the Creative Commons Attribution 4.0 License.
the Creative Commons Attribution 4.0 License.
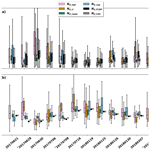
Evaluation of four ground-based retrievals of cloud droplet number concentration in marine stratocumulus with aircraft in situ measurements
Andrew M. Vogelmann
Edward Luke
Pavlos Kollias
Zhien Wang
William I. Gustafson Jr.
Susanne Glienke
Jason Tomlinson
Neel Desai
Cloud droplet number concentration (Nd) is crucial for understanding aerosol–cloud interactions (ACI) and associated radiative effects. We present evaluations of four ground-based Nd retrievals based on comprehensive datasets from the Atmospheric Radiation Measurement (ARM) Aerosol and Cloud Experiments in the Eastern North Atlantic (ACE-ENA) field campaign. The Nd retrieval methods use ARM ENA observatory ground-based remote sensing observations from a micropulse lidar, Raman lidar, cloud radar, and the ARM NDROP (Droplet Number Concentration) value-added product (VAP), all of which also retrieve cloud effective radius (re). The retrievals are compared against aircraft measurements from the fast cloud droplet probe (FCDP) and the cloud and aerosol spectrometer (CAS) obtained from low-level marine boundary layer clouds on 12 flight days during summer and winter seasons. Additionally, the in situ measurements are used to validate the assumptions and characterizations used in the retrieval algorithms. Statistical comparisons of the probability distribution function (PDF) of the Nd and cloud re retrievals with aircraft measurements demonstrate that these retrievals align well with in situ measurements for overcast clouds, but they may substantially differ for broken clouds or clouds with low liquid water path (LWP). The retrievals are applied to 4 years of ground-based remote sensing measurements of overcast marine boundary layer clouds at the ARM ENA observatory to find that Nd (re) values exhibit seasonal variations, with higher (lower) values during the summer season and lower (higher) values during the winter season. The ensemble of various retrievals using different measurements and retrieval algorithms such as those in this paper can help to quantify Nd retrieval uncertainties and identify reliable Nd retrieval scenarios. Of the retrieval methods, we recommend using the micropulse lidar-based method. This method has good agreement with in situ measurements, less sensitivity to issues arising from precipitation and low cloud LWP and/or optical depth, and broad applicability by functioning for both daytime and nighttime conditions.
- Article
(3712 KB) - Full-text XML
-
Supplement
(2383 KB) - BibTeX
- EndNote
Clouds play a crucial role in regulating the energy balance and water cycle of the Earth (Stephens et al., 2012). By reflecting incoming solar radiation back to space (the “albedo effect”) and trapping outgoing longwave radiation (the “greenhouse effect”), they cause both cooling and warming effects on Earth's climate. On a global scale, clouds have a net cooling effect of approximately 20 W m−2, which is more than 5 times greater than the warming effect caused by doubling the concentration of atmospheric CO2 (IPCC, 2021). Hence, even small changes in cloud properties, such as those induced by anthropogenic activities like aerosol emissions, can significantly impact Earth's climate sensitivity (Zelinka et al., 2017). Aerosols indirectly affect cloud properties by serving as cloud condensation nuclei (CCN) or ice nucleation particles. Such effects can increase the cloud droplet number concentration (Nd) and decrease their sizes, which can substantially alter cloud radiative properties and precipitation efficiency (Twomey, 1977; Albrecht, 1989). Recent studies have also revealed that aerosol–cloud interactions (ACI) are strongly influenced by atmospheric dynamics and thermodynamic conditions, as well as the physical properties and chemical compositions of aerosols (Chen et al., 2016; Fan et al., 2016). The uncertainty in the magnitude of ACI remains the largest source of uncertainty in estimates of climate forcing (IPCC, 2021; Regayre et al., 2014). Nd, which is a direct link between cloud properties and aerosol concentrations, is of utmost importance in improving our understanding of ACI processes and quantifying their effective radiative forcing (Rosenfeld et al., 2019).
To improve the representation of clouds in weather and climate models, it is essential to validate modeled Nd against observations (Storelvmo et al., 2006; Moore et al., 2013; Gryspeerdt et al., 2017). Although aircraft in situ instruments can measure Nd directly, these measurements are limited to specific regions and time periods during field campaigns. Collecting a large Nd database from these measurements is a challenging task, making it difficult to statistically study factors that influence the spatial and temporal variations in Nd and ACI processes across different climate zones and atmospheric thermodynamic conditions. Ground-based and spaceborne remote sensing techniques provide continuous observations of clouds and aerosols across different regions, and the latter includes global scales. Remote sensing measurements have been widely used to retrieve aerosol and cloud properties including Nd. Grosvenor et al. (2018) comprehensively reviewed passive satellite remote sensing retrievals of Nd from the retrieved cloud optical depth, cloud droplet effective radius (re), and cloud-top temperature. They concluded that satellite Nd retrievals could achieve a relative uncertainty of 78 % at the pixel level for single-layer warm stratiform and optically thick clouds. Ground-based Nd retrievals have higher temporal and spatial resolutions than satellite measurements. By taking advantage of more reliable retrievals of liquid water path (LWP) from passive microwave radiometers, ground-based Nd retrievals usually use cloud optical depth and LWP instead of re in the retrieval algorithms. These remote sensing data provide invaluable information for statistically studying ACI processes and have been used to validate and improve cloud representations in climate models (McComiskey et al., 2009; Rosenfeld et al., 2019; McCoy et al., 2020).
Passive remote sensing Nd retrievals, such as those noted above, commonly rely on reflected or transmitted sunlight measured from spaceborne and ground-based remote sensors, respectively. Therefore, these retrievals are limited to single-layer and optically thick clouds under conditions when the sun is high in the sky. These limitations can be alleviated by using active remote sensing measurements. Active remote sensors transmit electromagnetic waves at a specific visible, infrared, or microwave wavelength and receive reflected signals from the atmosphere in a narrow field of view. Therefore, active remote sensing measurements can be used for cloud property retrievals anytime (i.e., including nighttime) and under much broader atmospheric conditions (e.g., beneath cirrus cloud decks).
Ground-based active remote sensing Nd retrievals use either the cloud radar reflectivity factor (Z) or lidar extinction coefficient (βe) profiles, together with microwave-radiometer-retrieved LWP. A monomodal droplet size distribution (DSD) is usually assumed to connect these measured quantities. Radar-based Nd retrievals use the relationships between Z, liquid water content (LWC), DSD, and Nd (Dong et al., 1998; Mace and Sassen, 2000; Wu et al., 2020a). Since Z is proportional to the sixth power of the DSD, radar-based Nd retrievals are very sensitive to the assumed DSD, and it is challenging to retrieve Nd under drizzling conditions. Recently, lidar-based Nd retrievals have been developed by synergizing multiple instruments in a similar way to the radar-based retrievals (Boers et al., 2006, Martucci and O'Dowd, 2011; Snider et al., 2017; Zhang et al., 2019) by using dual-field-of-view lidar extinction profiles (Schmidt et al., 2013) or by using lidar multiple scattering measurements (Donovan et al., 2015). Since lidar measurements are proportional to the second moment of cloud DSD, lidar-based Nd retrievals are more sensitive to Nd than radar-based methods and have the potential to provide more accurate retrievals.
