 to 0.95 and was smaller than that over the ocean. g over land was from 0.6 to 0.75 and also smaller than that over the ocean. These differences between land and ocean are due to the presence of SS over the ocean because ω0 and g of SS are larger than those of the other aerosol components (Table 1). In the major biomass burning regions of the central and southern parts of South America as well as the southern part of Africa, ω0 and g of the CALIOP–MODIS retrieval are particularly small: from 0.85 to 0.90 and 0.65 to 0.70, respectively. These are consistent with the results of Kinne (2019). However, our retrieved ω0 is less than 0.90 over most parts of the land area and appears to be about 0.05 smaller than ω0 of Kinne (2019). In Sect. 4, it was shown that the CALIOP–MODIS retrieval tended to underestimate ω0. The tendency to underestimate ω0 might appear in the retrieval over land.
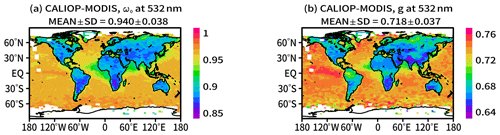
Figure 9Horizontal distributions of the annual means of (a) ω0 and (b) g in 2010 in the CALIOP–MODIS retrieval. At the top of each panel, MEAN ± SD indicates the global mean and its standard deviation.
Figure 10 depicts the horizontal distributions of τa of WS, LA, DS, and SS. Note that the ranges of τa depicted by color bars in Fig. 10 are different. τa of WS was large over South America and Africa, as well as western, southern, and eastern Asia and the ocean. The large τa of WS over the ocean might include contributions from fine SS particles and biogenic sulfate or organic compounds because a large τa of WS was also seen over regions where the surface wind speed is large, such as the sea around Antarctica. A large τa of LA was seen in South America, central Africa, and southern and eastern Asia, which are major sources of aerosols from anthropogenic and biomass burning sources. τa of DS was large around the desert regions of the northern part of Africa, as well as western, southern, and eastern Asia. Compared with WS, LA, and DS, τa of SS was smaller and was uniformly distributed over the ocean, but a peak was found in the Arabian Sea, where there are strong persistent southerly and southwesterly winds from June to September (Chaichitehrani and Allahdadi, 2018) and strong northerly winds, as well as shamal and makran winds, from October to January (Aboobacker et al., 2021). The global mean of τa was 0.072±0.085 for WS, 0.027±0.035 for LA, 0.025±0.054 for DS, and 0.023±0.020 for SS. We compared the global distributions of each component with the previous studies of Kinne (2019), Gkikas et al. (2021), and Korras-Carraca et al. (2021). The global distributions of τa of WS, LA, and SS match those of sulfate+organic, BC, and SS well in Fig. 6 of Kinne (2019) and Fig. 1 of Korras-Carraca et al. (2021). Here, we compared WS of this study with sulfate+organic of Kinne (2019) and Korras-Carraca et al. (2021) because our definition of WS (section “Forward model of aerosol physical and optical properties”) is similar to sulfate+organic. The global distribution of τa of DS was also consistent with those of Kinne (2019), Gkikas et al. (2021), and Korras-Carraca et al. (2021). The global mean of τa for fine particles (WS+LA) in the CALIOP–MODIS retrieval is 0.097, which is greater than 0.063 for fine particles (sulfate+organic+BC) in Kinne (2019) and 0.08 for fine particles (sulfate+organic+BC) in Korras-Carraca et al. (2021). We compared τa of fine particles because LA in this study is defined as an internal mixture of WS and BC, and it is different from the pure BC defined in Kinne (2019) and Korras-Carraca et al. (2021). The global mean of τa for SS was 0.028 in Kinne (2019) and 0.04 in Korras-Carraca et al. (2021). The global mean of τa for DS was 0.031 in Kinne (2019), 0.033 in Gkikas et al. (2021), and 0.03 in Korras-Carraca et al. (2021). Consequently, τa of SS and DS in the CALIOP–MODIS retrieval was slightly smaller than in previous studies, and τa of fine particles is larger than in previous studies. This study represents results from 2010, but the data in Kinne (2019) represent results from 2005, and the data in Korras-Carraca et al. (2021) are means in 1980–2019. Temporal change is one of the plausible causes for the above differences of the fine particles because the emissions of anthropogenic aerosols have large variability (Quaas et al., 2022).
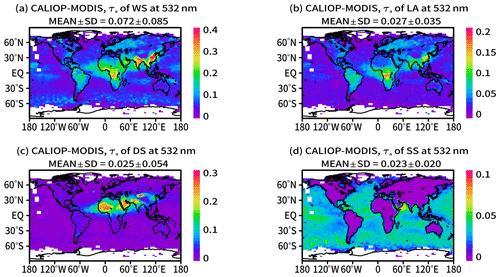
Figure 10Horizontal distributions of the annual means of τa of (a) WS, (b) LA, (c) DS, and (d) SS in 2010 in the CALIOP–MODIS retrieval. At the top of each panel, MEAN ± SD indicates the global mean and its standard deviation.
Figure 11 shows the zonal means of αa of WS, LA, DS, and SS. Note that the range of αa depicted by the color bar in Fig. 11b is smaller than those in Fig. 11a, c, and d. The distribution of WS is almost the same as that of total aerosols (Fig. 8b and d). αa of WS was largest among the four aerosol components, and αa of LA was smallest. The distribution of DS is concentrated between latitudes of 0 and 50∘ N, and the top altitude is about 5 km. SS is distributed across all latitudes, and its top altitude is about 1 km.
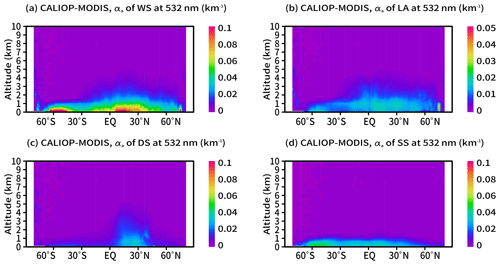
Figure 11Zonal means of αa of (a) WS, (b) LA, (c) DS, and (d) SS in 2010 in the CALIOP–MODIS retrieval.
Figure 12 shows rm,dry of WS, LA, DS, and SS particles. rm,dry of WS, LA, and DS is large over land and small over the ocean. This result indicates that particle size decreases away from the source regions due to dry deposition. rm,dry of SS is the result of the parameterization using the ocean surface wind speed. Because rm,dry of SS increases with an increase in wind speed, it is large in the midlatitudes, where cyclones caused by baroclinic instability occur frequently.
5.2 Comparisons with AERONET products
The CALIOP–MODIS retrieval results in 2010 were compared with the AERONET products. The CALIOP measurements are near nadir (∼3∘ off nadir) and include no swath observations. Most AERONET sites are far from the CALIPSO ground track. Because mesoscale variability is a common feature of lower-tropospheric aerosols (Anderson et al., 2003), Omar et al. (2013) introduced criteria for the coincidence: a CALIPSO overpass with an AERONET site ±2 h and within a 40 km radius of the AERONET site. Schuster et al. (2012) used the coincidence criteria of ±30 min within an 80 km radius and a CALIOP digital elevation model surface elevation within 100 m of the AERONET site elevation. In this study, we used coincidence criteria of ±2 h within a 40 km radius of an AERONET site and within ±100 m of the AERONET site elevation. We thus compared the means of CALIOP–MODIS retrievals satisfying these spatial criteria with the means of AERONET retrievals within ±2 h. A total of 91 samples for 51 AERONET stations (Fig. S1 in the Supplement) met these criteria. The columnar properties of τa at 532 and 1064 nm, ω0 at 532 nm, g at 532 nm, and the fine and coarse median radii of the volume size distribution at the ambient relative humidity were compared (Fig. 13). The AERONET optical properties at 532 and 1064 nm were calculated from the data at the AERONET wavelengths of 440, 500, 675, and 870 nm by linear interpolation and extrapolation in a log–log space. We used τa directly derived from the sun-direct measurements, and ω0, g, and the fine and coarse median radii of the volume size distribution are the results of the almucantar retrievals in the AERONET level 2 product. The fine and coarse median radii of the CALIOP–MODIS retrieval data were calculated from the column-integrated volume size distribution by the same method as that used for AERONET data (Dubovik et al., 2002).
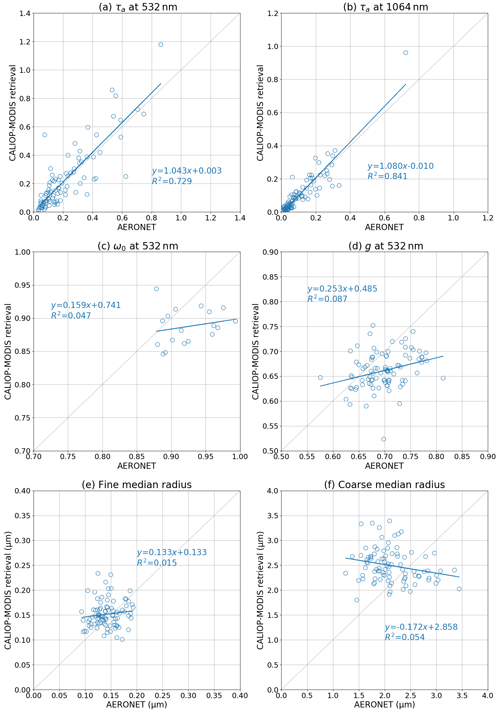
Figure 13Comparisons of the columnar properties between the AERONET products and CALIOP–MODIS retrieval: τa at (a) 532 nm and (b) 1064 nm, (c) ω0 at 532 nm, (d) g at 532 nm, (e) fine median radius, and (f) coarse median radius. The linear regression results are shown as equations in the form , and R2 is the coefficient of determination.
τa at 532 and 1064 nm of CALIOP–MODIS retrievals agreed well with those of AERONET; the slopes of the relationships were almost 1.0. The means and standard deviations of the relative differences between the CALIOP–MODIS retrievals and AERONET products were 9±80 % for τa at 532 nm and % for τa at 1064 nm.
