the Creative Commons Attribution 4.0 License.
the Creative Commons Attribution 4.0 License.
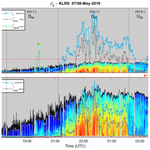
Detection and analysis of Lhù'ààn Mân' (Kluane Lake) dust plumes using passive and active ground-based remote sensing supported by physical surface measurements
Seyed Ali Sayedain
Norman T. O'Neill
James King
Patrick L. Hayes
Daniel Bellamy
Richard Washington
Sebastian Engelstaedter
Andy Vicente-Luis
Jill Bachelder
Malo Bernhard
There is growing recognition that high-latitude dust (HLD), originating from local drainage-basin flows, is the dominant source for certain important phenomena such as particle deposition on snow/ice. The analysis of such local plumes (including a better exploitation of remote sensing data) has been targeted as a key aerosol issue by the HLD community. The sub-Arctic Lhù'ààn Mân' (Kluane Lake) region in the Canadian Yukon is subject to regular drainage-basin, wind-induced dust plumes. This dust emission site is one of many current and potential proglacial dust sources in the Canadian north. In situ ground-based measurements are, due to constraints in accessing these types of regions, rare. Ground- and satellite-based remote sensing accordingly play an important role in helping to characterize local dust sources in the Arctic and sub-Arctic.
We compared ground-based passive and active remote sensing springtime (May 2019) retrievals with microphysical surface-based measurements in the Lhù'ààn Mân' region in order to better understand the potential for ground- and satellite-based remote sensing of HLD plumes. This included correlation analyses between ground-based coarse mode (CM) aerosol optical depth (AOD) retrievals from AERONET AOD spectra, CM AODs derived from co-located Doppler lidar profiles, and OPS (optical particle sizer) surface measurements of CM particle-volume concentration (vc(0)). An automated dust classification scheme was developed to objectively identify local dust events. The classification process helped distinguish lidar-derived CM AODs which covaried with vdust(0) (during recognized dust events) and those that varied at the same columnar scale as AERONET-derived CM AOD (and thus could be remotely sensed). False positive cloud events for dust-induced, high-frequency variations in lidar-derived CM AODs in cloudless atmospheres indicated that the AERONET cloud-screening process was rejecting CM dust AODs. The persistence of a positive lidar ratio bias in comparing the CIMEL/lidar-derived value with a prescribed value obtained from OPS-derived particle sizes coupled with dust-speciation-derived refractive indices led to the suggestion that the prescribed value could be increased to optically derived values of 20 sr by the presence of optically significant dust particles at an effective radius of 11–12 µm. Bimodal CM PSDs (see Appendix B for a glossary) from full-fledged AERONET inversions (the combination of AOD spectra and almucantar radiances) also showed CM peaks at ∼ 1.3 and 5–6.6 µm radius: this, we argue, was associated with springtime Asian dust and Lhù'ààn Mân' dust, respectively. Correlations between the CIMEL-derived fine mode (FM) AOD and FM OPS-derived particle-volume concentrations suggest that remote sensing techniques can be employed to monitor FM dust (which is arguably a better indicator of the long-distance transport of HLD).
- Article
(4192 KB) - Full-text XML
-
Supplement
(8373 KB) - BibTeX
- EndNote
Local, drainage-basin wind-induced dust events have recently been recognized as an important source of dust at high latitudes (Bullard et al., 2016). Groot Zwaaftink et al. (2016) employed FLEXPART simulations to argue that the contributions of Asian, African, and local dust in the Arctic are roughly evenly divided in terms of total atmospheric (columnar) dust loads, while surface concentrations are significantly more associated with dust of local origin. Meinander et al. (2022) employed two global dust-transport models supported by recent verification data to confirm the predominance of high-latitude dust (HLD) sources in terms of snow and ice deposition. This means, notably, that dust deposition on snow and ice and the attendant effects or early snowmelt are largely attributable to local dust. Additional impacts of local dust deposition include direct addition of nutrients, local health impacts for humans and wildlife, and indirect climate/radiative (altered cloud property) impacts (Meinander et al., 2022). These substantive impacts underscore the importance of understanding the dynamics of local dust transport and deposition.
Bullard et al. (2016) pointed out that local dust events in Canada are rarely monitored compared with other high-latitude countries. Low population density, limited numbers of meteorological stations, and problems with the use of satellite-based remote sensing (RS) data in high-latitude regions (e.g., cloud contamination) have led to less frequent observations of local dust events in Canada (Bullard et al., 2016). The next generation of active and passive polar-orbiting sensors targeted by the ESA EarthCARE mission and the NASA-led Atmosphere Observation System (AOS) promise a high quality of aerosol, cloud, and precipitation retrievals that will make significant instrumental, algorithmic, and scientific advances relative to the polar-orbiting A-Train constellation (the workhorse remote sensing constellation that has driven atmospheric science over the past 22 years). An intrinsic part of that system will be the ground-based Cal/Val sites that employ or will employ active and passive ground-based remote sensing sensors as well as microphysical measurements to monitor aerosols and clouds at existing and future sites across the Arctic. The Lhù'ààn Mân'1 (Kluane Lake) region of Yukon, Canada, will very likely be such a Cal/Val site in the HLD context: it is recognized for its frequent and strong springtime dust events induced by strong katabatic winds, highly erodible sediments, and the steep valley walls leading down to the lake (Bachelder et al., 2020).
Published results on the remote sensing of local dust in the Arctic using active or passive airborne or satellite-based platforms are rare. Specific examples include the use of airborne (532 nm) lidar profiles to detect local dust plumes over riverbed, fjord, and coastal regions of Svalbard (Dörnbrack et al., 2010); MODIS-based colour and AOD (aerosol optical depth) imagery for dust plumes emanating from Alaskan riverbed sediments in the Gulf of Alaska (Crusius et al., 2011); the investigations by Dagsson-Waldhauserova et al. (2019), who employed MODIS colour imagery and attempted to use satellite-based CALIOP lidar profiles to support their airborne particle size distribution measurements of Icelandic dust plumes; and MODIS, MISR, CloudSat/CALIOP optical depth and particle size characterization of local dust plumes over Lake Hazen in the high Canadian Arctic (Ranjbar et al., 2021). More recently, Kawai et al. (2023) reported on a multi-year CALIOP-derived climatology of zonally averaged estimates of local dust optical depth in the Arctic (as well as a unique, multi-year, simulated map of local-dust columnar concentration across the Arctic).
Ground-based data retrievals using solar extinction and sky radiometry data are widely used by AERONET (AErosol RObotic NETwork) to characterize the optical and microphysical properties of aerosols at local (site-focused) scales (Holben et al., 1998). AOD and almucantar sky radiance measurements are carried out using the CIMEL sun photometer/sky radiometer. Spectral deconvolution algorithm (SDA) retrievals can be employed to investigate high-frequency event-level studies (O'Neill et al., 2003), while the more comprehensive AERONET inversion algorithm (Dubovik and King, 2000) can be employed for low-frequency, climatological-scale analysis (see Hesaraki et al., 2017, for a more detailed discussion). Publications on the use of AERONET retrievals and/or ground-based lidar data to characterize the properties of local dust events at high latitudes are also rare. Yang et al. (2020) employed AERONET AODs and AERONET-derived particle size distributions, surface particle concentration measurements (PM2.5 and PM10), and Doppler lidar profiles (including the use of a particle-typing depolarization-ratio channel) and ceilometer profiles to characterize the dynamics of local dust events over Iceland. Rozwadowska et al. (2010), while acknowledging the episodic optical importance of local dust storms such as those detected by Dörnbrack et al. (2010), presented a long-range transport and local meteorology analysis of AERONET AODs at Hornsund: an analysis that implies that local dust is of secondary optical importance (at least in the context of total AOD: they did not pursue the analysis in terms of the possible impact of the local meteorology on their coarse mode2 (CM) AOD3).
Huck et al. (2023) employed meteorological measurements, along with AERONET AODs and Ångström exponents (AOD spectral information); a remote camera; and satellite imagery to investigate dust event detection limits in the Lhù'ààn Mân' region. One notable conclusion was that the oblique camera captured many dust-blowing incidents that were not being detected by their AERONET CIMEL instrument. Various explanations for this discrepancy were offered: these included (1) the notion that Lhù'ààn Mân' plumes are very locally inhomogeneous and that wind direction could influence AERONET dust detection, that (2) the CIMEL instrument missed plumes below its minimum angular elevation, that (3) AERONET sensitivity was frequently too coarse to detect weaker events, that (4) AERONET cloud screening removed 97.8 % of dust events and that (5) the CIMEL employed for the analysis (for which data acquisition occurred during the 2018 dust season) was incapable of making nighttime measurements.
Ground-based (and, even more so, satellite-based) remote sensing methods suffer from and benefit from their relatively coarse, column-based spatial scale and their minute to hourly to daily temporal scale. Columnar methods integrate the aerosol effects of the whole column and rely on other dimensions of information to separate out the optical influence of low-altitude dust plumes. This same coarseness can have the tendency to produce robust dust signals if the dust plume scale is commensurate with the remote sensing scale. Another challenge related specifically to ground-based and specifically AERONET retrievals is the excessively aggressive cloud screening that can screen out a dust event (Evan et al., 2021) and secondly, insufficiently aggressive cloud screening that leaves residual cloud OD to contaminate potential dust signals. Surface microphysical measurements such as PSDs and vertical profiling measurements such as lidar backscatter profiles can help to verify that dust events are predominantly coarse mode in nature.
We seek to employ ground-based passive and active (lidar) RS techniques to analyze the complementarity and redundancy of optical and microphysical retrievals relative to springtime CM measurements of local dust acquired using microphysical instruments at Lhù'ààn Mân'. The key ground-based instruments include a CIMEL sun photometer/sky radiometer, a Doppler lidar operating in the SWIR (short-wave infrared), and an optical particle sizer (OPS) for the measurement of near-surface particle-volume size distributions (PSDs). The coherencies and incoherencies between the passive AERONET retrievals and near-surface PSDs, between AERONET CM AODs and the lidar backscatter profiles and their derived CM AODs (largely generated by CM particle backscatter for the Doppler SWIR lidar) and between the lidar profiles and the CM portion of the near-surface PSDs will enable a better understanding of the optical and microphysical properties of local dust plumes and will help in improving ground-based and satellite-based remote sensing retrievals of local dust properties.
The Lhù'ààn Mân' region in the Canadian Yukon is subject to regular drainage-wind dust plumes emanating from the Slims River basin (Nickling, 1978). The recent Kaskawulsh Glacier retreat in the Slims River valley and the river reorganization event (Shugar et al., 2017) prompted the fall of water levels and the season-long exposure of the river delta and floodplain. This caused extended periods of dust emissions induced by aeolian erosion (Shugar et al., 2017). Typically, the period of the most intense dust events is the spring and summer of each year (Bachelder et al., 2020).