In the past decade, there has been significant progress in developing Nd retrieval algorithms; however, the validation of these algorithms against in situ measurements is still inadequate. Most Nd retrieval methods were developed and tested under specific conditions, making it crucial to evaluate their performance against in situ measurements from different locations and cloud conditions to understand better their uncertainties and to confidently extend these algorithms. The Department of Energy (DOE) Atmospheric Radiation Measurement (ARM) Aerosol and Cloud Experiments in the Eastern North Atlantic (ACE-ENA) field campaign offers an excellent opportunity to validate different Nd retrieval algorithms under the same range of cloud conditions. The ACE-ENA campaign (Wang et al., 2022) collected comprehensive data sets from the ARM Eastern North Atlantic (ENA) site, where the ARM Aerial Facility (AAF) research aircraft made in situ measurements over the Azores where the ENA atmospheric observatory routinely makes measurements from state-of-the-art remote sensing instruments. The flights during the ACE-ENA campaign were designed to take full advantage of the synergy between aircraft in situ measurements and ARM ground-based remote sensing observations. In this study, four Nd retrievals are evaluated, considering their potential for operational applications and ease of use across different locations. These methods cover major ground-based Nd retrieval algorithms including two lidar-based retrievals similar to Snider et al. (2017), a radar-based retrieval similar to Wu et al. (2020a), and the Nd retrieval from the ARM Droplet Number Concentration (NDROP) value-added product (VAP) available at https://www.arm.gov/capabilities/vaps/ndrop (last access: 3 December 2023). We did not include lidar-based Nd retrievals that either utilize dual-field-of-view lidar extinction profiles or rely on depolarization measurements from lidar multiple scattering. This is due to the specific requirements of the dual-field-of-view lidar configuration and the substantial calibration efforts needed for lidar depolarization measurements. This study evaluates the Nd retrieval algorithms against in situ data to enhance our understanding of their uncertainties and extend their application to other locations.
The paper is organized as following: Sect. 2 presents a brief introduction of the ARM ENA site, the lidar- and radar-based retrieval algorithms, the ARM NDROP VAP, and the ACE-ENA field campaign measurements; Sect. 3 shows evaluations of Nd retrievals with in situ probe measurements during the ACE-ENA field campaign, as well as a 4-year climatology of overcast marine boundary layer (MBL) cloud Nd climatology based on retrievals at the ENA observatory; and Sect. 4 presents the summary and conclusions.
The lidar-based retrievals, radar-based retrieval, and the ARM NDROP VAP use different remote sensing measurements and algorithms to retrieve Nd. Brief descriptions of these methods are presented in Sect. 2.2–2.4. These retrieval methods use both passive and active remote sensing measurements. We expect the ensemble of these peer-reviewed retrievals for the same cloud to indicate a reasonable range of the retrieved Nd. We refined the lidar-based retrieval method discussed in Sect. 2.2. Then we evaluated assumptions in each retrieval method and, for the first time, compared four different Nd retrievals with in situ measurements to evaluate the robustness of their performances.
2.1 The ARM ENA atmospheric observatory
Established in October 2013, the ARM ENA atmospheric observatory is located on Graciosa Island in the Azores, Portugal, at 39∘5′29.76′′ N, 28∘1′32.52′′ W. This region of the northeastern Atlantic Ocean is characterized by the presence of marine stratocumulus clouds and is subject to diverse meteorological and aerosol conditions (Wood et al., 2015). Consequently, the ARM ENA site presents an ideal opportunity to study the properties of clouds and precipitation in a remote marine environment, as well as the response of low clouds to natural and anthropogenic aerosols and meteorological conditions. Facilitating these studies, the ARM ENA atmospheric observatory has been equipped with a large array of advanced instruments capable of providing high-spatial- and high-temporal-resolution measurements of the atmospheric state, aerosols, clouds, precipitation, and radiation budget. These instruments include a variety of aerosol instrumentation, lidars (Muradyan and Coulter, 2020; Newsom et al., 2022), radars (Johnson et al., 2022), radiometers (Cadeddu, 2021; Hodges and Michalsky, 2016), and the Balloon-Borne Sounding System (SONDE) (Holdridge, 2020). Table 1 lists the key ground-based instruments and their measurements which were used for Nd retrievals in this study.
2.2 Lidar-based Nd retrieval
In this study, Raman lidar (RL) and micropulse lidar (MPL) data are used in separate lidar-based retrievals. The method for retrieving Nd employs the interrelationships among Nd, βe, LWC, and cloud DSD, where βe is the extinction coefficient (Snider et al., 2017). At an altitude z above the cloud base, Nd, βe, and LWC can be expressed as functions of the cloud DSD:
where Qext is the extinction efficiency, r is the cloud droplet radius, nd,z is the droplet number concentration within the size range between r and r+dr, and ρw is the density of liquid water. Since water droplet sizes are much larger than the lidar laser wavelength, Qext≈2. The cloud droplet effective radius re,z is defined as
To establish a connection between the properties that are a function of the second and third moment of the cloud DSD, respectively βe,z and LWCz, previous research has made the assumption that the cloud DSD follows either a gamma distribution or a lognormal distribution and has a constant spectrum width (Martucci and O'Dowd, 2011; Snider et al., 2017). Drawing inspiration from the passive remote sensing retrieval algorithms outlined by McComiskey et al. (2009), an empirical parameter k is introduced to link βe,z and LWCz, which is a measure of the width of the cloud DSD. This parameter represents the cube of the ratio between the volume radius and the effective radius:
To determine Nd, the k parameter is assumed to remain constant vertically within the cloud (Brenguier et al., 2011). Through the analysis of aircraft in situ probe measurements from five distinct field experiments, Brenguier et al. (2011) demonstrated that the k parameter values range from 0.7–0.9, with uncertainties between 10 % and 14 % across different cloud systems and various atmospheric conditions. By integrating Eqs. (2), (3), and (5), Nd,z can be derived as a function of βe,z and LWCz:
The newly derived Eq. (6) eliminates the need for assuming a specific DSD shape (e.g., gamma or lognormal distribution), which was necessary in previous studies.
To derive Nd, the LWCz in stratiform clouds is typically assumed to be a constant fraction (fad) of its adiabatic value (LWCz,ad): . The LWCz,ad profile can be determined from cloud-base temperature and pressure measurements. By analyzing 2 years of ground-based remote sensing data from Leipzig, Germany, Merk et al. (2016) show that fad values are 0.63 ± 0.22. In this study, fad is calculated as the ratio of the retrieved LWP from the MWRRETv2 VAP (https://www.arm.gov/capabilities/science-data-products/vaps/mwrretv2, last access: 3 December 2023) to the LWP calculated from the adiabatic LWC profile. The MWRRETv2 VAP retrieves LWP from microwave radiometer brightness temperature measurements at 23.8, 31.4, and 90 GHz using the retrieval algorithm developed by Turner et al. (2007). The third channel at 90 GHz provides additional sensitivity to liquid water, enabling an LWP uncertainty of ±10–15 g m−2 (Cadeddu et al., 2013).
Advanced lidar systems, such as the RL and high-spectral-resolution lidar, are absolutely calibrated by referencing to molecular scattering. These systems offer reliable estimates of particulate backscatter and extinction coefficients by solving the lidar equation (Thorsen and Fu, 2015; Marais et al., 2016). Our RL retrieval uses the RL-estimated βe,z from the ARM Raman Lidar Profiles – Feature detection and Extinction (RLPROF-FEX) VAP (https://www.arm.gov/capabilities/science-data-products/vaps/rlprof-fex, last access: 3 December 2023), which computes βe,z using the algorithms developed by Thorsen et al. (2015) and Thorsen and Fu (2015). However, due to the weak strength of the Raman scattering compared to the elastic scattering, noise poses a considerable challenge for the extinction coefficient retrieval. To enhance the signal-to-noise (SNR) ratio, the fine-resolution RL data at 10 s temporal and 7.5 m vertical resolutions are aggregated coarser resolutions of 2 min and 30 m, respectively. While enhancing the SNR, this coarser-resolution RLPROF-FEX βe,z may introduce additional uncertainty in Nd retrievals for broken clouds. It is important to note that advanced lidar systems are more costly and, as a result, are not widely available.