ω0 plots were fewer than those of the other parameters. ω0 retrieved from the sun–sky photometry has high uncertainty when τa is small (Sinyuk et al., 2020; Kudo et al., 2021), and the AERONET level 2 product does not provide the retrieved ω0 when τa at 440 nm is less than 0.4. The coefficient of determination in the ω0 comparison was small, and the CALIOP–MODIS retrievals were underestimated. The mean ± standard deviation of the absolute differences of ω0 at 532 nm was . The coefficient of determination for the g comparison was also small, and the CALIOP–MODIS retrievals were slightly underestimated. The mean ± standard deviation of the absolute differences of g at 532 nm was . The coefficient of determination for the fine median radius of the CALIOP–MODIS retrieval was small at 0.015. However, the fine median radii of both the CALIOP–MODIS retrieval and the AERONET product lay in the same range from 0.1 to 0.2 µm, and the mean ± standard deviation of the absolute differences was 0.01±0.03 µm. The comparison of the coarse median radius also showed a small coefficient of determination of 0.054. However, the mean ± standard deviation of the absolute difference was small at 0.35±0.62 µm because the coarse median radii of the CALIOP–MODIS retrieval and the AERONET product lay in a similar range from 1.0 to 3.5 µm.
In summary, τa at 532 and 1064 of the CALIOP–MODIS retrievals showed good agreement with those of the AERONET products. ω0, g, and fine and coarse median radii were not retrieved well, but their values were not far from those of the AERONET products. The vertical profile of αa was not compared with ground-based measurements in this study. In the future, we will compare the vertical profile of αa with HSRL and Raman lidar measurements in AD-Net (Nishizawa et al., 2017; Jin et al., 2022).
5.3 Influences of particle models
The assumed particle model is important in the retrieval of aerosols. We therefore investigated how different particle models influenced the retrievals by comparing the results when the spheroid particle model for DS was used in the retrievals instead of the Voronoi particle model. Figures S2 and S3 show the differences of the retrieval results between the spheroid and Voronoi particle models. τa of DS for the retrieval with the spheroid model was greater than that for the retrieval with the Voronoi model (Fig. S2). Because δa of the spheroid particle model is smaller than that of the Voronoi model (Table 1), a large amount of DS was required to fit δ calculated by the forward model to δ measurements when the spheroid model was used. τa of WS and LA was decreased to compensate for the increase in τa of DS. The retrieved rm,dry of DS was decreased (Fig. S3) by as much as about 0.6 µm in the heavy dust regions of Africa and western Asia. In Sect. 3.1.3, we showed that the median radius can be estimated from the spectral information of the scattering intensity. The scattering intensity ratio for spheroid particles changes from 0.8 to 3.0 in the range of the median radius from 1.0 to 5.0 µm, whereas the ratio of the scattering intensity for Voronoi particles changes from 0.8 to 2.6 in the median radius range from 1.0 to 5.0 µm (Fig. 2a). Since the scattering intensity ratio for spheroid particles is larger than that for Voronoi particles in the range from 1.0 to 5.0 µm, the retrieved rm,dry of DS in the retrieval with the spheroid particle model was smaller than that in the retrieval with the Voronoi model. rm,dry of WS and LA was not influenced by the particle model used for DS.
The fixed volume fraction of BC is one of the assumptions associated with large uncertainties in this study. We therefore conducted the retrieval using LA with a BC volume fraction of 15 % instead of 30 %. Table 5 and Fig. S4 show the difference in the retrieval results between BC volume fractions of 15 % and 30 %. τa of WS and LA was slightly decreased (Fig. S4b and c). The decrease in the global mean τa was less than 0.01, but the decrease was large (up to 0.03) in Africa and western, southern, and eastern Asia. These results can be explained by the changes in ω0 and Sa. ω0 of LA with a BC fraction of 15 % is greater than that with a BC fraction of 30 %, and Sa of LA with a BC fraction of 15 % is smaller than that with a BC fraction of 30 % (Table 1). Larger ω0 and smaller Sa induce an increase in the values of the MODIS radiances and the CALIOP backscatter coefficients calculated by the forward models. As a result, smaller τa and αa are retrieved. The influence of the BC volume fraction on the retrieved τa of DS and SS (Fig. S4d and e) and on rm,dry of the fine (WS and LA) and coarse (DS) particles was negligible (Table 5).
Table 5Means and standard deviations of the retrieval results using different particle models compared with the retrieval result using the Voronoi model for dust and the core–grey shell 30 %* model for light-absorbing particles.
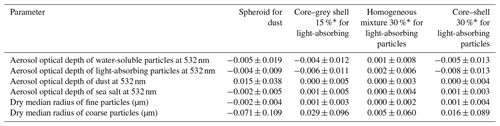
* Volume fraction of black carbon in a particle.
We also investigated the differences in retrievals when the CGS, CS, and MG models were used. The impacts on the retrieved τa are summarized in Table 5. The retrieval using MG slightly increased τa of LA because of a slightly large Sa (Table 1). Conversely, the retrieval using CS decreased τa of LA because Sa of CS was smaller than that of CGS (Table 1). Different mixture models affected only the WS and LA retrievals, and the impact on the global mean τa was less than 0.01.
5.4 Clear-sky shortwave direct radiative forcing
The clear-sky SDRE values of aerosols at the bottom and top of the atmosphere as well as the impacts of aerosols on the atmospheric heating rate were calculated from the retrieval results described in Sect. 5.1. The annual mean of the SDRE at the top of the atmosphere was W m−2 (Fig. 14). Korras-Carraca et al. (2019) summarized the SDRE obtained by previous studies based on CALIOP and MODIS observations and chemical transport models, and Korras-Carraca et al. (2021) show the SDRE of the 40-year climatology of the MERRA-2 reanalysis data. These previously obtained SDRE values ranged from −2.6 to −7.3 W m−2, for τa from 0.074 to 0.18, and for ω0 from 0.89 to 0.97. Our results are thus in the range of previously obtained values. The horizontal distribution of the SDRE was also similar to those of previous studies (Korras-Carraca et al., 2019, 2021), and positive forcing was observed over desert and snow–ice surfaces with a large surface albedo. An advantage of this study is that the SDRE of each aerosol component was determined. The global mean SDRE of WS was W m−2, whereas the global mean SDRE of LA was 0.22±0.94 W m−2, and the SDRE of LA was positive in almost all regions. The global mean SDRE of DS was W m−2, but the SDRE of DS was positive over desert and snow–ice surfaces. The SDRE of SS was negative worldwide at W m−2. Korras-Carraca et al. (2021) also show the global mean of the SDRE at the top of the atmosphere for each component, and the SDRE is −1.88 W m−2 for sulfate, −0.73 W m−2 for organic carbon, 0.19 W m−2 for BC, −0.83 W m−2 for DS, and −1.62 W m−2 for SS. The SDRE of WS in this study is close to the SDRE of sulfate+organic carbon of Korras-Carraca et al. (2021). However, note that a simple addition of the SDRE for sulfate and organic carbon does not accurately represent the SDRE of sulfate+organic carbon because the SDRE responds nonlinearly to the changes in the aerosol optical properties. The SDREs of LA and DS are also consistent with those of Korras-Carraca et al. (2021). Only the SDRE of SS in this study was smaller than that of Korras-Carraca et al. (2021) because τa of SS of Korras-Carraca et al. (2021) is 0.04 and is greater than this study.
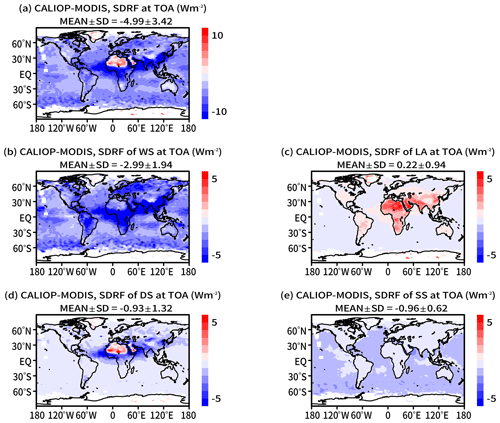
Figure 14Horizontal distributions of the annual means of the SDRE of (a) total aerosols, (b) WS, (c) LA, (d) DS, and (e) SS at top of the atmosphere (TOA) in 2010. At the top of each panel, MEAN ± SD indicates the global mean and its standard deviation.
The SDRE at the bottom of the atmosphere was negative in all regions, and the global mean was W m−2 (Fig. 15). Previously reported values ranged from −10.7 to −6.64 W m−2 (Korras-Carraca et al., 2019, 2021). The CALIOP–MODIS retrieval result was more negative than the previous study results. The global mean of the SDRE for each component was W m−2 for WS, W m−2 for LA, W m−2 for DS, and W m−2 for SS. Although τa of LA was smaller than τa of WS (Fig. 10), the SDRE of LA was largest. Furthermore, whereas τa of DS was comparable to that of SS, the SDRE of DS was larger than that of SS. The small ω0 of LA and DS decreases the diffuse irradiance reaching the surface, with the result that the SDRE at the bottom of the atmosphere becomes large (Kudo et al., 2010b). Korras-Carraca et al. (2021) show that the global mean of the SDRE at the bottom of the atmosphere is −1.86 W m−2 for sulfate, −0.91 W m−2 for organic carbon, −0.72 W m−2 for BC, −1.98 W m−2 for DS, and −1.74 W m−2 for SS. The global distributions of the SDRE for each component in this study were consistent with those of Korras-Carraca et al. (2021), but the magnitudes of the SDRE were significantly different, particularly in the results for fine particles (WS and LA). The value of τa for the fine mode is 0.099 in the CALIOP–MODIS retrieval (WS+LA) and 0.08 in Korras-Carraca et al. (2021) (sulfate+organic+BC). The difference of τa is small. Since a small value of ω0 results in large SDRE at the bottom of the atmosphere, the underestimation of ω0 in the CALIOP–MODIS retrieval (Sects. 4, and 5.2) is a possible cause. Further studies regarding the differences of the aerosol optical properties and the configuration of the radiative transfer model are necessary in the future. Figure 16 shows the zonal means of the aerosol impacts on the heating rate. The vertical distribution of the impacts of the total aerosols corresponds to the distribution of αa (Fig. 8). The maximum heating rate was about 0.5 K d−1. Korras-Carraca et al. (2019) also found that the aerosol impact on the heating rate was large in the boundary layer, with a maximum value of about 0.5 K d−1. LA had the largest impact on the heating rate because of its small ω0, despite its small αa (Fig. 11). The values at altitudes from 0 to 9 km and latitudes from 70 to 80∘ S in Fig. 16a, b, c, and d were unnatural. These unnatural values correspond to the unnatural αa described in Sect. 5.1. Cloud contamination and high surface albedo of ice are possible causes. We showed that the CALIOP–MODIS retrieval overestimates the amount of LA and underestimates ω0, and the SDRE at the bottom of the atmosphere is more negative than in previous studies. Considering these factors, the impacts of LA on the heating rate might be overestimated. The aerosol-induced changes in the atmospheric heating rate affect the atmospheric stability and regional dynamics (Yu et al., 2002; Huang et al., 2014; Kudo et al., 2018). Improvement in retrieving LA and single-scattering albedo (SSA) is necessary.