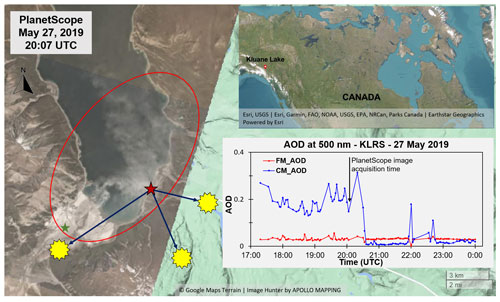
Figure 1Lhù'ààn Mân' (Kluane Lake) study area as seen in a 27 May 2019 PlanetScope satellite image (ESA/NASA). A dust plume can be seen emanating from the whitish Slims River basin. The KLRS and DV site positions are indicated by the red and green stars, respectively. The CM AOD and FM AOD time series in the bottom right-hand corner is a standard (SDA) AERONET Version 3, Level 1.0 (500 nm) product (the CM AOD at the lidar wavelength of 1.548 µm will only change by a moderate amount relative to the 500 nm value). The solar-viewing azimuth direction of the CIMEL is shown by yellow sun icons (clockwise from 17:00 UTC at the upper right to 20:07 and 00:00 UTC on 28 May). The coordinates of the AERONET/AEROCAN station are 61.027∘ N, 138.41∘ W.
The Kluane Lake Research Station (KLRS) is an established University of Calgary (Atmospheric Institute of North America) site on the southern shore of Lhù'ààn Mân' (see Fig. 1). The AERONET CIMEL, Doppler lidar, and OPS data employed in this paper were acquired at KLRS during the month of May 2019. The May 2019 campaign was an intense part of a continuous strategy to extract and analyze Lhù'ààn Mân' dust plume properties using a suite of optical, microphysical, and meteorological instruments (a major chemical and microphysical contribution to this ongoing analysis was reported in Bachelder et al., 2020).
Details on the CIMEL sun photometer/sky radiometer and associated processing protocols can be found in Giles et al. (2019). AEROCAN, the Canadian subnetwork of AERONET (maintained by Environment and Climate Change Canada), provides direct instrument troubleshooting and swapping out support for all Canadian AERONET sites. This instrument provides spectral AOD retrievals at a nominal high frequency of one (nine-band) AOD spectrum every 3 min (nine bands from 340 to 1640 nm).4 It also provides a comprehensive suite of low-frequency (nominally once per hour) microphysical and optical retrievals derived from almucantar radiance scans and four-band AODs.
The high-frequency (11 s time bin) Doppler (HALO Photonics) lidar (Pearson et al., 2009) was averaged to the 1 min CIMEL time bins. The vertical resolution of the lidar is 3 m based on 30 m overlapping gates with a typical range of < LCH (lidar ceiling height, which is equal to 9.6 km; Newsom and Krishnamurthy, 2022). Its 1.5 µm wavelength (more precisely 1.548 µm according to Newsom and Krishnamurthy, 2022) is largely the result of a strategic decision to minimize molecular contributions to the backscatter signal (see Newsom and Krishnamurthy, 2022, for example). The FM5 AOD at the lidar wavelength is typically negligible (see below).
The KLRS surface-PSD device is a TSI optical particle sizer (OPS) 3330 instrument that incorporates 16 size bins with bin-centre radii extending from 0.17 to 4.51 µm (bin-centre diameters from 0.34 to 9.02 µm). Two supporting FAI optical particle counter (OPC) instruments provided analogous PSD measurements6 at the Down Valley (DV) site about 7 km southwest of KLRS (see Fig. 1 for the DV position and Bachelder et al., 2020, for details on the OPC instruments). The KLRS OPS and the two DV OPC instruments often provided important redundancy information (as well as insightful information stemming from their differences) when we sought to investigate the presence and nature of a given CM event.
A dust event captured in a 27 May 2019 PlanetScope satellite image acquired over Lhù'ààn Mân' is shown in Fig. 1 (the KLRS and DV site positions are indicated by red and green stars, respectively). AERONET CM AOD retrievals are represented by the blue profile in the bottom right-hand corner of the image. The time of the PlanetScope image acquisition is indicated by the arrow on the AERONET plot. The sun-pointing CIMEL instrument was looking southeast at the time of the PlanetScope image acquisition while dust plumes can be seen flowing from the whitish Slims River basin and delta. The blue AERONET CM AOD shows quite large values during the period (late morning to early afternoon) when the optically significant dust plume intercepts the CIMEL line of sight (from the roughly eastern to western directions).
3.1 AERONET processing
We employed Version 3.0 Level 1.0 AODs and Level 1.5 AERONET inversions to extract retrieval products that could be compared with the lidar and the KLRS OPS data sets (see Giles et al., 2019, for an overview of Version 3.0 AERONET AOD products and Sinyuk et al., 2020, for an overview of Version 3 AERONET inversions). The algorithm used for comparisons with the Doppler lidar data was the high-frequency (nominally 1 min averaging bin, 3 min intersample gap) SDA+ product extrapolated to the Doppler SWIR wavelength of 1.548 µm (see O'Neill et al., 2008, for a discussion of the SDA+ algorithm). We label these AERONET-derived CM AODs as τc.
The low-frequency (nominally 1 h intersample gap) AERONET inversion products (Dubovik and King, 2000) include the particle-volume PSD per unit increment in logarithm of r () over 22 radius bin centres (stretching from 0.05 to 15 µm) along with pan-PSD refractive index, scattering phase function, and associated optical products such as the asymmetry factor across four spectral bands (440, 675, 870, and 1020 nm). These fundamental products are accompanied by derived products of CM and FM particle-volume concentration and effective radius as well as CM and FM AODs across the same four spectral bands.
An important intensive (per particle) AERONET inversion product is the (column averaged) CM effective radius (see Hansen and Travis, 1974, for a definition of the effective radius and AboEl-Fetouh et al., 2020, for a discussion of the FM and CM AERONET-inversion effective radius product). In the absence of clouds and in the presence of a strong columnar dust activity, this parameter may provide an important and robust indicator of column-averaged effective radius. The same can be said of the refractive index product with two notable riders: the pan-PSD nature of the product can become problematic in the presence of an (optically) competitive FM aerosol of distinctly different refractive index and the rapidly increasing refractive index and single-scattering albedo retrieval errors (notably for desert dust) with decreasing AOD (Sinyuk et al., 2020).
3.2 Lidar processing
Basic lidar definitions are given, for example, in Weitkamp (2005). The essential quantities that we require in order to transform the Doppler lidar profiles into CM extinction coefficient and lidar-derived CM optical depth () are the CM backscatter coefficient (βc) and the CM lidar ratio (Sc). The actual lidar outputs are (attenuated backscatter coefficient) profiles where T2(z) is the altitude-dependent (z-dependent) return (two-way) transmission of a lidar pulse from the ground (z=0). Optical depths for submicron particles at the lidar SWIR wavelength are negligible (we already know that to be true for molecular scattering, and it is generally a good approximation if dust optical depths are ≳ 0.1; see, for example, Fig. 2 of O'Neill et al., 2008).7
If we apply this CM dominance to the β′(z) and β(z) profiles, then
The two-way transmission can be approximated by
where τc is a CIMEL-derived value at the lidar wavelength (τc values interpolated to the nominal time of a given lidar profile), and amounts to a dynamic approximation of for that profile (the reader will note that, for the sake of nomenclature simplicity, we only employ the partial column argument “(0,z)” when it helps to underscore an explicit point). We can then approximate βc(z) by
The division by means (whether it is an approximation or not) that the derived βc(z) values will appear progressively brighter than values as z increases with an attendant potential for error as T2(z) and become very small. However, the values are increasingly pinioned (in addition to the near-surface restraint of near-unity transmission) by the CIMEL-estimated true value (exp [−2τc]) as z increases towards the upper extreme of the integration (top of a dust plume in the case of a clear-sky dust event).
3.2.1 Prescribed lidar ratio for dust
A prescribed KLRS-derived lidar ratio8 for dust (; results presented below) and a prescribed lidar ratio for cloud (Chiang et al., 2002) were employed to calculate for dust and cloud. If is defined as , then
can be computed from a priori or measurement information on refractive index, particle size, and particle shape. We discuss below (Sect. 4.3.1) how the prescribed KLRS-derived value for dust was arrived at and how much uncertainty was associated with that value.
3.2.2 Validation of prescribed lidar ratio
CIMEL values of τc were employed to validate the prescribed dust lidar ratio (effectively the replacement of by τc in Eq. 3 above). Details of the validation parameterization are given in Appendix A1. The process explicitly incorporates and as well as CIMEL-derived τc values to compute a CIMEL-referenced lidar ratio (Sc). It includes an accounting of the day-to-day correlations between and τc (which may or may not be strong during any given period of interest) and how those correlation results should be weighted.
3.3 OPS and OPC processing
The KLRS OPS and the DV OPC PSD measurements of (particle number/unit volume of air/unit increment in logarithmic diameter) were converted to (particle-volume/unit volume of air/unit increment in logarithmic diameter) assuming spherical particles.9 We integrated the OPS PSDs over the CM radius range (bin-centres of 0.78 to 4.51 µm) to yield the CM particle-volume concentration (νc(0): the volume of all CM particles per unit volume of air). The near-surface OPS and OPC particle-volume PSD are analogous to the columnar particle-volume PSDs () products of the AERONET inversion (columnar particle-volume per unit area), while the νc(0) parameter is analogous to the AERONET CM-integrated particle-volume density (“VolC-C” or Vc) product. Since we only consider events that are likely to be dust events (as evidenced by the lidar-measured presence of a dust plume), we will generally label νc(0) as νdust(0).
3.4 Correlation analysis
Indicators of the optical significance and remote sensing detectability of a dust event can, we would argue, be represented, respectively, by how dust-related correlates with the KLRS OPS measurements of νdust(0) and how well τc correlates with dust-related . Our dust (and cloud) classification methodology defined below is largely based on the coefficient of correlation between νdust(0), , and τc.
Results such as those of Hesaraki et al. (2017) empirically indicate that geometric statistics of AODs (histograms and statistics computed in log-AOD space) are more representative than the arithmetic statistics of linear-AOD space, and that a consequence of this is that R values should be larger (results that will, however, always be subject to the vagaries of low-sample or “low-N” statistics). In order to better understand the degree of coherency between the three different types of data, we analyzed their correlations in linear-AOD and log-AOD space as a function of different time-bin amplitudes (results are presented below).