In contrast, elastic-scattering lidars, such as the MPL and ceilometer, are available at all ARM observatories and numerous locations worldwide including the MPLNET and Cloudnet (Welton et al., 2001; Illingworth et al., 2007). These instruments provide high temporal and vertical measurements of the strong elastic scattering from atmospheric particles. However, elastic-scattering lidar measurements cannot be directly used to derive particulate backscatter and extinction coefficients since there is only one lidar equation (measurement) for these two variables, i.e., one equation with two unknowns. This issue is often addressed using the lidar extinction-to-backscatter ratio (S), which represents the relationship between particulate backscatter and extinction coefficients. Once S is determined, the lidar βe,z can be inverted from the MPL backscatter intensity measurements by analytically solving the lidar equation using the inversion method developed by Klett (1981) and Fernald (1984). For liquid cloud droplets, S is approximately 18.8 (O'Connor et al., 2004; Thorsen and Fu, 2015). To account for multiple scattering from liquid droplets, a multiple-scattering correction scheme developed by Hogan (2008) is applied. Sarna et al. (2021) demonstrated that, after all corrections to elastic-scattering lidar signals, the inversion method could obtain βe,z with an error of less than 5 % within 90 m above cloud base at the lidar wavelength of 355 nm. We assess the sensitivity and reliability of lidar-based Nd retrievals using both RL- and MPL-estimated βe,z.
It should be noted that Nd retrievals at cloud base (Zcb) are adversely impacted by noise introduced by turbulent mixing. Entrainment mixing may cause LWCcb to deviate significantly from the adiabatic value, resulting in considerable differences between the retrieved Nd,cb and Nd,z above the Zcb. Furthermore, lidar can only penetrate the low portion of the liquid cloud due to the strong attenuation by liquid droplets. The signal becomes fully attenuated when the optical depth reaches ∼ 3, which corresponds to 100 to 300 m above the Zcb. Consequently, our retrievals use βe,z and LWCz only within the range between Zcb + 30 m and Zcb + 90 m. For lightly drizzling maritime stratocumulus clouds, such as those with the column maximum radar reflectivity (Ze) < 0 dBZ, the contribution of drizzle particles to lidar extinction is negligible compared to that from liquid droplets; thus, the lidar-based retrievals can still be employed. Based on Eq. (4) and the assumptions that the cloud maintains a constant fraction of its adiabatic value and Nd remains vertically constant, re for the rest of the cloud layer can be estimated.
Using a similar lidar-based Nd retrieval approach, Snider et al. (2017) discovered that, in general, the lidar-based Nd retrievals were smaller than in situ probe measurements during the VAMOS Ocean–Cloud–Aerosol–Land Study Regional Experiment (VOCALS-REx) over the southeastern Pacific (Wood et al., 2011). It is worth noting that Snider et al. (2017) used the adiabatic LWC lapse rate without considering the subadiabaticity, which results in an overestimation of LWCz and consequently an underestimation of Nd based on Eq. (6). Therefore, in the present study, the bias of the Nd retrieval should not be as large since we consider cloud subadiabaticity.
2.3 Radar-based Nd retrieval
Obtaining Nd values from radar reflectivity poses challenges due to the frequent presence of drizzle within MBL clouds, which subsequently contributes significantly to the measured radar reflectivity (Zhu et al., 2022). Wu et al. (2020a) recently developed a method to separate drizzle and cloud droplet contributions to the measured radar reflectivity while simultaneously retrieving cloud and drizzle microphysical properties, including Nd, in precipitating MBL clouds. They distinguish between drizzle and cloud droplet contributions by identifying the height where Ze exceeds −15 dBZ when moving downward from the cloud top. This height marks the initiation of drizzle where, above this point, the measured Ze is solely attributed to cloud droplets. While it is convenient to use this threshold, it should be noted that a number of recent studies demonstrate that drizzle having significantly lower reflectivity than −15 dBZ can be observed within stratocumulus clouds (Kollias et al., 2011; Luke and Kollias, 2013; Zhu et al., 2022). The cloud contribution to Ze at the cloud base is calculated as the difference in Ze from the radar range gates above and below cloud base. Subsequently, they construct the cloud radar reflectivity (Zc) by assuming a linear increase in cloud liquid water content (LWCc) with height above cloud base (and thus a linear increase in if Nd is invariant with height). By assuming that the cloud droplet particle size distribution follows a lognormal distribution with a logarithmic width of σx, the relationship between Nd, LWCc, and Zc can be expressed as
The logarithmic width σx is set to 0.38 from Miles et al. (2000). However, under the assumption of a lognormal DSD, a value of 0.38 for σx is equivalent to a k value of 0.65. Martin et al. (1994) showed that k ranges from 0.67 ± 0.07 in continental air masses to 0.80 ± 0.07 in the marine ones. Consequently, we adopt σx of 0.23, which equates to a k value of 0.86 under a lognormal DSD condition. This is in line with the k value utilized in lidar-based retrievals and the NDROP VAP. ρw is the liquid water density. To determine Nd, Eq. (7) is further constrained by the cloud LWP, derived from the difference between the MWRRETv2 (total) LWP and the calculated drizzle water path, which is obtained from the retrieved drizzle water content profile. Subsequently, the re profile is derived from Nd and the LWCc profile.
To mitigate the impact of MWRRETv2 LWP uncertainties, cloud microphysical property retrievals were smoothed to a temporal resolution of 1 min. A sensitivity analysis conducted by Wu et al. (2020a) revealed that the retrieved Nd values are not sensitive to the selection of the radar reflectivity threshold of −15 dBZ. Using aircraft measurements from the ACE-ENA field campaign as a benchmark, the median Nd retrieval error is approximately ∼ 35 %.
2.4 The ARM NDROP VAP
The Nd retrieval method employed by the ARM NDROP VAP uses the relationship between LWP, cloud optical depth (τ), cloud DSD, and Nd. Following Lim et al. (2016), the layer-mean Nd can be expressed as
where k∗ is the cloud system k parameter, which is the cube of the ratio between the layer-mean volume radius and the layer-mean effective radius. As both τ and LWP represent vertical integrals through the entire cloud layer, Brenguier et al. (2011) propose using the cloud system k∗ parameter in Eq. (8). Consequently, the NDROP VAP retrievals utilize the cloud system k∗ parameter, while other methods deploy the local mean k parameter. In the case of a linearly stratified cloud with constant k within the cloud, k∗ can be derived as . The NDROP VAP adopts a k∗ value of 0.74, as recommended by Brenguier et al. (2011; Riihimaki et al., 2021).
The adiabatic LWC lapse rate, cw, can be calculated using cloud-base temperature and pressure from the ARM INTERPSONDE VAP (https://www.arm.gov/capabilities/vaps/interpsonde, last access: 3 December 2023) (Fairless et al., 2021), and τ is available from the ARM Cloud Optical Properties from the Multifilter Shadowband Radiometer (MFRSRCLDOD) VAP (https://www.arm.gov/capabilities/vaps/mfrsrcldod, last access: 3 December 2023), which retrieves τ for overcast liquid clouds from Multifilter Rotating Shadowband Radiometer (MFRSR) measurements using the retrieval algorithm developed by Min and Harrison (1996; Turner et al., 2014). The MFRSR measures both global and diffuse components of solar irradiance at multiple narrowband channels with a hemispheric viewing geometry. The retrieval algorithm employs the transmitted irradiance at 415 nm from the MFRSR, so the retrieved τ is available only during daytime. The retrieval assumes a single cloud layer comprised of liquid water drops and assumes the surface is not covered with snow or ice. Analyses show that τ from the MFRSRCLDOD VAP has uncertainties ranging from 0.5 to 2.5 (Turner et al., 2014). LWP is available from the ARM MWRRETv2 VAP as mentioned in Sect. 2.2. The MWR3C has a field of view of between 5 and 6∘. Since both the τ and LWP retrievals have significant relative uncertainties for optically thin clouds, this retrieval approach should be applied for overcast, optically thick liquid clouds.
Lim et al. (2016) evaluated Nd values retrieved using this approach by comparing them to aircraft in situ probe measurements obtained during the Routine ARM Aerial Facility (AAF) Clouds with Low Optical Water Depths (CLOWD) Optical Radiative Observations (RACORO) field campaign at the ARM Southern Great Plains (SGP) site (Vogelmann et al., 2012). Their findings indicate that the retrieved Nd values are substantially larger than the in situ measurements. This discrepancy may be attributed to the fact that clouds sampled during the RACORO campaign often exhibited small LWPs. Consequently, NDROP retrievals still require evaluation under optically thick cloud conditions.