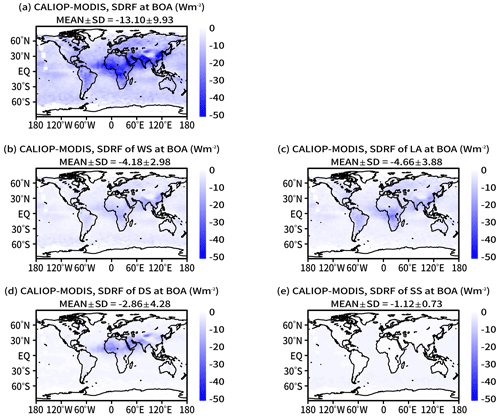
Figure 15Horizontal distributions of the annual means of the SDRE values of (a) total aerosols, (b) WS, (c) LA, (d) DS, and (e) SS at the bottom of the atmosphere (BOA) in 2010. At the top of each panel, MEAN ± SD indicates the global mean and its standard deviation.
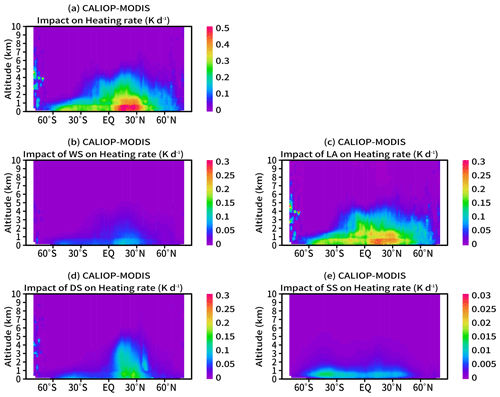
Figure 16Annual means of impacts of (a) total aerosols, (b) WS, (c) LA, (d) DS, and (e) SS on heating rates in 2010.
To summarize, the SDRE values calculated from the CALIOP–MODIS retrievals are consistent with those of previous studies. However, SDRE values at the bottom of the atmosphere were larger than in previous studies. LA had a significant impact on the SDRE at the top and bottom of the atmosphere and on the heating rate. However, the CALIOP–MODIS retrievals tended to overestimate the amount of LA and underestimate ω0. Thus, the retrieval of LA needs to be improved in the future.
We developed the CALIOP–MODIS retrieval method for the observation of the global three-dimensional distribution of aerosol composition. The CALIOP–MODIS retrieval optimizes the aerosol composition to both CALIOP and MODIS observations in the daytime. In this study, aerosols were assumed to consist of four components: WS, LA, DS, and SS. The CALIOP–MODIS retrieval optimizes the vertical profiles of Vdry of the four components and rm,dry of fine (WS and LA) and coarse (DS) particles to the CALIOP and MODIS observations. The outputs of the CALIOP–MODIS retrieval are the vertical profiles of αa, ω0, and g of total aerosols (WS+LA+DS+SS), as well as αa of WS, LA, DS, and SS, and their columnar integrated or mean values.
The uncertainties in the retrieval products were evaluated by using simulated data from the CALIOP and MODIS observations. Simulations were conducted for 16 aerosol vertical profile patterns by assuming the actual scenes in the daytime, including transport of dust, biomass burning, and polluted dust with different τa for total aerosols, different land (grass, desert, and snow) and ocean (different values of surface wind speed) surfaces, and different solar zenith angles. Random errors were also added to the CALIOP and MODIS observations, surface albedo, and surface wind speed. Overall, the performance of the CALIOP–MODIS retrievals was good. The retrieval results in the case of land surfaces were better than those for the ocean surface because three components, excluding SS, were retrieved over the land surface, whereas four components were retrieved over the ocean surface. The retrieval results became better when τa was increased. However, the amount of LA tended to be overestimated regardless of τa and land or ocean surface; hence, ω0 tended to be underestimated.
Daytime observation data from CALIOP and MODIS in 2010 were analyzed by the CALIOP–MODIS retrieval. The global mean of τa for total aerosols was 0.147±0.148. Comparison with the CALIPSO and MODIS standard products showed that τa of the CALIOP–MODIS retrieval was between those of the CALIPSO and MODIS standard products and was close to the MODIS standard product. Since previous studies show that the CALIPSO standard product tends to underestimate τa and the MODIS standard product has a small positive bias, the results obtained are reasonable and are better than the CALIOP standard product. The horizontal distribution of τa for total aerosols in the CALIOP–MODIS retrieval was generally similar to the distributions in the CALIPSO and MODIS standard products. However, there were some regional differences between the CALIOP–MODIS retrieval and the CALIOP and MODIS standard products. The vertical distribution of the CALIOP–MODIS retrieval was also similar to that in the CALIPSO standard product. Additionally, the unnaturally large αa due to cloud contamination and high surface albedo of ice was found in both polar regions in the CALIOP–MODIS retrievals.
The global means of τa for each component were 0.072±0.085 for WS, 0.027±0.035 for LA, 0.025±0.054 for DS, and 0.023±0.020 for SS. The global distributions of WS, LA, DS, and SS in this study were consistent with those for sulfate+organic, BC, DS, and SS in the previous studies of Kinne (2019), Gkikas et al. (2021), and Korras-Carraca et al. (2021). The global means of τa for DS and SS in this study were slightly smaller than those of Kinne (2019), Gkikas et al. (2021), and Korras-Carraca et al. (2021), whereas the global mean of τa for the fine particle (WS+LA) was greater than the fine particles (sulfate+organic+BC) of Kinne (2019) and Korras-Carraca et al. (2021). The data in Kinne (2019) represent results in 2005, and the data in Korras-Carraca et al. (2021) are means in 1980 to 2019, but this study represents results for 2010. Since anthropogenic aerosol emissions have large temporal variability (Quaas et al., 2022), we need to compare the result in the same period for further investigation.
Using the retrieval results for 2010, τa, ω0, g, and fine and coarse median radii of the CALIOP–MODIS retrievals were compared with the corresponding AERONET products. τa at 532 and 1064 nm of the CALIOP–MODIS retrieval agreed well with the AERONET product. The values of ω0, g, and fine and coarse median radii of the CALIOP–MODIS retrievals were not far from those of the AERONET products, but the variations were large and the coefficients of determination for linear regressions between the CALIOP–MODIS retrievals and the AERONET products were small. ω0 and g were underestimated by about 0.04 compared with those of the AERONET.
The assumed particle model used in the retrieval causes large uncertainties. We investigated the influences of the DS shapes (Voronoi or spheroid models), the volume fractions of BC in LA (15 % or 30 %), and the internal mixtures of LA (CGS, MG, and CS models). The DS shapes had large impacts on DS, WS, and LA, and the difference of global mean of τa was about 0.015 for DS and less than 0.01 for WS and LA. The influences of the volume fractions and internal mixtures of LA were small (less than 0.01 for the global mean τa of WS and LA), but the influences cannot be ignored at the regional scale. The difference of τa of LA was greater than 0.01 around the central part of Africa, which is a known biomass burning region.
The clear-sky SDRE of aerosols at the top and bottom of the atmosphere and the impact of aerosols on the heating rate were investigated using the retrievals for 2010. The SDRE values at the TOA and BOA were and W m−2, respectively. The SDRE at the TOA is in the range of previously reported values (from −2.6 to −7.3 W m−2). However, the SDRE at the BOA was greater than previously reported values (from −10.7 to −6.64 W m−2). The aerosol impact on the heating rate ranged from 0.0 to 0.5 K d−1, consistent with previously reported values. The horizontal distributions of the SDRE at the TOA and BOA, as well as the vertical distributions of the aerosol impacts on the heating rate, were consistent with those of previous studies. An advantage of this study was that the SDRE was estimated for each aerosol component. The aerosol optical depth (AOD) of WS was largest among the four aerosol components: the SDRE of WS at the TOA and BOA was large, but the impact of WS on the heating rate was small because WS is a less light-absorbing particle. In contrast, τa of LA was small, but its SDRE at the TOA was positive in most of the world, and its SDRE at the BOA and its impact on the heating rate were the largest among the four aerosol components. Thus, although the amount of LA was small, the impact on the SDRE was significant. The SDREs at the TOA and BOA for each component were compared with those of Korras-Carraca et al. (2021). The distributions of the SDRE at the TOA and BOA for each component were consistent with those of Korras-Carraca et al. (2021). Furthermore, the global mean values of the SDRE at the TOA agreed well with each other. However, the SDREs at the BOA for each component in this study were greater than those of Korras-Carraca et al. (2021), particularly for the fine particles (WS and LA). A possible reason except for the configuration of the radiative transfer model would be the differences of the aerosol optical properties. τa for the fine particle (WS+LA) was slightly greater than the fine particles (sulfate+organic+BC) of Korras-Carraca et al. (2021), and the CALIOP–MODIS retrieval overestimates the amount of LA and underestimates SSA. The smaller SSA results in more negative SDRE at the TOB.
Consequently, τa and αa of the CALIOP–MODIS retrieval in 2010 showed reasonable results when compared with the CALIPSO and MODIS standard products, the AERONET products, and previous studies. Furthermore, the SDRE values calculated from the CALIOP–MODIS retrievals were consistent with those of previous studies. However, there were some issues with the CALIOP–MODIS retrievals. τa of LA tended to be overestimated, and ω0 was underestimated. Because the LA has a large impact on the SDRE, the overestimation of LA should be improved in a future study. The unnaturally large αa in both polar regions is also an important issue. Further investigations for the cloud discrimination and the surface albedo of ice-covered regions are necessary. There were some differences of τa between the CALIOP–MODIS retrieval and the CALIOP and MODIS standard product at the regional scale. A further validation study using the AERONET product is required. Additionally, the vertical profile of αa was not validated in this study. We will compare the vertical profile of αa with ground-based measurements by HSRL and Raman lidar of AD-Net in the future.