3.5 Event classification
The classification methodology must clearly separate the optical effects of dust and clouds: the first step in this process is to isolate apparent dust plumes in the vertical profiles of the lidar. The dynamic process for arriving at a variable dust layer height (DLH) from the profiles is outlined in the Supplement (Sect. S2). An estimate of lidar-derived dust optical depth () can then be computed by vertical integrations of the profiles from the surface to the DLH. We then argue that values (or at least the CM optical depth of anything but local dust) are obtained by vertically integrating the profiles from the DLH to LCH.
We focused on two levels of correlation: the use of values as a means of identifying and characterizing optically significant dust events and values to identify those events that could be remotely sensed by a passive ground- or satellite-based instrument.10 In the latter case, the high-frequency values must be resampled to match the 1 min time bins (3 min intersample times) of the low-frequency τc values: this process is described in Appendix A3. Our justification for the use of such a dust event flagging protocol is that correlation is a necessary (if insufficient) indicator of the presence of a dust event and that it is largely impervious to systematic instrumental issues (calibration issues, for example). Verification of correlation-based dust event flagging is usually supported (contextualized) by other types of indicators (the recording of strong basin winds, for example). We attributed the following event-classification codes for different, event-level periods of interest (POIs).
3.5.1 Optically significant (D class) dust
Our fundamental classification is that of a dust (D) class that appears to be optically significant at the columnar optical depth scale. A POI was defined as belonging to the D class if
This class basically requires that local KLRS OPS variations at the surface be at the same scale of variation as the columnar lidar optical depths (i.e., something that would not likely be true for dust turbulence scales of approximately a few metres).
3.5.2 Optically insignificant (U class) dust
We labelled a POI that failed to satisfy the D class criterion as a U (unknown) event (this includes cases for which there were less than 10 matched OPS-lidar matched samples):
3.5.3 D and U subclasses
The D classification rule leads to two subclass children: class D events that can and cannot be remotely sensed (respectively DRS and DNRS). In the former case, we would argue that the potential for achieving the satellite-based remote sensing of a dust event must require that a passive ground-based measurement of a candidate dust plume (τc) must be significantly correlated with its lidar analogue. The DRS subclass was accordingly defined as
This means that the correlation must be roughly impervious to spatio-temporal sampling differences between the lidar and the photometric measure (including the fact that the photometric measure represents a completely different line of sight relative to zenith-looking lidar). The complementary DNRS subclass follows as
It includes, by default, cases for which there were less than N(τc)=10 retrievals or for which the lidar indicates the presence of cloud ().11 The D subclasses have their U subclass analogues: class U events that can and cannot be remotely sensed (respectively URS and UNRS) are
The URS subclass represents cases where the correlation between τc and indicates the presence of a detectable remote sensing event of an unknown nature (“unknown” at least in the context of a “D” classification that is tied to threshold values of ). The UNRS subclass represents a non-event that defies both a dust and a remote sensing label.
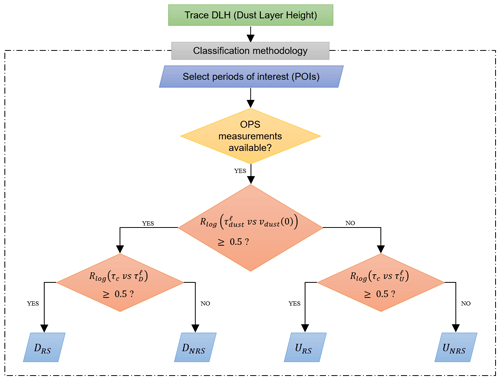
Figure 2Flowchart of the classification methodology described in the text. The different class symbols are defined directly above the flowchart. The N(τc) threshold is 10 samples. The DFPC and DGEN subclasses would be supplementary branches (not shown) from the “No” of the upper-level decision diamond.
Figure 2 summarizes the dust classification paradigm in a more heuristic flowchart manner. For the sake of simplicity, the special DFPC and DGEN subclasses defined immediately below have been omitted from the flowchart.
3.5.4 Special classes
A special false positive cloud subclass (DFPC) was defined for which the AERONET cloud-screening process appeared to eliminate real optical depth variations induced by a dust event: class D events for which N(τc) ≤ 10 and for which indicates no cloud. A special “generic” dust subclass (DGEN) was defined to include events that were identified, with a reasonable certainty using circumstantial evidence. The circumstantial evidence could include combinations of, for example, lidar profiles, reports from measuring teams on the ground, RGB satellite images that visually show dust plumes over Lhù'ààn Mân' (the PlanetScope image of Fig. 1, for example), information from auxiliary measurements such as downslope wind velocity or PM10 devices, etc.
4.1 Lidar measurement overview
Figure 3 shows a βc(z) profile12 over a time period in May of 2019 that includes a number of interesting events. Our intent here is (i) to illustrate the profile attributes (notably the logarithmic colour legend) during a diversity of events as well as the fact that dust plume heights were generally less than 2 km (dashed red line) and (ii) to give the reader an event-continuity perspective that is not always evident in the shorter term (POI-driven) daily profiles found in the Supplement (Sect. S6). One can observe a relatively strong-backscatter plume with a maximum height at ∼ 00:00 UTC on 8 May whose height decreases in altitude and optical impact during the typical nighttime13 shrinking of the boundary layer and then increases to a peak at ∼ 20:00 UTC on 8 May UTC (followed by an apparently strong but very low-altitude plume beginning around 00:00 UTC on 9 May). Cloud formation can be observed between about 5 and 9 km altitude during the nighttime.
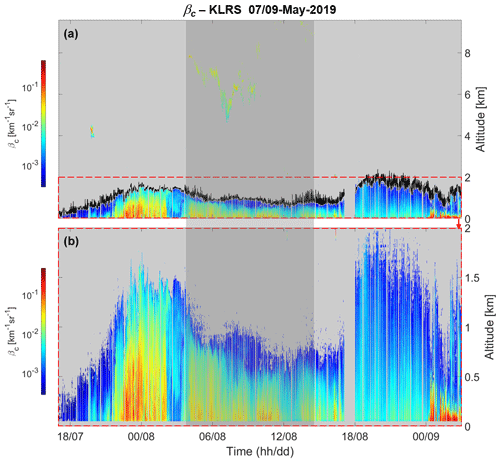
Figure 3βc(z) profile from 0.05 to 9.6 km (a) and a zoom from 0.05 to 2 km (b) during a selected time period in May 2019 (the dashed red rectangles underscore the 0.05 to 2 km range in both panels). The times are UTC (local “Pacific daylight time” is 7 h behind the UTC time). The grey background corresponds to lidar profile pixels that are set to NaN (not a number): these are the result of signal-to-noise ratio (SNR) threshold flags employed to eliminate excessively noisy data (Newsom and Krishnamurthy, 2022). The black colour that is evident at the top of the plume in panel (a) shows the automated estimate of dust layer height (DLH). The region of darker grey shading corresponds to the nighttime (see the text for details).
4.2 Classification of dust events
4.2.1 Results of the correlation analysis
In order to investigate the influences on variability (and thus better understand its impact on the classification methodology), we computed (as discussed in Sect. 3.4) both linear and logarithmic space (arithmetic and geometric) correlations as well as the dependence of those correlations on time-bin resolution. Sample arithmetic and geometric correlation coefficient values as a function of temporal-bin resolution are shown in the Supplement (Sect. S3). Geometric correlation coefficients (Rlog) were typically of the same order as or better than arithmetic coefficients (R) in the presence of more than one decade of variation: we accordingly used this as support for reporting correlation coefficients in geometric statistics space. This choice was supported by analyses showing that ground- and satellite-based AOD histograms were better described by geometric means and standard deviations (O'Neill et al., 2000; Sayer and Knobelspiesse, 2019; and references cited therein).
Aside from the obvious limitations of low-N (low sample number) statistics, no strong dependence on time-bin resolution was found. We accordingly chose to employ the highest bin resolution14 as the basis for our classification analysis (the selection of the high-bin-resolution case being also justified on the general principle of wishing to minimize the elimination of high-frequency lidar data that might have physical significance). We believe that this Rlog-dependent classification approach is as independent as it can be from issues such as instrument calibration or changes in the optical or microphysical strength of the dust plume: the verification of this affirmation in terms of other sites or other seasons is a step that we are actively pursuing.
4.2.2 Subclass statistics
The POI subclass results for the complete month of May 2019 are shown in Fig. 4b1 and b2, while the corresponding correlation coefficient values are shown in Fig. 4a1 and a2. As one might expect for a true dust event, the results often show a degree of correlation between the blue and orange bars.15 Other examples of note are the two DNRS events of negative (see Sect. 4.2.3 for optical details on one of those events). Details of an 8 May URS event are discussed in Sect. 4.2.4. We also note the existence of five DGEN events (identified using the lidar profiles of the Supplement (Sect. S6) as well as the PlanetScope image on 27 May).
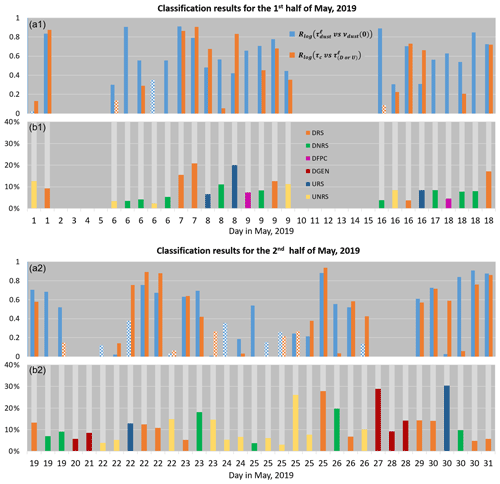
Figure 4May 2019 results: and for the upper profiles (a1, a2). DRS, DNRS, DFPC, DGEN, URS, and UNRS subclasses for the lower profiles (b1, b2) (the products of the classification scheme presented in Fig. 2). The subclasses are represented as duty cycles (event duration as a percent of 24 h). The bars with hatching of the upper profiles (a1, a2) represent negative R values. The light grey bars highlight the link between the subclasses and the Rlog values that served to define those subclasses. See Sect. 3.5 for details on the Rlog correlation coefficients as well as the D and U subclasses.
4.2.3 Cloud and pseudo-cloud illustrations during class D events
In many cases, the CIMEL processing resulted in no or very few AODs during periods when clouds were identified in the lidar profile (in spite of there being no nominal cloud screening for the Level 1.0 SDA+ product). However, there is a “triplet” filter at the beginning of the data processing chain which eliminates highly variable triplet ODs during the CIMEL 1 min bin-averaging period (see Giles et al., 2019). These highly variable ODs are not only restricted to clouds: they also included extremely variable CM dust AODs during periods of the day that were largely cloud free (what would amount to strong spatially variant dust plumes crossing the field of view (FOV) of the CIMEL).16 This problem was also reported by Evan et al. (2021).