For passive remote sensing retrievals, the layer-mean re (rem) between the cloud layer top and base can be determined using the relationship among rem, τ, and LWP:
and rem is available from the ARM MFRSRCLDOD VAP (https://www.arm.gov/capabilities/science-data-products/vaps/mfrsrcldod, last access: 3 December 2023). To compare re retrievals with those from different approaches and with in situ measurements, we use rem for comparisons for the rest of the discussion following previous studies (Chiu et al., 2012; Grosvenor et al., 2018); rem is derived by averaging re at each layer between the cloud top and base from lidar- and radar-based retrievals and in situ measurements.
2.5 ACE-ENA in situ measurements
The ACE-ENA field campaign deployed the ARM AAF Gulfstream-159 (G-1) research aircraft over the Azores during the two intensive operational periods (IOPs) in early summer 2017 (June to July) and winter 2018 (January to February). The G-1 was equipped with a range of in situ sensors, enabling comprehensive measurements of aerosol particles, cloud droplets, precipitation, and atmospheric conditions. Cloud probes particularly relevant to Nd measurements include the fast cloud droplet probe (FCDP) and the cloud and aerosol spectrometer (CAS). The FCDP measures cloud droplets in the diameter size range of 1.5–50 µm with a temporal resolution of 1 or 0.1 s. The CAS provides measurements of aerosol or cloud droplets in the 0.5–50 µm diameter size range with a temporal resolution of 1 s. Given the different particle size ranges measured by the various probes, we used in situ Nd data for the particle size between 3–50 µm. It is noted that although in situ probes provide reliable Nd measurements, they also have uncertainties ranging from 10 %–30 % as presented by Baumgardner et al. (2017). Therefore, we include both FCDP and CAS measurements for evaluating Nd retrievals.
During the ACE-ENA campaign, the G-1 aircraft conducted both vertical profiling flights and horizontal flights at physically important levels, such as near the ocean surface, just below clouds, within clouds, and at and above the cloud top (Wang et al., 2022). These flights were specifically designed to maximize synergy between G-1 aircraft measurements and ENA ground-based remote sensing observations, offering an ideal dataset for evaluating Nd retrievals. Most G-1 flights employed an L-shaped pattern, including both upwind and crosswind legs at different altitudes, with the L “corner” over the ENA site. Additionally, four G-1 flights used a “Lagrangian drift” pattern, starting upwind of the ENA site and performing crosswind measurements while drifting with the prevailing boundary layer winds (Wang et al., 2022). In total, 39 flights were conducted during the two IOPs.
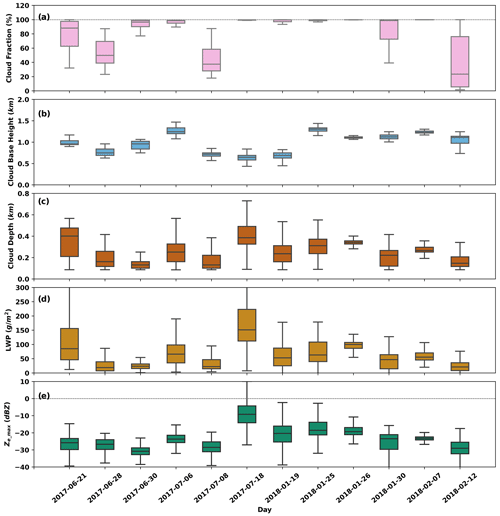
Figure 1Cloud properties of the 12 selected flight days derived from the ENA ground-based remote sensing observations during aircraft measurement periods: (a) fractional sky cover obtained from total sky imager (TSI) observations; (b) cloud-base height determined from MPL measurements; (c) cloud depth; (d) LWP obtained from the MWRRETv2 VAP; and (e) column maximum Ze (Ze_max) from KAZR measurements. The box-and-whisker plots display the 5th, 25th, 50th, 75th, and 95th percentiles. Dashed lines in (a) and (e) represent 100 % cloud fraction and Ze_max of 0 dBZ, respectively.
Among those flights, 12 flight days featuring multiple in-cloud flight legs under single-layer stratiform cloud conditions were selected for this study. Each selected day had at least one complete traversal from the cloud base to the cloud top. Heavily drizzling stratocumulus flight days were excluded. Table 2 provides the date, in-cloud flight time, and cloud conditions for the 12 flight days. In-cloud measurements are defined as those when the FCDP-measured Nd values are larger than 10 cm−3. Approximately 11 total hours of in-cloud flight measurements were used to evaluate the Nd retrievals. Figure 1 illustrates cloud properties of the 12 selected flight days, including fractional sky cover, cloud-base height, cloud depth, LWP, and column maximum Ze (Ze_max) derived from the ENA ground-based remote sensing observations. The box-and-whisker plots display the 5th, 25th, 50th, 75th, and 95th percentiles. Of the 12 selected flight days, 9 have overcast cloud conditions and 3 have broken-cloud conditions (28 June 2017, 8 July 2017, 12 February 2018). These 3 broken-cloud days had among the smallest LWPs, as shown in Fig. 1d. Cloud-base heights ranged from 0.5 to 1.5 km with variations often smaller than 0.3 km on a given flight day. Overall, these clouds had LWPs less than 200 g m−2 and Ze_max values smaller than 0 dBZ, which are typical of marine low-level clouds.
3.1 Evaluation of retrieval assumptions
In the lidar-based, radar-based, and NDROP VAP Nd retrievals, several assumptions are made regarding the vertical Nd variation, the k and k∗ parameters, and the LWC profile, as described in Sect. 2.2 and 2.3. We test these assumptions in this section. Figure 2 presents statistics of these cloud properties from in situ and ground-based measurements during the 12 selected flight days. For example, Fig. 2a shows that the mean Nd normalized by the flight average is close to 1, with standard deviations of approximately 0.4 through the cloud layer, which supports the assumption that Nd can be treated as constant within the cloud layer.
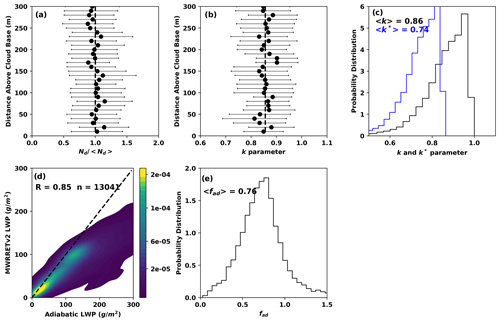
Figure 2Statistics of cloud properties used in the retrieval algorithms from in situ and ground-based measurements during the 12 selected flight days: (a) the mean and standard deviation of the FCDP-measured Nd normalized by the flight average; (b) the mean and standard deviation of the derived k parameter profile within clouds; (c) the PDFs of the k and k∗ parameters; (d) the regression between LWPs from MWRRETv2 retrievals and those calculated assuming an adiabatic cloud, where R is the Pearson correlation coefficient and n the total number of profiles; and (e) PDF of fad.
The k parameter is assumed to be vertically constant. Some previous studies find that the k parameter increases with height (Brenguier et al., 2011), while others suggest that the k parameter can either increase or decrease with height (Pawlowska et al., 2006; Painemal and Zuidema, 2010). Our analysis shows that the mean k parameter remains essentially constant with height (Fig. 2b). The probability distribution functions (PDFs) of the k and k∗ parameters (Fig. 2c) reveal that k (k∗) ranges between 0.6 (0.5) and 1.0 (0.86), with a mean value of 0.86 (0.74) and a standard deviation of 0.10 (0.09). Since Nd is inversely proportional to k (k∗) as shown in Eqs. (6) and (8), an uncertainty of 0.10 in the k value alone could cause an uncertainty of ∼ 12 % in the retrieved Nd value from the uncertainty propagation analysis. The lidar-based Nd retrievals in this study use a k value of 0.86. We note that the k∗ value of 0.74 used in the NDROP VAP is well justified. As the k value of 0.86 corresponds exactly to the recommended k∗ value of 0.74 by Brenguier et al. (2011), where their value is based on data from five field program locations, it suggests that a k value of 0.86 might be more broadly applicable for lidar-based Nd retrievals of boundary layer clouds at other locations.