The Earth Clouds, Aerosol and Radiation Explorer (EarthCARE) satellite is a joint mission of the European Space Agency and the Japanese Aerospace Exploration Agency (Illingworth et al., 2015). The atmospheric lidar (ATLID) on board EarthCARE is a linearly polarized HSRL transmitting a spectrally narrow laser beam at 355 nm. The multispectral imager (MSI) on board EarthCARE is an imager with seven bands from visible to infrared wavelengths. We are developing the application of the CALIOP–MODIS retrieval to the ATLID and MSI observations. The lidar ratio is an optical parameter related to ω0 and can be directly retrieved from the ATLID measurements. Hereby, the EarthCARE observations may improve the retrieval of ω0 and LA. Furthermore, we plan to investigate long-term changes in the aerosol composition by using the CALIOP and MODIS observations together with ATLID and MSI observations. The results will contribute to our understanding of climate changes due to aerosols.
BC has a complex morphology and forms mixtures with weakly light-absorbing particles. Previous studies have developed various simplified models, such as externally mixed homogeneous spheres, an internally mixed homogeneous sphere, and the CS model. Comparison with realistic encapsulated aggregate models has shown that the externally mixed homogeneous spheres and the CS model underestimate the absorption cross section and that the internally mixed homogeneous sphere overestimates the absorption cross section (Kahnert et al., 2012). The CGS model, developed by Kahnert et al. (2013), has a CS geometry, but compared with the original CS model with the same volume of BC and weakly light-absorbing particles, the volume fraction of the BC core to the total BC volume in a particle (fcore) is smaller than the one in the CGS model, and the remaining BC (1−fcore) is homogeneously mixed with weakly light-absorbing particles in the shell. The Maxwell Garnett mixing rule is used for the homogeneous mixing in the shell. The optical properties of the CGS model are better representations of a realistic encapsulated aggregate model than the externally mixed homogeneous spheres, internally mixed homogeneous sphere, and the CS model.
Kahnert et al. (2013) considered the CGS model to consist of BC and sulfate, and the value of fcore was optimized to the optical properties of a realistic encapsulated aggregate model. However, we assumed that LA is a mixture of BC and WS, instead of BC and sulfate. WS is defined as a mixture of sulfates, nitrates, and organic and water-soluble substances (Hess et al., 1998), and ω0 of WS is smaller than that of pure sulfate. The optimized values of fcore in Kahnert et al. (2013) cannot be applied in this study. Therefore, we optimized fcore to the optical properties of the Voronoi aggregate model with BC and WS (Ishimoto et al., 2019). The core of the model is a BC aggregate with a polyhedral Voronoi structure, and the adhering WS shell is created by a simple model of surface tension derived from the artificial surface potential. The refractive index of the BC was obtained from the measurements of Chang and Charalampopoulos (1990). The refractive index of WS depends on the relative humidity and was obtained from the OPAC database (Hess et al., 1998). The optical properties of the Voronoi aggregate model were computed by the finite-difference time-domain method (Ishimoto et al., 2012) and discrete-dipole approximation (DDSCAT version 7.3; https://code.google.com/archive/p/ddscat/, last access: 25 December 2018; Draine and Flatau, 1994). The database of optical properties of the Voronoi aggregate model was created under the following conditions. The volume ratio of shell to core (VR) was 0.0, 2.0, 5.0, 10.0, and 20.0. The volume-equivalent sphere radius was 10 sizes for each VR, and the radius range was from 0.02 to 0.2 µm for VR =0.0 and from 0.06 to 0.6 µm for VR =20.0. The relative humidity was 0, 50, 90, and 98 %. The wavelength was 340, 355, 380, 400, 500, 532, 675, 870, 1020, and 1064 nm. These are typical wavelengths of lidar and sky radiometer (Nakajima et al., 2020) measurements. The value of fcore was optimized to the optical properties of the Voronoi aggregate model by the following procedure.
- 1.
fcore was changed from 0 to 1 with a step of 0.1.
- 2.
Optical properties (absorption cross section, ω0, and g) of the CGS model with different values of fcore were calculated.
- 3.
The following objective function was calculated from the optical properties of the CGS and Voronoi aggregate models:
where CGS and Voronoi indicate the CGS and Voronoi aggregate models, σabs is the absorption cross section, r is the volume-equivalent sphere radius, RH is relative humidity, and VR is the volume ratio of shell to core. The objective function was calculated for each wavelength and for two particle size ranges, r<0.1 µm and r≥0.1 µm.
Table A1Objective function (Eq. A1) for different volume fractions of BC core (fcore) in a particle, volume-equivalent sphere radius ranges, and wavelengths. Bold text indicates the minimum value of the objective function in each row.
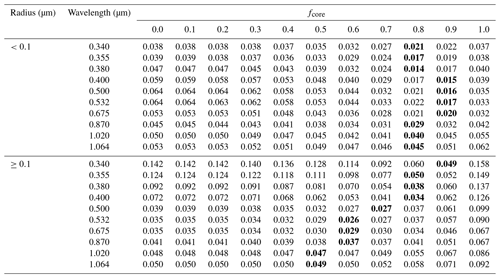
Table A1 shows the objective functions for different values of fcore. The values of fcore=0 and fcore=1 correspond to an internally mixed homogeneous sphere and the CS model, respectively. For r<0.1 µm, the optimized values of fcore were 0.8 or 0.9, and the optimized CGS was close to CS. This result is caused by the fact that there are few monomers composing the Voronoi aggregate model when the particle radius is small, and the geometry of the Voronoi aggregate model is close to CS. For r≥0.1 µm, the fcore results were from 0.5 to 0.9. The optimized CGS approached that for internally mixed homogeneous spheres as the wavelength increased. The same wavelength dependency was seen in the results of Kahnert et al. (2013). The optimized fcore in Table A1 was used for the calculation of the optical properties of the CGS.
The results of the CALIOP–MODIS retrievals are available on request by contacting the first author of the paper.
The supplement related to this article is available online at: https://doi.org/10.5194/amt-16-3835-2023-supplement.
RK developed the codes of the CALIOP–MODIS retrievals, performed the numerical experiments, and analyzed the retrieval results. AH, EO, and MF processed the CALIOP and MODIS measurements and ancillary data before the retrievals. HI developed the databases of the Voronoi particle models. TN planned the synergistic remote sensing by CALIOP and MODIS and managed this project. RK prepared the paper with contributions from all co-authors.
The contact author has declared that none of the authors has any competing interests.
Publisher's note: Copernicus Publications remains neutral with regard to jurisdictional claims in published maps and institutional affiliations.
We gratefully acknowledge the use of PSTAR of the OpenCLASTR (https://ccsr.aori.u-tokyo.ac.jp/~clastr/, last access: 15 August 2023) and GRASP spheroid package (https://www.grasp-open.com, last access: 15 August 2023). We thank the teams involved with CALIPSO, MODIS, and MERRA-2 for their efforts in providing their products. We also thank the AERONET principal investigators and their staff for establishing and maintaining AERONET at the 51 sites used in this investigation.
This research has been supported by the Japan Society for the Promotion of Science (grant no. 15H02808).
This paper was edited by Vassilis Amiridis and reviewed by four anonymous referees.
Aboobacker, V. M., Shanas, P. R., Al-Ansari, E. M. A. S., Kumar, V. S., and Vethamony, P.: The maxima in northerly wind speeds and wave heights over the Arabian Sea, the Arabian/Persian Gulf and the Red Sea derived from 40 years of ERA5 data, Clim. Dynam., 56, 1037–1052, https://doi.org/10.1007/s00382-020-05518-6, 2021.
Ackerman, S. A., Frey, R., Strabala, K., Liu, Y., Gumley, L., Baum, B., and Menzel, P.: MODIS Atmosphere L2 Cloud Mask Product, NASA MODIS Adaptive Processing System, Goddard Space Flight Center [data set], USA, https://doi.org/10.5067/MODIS/MOD35_L2.006, 2015.
Amiridis, V., Marinou, E., Tsekeri, A., Wandinger, U., Schwarz, A., Giannakaki, E., Mamouri, R., Kokkalis, P., Binietoglou, I., Solomos, S., Herekakis, T., Kazadzis, S., Gerasopoulos, E., Proestakis, E., Kottas, M., Balis, D., Papayannis, A., Kontoes, C., Kourtidis, K., Papagiannopoulos, N., Mona, L., Pappalardo, G., Le Rille, O., and Ansmann, A.: LIVAS: a 3-D multi-wavelength aerosol/cloud database based on CALIPSO and EARLINET, Atmos. Chem. Phys., 15, 7127–7153, https://doi.org/10.5194/acp-15-7127-2015, 2015.
Anderson, T. L., Charlson, R. J., Winker, D. M., Ogren, J. A., and Holmén, K.: Mesoscale variations of tropospheric aerosols, J. Atmos. Sci., 60, 119–136, https://doi.org/10.1175/1520-0469(2003)060<0119:MVOTA>2.0.CO;2, 2003.
Aoki, T., Tanaka, T. Y., Uchiyama, A., Chiba, M., Mikami, M, Yabuki, S., and Key, J. R.: Sensitivity experiments of direct radiative forcing caused by mineral dust simulated with a chemical transport model, J. Meteorol. Soc. Jpn., 83A, 315–331, https://doi.org/10.2151/jmsj.83A.315, 2005.