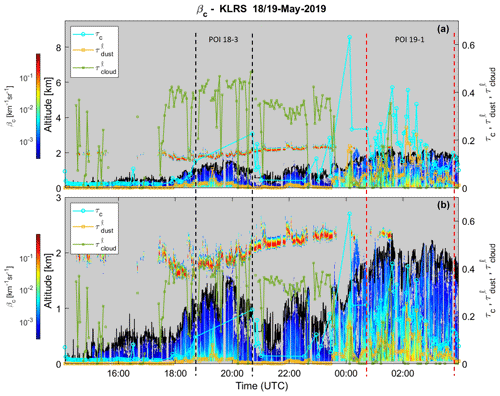
Figure 5D subclass events of 18 May and the beginning of 19 May 2019. Panel (a) shows the entire lidar altitude range, while panel (b) is restricted to the 0–3 km range in order to better appreciate the details of the dust plume (below ∼ 2 km) and the thin cloud layer hovering around 2 km. The dashed black and red vertical lines encompass two D subclass events (POI 18-3 and POI 19-1). The former event shows that no τc value survived the triplet filtering, while the latter DRS case indicates substantial correlation .
Figure 5 illustrates two types of D subclasses (between the dashed black and red vertical lines). The lidar captures cloud and dust intrusions between the black vertical lines (cloud layer ∼ 2 km and dust layers below 2 km) during which there are no SDA+ retrievals. This is an example of largely legitimate cloud screening by triplet filtering of AODs prior to promotion to Level 1.0 AODs (the significant high-frequency variation of the green curve supports this affirmation). The triplet filtering between the red vertical lines (encompassing a large cloud-free event) retains, on the other hand, a significant number of dust-correlated τc values to yield a DRS event. Note, however, that the Level 1.5 product (not shown) eliminates nearly all retrievals associated with the Level 1.0 DRS event: this amounts to an example of a DFPC event for Level 1.5 AODs (Fig. 4 of Evan et al., 2021, illustrates what is likely a DFPC event resulting from aggressive cloud screening being applied to Level 1.0 AODs). This discrepancy between the Level 1.0 and Level 1.5 subclasses indicates an excessive sensitivity of Level 1.5 cloud screenings to optical variations that are most likely local dust variations. This is, in fact, a situation where it is better to minimize cloud-screening protocols if the ultimate goal is detection and characterization of highly variable local dust.
Another cloud impact example is associated with the two negative cases referred to above: it is instructive to understand the optical dynamics of one of these cases (both of which were plagued by the presence of multi-altitude cloud). The POI 16-1 event at the beginning of 16 May (cf. the upper profile on p. 8 of the Supplement, Sect. S6) showed strong variation associated with17 vertically thin but optically thick clouds at ≲ 2 km altitude whose triplet impact appeared to create large gaps in the temporal variation of τc. In contrast, more homogeneous clouds at ∼ 4 km (whose values appear between gaps of the 2 km clouds but which themselves were interspersed with gaps induced by the strong attenuation of the 2 km clouds) sometimes evaded the triplet filtering. The coupling of these two different types of cloud interference did much to eliminate any possible covariation between the remaining τc points and .
4.2.4 Remote sensing threshold for the detection of local dust
Figure 6 shows a plot of vs. vdust(0) for all class D and U events during May of 2019. The graph suggests, for a certain fraction of the events, an apparent insensitivity (“bottoming out”) to vdust(0) variations below an apparent vdust(0) threshold of ∼ 10−11 µm3 µm−3. However the subclass scattergrams of the Supplement (Sect. S4) suggest a more nuanced threshold: the URS and UNRS scattergrams, in particular, show bottoming out vdust(0) thresholds that can be anywhere in the 10−11 to 10−10 µm3 µm−3 range with a respective range of 0.001 to 0.1. AboEl-Fetouh et al. (2020) showed pan-Arctic τc values (regional-scale, multi-year geometric means) whose τc variation18 was as small as ∼ 0.001. The fact that the bottoming-out values of the URS and UNRS scattergrams are generally well above this value suggests that the apparent lack of variability is attributable to very local, near-surface dust dynamics that are at the margins of column-scale detectability. This suggestion is consistent with the observation of Huck et al. (2023) on the importance of local-scale variability during Lhù'ààn Mân' dust storms.
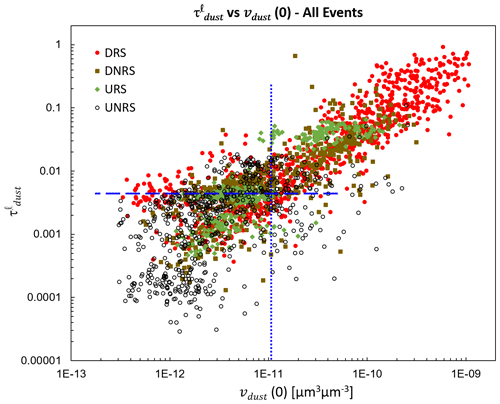
Figure 6Plot of vs. vdust(0) for all class D and U events during May of 2019. Note that this ensemble of data excludes points belonging to the special DFPC and DGEN subclasses. The dashed blue horizontal line represents the apparent bottoming-out effect, while the dotted vertical line represents the vdust(0) threshold (see text for details).
The POI 8-3 event of Fig. S1 in the Supplement underscores the relevance of the URS subclass assignment (of being large): the apparent bottoming out of all the point clusters in the URS scattergram of Fig. S4 is more about an apparently narrow spread driven by the densely packed log scale. The appearance of the POI 8-3 lidar profile and the temporal covariation of τc and (associated with the orange points of Fig. S4) is strongly suggestive of an optically weak dust plume for which the covariation of τc and is not some statistical artefact. This does not detract from the argument of local-scale vdust(0) variability being uncoupled from the columnar variability; it simply says that the physical significance of the columnar variability can occur within the (apparently narrow) spread of the point clusters in the URS scattergram.
4.3 Parameterization of dust plume properties
4.3.1 Lidar ratio
Prescribed lidar ratio
The CM reff values for the whole month of May 2019 were calculated for all individual KLRS OPS PSDs that were part of class D POI events. A temporal plot of those individual ) values can be seen in Fig. 7. The averaged class D results for the month of May were (as per the Fig. 7 legend) found to be µm.
Clay, feldspar, and then quartz minerals are the major constituents of the PM10 dust particles from the emissions in the Kluane Lake region (Bachelder et al., 2020; James King, unpublished data, 2023). To derive the mean refractive index and the uncertainty for those values, we followed the method of Baldo et al. (2020) using the volumetric-average refractive index based on the relative volume fraction weighted refractive indices of the minerals found within the dust sampled at the site, through X-ray diffraction, assuming an internally mixed sample (Formenti et al., 2014). The result, which we took to be representative of the Lhù'ààn Mân' region, was a mean refractive index of at the 1.548 µm lidar wavelength.
Figure 8 shows the range of lidar ratio curves given the mean and uncertainties of the Down Valley estimates of refractive index and a 0–12 µm range of reff,D values (the OPS-derived reff,D values and their uncertainties appear as orange circles). All lidar ratios were computed using a (spherical particle) Mie code (Blair T. N. Evans, personal communication, 1994) integrated over a lognormal PSD (see Sokolik and Toon, 1999, for a typical formulation and values of that analytical PSD). We employed Sokolik and Toon's geometric standard deviation for dust (“σj” = 2) and forced the lognormal geometric mean (“r0j”) to yield a computed lognormal-PSD effective radius that matched the OPS-derived 〈reff,D〉 values. The coloured curves of Fig. 8 nicely demonstrate the impact of the real and imaginary parts of the refractive index on the lidar ratio computations (a somewhat balanced impact at small reff,D in the neighbourhood of the OPS-derived values of reff,D with a much stronger impact of the complex part of the refractive index at larger radii). This yielded a prescribed lidar ratio estimate of sr (where the uncertainties were derived by combining the reff,D and refractive index uncertainties).
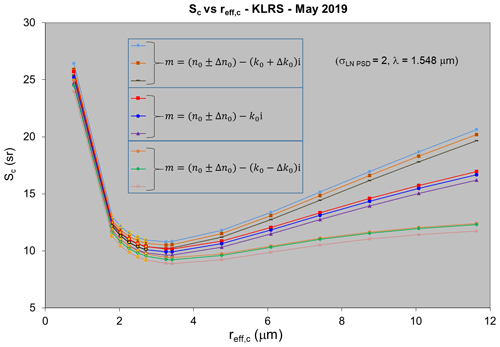
Figure 8Mie (spherical-particle) computations of lidar ratio based on the refractive index (n) value from above. The orange circles represent reff,D changes from to in increments of σ(reff,D). The refractive index parameters of the figure are n0=1.5371, Δn0=0.0028, k0=0.00075, and Δk0=0.00072. σLN PSD refers the standard deviation of the lognormal PSD that was employed in the Mie computations.
Evaluation/validation of the prescribed lidar ratio
Individual values for each DRS event were computed as per Appendix A1 (i.e., weighted best estimates of separate computations for each event where weights are defined by the inverse square of regression residuals). The overall weighted result for the entire month of May 2019 was sr. The weighted mean, , is roughly 3 times the value of 10.7±0.9 sr reported above (i.e., shows a positive bias). While the overall apparent effect of the weighting scheme, as seen in Fig. S5, was to reduce the variation as a function of increasing weight, it did not objectively achieve satisfactory agreement with . A measure of closer agreement with the value (a lesser positive bias) was obtained by setting a higher standard on the regressions or the amplitude of the slant path lidar optical depth:19 these two constraints yielded values of 19.1±2.3 and 20.3±2.6 sr for Rlog>0.9 or slant path optical depth >0.05, respectively. We note that the comment by Huck et al. (2023) concerning the frequent missing of plumes by the CIMEL (or presumably only capturing a portion of the plume in the CIMEL FOV) is actually a counter dynamic to the positive bias: a problem of partially missing the plume would actually help to reduce the bias.