As aircraft in situ probes are unable to provide continuous cloud-base height measurements and the LWCad or cw profile is sensitive to cloud-base height, it is challenging to determine fad and its vertical variations within a cloud. Instead, we use the ratio of the MWRRETv2 LWP to the computed adiabatic LWP to calculate fad. As seen in Fig. 2d, the LWP from MWRRETv2 and the adiabatic LWP show a strong correlation, evidenced by a Pearson correlation coefficient of 0.85. Adiabatic LWPs are generally larger than MWRRETv2 LWPs, especially when the LWP is above 150 g m−2, but they correlate well. The PDF of fad shows that fad has a mean value of 0.76, with a standard deviation of 0.42 (Fig. 2e). Since cloud LWP should not exceed the adiabatic LWP, fad is set to 1 when it is larger than 1. Those values, and possibly those at the extreme lower end of fad, appear to be affected primarily by uncertainties in cloud thickness for thin clouds (< 200 m) and by uncertainties in low MWRRETv2 LWPs (< 75 g m−2), based on scatter plots of fad vs. these respective properties (not shown). On the other end, when the LWP is above ∼ 150 g m−2, the cloud could contain drizzle. The current MWRRETv2 retrieval does not account for drizzle scattering effects at frequencies above 90 GHz, which could cause overestimation of the LWP by 10 %–15 %, as outlined in the study by Cadeddu et al. (2020). We did not implement corrections to this bias due to two reasons: firstly, there are currently no reliable methods to correct such bias; secondly, we removed strong drizzling stratocumulus cases by excluding clouds with Ze_max larger than 0 dBZ, as discussed in Sect. 2.
3.2 Evaluation of Nd retrievals
For convenience, we label Nd(rem) retrievals from the MPL, RL, and KAZR radar measurements and from the NDROP (MFRSRCLDOD) VAP as Nd_mpl(rem_mpl), Nd_rl(rem_rl), Nd_radar(rem_radar), and Nd_vap(rem_vap), respectively, and in situ measured Nd(rem) from FCDP and CAS as Nd_FCDP(rem_FCDP), and Nd_cas(rem_CAS). Figure 3 shows an example of ground-based remote sensing measurements and Nd and re retrievals on 26 January 2018. The cloud is a typical stratiform MBL cloud with a cloud-base height of ∼ 1.1 km, and a cloud-top height of ∼ 1.5 km. The cloud system persisted for more than 55 h from ∼ 05:00 UTC on 25 January to ∼ 12:00 UTC on 27 January (full period not shown in Fig. 3). From the mean sea level pressure distribution (Fig. S1 in the Supplement), the Azores high was located to the northeast of the Azores. Near-surface winds were south to southeast across the ENA observatory. The synoptic environment created a strong stable boundary layer condition that was favorable for the maintenance of marine boundary layer stratocumulus. From Fig. 3a and b, large βe and its rapid attenuation indicate the presence of the liquid layer. Figure 3c shows radar reflectivity up to −20 dBZ below the liquid layer, indicating that drizzle frequently forms and falls out of the liquid layer. The mean MWRRETv2 LWP (LWPmwr) and calculated adiabatic cloud LWP (LWPad) are 107 and 119 g m−2, respectively. Figure 3d shows that LWPad and LWPmwr correlate very well and are close in magnitude, indicating the cloud is nearly adiabatic. Retrieved Nd_mpl, Nd_rl, Nd_radar, and Nd_vap are shown in Fig. 3e. For this case, Nd_mpl and Nd_radar have a similar magnitude at ∼ 50 cm−3 but are smaller than Nd_rl and Nd_vap. Derived rem_mpl, rem_rl, rem_radar, and rem_vap are very close at ∼ 10.5 µm, as shown in Fig. 3f.
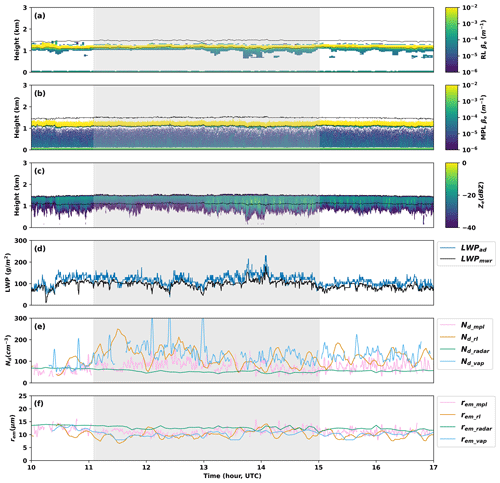
Figure 3An example of ground-based remote sensing measurements and Nd retrievals on 26 January 2018: (a) RL extinction coefficient (βe) profiles from the RLPROF-FEX VAP; (b) MPL βe profiles; (c) KAZR radar reflectivity profiles; (d) LWPs from MWRRETv2 retrievals (LWPmwr) calculated assuming an adiabatic cloud liquid water content vertical profile (LWPad); (e) retrieved Nd_mpl, Nd_rl, Nd_radar, and Nd_vap; and (f) derived layer-mean re (rem) per retrieval (rem_mpl, rem_rl, rem_radar, rem_vap). Black lines in (a), (b), and (c) are cloud top and base detected with combined lidar and radar measurements. The gray zone indicates the time of concurrent aircraft in situ measurements.
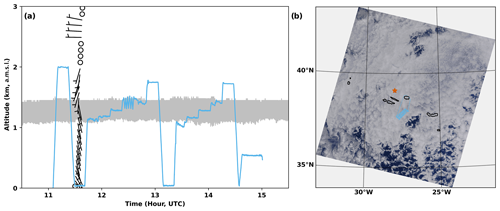
Figure 4(a) The G-1 aircraft flight track on 26 January 2018. The gray zone represents the cloud layer. Wind barbs are from the ARM radiosonde measurements at 11:30 UTC at the ENA observatory. On the y axis, a.m.s.l. refers to above mean sea level. (b) Moderate Resolution Imaging Spectroradiometer (MODIS) true color image of clouds between 13:00–13:10 UTC on 26 January 2018. The red star indicates the location of the ENA observatory. The black circular regions represent islands. The blue lines in (a) and (b) represent the aircraft flight track.
This case is one of the four “Lagrangian drift” flights during the entire ACE-ENA field campaign. The prevailing boundary layer winds were south- to southeastward. Boundary layer wind speeds were generally less than 10 m s−1, based on radiosonde measurements at 11:30 UTC at the ENA observatory (Fig. 4a). The G-1 aircraft took off at approximately 11:05 UTC upwind of the ENA observatory and landed at around 15:00 UTC (Fig. 4). During the 4 h flight, the G-1 aircraft made about 1 h and 37 min of in-cloud measurements, including several horizontal legs just below cloud top, within the cloud layer, and just above cloud base, as well as several spirals. Satellite imagery from the Moderate Resolution Imaging Spectroradiometer (MODIS) shows that closed-cellular stratocumulus clouds are dominant in the region (Fig. 4b).
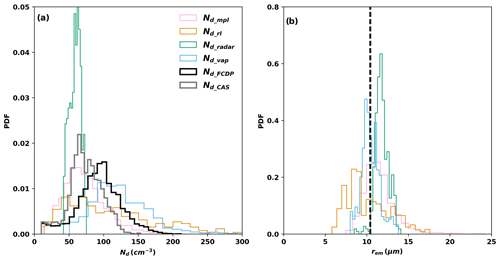
Figure 5Evaluation of ground-based retrievals of Nd and re with aircraft in situ measurements for the case on 26 January 2018. (a) PDFs of Nd_mpl, Nd_rl, Nd_radar, Nd_vap, Nd_FCDP, and Nd_CAS during the time of concurrent aircraft measurements; (b) PDFs of rem_mpl, rem_rl, rem_radar, rem_vap, and derived rem_FCDP and rem_CAS from in situ probe measurements. The colors of rem lines in (b) correspond to those given in (a). Dashed lines in (b) are mean re from FCDP and CAS measurements during all in-cloud penetrations.