Arias, P. A., Bellouin, N., Coppola, E., Jones, R. G., Krinner, G., Marotzke, J., Naik, V., Palmer, M. D., Plattner, G.-K., Rogelj, J., Rojas, M., Sillmann, J., Storelvmo, T., Thorne, P. W., Trewin, B., Achuta Rao, K., Adhikary, B., Allan, R. P., Armour, K., Bala, G., Barimalala, R., Berger, S., Canadell, J. G., Cassou, C., Cherchi, A., Collins, W., Collins, W.D., Connors, S. L., Corti, S., Cruz, F., Dentener, F. J., Dereczynski, C., Di Luca, A., Diongue Niang, A., Doblas-Reyes, F. J., Dosio, A., Douville, H., Engelbrecht, F., Eyring, V., Fischer, E., Forster, P., Fox-Kemper, B., Fuglestvedt, J. S., Fyfe, J. C., Gillett, N. P., Goldfarb, L., Gorodetskaya, I., Gutierrez, J. M., Hamdi, R., Hawkins, E., Hewitt, H. T., Hope, P., Islam, A. S., Jones, C., Kaufman, D. S., Kopp, R. E., Kosaka, Y., Kossin, J., Krakovska, S., Lee, J.-Y., Li, J., Mauritsen, T., Maycock, T. K., Meinshausen, M., Min, S.-K., Monteiro, P. M. S., Ngo-Duc, T., Otto, F., Pinto, I., Pirani, A., Raghavan, K., Ranasinghe, R., Ruane, A.C., Ruiz, L., Sallée, J.-B., Samset, B. H., Sathyendranath, S., Seneviratne, S. I., Sörensson, A. A., Szopa, S., Takayabu, I., Tréguier, A.-M., van den Hurk, B., Vautard, R., von Schuckmann, K., Zaehle, S., Zhang, X., and Zickfeld, K.: Technical Summary, in: Climate Change 2021: The Physical Science Basis. Contribution of Working Group I to the Sixth Assessment Report of the Intergovernmental Panel on Climate Change, edited by: Masson-Delmotte, V., Zhai, P., Pirani, A., Connors, S. L., Péan, C., Berger, S., Caud, N., Chen, Y., Goldfarb, L., Gomis, M. I., Huang, M., Leitzell, K., Lonnoy, E., Matthews, J. B. R., Maycock, T. K., Waterfield, T., Yelekçi, O., Yu, R., and Zhou, B., Cambridge University Press, Cambridge, United Kingdom and New York, NY, USA, 33–144, https://doi.org/10.1017/9781009157896.002, 2021.
Asano, S. and Shiobara, M.: Aircraft measurements of the radiative effects of tropospheric aerosols: I. Observational results of the radiation budget, J. Meteorol. Soc. Jpn., 67, 847–861, https://doi.org/10.2151/jmsj1965.67.5_847, 1989.
Bösenberg, J., Matthias, V., Amodeo, A., Amoiridis, V., Ansmann, A., Baldasano, J. M., Balin, I., Balis, D., Böckmann, C., Boselli, A., Carlsson, G., Chaikovsky, A., Chourdakis, G., Comerón, A., De Tomasi, F., Eixmann, R., Freudenthaler, V., Giehl, H., Grigorov, I., Hågård, A., Iarlori, M., Kirsche, A., Kolarov, G., Komguem, L., Kreipl, S., Kumpf, W., Larchevêque, G., Linné, H., Matthey, R., Mattis, I., Mekler, A., Mironova, I., Mitev, V., Mona, L., Müller, D., Music, S., Nickovic, S., Pandolfi, M., Papayannis, A., Pappalardo, G., Pelon, J., Pérez, C., Perrone, R. M., Persson, R., Resendes, D. P., Rizi, V., Rocadenbosch, F., Rodrigues, A., Sauvage, L., Schneidenbach, L., Schumacher, R., Shcherbakov, V., Simeonov, V., Sobolewski, P., Spinelli, N., Stachlewska, I., Stoyanov, D., Trickl, T., Tsaknakis, G., Vaughan, G., Wandinger, U., Wang, X., Wiegner, M., Zavrtanik, M., and Zerefos, C.: EARLINET: A European Aerosol Research Lidar Network to Establish an Aerosol Climatology, Max-Planck-Institut Report, No. 348, ISSN 0937 1060, 2003.
Chaichitehrani, N. and Allahdadi, M. N.: Overview of wind climatology for the Gulf of Oman and the northern Arabian Sea, American Journal of Fluid Dynamics, 8, 1–9, 2018.
Chaikovsky, A., Dubovik, O., Holben, B., Bril, A., Goloub, P., Tanré, D., Pappalardo, G., Wandinger, U., Chaikovskaya, L., Denisov, S., Grudo, J., Lopatin, A., Karol, Y., Lapyonok, T., Amiridis, V., Ansmann, A., Apituley, A., Allados-Arboledas, L., Binietoglou, I., Boselli, A., D'Amico, G., Freudenthaler, V., Giles, D., Granados-Muñoz, M. J., Kokkalis, P., Nicolae, D., Oshchepkov, S., Papayannis, A., Perrone, M. R., Pietruczuk, A., Rocadenbosch, F., Sicard, M., Slutsker, I., Talianu, C., De Tomasi, F., Tsekeri, A., Wagner, J., and Wang, X.: Lidar-Radiometer Inversion Code (LIRIC) for the retrieval of vertical aerosol properties from combined lidar/radiometer data: development and distribution in EARLINET, Atmos. Meas. Tech., 9, 1181–1205, https://doi.org/10.5194/amt-9-1181-2016, 2016.
Chang, H. and Charalampopoulos, T. T.: Determination of the wavelength dependence of refractive indices of flame soot, Proc. R. Soc. Lond. A, 430, 577–591, https://doi.org/10.1098/rspa.1990.0107, 1990.
Dey, S., Tripathi, S. N., Singh, R. P., and Holben, B. N.: Retrieval of black carbon and specific absorption over Kanpur city, northern India during 2001–2003 using AERONET data, Atmos. Environ., 40, 445–456, https://doi.org/10.1016/j.atmosenv.2005.09.053, 2006.
Draine, B. T. and Flatau, P. J.: Discrete-Dipole Approximation For Scattering Calculations, J. Opt. Soc. Am. A, 11, 1491–1499, https://doi.org/10.1364/JOSAA.11.001491, 1994
Dubovik, O. and King, M. D.: A flexible inversion algorithm for retrieval of aerosol optical properties from sun and sky radiance measurements, J. Geophys. Res., 105, 20673–20696, https://doi.org/10.1029/2000JD900282, 2000.
Dubovik, O., Holben, B., Eck, T. F., Smirnov, A., Kaufman, Y. J., King, M. D., Tanré, D., and Slutsker, I.: Variability of absorption and optical properties of key aerosol types observed in worldwide locations, J. Atmos. Sci., 59, 590–608, https://doi.org/10.1175/1520-0469(2002)059<0590:voaaop>2.0.co;2, 2002.
Dubovik, O., Sinyuk, A., Lapyonok, T., Holben, B. N., Mishchenko, M., Yang, P., Eck, T. F., Volten, H., Muñoz, O., Veilhelmann, B., van der Zande, W. J., Leon, J. F., Sorokin, M., and Slutsker, I.: Application of spheroid models to account for aerosol particle nonsphericity in remote sensing of desert dust, J. Geophys. Res., 111, D11208, https://doi.org/10.1029/2005JD006619, 2006.
Eibedingil, I. G., Gill, T. E., Van Pelt, R. S., and Tong, D. Q.: Comparison of aerosol optical depth from MODIS product collection 6.1 and AERONET in the western united states, Remote. Sens., 13, 2316, https://doi.org/10.3390/rs13122316, 2021.
Erickson III, D. J. and Duce, R. A.: On the global flux of atmosphere sea salt, J. Geophys. Res., 93, 14079–14088, https://doi.org/10.1029/JC093iC11p14079, 1988.
Fujikawa, M., Kudo, R., Nishizawa, T., Oikawa, E., Higrashi, A., and Okamoto, H.: Long-term analyses of aerosol optical thickness using CALIOP, EPJ Web Conf., 237, 02023, https://doi.org/10.1051/epjconf/202023702023, 2020.
Gelaro, R., McCarty, W., Suárez, M. J., Todling, R., Molod, A., Takacs, L., Randles, C. A., Darmenov, A., Bosilovich, M. G., Reichle, R., Wargan, K., Coy, L., Cullather, R., Draper, C., Akella, S., Buchard, V., Conaty, A., da Silva, A. M., Gu, W., Kim, G.-K., Koster, R., Lucchesi, R., Merkova, D., Nielsen, J. E., Partyka, G., Pawson, S., Putman, W., Rienecker, M., Schubert, S. D., Sienkiewicz, M., and Zhao, B.: The Modern-Era Retrospective analysis for Research and Applications, version 2 (MERRA-2), J. Climate, 30, 5419–5454, 2017.
Getzewich, B. J., Vaughan, M. A., Hunt, W. H., Avery, M. A., Powell, K. A., Tackett, J. L., Winker, D. M., Kar, J., Lee, K.-P., and Toth, T. D.: CALIPSO lidar calibration at 532 nm: version 4 daytime algorithm, Atmos. Meas. Tech., 11, 6309–6326, https://doi.org/10.5194/amt-11-6309-2018, 2018.
Giles, D. M., Sinyuk, A., Sorokin, M. G., Schafer, J. S., Smirnov, A., Slutsker, I., Eck, T. F., Holben, B. N., Lewis, J. R., Campbell, J. R., Welton, E. J., Korkin, S. V., and Lyapustin, A. I.: Advancements in the Aerosol Robotic Network (AERONET) Version 3 database – automated near-real-time quality control algorithm with improved cloud screening for Sun photometer aerosol optical depth (AOD) measurements, Atmos. Meas. Tech., 12, 169–209, https://doi.org/10.5194/amt-12-169-2019, 2019.
Gkikas, A., Proestakis, E., Amiridis, V., Kazadzis, S., Di Tomaso, E., Tsekeri, A., Marinou, E., Hatzianastassiou, N., and Pérez García-Pando, C.: ModIs Dust AeroSol (MIDAS): a global fine-resolution dust optical depth data set, Atmos. Meas. Tech., 14, 309–334, https://doi.org/10.5194/amt-14-309-2021, 2021.
Hess, M., Koepke, P., and Schult, I.: Optical properties of aerosols and clouds: the software package OPAC, B. Am. Meteorol. Soc., 79, 831–844, https://doi.org/10.1175/1520-0477(1998)079<0831:OPOAAC>2.0.CO;2, 1998.
Higurashi, A. and Nakajima, T.: Detection of aerosol types over the East China sea near Japan from four-channel satellite data, Geophys. Res. Lett., 29, 1836, https://doi.org/10.1029/2002GL015357, 2002.
Holben, B. N., Eck, T. F., Slutsker, I., Tanré, D., Buis, J. P., Setzer, A., Vermote, E., Reagan, J. A., Kaufman, Y. J., Nakajima, T., Lavenu, F., Jankowiak, I., and Smirnov, A.: AERONET – A federated instrument network and data archive for aerosol characterization, Remote Sens. Environ., 66, 1–16, https://doi.org/10.1016/S0034-4257(98)00031-5, 1998.
Hsu, N. C., Jeong, M.-J., Betternhausen, C., Sayer, A. M., Hansell, R., Seftor, C. S., Huang, J., and Tsay, S.-C.: Enhanced deep blue aerosol retrieval algorithm: the second generation, J. Geophys. Res., 118, 9296–9315, https://doi.org/10.1002/jgrd.50712, 2013.