Given the persistence of this positive bias, we sought to determine whether could, in fact, be underestimated. A significant increase in its computed value can be obtained by assuming the general presence of larger, optically significant dust particles in the plumes. Figure 8 indicates that an reff,D value of ∼ 11–12 µm and the higher value of the imaginary index of refraction determined from the dust speciation result would push the to values of ∼ 20 sr (a value commensurate with the “higher standard” argument in the previous paragraph). The OPS instrument with its upper limit of 5 µm radius (see the subsection “Intensive parameters from AERONET inversions” below) is incapable of measuring such a PSD contribution, while the few AERONET inversions that were available showed a dust mode of increasing radius (up to 7 µm) with increasing (but weak) values of dust optical depth (see the following section). Ranjbar et al. (2021) reported on the presence of dust particles ≳ 15 µm radius20 for CloudSat/CALIOP (DARDAR) retrievals over Lake Hazen dust plumes in the Canadian high Arctic (a drainage-basin environment analogous to that of Lhù'ààn Mân'). At southern latitudes, optically significant lidar profiles whose volume-median radius was ≳ 8 µm have been observed for near-source Saharan dust plumes (the Fig. 13, 20–28 May results of Weinzierl et al., 2009, and, as evidence of optical significance, the corresponding column averaged lidar ratios of Esselborn et al., 2009).
4.3.2 AERONET inversions
Table 1 lists key AERONET CM inversion products acquired during D class events for the month of May 2019 (optically based inversion products that were spectrally extrapolated to the 1.548 µm lidar wavelength from values at the shorter inversion wavelengths). The relatively few retrievals underscore the sometimes-frustrating disparity of dealing with the information-rich AERONET-inversion product when it is associated with few inversions during an event of interest (coupled with the fact that the CM AODs were generally weak: τc,inv < 0.15). This speaks to the difficulty in extracting significant numbers of AERONET retrievals even on the Table 1 days with the largest τc,inv values. However, if the information we want is of an intensive-parameter nature (effective radius, PSD radius attributes like PSD-peak positions, etc.) then we can aspire to extract representative values that vary little across a month (that do not need to be monitored at a high sampling rate).
Table 1AERONET May 2019 CM inversion products for all retrievals classified as DRS events (ordered according to decreasing τc,inv). With respect to the single URS (POI 8-3) case, the rather dominant τc,inv value and the appearance of its lidar profile in the Supplement (Sect. S6) suggest that it is most likely a dust event (it could, for example, be attributed a DGEN subclassification). The τc,inv values were derived from a spectral extrapolation (using log–log regressions) from the four AERONET inversion wavelengths to the 1.548 µm lidar wavelength. The colours associated with the different dates represent retrievals for the six largest τc,inv values (the same colours are used to identify the associated PSDs of Fig. 9).
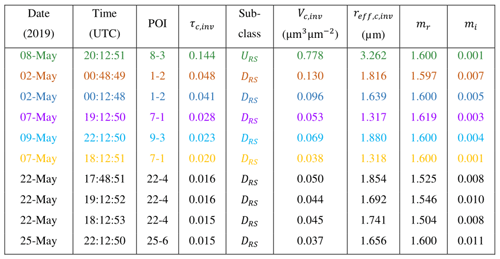
Intensive parameters from AERONET inversions
Figure 9 shows the set of PSDs corresponding to the retrievals of Table 1. One can observe bimodal CM peak positions near 1.3 and 5.0/6.6 µm (bin centres of bins 13 and 18/19, respectively, of the AERONET inversion). In general, there appears to be a trade-off between the 1.3 and 5.0/6.6 µm peaks depending on the strength of the relative contributions to each peak. AboEl-Fetouh et al. (2020) ascribed the presence of a 1.3 µm component to the springtime incursion of Asian dust over six AERONET stations spread across the North American and European Arctic (springtime being largely represented by April and May in their monthly averaged PSDs). The six largest τc,inv cases of Table 1 arguably represent the strongest local dust contributions. Four of these values are dominated by the 5.0–6.6 µm peak, while the other two are sufficiently weak to be dominated by a stronger 1.3 µm peak. The reff,c values of Table 1 represent a compromise driven by the 1.3 and 5.0–6.6 µm peak positions (where the importance of the latter peak tends to increase with increasing τc,inv). These reff,c values (with an average of 1.87 µm) are somewhat less than the OPS-derived mean of 2.26 µm.
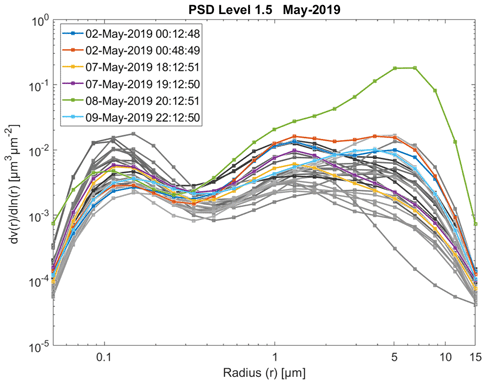
Figure 9AERONET-inversion PSDs () corresponding to the retrievals listed in Table 1 (the coloured PSD curves correspond to the colours of Table 1). The grey curves include the non-coloured entries of Table 1 as well as all other May 2019 retrievals that were not assigned to the DRS subclass.
The suggestion that the AERONET 1.3 µm PSD peak is due to Asian dust needs to be contextualized by a relatively consistent, neighbouring peak at the 1.75 µm OPC bin (radius) centre that was often recorded by the two DV units (see the Supplement (Sect. S7) to observe the daily ensemble of OPS PSDs at KLRS and the OPC PSDs at the DV site on the 6 measurement days corresponding to the six largest τc,inv events of Table 1). That peak was not observed by the OPS: the KLRS ensemble of PSDs on the 6 measurement days in question could be best described as a broad modal feature whose form was quite stable but whose amplitude was highly variable. The broad nature of that modal feature inhibits any simple characterization (one cannot readily select a significantly robust modal peak that stands above the other PSD values in neighbouring bins). In fact, it appears to be common to the KLRS and DV sites if one views the 1.75 µm DV peak as a perturbation atop of that feature. Given the lack of even a 1.75 µm peak at the KLRS site and the ubiquitous nature of the 1.3 µm peak over the North American and European Arctic (AboEl-Fetouh et al., 2020), it is likely that the 1.3 µm AERONET peak is associated with springtime Asian dust. Finally, we would note that the broad CM feature might well be dynamically associated with the 5.0–6.6 µm AERONET inversion peak (with the OPS effective radius arguably being smaller than the AERONET inversion effective radius due to its 4.5 µm, bin-centre, cutoff radius).
The significance of this result (in spite of our intensive parameter arguments at the beginning of this section) is necessarily brought into question by having only a handful of PSD retrievals over the whole month of May (and only one single retrieval with a dominant 6.6 µm component). In addition to this constraint on the number of retrieved PSDs, the τc,inv contribution of a 6.6 µm peak will generally be dominated by the τc,inv contribution of the 1.3 µm peak.21 The few retrievals and their low τc,inv values are likely attributable to the cloud-screening process of the AERONET inversions (whose constraints are commonly known to be more stringent than the cloud-screening protocol for AODs; this arguably amounts to the assignment of a DFPC subclass in the case of the inversion processing stream).
The values of the real part of the refractive index in Table 1 are clearly susceptible to retrieval saturation issues (most notably the evident flatlining at a value of 1.6). The values of the imaginary part of the refractive index are highly variable. In general, one cannot ascribe much confidence to the May 2019 refractive index values: the AERONET U27 inversion-error product described by Sinyuk et al. (2020) shows no values (corresponding to a code of “−999”) for the real and imaginary parts of the refractive index (and the derived single-scattering albedo). The authors indicate that no U27 values are reported “for any of the retrieved parameters” subject to a “boundary hit” (lower and upper limits of 1.33 to 1.6 represent such boundaries for the real part of the refractive index). Finally, we would note that the comments made above concerning the behaviour of the Fig. 9 PSDs are virtually unchanged if we restrict the retrieved PSDs to those that are not subject to boundary hits of the real part of the refractive index.
4.3.3 The remote sensing detectability of fine mode dust
The FM part of the KLRS and DV PSDs clearly covary with their CM analogue (see the PSDs in the Supplement, Sect. S7). This suggests a weak but robust presence of FM dust. While FM dust is likely a relatively minor optical component (especially at the lidar wavelength), its transport dynamics would likely differ from CM dust, and it could add to the long-distance spread of local dust in the Arctic. Figure 10 is a temporal plot of the 500 nm τf and τc AERONET product, as well the FM-integrated OPS particle-volume concentration (νf(0)) on the DRS (POI 7-1 and POI 7-2) day of 7 May 2019.22 Significant correlation of low and high frequency can be observed between τf and τc and between τf and νf(0). It should be noted that one expects a significant τf background (bottoming out) in the Arctic (see AboEl-Fetouh et al., 2020, for example), and this would tend to disrupt the dust-based correlation with τc and with νf(0) prior to the beginning of the dust event (around 19:20 UTC). This suggests that a satellite-based AOD remote sensing technique at a few visible and near-IR wavelengths (the equivalent of what is employed by AERONET to extract τf and τc at 500 nm) would have a reasonable chance of extracting a τf dust component and thus contribute to the type of spatial information that one could aspire to extract from satellite-based remote sensing (one can, specifically, aspire to extract the MODIS fine mode fraction product of that can then be converted to a τf estimate).
We reported on an automated dust classification scheme for which the first steps were the derivation of the dust plume height followed by the definition of a class of optically significant (class “D”) dust events. This higher-level classification scheme employed short-wave infrared (1.548 µm) lidar profiles (that were predominantly sensitive to coarse mode (CM) particles) coupled with surface-based microphysical measurements of CM particle-volume concentration. We defined two class-D subclasses (DRS and DNRS) as well as two complementary subclasses (DGEN and DFPC): these represented, respectively, events that could be remotely sensed from a passive ground-based (AERONET-type) spectral AOD sensor (and thus, for example, could likely be remotely sensed by a passive satellite sensor), dust events that could likely not be remotely sensed (NRS), dust events that were identified using data other than OPS data (and/or qualitative evidence such as RGB satellite images), and events that could not be remotely sensed because they were classified as clouds and thus eliminated from analytical consideration by the AERONET cloud-screening protocol. We also defined an unclassified (U) class: a URS subclass represented events whose surface-based CM particle-volume concentration measurements were speculated to be excessively influenced by local dust dynamics (i.e., not in the same dynamics scale as the remotely sensed (columnar) dust optical depths). The associated lack of dust sensitivity was estimated to have an upper limit threshold to be roughly in the 10−11 to 10−10 µm3 µm−3 range corresponding, respectively, to a CM optical depth of 0.001 to 0.1.