Due to the continuous movement of the G-1 aircraft near the ENA observatory, establishing direct one-to-one comparisons between ground-based retrievals and aircraft in situ measurements is challenging. Instead, we evaluate the PDFs of Nd retrievals against those from the aircraft in situ measurements. Figure 5a shows the comparison of ground-based Nd retrievals Nd_mpl, Nd_rl, Nd_radar, and Nd_vap during the time of the concurrent aircraft flight against in situ FCDP (Nd_FCDP) and CAS (Nd_CAS) measurements for the case on 26 January 2018. It should be noted that measurements from the two in situ probes show slightly different Nd distributions. Nd_CAS is generally less than Nd_FCDP with a median of 74 cm−3 and a narrower distribution with a standard deviation of 24 cm−3, while Nd_FCDP has a median of 97 cm−3 and a standard deviation of 34 cm−3. Among the four Nd retrievals, Nd_mpl shows a very similar distribution to Nd_cas, with a median of 73 cm−3 and a standard deviation of 31 cm−3. Nd_rl shows a broader distribution with a median of 114 cm−3 and a standard deviation of 71 cm−3, probably because the retrieved RL βe has a larger random noise than that of MPL βe. Nd_radar has the narrowest distribution, with a median of 62 cm−3 and a standard deviation of 13 cm−3. The Nd_vap retrieval exhibits the highest values, with a median of 127 cm−3 and a standard deviation of 46 cm−3.
As re changes with distance above cloud base and it is challenging to know instantaneous cloud-base height from aircraft measurements, it is more difficult to conduct one-to-one comparisons between ground-based re retrievals and aircraft in situ measurements. Therefore, we compare PDFs of the rem retrievals against aircraft in situ measurements during all in-cloud penetrations. Figure 5b shows that the median re_FCDP and re_CAS are almost the same, around 10.4 µm. The medians (standard deviations) of rem_mpl, rem_rl, rem_radar, and re_vap are 11.5 µm (1.9 µm), 10.2 µm (2.6 µm), 9.3 µm (0.7 µm), and 10.5 µm (1.1 µm), respectively. Although median rem values from different retrieval methods are very close, rem_mpl and rem_rl have broader distributions than rem_radar and re_vap.
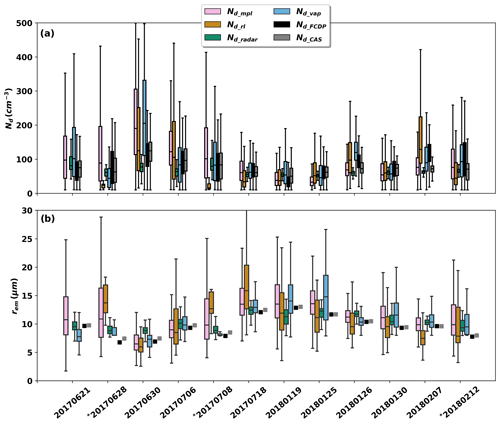
Figure 6Evaluation of ground-based retrievals of Nd and re with aircraft in situ measurements for the 12 selected flight days. (a) Boxplots of Nd_mpl, Nd_rl, Nd_radar, Nd_vap, Nd_FCDP, and Nd_CAS during the time of concurrent aircraft measurements; (b) boxplots of rem_mpl, rem_rl, rem_radar, re_vap, and derived rem_FCDP and rem_CAS from in situ probe measurements. The colors of rem boxplots in (b) correspond to those given in (a). The ∗ indicates broken-cloud conditions.
Table 3Median Nd values for the 12 selected flight days. Nd_rl values were not available (labeled as “NA”) on 21 June 2017 because Raman lidar data were missing. The percentages in parentheses represent the relative difference in the Nd retrievals compared to Nd_FCDP.
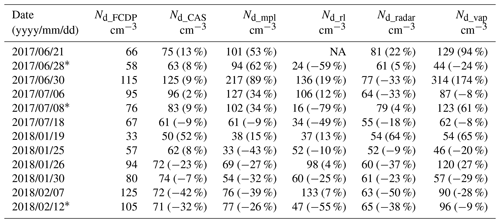
The ∗ indicates broken-cloud conditions.
Figure 6 presents the comparison of ground-based Nd and rem retrievals against in situ FCDP and CAS measurements for the 12 selected flight days. Table 3 displays the median Nd of the 12 selected flight days and their relative differences with respect to Nd_FCDP. In accordance with prior studies of cloud microphysical properties (Yeom et al., 2021; Zhang et al., 2021), we consider FCDP measurements as the benchmark. The median Nd_FCDP for the 12 d ranges from 33 to 125 cm−3. There are substantial variations in Nd from in situ measurements among the 12 d, with generally higher Nd observed on summer IOP days and lower Nd on winter IOP days. This agrees with the analysis in Wang et al. (2022) of all in situ Nd measurements during the ACE-ENA field campaign, which reveals that the flight-mean Nd ranges from 20–50 cm−3 and that summer IOP Nd is generally larger than that of the winter IOP. Encouragingly, ground-based Nd retrievals generally follow the same seasonal variation trend as shown in Fig. 6a.
Between the two in situ probe measurements, Nd_FCDP and Nd_CAS show good agreement. The median Nd relative differences in Nd_CAS with respect to Nd_FCDP are smaller than 10 % for most flights (Table 3). However, significant differences are observed for several flights, such as on 19 January and 7 February 2018, when the median Nd relative differences are larger than 40 %. Nd_mpl compares well with in situ probe measurements, with the median Nd relative differences in Nd_mpl with respect to Nd_FCDP ranging from 9 % to 89 %. Interestingly, Fig. 6a reveals that Nd_mpl overestimates Nd during the summer IOP but underestimates Nd during the winter IOP, partially because the k parameter values were smaller (larger) during the summer (winter) IOP than the default value of 0.86 used in the retrieval algorithms (Fig. S2). Nd_rl compares well with in situ probe measurements for overcast clouds but significantly underestimates Nd for broken clouds (28 June 2017, 8 July 2017, and 12 February 2018), which is likely due to the coarse temporal resolution of RLPROF-FEX extinction data. Similar to the 26 January 2018 case, Nd_radar values for other flight days consistently have a narrower range and are generally smaller than in situ probe measurements. Nd_vap considerably overestimates Nd for either broken clouds or when clouds have low LWPs, such as on 21 June, 28 June, and 8 July 2017. For overcast clouds with LWPs greater than ∼ 25 g m−2, Nd_vap compares well with in situ probe measurements. Overall, retrieved Nd values have a larger spread and poorer comparison with in situ probe measurements during the summer IOP than those of the winter IOP, likely because more broken low-level clouds are present during summer at the ENA observatory (Fig. 1a).
Figure 6b reveals significant differences in re between the two IOPs, with smaller re values during the summer IOP and larger re values during the winter IOP. This is in line with the differences in Ze_max between two IOPs as shown in Fig. 1d since Ze_max is highly sensitive to the presence of large particles. In situ probe-derived re values are very close to each other, with differences between rem_FCDP and rem_CAS being less than 1 µm for all the 12 selected flight days (Table S1). Retrieved rem_mpl, rem_rl, rem_radar, and rem_vap all correspond well with the rem_FCDP variations. The rem_mpl values are slightly larger than rem_FCDP, with absolute differences usually within 2 µm. This is likely because rem_mpl is calculated assuming a constant subadiabatic LWC profile, leading to a linear increase in re from cloud base to cloud top. In reality, cloud re increases above cloud base but decreases slightly at cloud top due to entrainment mixing of dry air (Wang et al., 2022). The rem_rl values compare well with rem_FCDP for most cases but are significantly larger than rem_FCDP for flight days when the retrieved Nd_rl values are considerably smaller than Nd_FCDP due to broken clouds and the coarse temporal resolution of the RL extinction data. The re_radar values are also within 2 µm of re_FCDP, which can be either larger or smaller. The values of re_vap are also slightly greater than those of re_FCDP in general. This is primarily because re_vap is calculated from measured LWP and τ, both of which are more heavily influenced by the cloud's upper regions where larger droplet particles are prevalent.