Huang, G., Chen, Y., Li, Z., Liu, Q., Wang, Y., He, Q., Liu, T., Liu, X., Zhnag, Y., Gao, J., and Yao, Y.: Validation and accuracy analysis of the collection 6.1 MODIS aerosol optical depth over the westernmost city in China based on the Sun-sky radiometer observations from SONET, Earth and Space Science, 7, e2019EA001041, https://doi.org/10.1029/2019EA001041, 2020.
Huang, J., Wang, T., Wang, W., Li, Z., and Yan, H.: Climate effects of dust aerosols over East Asian arid and semiarid regions, J. Geophys. Res. Atmos., 119, 11398-11416, doi:10.1002/2014JD021796, 2014.
Inness, A., Ades, M., Agustí-Panareda, A., Barré, J., Benedictow, A., Blechschmidt, A.-M., Dominguez, J. J., Engelen, R., Eskes, H., Flemming, J., Huijnen, V., Jones, L., Kipling, Z., Massart, S., Parrington, M., Peuch, V.-H., Razinger, M., Remy, S., Schulz, M., and Suttie, M.: The CAMS reanalysis of atmospheric composition, Atmos. Chem. Phys., 19, 3515–3556, https://doi.org/10.5194/acp-19-3515-2019, 2019.
Illingworth, A. J., Barker, H. W., Beljaars, A., Ceccaldi, M., Chepfer, H., Clerbaux, N., Cole, J. Delanoë, J., Domenech, C., Donovan, D. P., Fukuda, S., Hirakata, M., J. Hogan, R., Huenerbein, A., Kollias, P., Kubota, T., Nakajima, T., Nakajima, T. Y., Nishizawa, T., Ohno, Y., Okamoto, H., Oki, R., Sato, K., Satoh, M., Shephard, M. W., Velázquez-Blázquez, A., Wandinger, U., Wehr, T., and Van Zadelhoff, G. J.: The EarthCARE satellite: The next step forward in global measurements of clouds, aerosols, precipitation, and radiation, B. Am. Meteorol. Soc., 96, 1311–1332, https://doi.org/10.1175/BAMS-D-12-00227.1, 2015.
Ishimoto, H., Zaizen, Y., Uchiyama, A., Masuda, K., and Mano, Y.: Shape modeling of mineral dust particles for light-scattering calculations using the spatial Poisson–Voronoi tessellation, J. Quant. Spectrosoc. Ra., 111, 2434–2443, https://doi.org/10.1016/j.jqsrt.2010.06.018, 2010.
Ishimoto, H., Masuda, K., Mano, Y., Orikasa, N., and Uchiyama, A.: Irregularly shaped ice aggregates in optical modeling of convectively generated ice clouds, J. Quant. Spectrosc. Ra., 113, 632–643, https://doi.org/10.1016/j.jqsrt.2012.01.017, 2012.
Ishimoto, H., Kudo, R., and Adachi, K.: A shape model of internally mixed soot particles derived from artificial surface tension, Atmos. Meas. Tech., 12, 107–118, https://doi.org/10.5194/amt-12-107-2019, 2019.
Jin, Y., Nishizawa, T., Sugimoto, N., Takakura, S., Aoki, M., Ishii, S., Yamazaki, A., Kudo, R., Yumimoto, K., Sato, K., and Okamoto, H.: Demonstration of aerosol profile measurement with a dual-wavelength high-spectral-resolution lidar using a scanning interferometer, Appl. Optics, 61, 3523–3532, https://doi.org/10.1364/AO.451707, 2022.
Kahnert, M., Nousiainen, T., Lindqvist, H., and Ebert, M.: Optical properties of light absorbing carbon aggregates mixed with sulfate: assessment of different model geometries for climate forcing calculations, Opt. Express, 20, 10042–10058, https://doi.org/10.1364/OE.20.010042, 2012.
Kahnert, M., Nousiainen, T., and Lindqvist, H.: Models for integrated and differential scattering optical properties of encapsulated light absorbing carbon aggregates, Opt. Express, 21, 7974–7933, https://doi.org/10.1364/OE.21.007974, 2013.
Kar, J., Vaughan, M. A., Lee, K.-P., Tackett, J. L., Avery, M. A., Garnier, A., Getzewich, B. J., Hunt, W. H., Josset, D., Liu, Z., Lucker, P. L., Magill, B., Omar, A. H., Pelon, J., Rogers, R. R., Toth, T. D., Trepte, C. R., Vernier, J.-P., Winker, D. M., and Young, S. A.: CALIPSO lidar calibration at 532 nm: version 4 nighttime algorithm, Atmos. Meas. Tech., 11, 1459–1479, https://doi.org/10.5194/amt-11-1459-2018, 2018.
Kaufman, Y. J., Tanré, D., Léon, J.-F., and Pelon, J.: Retrievals of profiles of fine and coarse aerosols using lidar and radiometric space measurements, IEEE T. Geoscience Remote, 41, 1743–1754, https://doi.org/10.1109/TGRS.2003.814138, 2003.
Kim, J., Lee, J., Lee, H. C., Higurashi, A., Takemura, T., and Song, C. H.: Consistency of the aerosol type classification from satellite remote sensing during the atmospheric brown cloud-East Asia regional experiment campaign, J. Geophys. Res., 112, D22S33, https://doi.org/10.1029/2006JD008201, 2007.
Kim, M.-H., Omar, A. H., Tackett, J. L., Vaughan, M. A., Winker, D. M., Trepte, C. R., Hu, Y., Liu, Z., Poole, L. R., Pitts, M. C., Kar, J., and Magill, B. E.: The CALIPSO version 4 automated aerosol classification and lidar ratio selection algorithm, Atmos. Meas. Tech., 11, 6107–6135, https://doi.org/10.5194/amt-11-6107-2018, 2018.
Kinne, S.: Aerosol radiative effects with MACv2, Atmos. Chem. Phys., 19, 10919–10959, https://doi.org/10.5194/acp-19-10919-2019, 2019.
Kinne, S., Schulz, M., Textor, C., Guibert, S., Balkanski, Y., Bauer, S. E., Berntsen, T., Berglen, T. F., Boucher, O., Chin, M., Collins, W., Dentener, F., Diehl, T., Easter, R., Feichter, J., Fillmore, D., Ghan, S., Ginoux, P., Gong, S., Grini, A., Hendricks, J., Herzog, M., Horowitz, L., Isaksen, I., Iversen, T., Kirkevåg, A., Kloster, S., Koch, D., Kristjansson, J. E., Krol, M., Lauer, A., Lamarque, J. F., Lesins, G., Liu, X., Lohmann, U., Montanaro, V., Myhre, G., Penner, J., Pitari, G., Reddy, S., Seland, O., Stier, P., Takemura, T., and Tie, X.: An AeroCom initial assessment – optical properties in aerosol component modules of global models, Atmos. Chem. Phys., 6, 1815–1834, https://doi.org/10.5194/acp-6-1815-2006, 2006.
Koren, I., Kaufman, Y. J., Washington, R., Todd, M. C., Rudich, Y., Martins, J. V., and Rosenfeld, D.: The Bodélé depression: a single spot in the Sahara that provides most of the mineral dust to the Amazon forest, Environ. Res. Lett., 1, 014005, https://doi.org/10.1088/1748-9326/1/1/014005, 2006.
Korras-Carraca, M. B., Pappas, V., Hatzianastassiou, N., Vardavas, I., and Matsoukas, C.: Global vertically resolved aerosol direct radiation effect from three years of CALIOP data using the FORTH radiation transfer model, Atmos. Res., 224, 138–156, https://doi.org/10.1016/j.atmosres.2019.03.024, 2019.
Korras-Carraca, M. B., Gkikas, A., Matsoukas, C., and Hatzianastassiou, N.: Global clear-sky aerosol speciated direct radiative effects over 40 years (1980–2019), Atmosphere, 12, 1254, https://doi.org/10.3390/atmos12101254, 2021.
Kudo, R., Uchiyama, A., Yamazaki, A., Sakami, T., and Kobayashi, E.: From solar radiation measurements to optical properties: 1998–2008 trends in Japan, Geophys. Res. Lett., 37, L04805, https://doi.org/10.1029/2009GL041794, 2010a.
Kudo, R., Uchiyama, A., Yamazaki, A., and Kobayashi, E.: Seasonal characteristics of aerosol radiative effect estimated from ground-based solar radiation measurements in Tsukuba, Japan, J. Geophys. Res., 115, D01204, https://doi.org/10.1029/2009JD012487, 2010b.
Kudo, R., Uchiyama, A., Yamazaki, A., Sakami, T., and Ijima, O.: Decadal changes in aerosol optical thickness and single scattering albedo estimated from ground-based broadband radiometers: A case study in Japan, J. Geophys. Res., 116, D03207, https://doi.org/10.1029/2010JD014911, 2011.
Kudo, R., Nishizawa, T., and Aoyagi, T.: Vertical profiles of aerosol optical properties and the solar heating rate estimated by combining sky radiometer and lidar measurements, Atmos. Meas. Tech., 9, 3223–3243, https://doi.org/10.5194/amt-9-3223-2016, 2016.
Kudo, R., Aoyagi, T., and Nishizawa, T.: Characteristics of aerosol vertical profiles in Tsukuba, Japan, and their impacts on the evolution of the atmospheric boundary layer, Atmos. Meas. Tech., 11, 3031–3046, https://doi.org/10.5194/amt-11-3031-2018, 2018.
Kudo, R., Diémoz, H., Estellés, V., Campanelli, M., Momoi, M., Marenco, F., Ryder, C. L., Ijima, O., Uchiyama, A., Nakashima, K., Yamazaki, A., Nagasawa, R., Ohkawara, N., and Ishida, H.: Optimal use of the Prede POM sky radiometer for aerosol, water vapor, and ozone retrievals, Atmos. Meas. Tech., 14, 3395–3426, https://doi.org/10.5194/amt-14-3395-2021, 2021.
Lewis, E. R. and Schwartz, S. E.: Fundamentals in “Sea salt aerosol production: mechanisms, methods, measurements and models”, Geophysical Monograph Series, American Geophysical Union, 152, 9–99, ISBN: 9781118666050, 2004.
Li, M., Liu, J., Wang, Z., Wang, H., Zhang, Z., Zhang, L., and Yang, Q.: Assessment of sea surface wind from NWP reanalysis and satellites in the southern ocean, 1842–1853, https://doi.org/10.1175/JTECH-D-12-00240.1, 2013.