The average OPS effective radius of class-D events as measured at the KLRS site (near the CIMEL and lidar instruments) during the month of May 2019 was estimated to be 2.26±0.23 µm. A value of dust refractive index at the lidar wavelength was estimated as from an analysis of Lhù'ààn Mân' dust speciation classes. A prescribed lidar ratio of 10.7±0.9 sr was derived from Mie computations employing the OPS-derived effective radius average and the computed refractive index information as input. The CIMEL-derived lidar ratio showed a positive bias that was anywhere from 2 to 3 times the prescribed ratio depending on QA constraints placed upon the statistical data employed for its derivation. The persistence of this positive bias led to a hypothesis that the prescribed value could be increased to the CIMEL-derived values of 20 sr by hypothesizing the presence of optically significant dust particles at an effective radius of ∼ 11–12 µm. This large-particle hypothesis is not incoherent with OPS measurements (such a particle size is greater than its 4.5 µm upper limit) and is coherent with the fact that the AERONET PSD retrievals showed a CM whose peak radius increased with increasing dust optical depth.
AERONET inversions were few and far between (and generally weak in terms of τc amplitude during the whole month of May 2019). The available inversions showed bimodal CM PSDs with (AERONET bin-centre) peaks at radii ∼ 1.3 and 5.0–6.6 µm and a tendency to be progressively more dominated by the latter peak as τc,inv increased. Those larger τc,inv values generally occurred during DRS events. The smaller 1.3 µm radius peak was, we argued, likely to be the ubiquitous CM peak that AboEl-Fetouh et al. (2020) ascribed to springtime Asian dust. The latter 5.0–6.6 µm peak is arguably associated with a local Lhù'ààn Mân' dust peak represented by a broad 2.0–4.5 µm peak in the KLRS OPS data (but, arguably, of lesser effective radius because of the 4.5 µm (bin-centre) cutoff of the OPS PSDs).
Finally, we discussed the possibility of extracting a FM dust component using satellite-based remote sensing. This could yield a unique spatial perspective on the opto-physical dynamics of FM dust, a component that is not well understood (see, for example, Cottle et al., 2013) but might well play an important role in the spread of dust around the Arctic. Moving beyond the relatively coarse spatial resolution of the workhorse MODIS sensor, both the CM and FM AOD dust products could be derived for high-spatial-resolution satellites such as those of the PlanetScope constellation.
A1 Theoretical background for validation
Let be the current prescribed (constant) value of the dust lidar ratio that was employed to compute .23 The AERONET-derived value (Sc) for the DRS class would then be
If we are employing regressions over the POI,24 then
where a and b are the regression slope and intercept. Accordingly,
Employing Eq. (A2b) in the “reg” version of Eq. (A1) yields
This corresponds to a situation where a given data pair, e.g., , is forced to lie on the regression line (i.e., Eq. A2a is employed to compute the point ). An objective and pragmatic quality indicator of the lidar ratio would be the lidar ratio residuals (subscript “r”) given by
where Sc is the Sc of Eq. (A1), and where one would compute the average (ΔSc,r) and standard deviation (σ(ΔSc,r)) over each POI. In practice, there are large variations in ΔSc,r and attendant large variations of Sc. Weights defined as are an indicator of confidence in a given Sc value which places considerably more confidence in points that are close to the regression line (the weighting factor is inspired by the classical standard deviation weighting factors; see, for example, Taylor, 1997). The weighted means and standard deviation over a given DRS event would be then computed as
where we define . The weighting can produce excessively non-linear weights for single measurements very near the regression line. However, their impact is considerably dampened out, because we average over the POIs and then average those averages over the month.
A2 Processing-related notes of AERONET AODs
A2.1 SDA and SDA+ retrievals
The SDA and SDA+ retrievals are defined in O'Neill et al. (2003) and O'Neill et al. (2008), respectively. The SDA+ input wavelengths are 380, 440, 500, 675, 870, 1020, and 1640 nm. For the purposes of comparing AERONET data with the KLRS Doppler lidar, the SDA+ output wavelength was taken as 1548 nm.
A2.2 Backscatter coefficient (βc) profiles
The lidar profiles begin at a nominal altitude of 13 m. The minimum reliable measured range is < 100 m (typically 75 m) (Newsom and Krishnamurthy, 2022). Our investigation into the KLRS lidar measurements shows that column measurements < 51 m often appear to be artefactual (abnormally large and discontinuous βcT2 values as in the case of 1 May). Hence, we chose to assign NaN to βcT2 values in this altitude range (notably to eliminate their contribution to ).
A3 Measurements bins and intersample times: (i) native and (ii) common-bin configuration
To match the measurement times of all the ground-based CIMEL, lidar, and OPS instruments at the KLRS site, we employed the nominal CIMEL times (i.e., the centres of the “1m” CIMEL triplet bins) to generate a linear interpolation of lidar optical depths at their nominal times (the centre of their 11 s time bins) and of OPS CM volume densities at their nominal times (centre of their 1 min time bins) to the nominal CIMEL times.
CIMEL timing protocols
The CIMEL timing protocols, which are the basis for the τc time bins (actually any AERONET AOD time bin to be precise), can be found in Giles et al. (2019). Accordingly, a triplet AERONET measurement is performed every 3 min to separate homogeneously dispersed aerosols from highly temporally variable clouds. This is effected through a filtering process that measures triplet variability. As stated by Giles et al. (2019), a triplet is a
series of measurements of all filters starting at 0 s of the minute for a duration of about 8 s and then repeating this measurement sequence at 30 s and 60 s from the initial measurement time. The resulting 1 min averaged measurement [1 min average of the digital number for each filter of the three sets of the 8 s measurements] sequence is defined as a triplet measurement, and the maximum to minimum range of these measurements [between individual filter measurements of the 8 s sets] is termed the triplet variability …. The triplet measurements are performed … every 3 min for newer … CE318-T instruments
In this glossary, we employ, for the sake of simplicity, an argument of “(z)” to represent “(0,z)” for transmission (T) expressions and no argument for optical depth parameters when their columnar extent is made evident by their subscript.
AEROCAN | Canadian subnetwork of AERONET |
AERONET | AErosol RObotic NETwork of CIMEL sun photometer/sky radiometers |
β′(z) | Attenuated backscatter coefficient ( with units of km−1 sr−1) |
AOD | Aerosol optical depth (unitless) |
β′(z) | Attenuated backscatter coefficient ( with units of km−1 sr−1) |
βc(z) | Coarse mode backscatter coefficient (units of km−1 sr−1) |
Coarse mode attenuated backscatter coefficient ( with units of km−1 sr−1) | |
βc approximation given by Eq. (2) | |
Cal/Val | Calibration/validation |
CALIOP | Cloud-Aerosol LIdar with Orthogonal Polarization |
CloudSat | Satellite whose radar profiler and IR imager are dedicated to the remote sensing of cloud properties |
CM | Coarse mode (generally supermicron radius; see O'Neill et al., 2023, for a general discussion of microphysical and optical considerations) |
D | Classification symbol for optically significant dust event |
DFPC | “D” subclass symbol for an FPC event |
DGEN | “D” subclass symbol for dust than has been identified using alternate (“generic”) information |
DNRS | “D” subclass symbol for optically significant dust that cannot be remotely sensed |
DRS | “D” subclass symbol for optically significant dust that can be remotely sensed |
DARDAR | raDAR/liDAR; retrieving cloud and aerosol properties by combining the CloudSat radar and the CALIPSO lidar measurements |
DLH | Dust layer height (units of km) |
DV | Down Valley |
Particle-volume distribution (µm3 µm−3 per unit increment in log diameter). This PSD is derived from the particle-number PSD output of the OPS instruments ( where dn is the particle number in a given size bin). Note that . | |
Particle-volume distribution (µm3 µm−3 per unit increment in ln r). Note that . | |
Columnar particle-volume per unit area in the differential bin dln r (this is an AERONET inversion product in units of µm3 µm−2) | |
FLEXPART | FLEXible PARTicle dispersion model |
FPC | Subclass subscript acronym for a dust-induced false positive cloud event |
FM | Fine mode (generally submicron radius; see O'Neill et al., 2023, for a general discussion of microphysical and optical considerations) |
HLD | High-latitude dust |
KLRS | Kluane Lake (Lhù'ààn Mân') Research Station |
LCH | Lidar ceiling height (9.6 km) |
MCA | Minimum cloud altitude (units of km) |
MISR | Multi-angle Imaging SpectroRadiometer |
NaN | Not a number |
OD | Optical depth |
OPC | Optical particle counter (from which νdust(0) is determined) |
OPS | Optical particle sizer (from which νdust(0) is determined) |
POI | Period of interest |
PSD | Particle size distribution |
QA | Quality assurance |
r | Radius (µm) |
Rlog | Correlation coefficient in log–log space ( vs. log τc, for example) |
SDA | Spectral deconvolution algorithm |
Sc | AERONET-derived lidar ratio |
Prescribed lidar ratio computed from Mie calculation using surface measured estimates of dust-particle effective radius and refractive index | |
Sc,reg | AERONET-derived lidar ratio where we force a given data pair, e.g., , to lie on the regression line (subclass DRS data pairs: the “c” subscript is used to simplify the nomenclature) |
σ(x) | Arithmetic standard deviation of the parameter x |
Tc | Altitude dependent (one-way) transmission of a lidar pulse |
Tc(z) approximation obtained by employing the ratio as the altitude-defining profile of τc(0,z) | |
Column-integrated CM backscatter coefficient (coarse mode backscatter optical depth) | |
Column-integrated CM attenuated backscatter coefficient (CM attenuated backscatter optical depth) | |
τc | CM AOD at 1548 nm (AERONET SDA+ product) |
τc,inv | CM AOD at 1548 nm (extrapolation of the four-band AERONET-inversion product) |
CM lidar AOD at 1548 nm with integrating range of 0–LCH | |
CM lidar AOD at 1548 nm where we force a given data pair, e.g., , to lie on the regression line | |
CM lidar AOD at 1548 nm with integrating range of 0–DLH | |
Sequence of values that are promoted to a class D POI | |
CM lidar AOD at 1548 nm with integrating range of DLH–LCH (cloud region) | |
U | Classification acronym for events that failed to achieve a D classification |
νdust | Surface (z=0) CM OPS particle-volume concentration at KLRS (units of µm3 µm−3). The CM OPS integration was computed over nine bins whose bin-centre radii ranged from 0.78 to 4.51 µm. |
Vc | AERONET-derived, CM columnar particle-volume concentration (units of µm3 µm−2). The CM integration of the retrieval is computed from the bin containing the minimum (that dynamic minimum being constrained to a bin-centre radius range from 0.439 to 0.992 µm) to the largest retrieval bin (15 µm bin-centre radius). |
〈x〉 | Arithmetic mean of the parameter x |
z | Altitude above ground level (units of km) |
The “IPHASE” Mie code that we employed for this paper was written by Blair T. N. Evans, a former scientist of the Dept. of National Defense, Defense Research Establishment in Val Cartier (DREV), Quebec, Canada. This person has since left the service and no longer maintains the IPHASE code. We have, over the years, diligently verified every aspect of the code options that affected our analyses (often in consultation with Blair T. N. Evans). Those verifications can be made available upon request. The code is not publicly available: a copy can, however, be obtained via a special request addressed to co-author Norm O'Neill (norm.oneill@usherbrooke.ca).