3.3 Implementing Nd retrievals to multiple years of ENA data
A significant advantage of ground-based Nd retrievals is their applicability to long-term, continuous, and high-temporal-resolution remote sensing measurements, facilitating process-level understanding of cloud microphysical properties and their climatology. The Nd retrievals are applied to 4 years of ground-based remote sensing measurements of overcast MBL clouds at the ENA observatory between 2016 and 2019. MBL clouds are identified as those with base heights lower than 4 km above sea level (a.s.l.). Considering the limitation of RL and NDROP retrievals, we selected single-layer overcast MBL cloud systems that persist longer than 20 min with a concurrent total sky imager (TSI) fractional sky cover greater than 95 % and an LWP greater than 25 g m−2. To avoid heavily precipitating cloud systems, we excluded clouds with Ze_max larger than 0 dBZ. Since the RL data and retrievals have the coarsest temporal resolution of 2 min, other retrievals were subsampled to the same temporal resolution as RL data. In total, approximately 245 000 retrieved Nd and re data samples were collected.
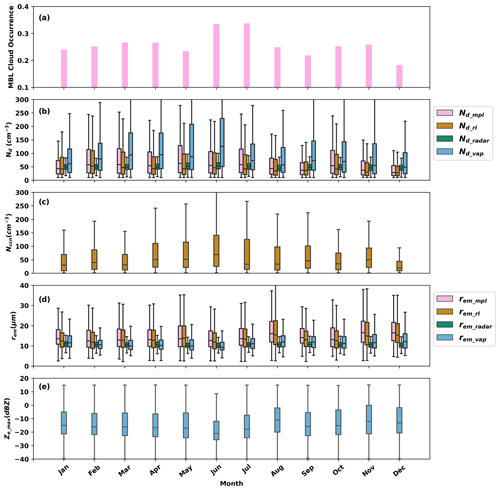
Figure 7Monthly variations in overcast marine boundary layer cloud Nd and re using 4 years of ground-based measurements at the ENA observatory. (a) Occurrence of MBL clouds; (b) retrieved Nd_mpl, Nd_rl, Nd_radar, and Nd_vap; (c) cloud condensation nuclei (Nccn) from the ARM CCN counter (CCN-100) of the aerosol observing system (AOS) at a supersaturation of 0.1 %; (d) derived rem_mpl, rem_rl, rem_radar, and rem_vap; and (e) Ze_max.
Figure 7a displays the monthly occurrence of overcast MBL clouds at the ENA observatory meeting the above-stated criteria. The annual mean occurrence of these clouds is approximately 0.26 with higher monthly mean occurrences in June and July and a lower occurrence during December. The mean MBL cloud occurrence and its seasonal variations align closely with those of the low-level cloud presented in Wu et al. (2020b), who used a similar dataset to study MBL cloud and drizzle properties at the ENA observatory but for cloud-top height below 3 km. Monthly Nd statistics are shown in Fig. 7b. The annual median Nd_mpl, Nd_rl, Nd_radar, and Nd_vap are approximately 79.7, 75.9, 54.4, and 116.9 cm−3, respectively. As with the evaluations for the ACE-ENA field campaign, Nd_vap values at the ENA observatory are consistently larger than other retrievals. Lim et al. (2016) suggested that unrealistically high Nd_vap values over 2000 cm−3 generally occur when LWP is low. By limiting retrievals to only MBL systems with LWP greater than 25 g m−2, we do not find Nd_vap larger than 500 cm−3. However, the systematically larger Nd_vap compared to other retrievals indicates that cloud optical depth retrievals might also be biased by off-zenith clouds, which are not considered in the cloud optical depth retrievals. Nd_mpl and Nd_rl are generally very close to each other, suggesting that cloud droplet particulate extinction inversion using either the Fernald method or RL data is reasonably reliable. Nd_radar compares well with lidar-based retrievals and has the narrowest distributions each month and the smallest monthly variations. All retrievals show slightly seasonal Nd variations with higher Nd during the summer season and lower Nd during the winter season, consistent with the Nd differences between the summer IOP and winter IOP during the ACE-ENA field campaign, as discussed in Sect. 3.2. Wang et al. (2022) suggested that Nd is positively correlated to the boundary layer accumulation mode aerosol concentration, but the ratio of summer to winter Nd is smaller than the seasonal variations in accumulation mode aerosol concentration. Figure 7c shows the monthly distributions of cloud condensation nuclei (Nccn) at the supersaturation of 0.1 % from the ARM CCN counter (CCN-100) of the surface aerosol observing system (AOS). Nccn has similar seasonal variations to Nd with larger values in June and July and smaller values in December, but its seasonal variations are much larger than those of Nd, consistent with the findings of Wang et al. (2022).
Figure 7d presents the monthly distributions of retrieved rem values. The annual median rem_mpl, rem_rl, rem_radar, and rem_vap are 14.5, 13.8, 10.4, and 11.7 µm, respectively. Both rem_mpl and rem_rl are slightly larger than rem_radar and rem_vap due to the assumption of a constant subadiabatic LWC profile when calculating rem_mpl and rem_rl, as discussed in Sect. 3.2. Also note that the Wu et al. (2020a) method retrieves cloud and drizzle drop size separately and that rem_radar is the effective radius solely for cloud droplets and does not account for drizzle particle size. Thus, the smaller rem_radar with respect to other retrievals is expected. While rem_radar and rem_vap do not exhibit significant monthly variations, rem_mpl and rem_rl are slightly smaller in June and July and slightly larger in November and December, displaying an opposite seasonal variation pattern compared to that of Nd_mpl and Nd_rl in Fig. 7b. Figure 7e illustrates the monthly statistics of Ze_max, which shares a similar seasonal variation pattern with rem_mpl and rem_rl in Fig. 7d, reinforcing the observed rem_mpl and rem_rl seasonal variation pattern.
Remote sensing techniques offer extensive cloud properties for studying ACI processes and validating climate model simulations. Validating Nd retrieval algorithms against in situ probe measurements is needed to understand their uncertainties. The ARM ACE-ENA field campaign offers a unique opportunity to validate four different Nd ground-based retrieval algorithms, which use ENA atmospheric observatory data, against G-1 research aircraft observations, which were made over the Azores during intensive IOPs in early summer 2017 and winter 2018. A total of 12 flight days under single-layer stratiform low-level cloud conditions were selected, with 6 d in the summer IOP and 6 d in the winter IOP. Approximately 11 total hours of in-cloud flight measurements were used to evaluate Nd retrievals.
Several assumptions used in the retrieval algorithms were assessed or characterized.
-
Cloud DSD shape. For the lidar-based Nd retrieval, we demonstrate in Eq. (6) that using the k parameter can eliminate the need to assume a shape of the cloud DSD (e.g., gamma or lognormal distribution). The k parameter is the cube of the ratio of the volume radius to the effective radius (re) of the cloud droplets, representing the width of cloud DSD.
-
Constant Nd with height. Aircraft in situ measurements confirm that Nd can be treated as constant through the cloud layer for stratiform MBL clouds, with the mean k parameter remaining constant with height. The k value ranges between 0.6–1.0 with a mean of 0.86, which is very close to k values at other geographic locations (Brenguier et al., 2011).
-
Treating subadiabatic LWC. The ratio of the retrieved LWP from the MWRRETv2 VAP divided by the LWP calculated from the adiabatic LWC profile is used to estimate the subadiabaticity fraction, fad. The mean value of fad is 0.76 at the ENA observatory during the ACE-ENA campaign period.