Liu, Z., Vaughan, M. A., Winker, D. M., Kittaka, C., Getzewich, B. J., Kuehn, R. E., Omar, A., Powell, K., Trepte, C. R., and Hostetler, C. A.: The CALIPSO lidar cloud and aerosol discrimination: Version 2 algorithm and initial assessment of performance, J. Atmos. Ocean. Tech., 26, 1198–1213, 2009.
Liu, Z., Kar, J., Zeng, S., Tackett, J., Vaughan, M., Avery, M., Pelon, J., Getzewich, B., Lee, K.-P., Magill, B., Omar, A., Lucker, P., Trepte, C., and Winker, D.: Discriminating between clouds and aerosols in the CALIOP version 4.1 data products, Atmos. Meas. Tech., 12, 703–734, https://doi.org/10.5194/amt-12-703-2019, 2019.
Lopatin, A., Dubovik, O., Chaikovsky, A., Goloub, P., Lapyonok, T., Tanré, D., and Litvinov, P.: Enhancement of aerosol characterization using synergy of lidar and sun-photometer coincident observations: the GARRLiC algorithm, Atmos. Meas. Tech., 6, 2065–2088, https://doi.org/10.5194/amt-6-2065-2013, 2013.
Matsui, H., Koike, M., Kondo, Y., Moteki, N., Fast, J. D., and Zaveri, R. A.: Development and validation of a black carbon missing state resolved three-dimensional model: Aging process and radiative impact, J. Geophys. Res., 118, 2304–2326, https://doi.org/10.1029/2012JD018446, 2013.
Matsui, H., Hamilton, D. S., and Mahowald, N. M.: Black carbon radiative effects highly sensitive to emitted particle size when resolving mixing-state diversity, Nat. Commun., 9, 3446, https://doi.org/10.1038/s41467-018-05635-1, 2018.
Maxwell Garnet, J. C.: Colours in metal glasses and in metallic films, Philos. Trans. R. Soc. A, 203, 283–420, 1904.
Moteki, N., Kondo, Y., Miyazaki, Y., Takegawa, N., Komazaki, Y., Kurata, G., Shirai, T., Blake, D. R., Miyakawa, T., and Koike, M.: Evolution of mixing state of black carbon particles: Aircraft measurements over the western Pacific in March 2004, Geophys. Res. Lett., 34, L11803, https://doi.org/10.1029/2006GL028943, 2007.
Nakajima, T. and Tanaka, M.: Effect of wind-generated waves on the transfer of solar radiation in the atmosphere-ocean system, J. Quant. Spectrosoc. Ra., 29, 521–537, https://doi.org/10.1016/0022-4073(83)90129-2, 1983.
Nakajima, T., Campanelli, M., Che, H., Estellés, V., Irie, H., Kim, S.-W., Kim, J., Liu, D., Nishizawa, T., Pandithurai, G., Soni, V. K., Thana, B., Tugjsurn, N.-U., Aoki, K., Go, S., Hashimoto, M., Higurashi, A., Kazadzis, S., Khatri, P., Kouremeti, N., Kudo, R., Marenco, F., Momoi, M., Ningombam, S. S., Ryder, C. L., Uchiyama, A., and Yamazaki, A.: An overview of and issues with sky radiometer technology and SKYNET, Atmos. Meas. Tech., 13, 4195–4218, https://doi.org/10.5194/amt-13-4195-2020, 2020.
Nishizawa, T., Asano, S., Uchiyama, A., and Yamazaki, A.: Seasonal variation of aerosol direct radiative forcing and optical properties estimated from ground-based solar radiation measurements, J. Atmos. Sci., 61, 57–72, https://doi.org/10.1175/1520-0469(2004)061<0057:SVOADR>2.0.CO;2, 2004.
Nishizawa, T., Okamoto, H., Sugimoto, N., Matsui, I., Shimizu, A, and Aoki, K.: An algorithm that retrieves aerosol properties from dual-wavelength polarized lidar measurements, J. Geophys. Res., 112, D06212, https://doi.org/10.1029/2006JD007435, 2007.
Nishizawa, T., Sugimoto, N., Matsui, I., Shimizu, A., Tatarov, B., and Okamoto, H.: Algorithm to retrieve aerosol optical properties from High-Spectral-Resolution-Lidar and polarization Mie-Scattering Lidar measurements, IEEE T. Geosci. Remote, 46, 4094–4103, https://doi.org/10.1109/TGRS.2008.2000797, 2008.
Nishizawa, T., Sugimoto, N., Matsui, I., Shimizu, A., and Okamoto, H.: Algorithms to retrieve optical properties of three component aerosols from two-wavelength backscatter and one-wavelength polarization lidar measurements considering nonsphericity of dust, J. Quant. Spectrosoc. Ra., 112, 254–267, https://doi.org/10.1016/j.jqsrt.2010.06.002, 2011.
Nishizawa, T., Sugimoto, N., Matsui, I., Shimizu, A., Hara, Y., Uno, I., Yasunaga, K., Kudo, R., and Kim, S.-W.: Ground-based network observation using Mie–Raman lidars and multi-wavelength Raman lidars and algorithm to retrieve distributions of aerosol components, J. Quant. Spectrosoc. Ra., 188, 79–93, https://doi.org/10.1016/j.jqsrt.2016.06.031, 2017.
Oikawa, E., Nakajima, T., and Winker, D.: An evaluation of the shortwave direct aerosol radiatve forcing using CALIOP and MODIS observations, J. Geophys. Res., 123, 1211–1233, https://doi.org/10.1002/2017JD027247, 2018.
Omar, A. H., Winker, D. M., Kittaka, C., Vaughan, M. A., Liu, Z., Hu, Y., Trepte, C. R., Rogers, R. R., Ferrare, R. A., Lee, K.-P., Kuehn, R. E., and Hostetler, C. A.: The CALIPSO automated aerosol classification and lidar ratio selection algorithm, J. Atmos. Ocean. Tech., 26, 1994–2014, https://doi.org/10.1175/2009JTECHA1231.1, 2009.
Omar, A. H., Winker, D. M., Tackett, J. L., Giles, D. M., Kar, J., Liu, Z., Vaughan, M. A., Powell, K. A., and Trepte, C. R.: CALIOP and AERONET aerosol optical depth comparisons: one size fits none, J. Geophys. Res., 118, 4748–4766, https://doi.org/10.1002/jgrd.50330, 2013.
Oshima, N., Koike, M., Zhang, Y., Kondo, Y., Moteki, N., Takegawa, N., and Miyazaki, Y.: Aging of black carbon in outflow from anthropogenic sources using a mixing state resolved model: Model development and evaluation, J. Geophys. Res., 114, D06210, https://doi.org/10.1029/2008JD010680, 2009.
Ota, Y., Higurashi, A., Nakajima, T., and Yokota, T.: Matrix formulations of radiative transfer including the polarization effect in a coupled atmosphere-ocean system, J. Quant. Spectrosoc. Ra., 111, 878–894, https://doi.org/10.1016/j.jqsrt.2009.11.021, 2010.
Pappalardo, G., Amodeo, A., Apituley, A., Comeron, A., Freudenthaler, V., Linné, H., Ansmann, A., Bösenberg, J., D'Amico, G., Mattis, I., Mona, L., Wandinger, U., Amiridis, V., Alados-Arboledas, L., Nicolae, D., and Wiegner, M.: EARLINET: towards an advanced sustainable European aerosol lidar network, Atmos. Meas. Tech., 7, 2389–2409, https://doi.org/10.5194/amt-7-2389-2014, 2014.
Platnick, S., King, M., and Hubanks, P.: MODIS Atmosphere L3 Monthly Product, NASA MODIS Adaptive Processing System, Goddard Space Flight Center [data set], USA, https://doi.org/10.5067/MODIS/MYD08_M3.061, 2015.
Quaas, J., Jia, H., Smith, C., Albright, A. L., Aas, W., Bellouin, N., Boucher, O., Doutriaux-Boucher, M., Forster, P. M., Grosvenor, D., Jenkins, S., Klimont, Z., Loeb, N. G., Ma, X., Naik, V., Paulot, F., Stier, P., Wild, M., Myhre, G., and Schulz, M.: Robust evidence for reversal of the trend in aerosol effective climate forcing, Atmos. Chem. Phys., 22, 12221–12239, https://doi.org/10.5194/acp-22-12221-2022, 2022.
Redemann, J., Wood, R., Zuidema, P., Doherty, S. J., Luna, B., LeBlanc, S. E., Diamond, M. S., Shinozuka, Y., Chang, I. Y., Ueyama, R., Pfister, L., Ryoo, J.-M., Dobracki, A. N., da Silva, A. M., Longo, K. M., Kacenelenbogen, M. S., Flynn, C. J., Pistone, K., Knox, N. M., Piketh, S. J., Haywood, J. M., Formenti, P., Mallet, M., Stier, P., Ackerman, A. S., Bauer, S. E., Fridlind, A. M., Carmichael, G. R., Saide, P. E., Ferrada, G. A., Howell, S. G., Freitag, S., Cairns, B., Holben, B. N., Knobelspiesse, K. D., Tanelli, S., L'Ecuyer, T. S., Dzambo, A. M., Sy, O. O., McFarquhar, G. M., Poellot, M. R., Gupta, S., O'Brien, J. R., Nenes, A., Kacarab, M., Wong, J. P. S., Small-Griswold, J. D., Thornhill, K. L., Noone, D., Podolske, J. R., Schmidt, K. S., Pilewskie, P., Chen, H., Cochrane, S. P., Sedlacek, A. J., Lang, T. J., Stith, E., Segal-Rozenhaimer, M., Ferrare, R. A., Burton, S. P., Hostetler, C. A., Diner, D. J., Seidel, F. C., Platnick, S. E., Myers, J. S., Meyer, K. G., Spangenberg, D. A., Maring, H., and Gao, L.: An overview of the ORACLES (ObseRvations of Aerosols above CLouds and their intEractionS) project: aerosol–cloud–radiation interactions in the southeast Atlantic basin, Atmos. Chem. Phys., 21, 1507–1563, https://doi.org/10.5194/acp-21-1507-2021, 2021.