AERONET data are available for download at https://doi.org/10.17616/R3VK9T (Lind and Gupta, 2023). The KLRS (Kluane Lake Research Station) OPS (volumetric-sampling) particle size distributions, SDA+ retrievals of coarse mode aerosol optical depth (CM AOD), and the temporally matched AERONET and Lidar CM AODs of May 2019 can be found at the following DOI: https://doi.org/10.5281/zenodo.8310097 (Sayedain and O'Neill, 2023).
The supplement related to this article is available online at: https://doi.org/10.5194/amt-16-4115-2023-supplement.
SAS: writing (original draft preparation, review and editing), visualization, investigation, conceptualization, methodology, formal analysis, data curation, validation, software. NTO'N: writing (review and editing), supervision, visualization, conceptualization, methodology, formal analysis, data curation, validation, funding acquisition, resources. JK: writing (review and editing), supervision, data curation, funding acquisition, resources. PLH: writing (review and editing), data curation, resources. DB: writing (review and editing), data curation, validation. RW: writing (review and editing), data curation, resources. SE: writing (review and editing), data curation, resources. AVL: writing (review and editing), validation. JB: validation. MB: validation.
The contact author has declared that none of the authors has any competing interests.
Publisher's note: Copernicus Publications remains neutral with regard to jurisdictional claims in published maps and institutional affiliations.
Support was provided by the Canadian Mountain Network (CMN) and the Discovery Grant program of the Canadian National Sciences and Engineering Research Council (NSERC). Valuable in-kind support was provided, respectively, by the AEROCAN network of Environment and Climate Change Canada (ECCC) and NASA AERONET. Valuable AERONET processing advice was provided by Tom Eck and Sasha Smirnov of the AERONET team.
This research has been supported by the Natural Sciences and Engineering Research Council of Canada (grant nos. RGPIN-2017-05531 and RGPIN-2016-05417), the Canadian Mountain Network (Networks of the Centres of Excellence of Canada) and the Canada Foundation for Innovation (grant no. 36564).
This paper was edited by Andrew Sayer and reviewed by Pavla Dagsson Waldhauserova and two anonymous referees.
AboEl-Fetouh, Y., O'Neill, N. T., Ranjbar, K., Hesaraki, S., Abboud, I., and Sobolewski, P. S.: Climatological-Scale Analysis of Intensive and Semi-intensive Aerosol Parameters Derived From AERONET Retrievals Over the Arctic, J. Geophys. Res.-Atmos., 125, e2019JD031569, https://doi.org/10.1029/2019jd031569, 2020.
Bachelder, J., Cadieux, M., Liu-Kang, C., Lambert, P., Filoche, A., Galhardi, J. A., Hadioui, M., Chaput, A., Bastien-Thibault, M. P., Wilkinson, K. J., King, J., and Hayes, P. L.: Chemical and microphysical properties of wind-blown dust near an actively retreating glacier in Yukon, Canada, Aerosol Sci. Tech., 54, 2–20, https://doi.org/10.1080/02786826.2019.1676394, 2020.
Baldo, C., Formenti, P., Nowak, S., Chevaillier, S., Cazaunau, M., Pangui, E., Di Biagio, C., Doussin, J.-F., Ignatyev, K., Dagsson-Waldhauserova, P., Arnalds, O., MacKenzie, A. R., and Shi, Z.: Distinct chemical and mineralogical composition of Icelandic dust compared to northern African and Asian dust, Atmos. Chem. Phys., 20, 13521–13539, https://doi.org/10.5194/acp-20-13521-2020, 2020.
Bullard, J. E., Matthew, B., Tom, B., John, C., Eleanor, D., Diego, G., Santiago, G., Gudrun, G., Richard, H., Robert, M., Cheryl, M.-N., Tom, M., Helena, S., and Thorsteinsson, T.: High latitude dust in the Earth system, Rev. Geophys., 54, 447–485, https://doi.org/10.1002/2016RG000518, 2016.
Chiang, C.-W., Nee, J.-B., and Chen, W.-N.: Lidar ratio and depolarization ratio for cirrus clouds, Appl. Optics, 41, 6470–6476, https://doi.org/10.1364/AO.41.006470, 2002.
Cottle, P., Strawbridge, K., McKendry, I., O'Neill, N., and Saha, A.: A pervasive and persistent Asian dust event over North America during spring 2010: lidar and sunphotometer observations, Atmos. Chem. Phys., 13, 4515–4527, https://doi.org/10.5194/acp-13-4515-2013, 2013.
Crusius, J., Schroth, A. W., Gassó, S., Moy, C. M., Levy, R. C., and Gatica, M.: Glacial flour dust storms in the Gulf of Alaska: Hydrologic and meteorological controls and their importance as a source of bioavailable iron, Geophys. Res. Lett., 38, 1–5, https://doi.org/10.1029/2010GL046573, 2011.
Dagsson-Waldhauserova, P., Renard, J. B., Olafsson, H., Vignelles, D., Berthet, G., Verdier, N., and Duverger, V.: Vertical distribution of aerosols in dust storms during the Arctic winter, Sci. Rep., 9, 1–11, https://doi.org/10.1038/s41598-019-51764-y, 2019.
Dörnbrack, A., Stachlewska, I. S., Ritter, C., and Neuber, R.: Aerosol distribution around Svalbard during intense easterly winds, Atmos. Chem. Phys., 10, 1473–1490, https://doi.org/10.5194/acp-10-1473-2010, 2010.
Dubovik, O. and King, M. D.: A flexible inversion algorithm for retrieval of aerosol optical properties from Sun and sky radiance measurements, J. Geophys. Res.-Atmos., 105, 20673–20696, https://doi.org/10.1029/2000JD900282, 2000.
Esselborn, M., Wirth, M., Fix, A., Weinzierl, B., Rasp, K., Tesche, M., and Petzold, A.: Spatial distribution and optical properties of Saharan dust observed by airborne high spectral resolution lidar during SAMUM 2006, Tellus B, 61, 131–143, https://doi.org/10.1111/j.1600-0889.2008.00394.x, 2009.
Evan, A., Walkowiak, B., and Frouin, R.: On the Misclassification of Dust as Cloud at an AERONET site in the Sonoran Desert, J. Atmos. Ocean. Tech., 1, 181–191, https://doi.org/10.1175/JTECH-D-21-0114.1, 2021.
Formenti, P., Caquineau, S., Desboeufs, K., Klaver, A., Chevaillier, S., Journet, E., and Rajot, J. L.: Mapping the physico-chemical properties of mineral dust in western Africa: mineralogical composition, Atmos. Chem. Phys., 14, 10663–10686, https://doi.org/10.5194/acp-14-10663-2014, 2014.
Giles, D. M., Sinyuk, A., Sorokin, M. G., Schafer, J. S., Smirnov, A., Slutsker, I., Eck, T. F., Holben, B. N., Lewis, J. R., Campbell, J. R., Welton, E. J., Korkin, S. V., and Lyapustin, A. I.: Advancements in the Aerosol Robotic Network (AERONET) Version 3 database – automated near-real-time quality control algorithm with improved cloud screening for Sun photometer aerosol optical depth (AOD) measurements, Atmos. Meas. Tech., 12, 169–209, https://doi.org/10.5194/amt-12-169-2019, 2019.
Groot Zwaaftink, C. D., Grythe, H., Skov, H., and Stohl, A.: Substantial contribution of northern high-latitude sources to mineral dust in the Arctic, J. Geophys. Res., 121, 13678–13697, https://doi.org/10.1002/2016JD025482, 2016.
Hansen, J. E. and Travis, L. D.: Light scattering in planetary atmospheres, Space Sci. Rev., 16, 527–610, https://doi.org/10.1007/BF00168069, 1974.
Hesaraki, S., O'Neill, N. T., Lesins, G., Saha, A., Martin, R. V., Fioletov, V. E., Baibakov, K., and Abboud, I.: Comparisons of a Chemical Transport Model with a Four-Year (April to September) Analysis of Fine- and Coarse-Mode Aerosol Optical Depth Retrievals Over the Canadian Arctic, Atmos.-Ocean, 55, 213–229, https://doi.org/10.1080/07055900.2017.1356263, 2017.
Holben, B. N., Eck, T. F., Slutsker, I., Tanré, D., Buis, J. P., Setzer, A., Vermote, E., Reagan, J. A., Kaufman, Y. J., Nakajima, T., Lavenu, F., Jankowiak, I., and Smirnov, A.: AERONET – A federated instrument network and data archive for aerosol characterization, Remote Sens. Environ., 66, 1–16, https://doi.org/10.1016/S0034-4257(98)00031-5, 1998.
Huck, R., Bryant, R. G., and King, J.: The (mis)identification of high-latitude dust events using remote sensing methods in the Yukon, Canada: a sub-daily variability analysis, Atmos. Chem. Phys., 23, 6299–6318, https://doi.org/10.5194/acp-23-6299-2023, 2023.
Kawai, K., Matsui, H., and Tobo, Y.: Dominant Role of Arctic Dust With High Ice Nucleating Ability in the Arctic Lower Troposphere, Geophys. Res. Lett., 50, 1–10, https://doi.org/10.1029/2022GL102470, 2023.
Lind, E. and Gupta, P.: AERONET, Registry of Research Data Repositories [data set], https://doi.org/10.17616/R3VK9T, 2023.
Meinander, O., Dagsson-Waldhauserova, P., Amosov, P., Aseyeva, E., Atkins, C., Baklanov, A., Baldo, C., Barr, S. L., Barzycka, B., Benning, L. G., Cvetkovic, B., Enchilik, P., Frolov, D., Gassó, S., Kandler, K., Kasimov, N., Kavan, J., King, J., Koroleva, T., Krupskaya, V., Kulmala, M., Kusiak, M., Lappalainen, H. K., Laska, M., Lasne, J., Lewandowski, M., Luks, B., McQuaid, J. B., Moroni, B., Murray, B., Möhler, O., Nawrot, A., Nickovic, S., O’Neill, N. T., Pejanovic, G., Popovicheva, O., Ranjbar, K., Romanias, M., Samonova, O., Sanchez-Marroquin, A., Schepanski, K., Semenkov, I., Sharapova, A., Shevnina, E., Shi, Z., Sofiev, M., Thevenet, F., Thorsteinsson, T., Timofeev, M., Umo, N. S., Uppstu, A., Urupina, D., Varga, G., Werner, T., Arnalds, O., and Vukovic Vimic, A.: Newly identified climatically and environmentally significant high-latitude dust sources, Atmos. Chem. Phys., 22, 11889–11930, https://doi.org/10.5194/acp-22-11889-2022, 2022.
Newsom, R. K. and Krishnamurthy, R.: Doppler Lidar (DL) Instrument Handbook, U.S. Department of Energy, Atmospheric Radiation Measurement user facility, Richland, Washington, DOE/SCARM-TR-101, https://doi.org/10.2172/1034640, 2022.
Nickling, W. G.: Eolian Sediment Transport During Dust Storms: Slims River Valley, Yukon Territory, Can. J. Earth Sci., 15, 1069–1084, https://doi.org/10.1139/e78-114, 1978.
O'Neill, N. T., Ignatov, A., Holben, B. N., and Eck, T. F.: The lognormal distribution as a reference for reporting aerosol optical depth statistics; Empirical tests using multi-year, multi-site AERONET sunphotometer data, Geophys. Res. Lett., 27, 3333–3336, https://doi.org/10.1029/2000GL011581, 2000.
O'Neill, N. T., Eck, T. F., Smirnov, A., Holben, B. N., and Thulasiraman, S.: Spectral discrimination of coarse and fine mode optical depth, J. Geophys. Res.-Atmos., 108, 1–15, https://doi.org/10.1029/2002jd002975, 2003.
O'Neill, N. T., Eck, T. F., Reid, J. S., Smirnov, A., and Pancrati, O.: Coarse mode optical information retrievable using ultraviolet to short-wave infrared Sun photometry: Application to United Arab Emirates Unified Aerosol Experiment data, J. Geophys. Res.-Atmos., 113, 1–11, https://doi.org/10.1029/2007JD009052, 2008.
O'Neill, N. T., Ranjbar, K., Ivănescu, L., Eck, T. F., Reid, J. S., Giles, D. M., Pérez-Ramírez, D., and Chaubey, J. P.: Relationship between the sub-micron fraction (SMF) and fine-mode fraction (FMF) in the context of AERONET retrievals, Atmos. Meas. Tech., 16, 1103–1120, https://doi.org/10.5194/amt-16-1103-2023, 2023.
Pearson, G., Davies, F., and Collier, C.: An analysis of the performance of the UFAM pulsed Doppler lidar for observing the boundary layer, J. Atmos. Ocean. Tech., 26, 240–250, https://doi.org/10.1175/2008JTECHA1128.1, 2009.
Ranjbar, K., O'Neill, N. T., Ivanescu, L., King, J., and Hayes, P. L.: Remote sensing of a high-Arctic, local dust event over Lake Hazen (Ellesmere Island, Nunavut, Canada), Atmos. Environ., 246, 118102, https://doi.org/10.1016/j.atmosenv.2020.118102, 2021.
Rozwadowska, A., Zieliński, T., Petelski, T., and Sobolewski, P.: Cluster analysis of the impact of air back-trajectories on aerosol optical properties at Hornsund, Spitsbergen, Atmos. Chem. Phys., 10, 877–893, https://doi.org/10.5194/acp-10-877-2010, 2010.
Sayedain, S. A. and O'Neill, N. T.: OPS particle size distribution, AERONET and Lidar aerosol optical depth data employed in amt-2023-67, Zenodo [data set], https://doi.org/10.5281/zenodo.8310097, 2023.
Sayer, A. M. and Knobelspiesse, K. D.: How should we aggregate data? Methods accounting for the numerical distributions, with an assessment of aerosol optical depth, Atmos. Chem. Phys., 19, 15023–15048, https://doi.org/10.5194/acp-19-15023-2019, 2019.
Shugar, D. H., Clague, J. J., Best, J. L., Schoof, C., Willis, M. J., Copland, L., and Roe, G. H.: River piracy and drainage basin reorganization led by climate-driven glacier retreat, Nat. Geosci., 10, 370–375, https://doi.org/10.1038/ngeo2932, 2017.
Sinyuk, A., Holben, B. N., Eck, T. F., Giles, D. M., Slutsker, I., Korkin, S., Schafer, J. S., Smirnov, A., Sorokin, M., and Lyapustin, A.: The AERONET Version 3 aerosol retrieval algorithm, associated uncertainties and comparisons to Version 2, Atmos. Meas. Tech., 13, 3375–3411, https://doi.org/10.5194/amt-13-3375-2020, 2020.
Sokolik, I. N. and Toon, O. B.: Incorporation of mineralogical composition into models of the radiative properties of mineral aerosol from UV to IR wavelengths, J. Geophys. Res.-Atmos., 104, 9423–9444, https://doi.org/10.1029/1998JD200048, 1999.
Taylor, J. R.: An introduction to error analysis: the study of uncertainties in physical measurements, University Science Books, 327 pp., ISBN 093570275X, 1997.
Weinzierl, B., Petzold, A., Esselborn, M., Wirth, M., Rasp, K., Kandler, K., Schütz, L., Koepke, P., and Fiebig, M.: Airborne measurements of dust layer properties, particle size distribution and mixing state of Saharan dust during SAMUM 2006, Tellus B, 61, 96–117, https://doi.org/10.1111/j.1600-0889.2008.00392.x, 2009.
Weitkamp, C.: Lidar: range-resolved optical remote sensing of the atmosphere, in: Springer series in optical sciences, Springer New York, NY, https://doi.org/10.1007/b106786, 2005.
Yang, S., Preißler, J., Wiegner, M., von Löwis, S., Petersen, G. N., Parks, M. M., and Finger, D. C.: Monitoring dust events using doppler lidar and ceilometer in Iceland, Atmosphere, 11, 1–23, https://doi.org/10.3390/atmos11121294, 2020.
Lhù'ààn Mân' is the Southern Tutchone name for Kluane Lake. Southern Tutchone is one of seven Athapaskan languages in the Yukon and is spoken by Kluane First Nation people.
Particles whose radius is microphysically or (in an indirect fashion) optically ≳ 1 µm (see, for example, O'Neill et al., 2023).
The AERONET CM AOD product being, on average, significantly less than the total AOD (AboEl-Fetouh et al., 2020) and, in principle, much more sensitive to local CM aerosols such as sea salt and local dust.
Including two bands at 1020 nm that are associated with the overlap region of the two CIMEL detectors (the VIS–NIR and NIR–SWIR detectors).
Particles whose radius is microphysically or (in an indirect fashion) optically ≲ 1 µm (O'Neill et al., 2023).
Across 22 size bins whose bin-centre radii extend from 0.14 to 5.0 µm (bin-centre diameters from 0.28 to 10 µm).
For FM AODs of ≲ 0.4 at 500 nm (A maximum FM AOD of 0.4 is generally greater than any values that could be found, in the absence of the easily detectible influence of biomass burning smoke, at Lhù'ààn Mân'.) During the month of May 2019, there were FM AOD events on 7/8 and 24 May (associated, it would appear, with CM dust events) for which the 500 nm FM AOD for the former case was as large as ∼ 0.2.
To be clear about the definition of lidar ratio, we note that its general definition is (with a Rayleigh/molecular value of ), and that ω0 and p(π) are, respectively, the single-scattering albedo and scattering phase function at a scattering angle of π radians and where the integration of over 4π steradians is unity.
Or more precisely, the radii of spherical particles that would be optically equivalent to irregularly shaped particles.
The latter correlation is complicated by AERONET cloud-screening protocols: potentially strong dust-induced correlations might, for example, be inadvertently eliminated by the Level 1.0 triplet processing or the Level 1.5 cloud-screening process.
The 0.001 minimum is an order of magnitude estimate of τc sensitivity to real physical CM changes (an estimate based on experiential evidence such as the seasonal variations observed by AboEl-Fetouh et al., 2020).
Actually the profile is that of the parameter of Eq. (2): we dropped the “∼” subscript in our profile figures for the sake of simplicity.
The “white-night” period that we defined as the sun being below 10∘ elevation (indicated by the darker grey shading).
Where the lidar OPS measurements are resampled to CIMEL averaging bins of 1 min and intersample gaps of 3 min.
Between the class-determining correlation coefficients of and .
At the other cloud-screening extreme are false negative clouds associated with homogeneous clouds such as cirrus that go undetected. V3 processing incorporated some significant changes in an attempt to deal with this problem (Giles et al., 2019).
∼ 100 m in vertical thickness and optical depths ≳ 1. The βcloud values associated with those clouds are removed in the lower profile of slide 8 (as part of the DLH process).
Variations that appeared to be robustly sensitive to certain natural Asian dust events.
where 〈m〉 is the POI-averaged air mass of the sun: the hypothetical case where the lidar is probing a plane-parallel plume at the same zenith angle as the sun.
The lower limit of 15 µm being determined by CloudSat radar detection limitations.
It is easy to approximately show, using Mie calculations and a refractive index ∼ 1.6 (employing the approximate AERONET retrieval value for optical consistency), that the extinction efficiency associated with the AERONET particle-volume distribution (whose product, integrated over radius, would yield τc,inv) is significantly larger at 1.3 µm radius (see, for example, Hansen and Travis, 1974, for a definition of “efficiency factor”, which is the parameter analogous to what we call extinction efficiency).
The limitation of τc to 500 nm applies only to this specific case: otherwise τc is computed at the lidar wavelength of 1.548 µm in the rest of the paper.
Note that we have deliberately avoided using the DRS classification symbol to minimize the complexity of the equations.
In the absence of instrumental or environmental artefacts, τc and should be governed by Eq. (A1) for a given POI (i.e., assuming Sc does not change during that POI). Accordingly, , which is the analogue of Eq. (A2a) with a = 1. Inserting this expression into Eq. (A1) yields . Accordingly, in the absence of instrumental or environmental artefacts, an (Eq. A2a) regression slope of a→1 and Rlog→1 should yield better estimates of Sc.
- Abstract
- Introduction
- Research site and instrumentation
- Methodology
- Results
- Conclusions
- Appendix A
- Appendix B: Acronym and symbol glossary
- Code availability
- Data availability
- Author contributions
- Competing interests
- Disclaimer
- Acknowledgements
- Financial support
- Review statement
- References
- Supplement
- Abstract
- Introduction
- Research site and instrumentation
- Methodology
- Results
- Conclusions
- Appendix A
- Appendix B: Acronym and symbol glossary
- Code availability
- Data availability
- Author contributions
- Competing interests
- Disclaimer
- Acknowledgements
- Financial support
- Review statement
- References
- Supplement