Rogers, R. R., Hostetler, C. A., Hair, J. W., Ferrare, R. A., Liu, Z., Obland, M. D., Harper, D. B., Cook, A. L., Powell, K. A., Vaughan, M. A., and Winker, D. M.: Assessment of the CALIPSO Lidar 532 nm attenuated backscatter calibration using the NASA LaRC airborne High Spectral Resolution Lidar, Atmos. Chem. Phys., 11, 1295–1311, https://doi.org/10.5194/acp-11-1295-2011, 2011.
Sayer, A. M., Hsu, N. C., Lee, J., Kim, W. V., and Dutcher, S. T.: Validation, stability, and consistency of MODIS collection 6.1 and VIIRS version 1 deep blue aerosol data over land, J. Geophys. Res., 124, 4658–4688, https://doi.org/10.1029/2018JD029598, 2019.
Schaaf, C. B., Gao, F., Strahler, A. H., Lucht, W., Li, X., Tsang, T., Strugnell, N. C., Zhang, X., Jin, Y., Muller, J.-P., Lewis, P., Barnsley, M., Hobson, P., Disney, M., Roberts, G., Dunderdale, M., Doll, C., d'Entremont, R. P., Hu, B., Liang, S., Privette, J. L., and Roy, D.: First operational BRDF, albedo nadir reflectance products from MODIS, Remote Sens. Environ., 83, 135–148, https://doi.org/10.1016/S0034-4257(02)00091-3, 2002.
Schuster, G. L., Dubovik, O., and Holben, B. N.: Inferring black carbon content and specific absorption from Aerosol Robotic Network (AERONET) aerosol retrievals, J. Geophys. Res., 110, D10S17, https://doi.org/10.1029/2004JD004548, 2005.
Schuster, G. L., Vaughan, M., MacDonnell, D., Su, W., Winker, D., Dubovik, O., Lapyonok, T., and Trepte, C.: Comparison of CALIPSO aerosol optical depth retrievals to AERONET measurements, and a climatology for the lidar ratio of dust, Atmos. Chem. Phys., 12, 7431–7452, https://doi.org/10.5194/acp-12-7431-2012, 2012.
Sekiguchi, M. and Nakajima, T.: A k-distribution-based radiation code and its computational optimization for an atmospheric general circulation model, J. Quant. Spectrosc. Ra., 109, 2779–2793, https://doi.org/10.1016/j.jqsrt.2008.07.013, 2008.
Sharma, V., Ghosh, S., Bilal, M., Dey, S., and Singh, S.: Performance of MODIS C6.1 dark target and deep blue aerosol products in Delhi national capital region, India: Application for aerosol studies, Atmos. Poll. Res., 12, 65-74, https://doi.org/10.1016/j.apr.2021.01.023, 2021.
Shi, H., Xiao, Z., Zhan, X., Ma, H., and Tian, X.: Evaluation of MODIS and two reanalysis aerosol optical depth products over AERONET sites, Atmos. Res., 220, 75–80, https://doi.org/10.1016/j.atmosres.2019.01.009, 2019.
Shimizu, A., Nishizawa, T., Jin, Y., Kim, S.-W., Wang, Z., Batdorj, D., and Sugimoto, N.: Evolution of a lidar network for tropospheric aerosol detection in East Asia, Opt. Eng., 56, 031219, https://doi.org/10.1117/1.OE.56.3.031219, 2016.
Sinyuk, A., Holben, B. N., Eck, T. F., Giles, D. M., Slutsker, I., Korkin, S., Schafer, J. S., Smirnov, A., Sorokin, M., and Lyapustin, A.: The AERONET Version 3 aerosol retrieval algorithm, associated uncertainties and comparisons to Version 2, Atmos. Meas. Tech., 13, 3375–3411, https://doi.org/10.5194/amt-13-3375-2020, 2020.
Smirnov, A., Holben, B. N., Slutsker, I., Giles, D. M., McClain, C. R., Eck, T. F., Sakerin, S. M., Macke, A., Croot, P., Zibordi, G., Quinn, P. K., Sciare, J., Kinne, S., Harvey, M., Smyth, T. J., Piketh, S., Zielinski, T., Proshutinsky, A., Goes, J. I., Nelson, N. B., Larouche, P., Radionov, V. F., Goloub, P., Krishna Moorthy, K., Matarrese, R., Robertson, E. J., and Jourdin, F.: Maritime Aerosol Network as a component of Aerosol Robotic Network, J. Geophys. Res., 114, D06204, https://doi.org/10.1029/2008JD011257, 2009.
Stroeve, J., Box, J. E., Gao, F., Liang, S., Nolin, A., and Schaaf, C.: Accuracy assessment of the MODIS 16-day albedo product for snow: comparisons with Greenland in situ measurements, Remote Sens. Environ., 94, 46–60, https://doi.org/10.1016/j.rse.2004.09.001, 2005.
Stroeve, J., Box, J. E., Wang, Z., Schaaf, C., and Barrett, A.: Re-evaluation of MODIS MCD43 Greenland albedo accuracy and trends, Remote Sens. Environ., 138, 199–214, https://doi.org/10.1016/j.rse.2013.07.023, 2013.
Sugimoto, N., Nishizawa, T., Shimizu, A., Matsui, I., Higurashi, A., Uno, I., Hara, Y., Yumimoto, K., and Kudo, R.: Continuous observations of atmospheric aerosols across East Asia, SPIE Newsroom, https://doi.org/10.1117/2.1201510.006178, 21 October 2015.
Tackett, J. L., Winker, D. M., Getzewich, B. J., Vaughan, M. A., Young, S. A., and Kar, J.: CALIPSO lidar level 3 aerosol profile product: version 3 algorithm design, Atmos. Meas. Tech., 11, 4129–4152, https://doi.org/10.5194/amt-11-4129-2018, 2018.
Vaughan, M., Garnier, A., Josset, D., Avery, M., Lee, K.-P., Liu, Z., Hunt, W., Pelon, J., Hu, Y., Burton, S., Hair, J., Tackett, J. L., Getzewich, B., Kar, J., and Rodier, S.: CALIPSO lidar calibration at 1064 nm: version 4 algorithm, Atmos. Meas. Tech., 12, 51–82, https://doi.org/10.5194/amt-12-51-2019, 2019.
Wang, Z., Schaaf, C. B., Strahler, A. H., Chopping, M. J., Román, M. O., Shuai, Y., Woodcock, C. E., Hollinger, D. Y., and Fitzjarrald, D. R.: Evaluation of MODIS albedo product (MCD43A) over grassland, agriculture and forest surface types during dormant and snow-covered periods, Remote Sens. Environ., 140, 60–77, https://doi.org/10.1016/j.rse.2013.08.025, 2014.
Wang, Z., Schaaf, C. B., Sun, Q., Shuai, Y., and Román, M. O.: Capturing rapid land surface dynamics with Collection V006 MODIS BRDF/NBAR/Albedo (MCD43) products, Remote Sens. Environ., 50–64, https://doi.org/10.1016/j.rse.2018.02.001, 2018.
Wei, J., Li, Z., Sun, L., Peng, Y., Liu, L., He, L., Qin, W., and Gribb, M.: MODIS collection 6.1 3 km resolution aerosol optical depth product: global evaluation and uncertainty analysis, Atmos. Environ., 240, 117768, https://doi.org/10.1016/j.atmosenv.2020.117768, 2020.
Weingartner, E., Burtscher, H., and Baltensperger, U.: Hygroscopic properties of carbon and diesel soot particles, Atmos. Environ., 31, 2311–2327, https://doi.org/10.1016/S1352-2310(97)00023-X, 1997.
Williamson, S. N., Copland, L., and Hik, D. S.: The accuracy of satellite-derived albedo for northern alpine and glaciated land covers, Polar Science, 10, 262–269, https://doi.org/10.1016/j.polar.2016.06.006, 2016.
Winker, D. M., Pelon, J., Coakley Jr., J. A., Ackerman, S. A., Charlson, R. J., Colarco, P. R., Flamant, P., Fu, Q., Hoff, R. M., Kittaka, C., Kubar, T. L., Le Treut, H., McCormick, M. P., Megie, G., Poole, L., Powell, K., Trepte, C., Vaughan, M. A., and Wielicki, B. A.: The CALIPSO mission: A Global 3D view of aerosols and clouds, B. Am. Meteorol. Soc., 91, 1211–1229, https://doi.org/10.1175/2010BAMS3009.1, 2010.
Xu, F., Gao, L., Redemann, J., Flynn, C. J., Espinosa, W. R., da Silva, A. M., Stamnes, S., Burton, S. P., Liu, X., Ferrare, R., Cairns, B., and Dubovik, O.: A combined lidar-polarimeter inversion approach for aerosol remote sensing over ocean, Front. Remote. Sens., 21, 620871, https://doi.org/10.3389/frsen.2021.620871, 2021.
Yu, H., Liu, S. C., and Dickinson, R. E.: Radiative effects of aerosols on the evolution of the atmospheric boundary layer, J. Geophys. Res., 107, 4142, https://doi.org/10.1029/2001JD000754, 2002.
Yu, H., Kaufman, Y. J., Chin, M., Feingold, G., Remer, L. A., Anderson, T. L., Balkanski, Y., Bellouin, N., Boucher, O., Christopher, S., DeCola, P., Kahn, R., Koch, D., Loeb, N., Reddy, M. S., Schulz, M., Takemura, T., and Zhou, M.: A review of measurement-based assessments of the aerosol direct radiative effect and forcing, Atmos. Chem. Phys., 6, 613–666, https://doi.org/10.5194/acp-6-613-2006, 2006.
Yumimoto, K., Tanaka, T. Y., Oshima, N., and Maki, T.: JRAero: the Japanese Reanalysis for Aerosol v1.0, Geosci. Model Dev., 10, 3225–3253, https://doi.org/10.5194/gmd-10-3225-2017, 2017.
- Abstract
- Introduction
- Data
- Methods
- Evaluation of retrieval uncertainties using simulation data
- Retrieval results from the CALIOP and MODIS observations in 2010
- Summary and conclusions
- Appendix A: Optimization of the core–grey shell model
- Data availability
- Author contributions
- Competing interests
- Disclaimer
- Acknowledgements
- Financial support
- Review statement
- References
- Supplement
- Abstract
- Introduction
- Data
- Methods
- Evaluation of retrieval uncertainties using simulation data
- Retrieval results from the CALIOP and MODIS observations in 2010
- Summary and conclusions
- Appendix A: Optimization of the core–grey shell model
- Data availability
- Author contributions
- Competing interests
- Disclaimer
- Acknowledgements
- Financial support
- Review statement
- References
- Supplement