Retrieved Nd (Nd_mpl, Nd_rl, Nd_radar, and Nd_vap) and cloud layer-mean re (rem_mpl, rem_rl, rem_radar, and rem_vap) are evaluated against aircraft in situ probe measurements of Nd (Nd_FCDP, Nd_CAS) and rem (rem_FCDP, rem_CAS). To manage the challenge of direct one-to-one comparisons between ground-based retrievals and aircraft in situ measurements, we compare the PDFs of the retrievals with aircraft measurements. Analyses of the in situ measurements and retrievals for the 12 flight days reveal the following.
-
There is good agreement in the Nd in situ probe measurements, Nd_FCDP and Nd_CAS, with the relative differences in the median Nd often being smaller than 10 % for most flights (albeit with larger differences in some cases).
-
Ground-based Nd retrievals generally follow the same day-to-day variation in the in situ measurements.
-
The assessment of the Nd retrievals with the in situ measurements reveals the following:
- a.
Nd_mpl compares well overall with the aircraft measurements, but it overestimates Nd during the summer IOP and underestimates it during the winter IOP.
- b.
Nd_rl compares well for overcast clouds but underestimates Nd for broken clouds.
- c.
Nd_radar values are consistently smaller and have a narrower range than in situ measurements.
- d.
Nd_vap overestimates Nd for broken clouds or clouds with low LWPs.
- a.
-
There is good agreement in the rem in situ probe measurements, rem_FCDP and rem_CAS. The evaluations of rem show that the retrievals follow the variations of rem_FCDP. There is a tendency for rem_mpl to be slightly larger than rem_FCDP.
These retrieval algorithms are further applied to 4 years of continuous ground-based remote sensing measurements of overcast MBL clouds at the ENA observatory. Monthly statistics of Nd (rem) show slightly seasonal variations with a tendency towards higher (lower) values during the summer season and lower (higher) values during the winter season. Nd_mpl and Nd_rl are generally very close to each other. Nd_vap is found to be systematically larger than other retrievals, which might arise from the dissimilar fields of view (FOVs) for the cloud optical depth and LWP retrievals, where the former is a hemispheric FOV, while the latter is a zenith radiance. Nd_radar compares well with lidar-based retrievals and has the narrowest distributions each month with the smallest monthly variations. Both rem_mpl and rem_rl are found to be slightly larger than rem_radar and rem_vap.
Nd retrievals evaluated in this study used various remote sensing measurements and employed different retrieval algorithms. Consequently, the ensemble of these retrievals for the same cloud can help us to quantify Nd retrieval uncertainties and identify reliable retrievals, such as when the ensemble of all retrievals has a narrow range (Zhao et al., 2012). Out of the four retrieval methods, we recommend using the MPL lidar-based method because it has a good agreement with in situ measurements, it has less sensitivity to issues arising from precipitation and low cloud LWP and/or optical depth, and it has broad applicability by functioning under both daytime and nighttime conditions. Ground-based Nd retrievals can be used to enhance our understanding of local cloud microphysical processes and can provide long-term verification of spaceborne Nd retrievals that can provide a global dataset needed for validating and improving global climate model simulations of clouds (Bennartz and Rausch, 2017)
The ARM NDROP VAP data used in this study can be downloaded from the ARM data archive site: https://doi.org/10.5439/1131339 (Riihimaki et al., 2023). Nd_mpl and Nd_rl data can be downloaded at https://adc.arm.gov/discovery/#/results/s::droplet%20number%20concentration (Zhang, 2023). Nd_radar data are available upon request. Nd_CAS and Nd_FCDP data can be downloaded from the ARM IOP data webpage: https://doi.org/10.5439/1438488 (Cromwell et al., 2023) and https://doi.org/10.5439/1417472 (Mei et al., 2023).
The supplement related to this article is available online at: https://doi.org/10.5194/amt-16-5827-2023-supplement.
Conceptualization: DZ and AMV; methodology: DZ, ZW, PW, AMV; software: DZ; validation: DZ; formal analysis: DZ, ZW, PW, AMV; investigation: DZ; resources: DZ; data curation: DZ and PW; writing – original draft preparation: DZ; writing – review and editing: all co-authors; visualization: DZ; supervision: DZ; project administration: DZ; funding acquisition: WIG Jr. and AMV. All authors have read and agreed to the published version of the manuscript.
The contact author has declared that none of the authors has any competing interests.
Publisher’s note: Copernicus Publications remains neutral with regard to jurisdictional claims made in the text, published maps, institutional affiliations, or any other geographical representation in this paper. While Copernicus Publications makes every effort to include appropriate place names, the final responsibility lies with the authors.
This article is part of the special issue “Marine aerosols, trace gases, and clouds over the North Atlantic (ACP/AMT inter-journal SI)”. It is not associated with a conference.
We thank the ACE-ENA field campaign team for their data collection during challenging operations. Data were obtained from the ARM user facility, a U.S. DOE Office of Science user facility managed by the Biological and Environmental Research (BER) program.
This research has been supported by the Biological and Environmental Research program (grant no. DE-AC05-76RL01830).
This paper was edited by André Ehrlich and reviewed by two anonymous referees.
Albrecht, B. A.: Aerosols, cloud microphysics, and fractional cloudiness, Science, 245, 1227–1230, https://doi.org/10.1126/science.245.4923.1227, 1989.
Baumgardner, D., Abel, S. J., Axisa, D., Cotton, R., Crosier, J., Field, P., Gurganus, C., Heymsfield, A., Korolev, A., Krämer, M., Lawson, P., McFarquhar, G., Ulanowski, Z., and Um, J.: Cloud Ice Properties: In Situ Measurement Challenges, Chap. 9, in: Ice Formation and Evolution in Clouds and Precipitation: Measurement and Modeling Challenges, Meteor. Mon., 58, 9.1–9.23, https://doi.org/10.1175/AMSMONOGRAPHS-D-16-0011.1, 2017.
Bennartz, R. and Rausch, J.: Global and regional estimates of warm cloud droplet number concentration based on 13 years of AQUA-MODIS observations, Atmos. Chem. Phys., 17, 9815–9836, https://doi.org/10.5194/acp-17-9815-2017, 2017.
Boers, R., Acarreta, J. R., and Gras, J. L.: Satellite monitoring of the first indirect aerosol effect: Retrieval of the droplet concentration of water clouds, J. Geophys. Res.-Atmos., 111, D22208, https://doi.org/10.1029/2005JD006838, 2006.
Brenguier, J.-L., Burnet, F., and Geoffroy, O.: Cloud optical thickness and liquid water path – does the k coefficient vary with droplet concentration?, Atmos. Chem. Phys., 11, 9771–9786, https://doi.org/10.5194/acp-11-9771-2011, 2011.
Cadeddu, M.: Microwave Radiometer – 3-Channel (MWR3C) Instrument Handbook, ARM Technical Report DOE/SC-ARM-TR-108, DOE Office of Science Atmospheric Radiation Measurement (ARM) Program, https://doi.org/10.2172/1039668, 2021.
Cadeddu, M. P., Liljegren, J. C., and Turner, D. D.: The Atmospheric radiation measurement (ARM) program network of microwave radiometers: instrumentation, data, and retrievals, Atmos. Meas. Tech., 6, 2359–2372, https://doi.org/10.5194/amt-6-2359-2013, 2013.
Cadeddu, M. P., Ghate, V. P., and Mech, M.: Ground-based observations of cloud and drizzle liquid water path in stratocumulus clouds, Atmos. Meas. Tech., 13, 1485–1499, https://doi.org/10.5194/amt-13-1485-2020, 2020.
Chen, J., Liu, Y., Zhang, M., and Peng, Y.: New understanding and quantification of the regime dependence of aerosol-cloud interaction for studying aerosol indirect effects, Geophys. Res. Lett., 43, 1780–1787, https://doi.org/10.1002/2016GL067683, 2016.
Chiu, J. C., Marshak, A., Huang, C.-H., Várnai, T., Hogan, R. J., Giles, D. M., Holben, B. N., O'Connor, E. J., Knyazikhin, Y., and Wiscombe, W. J.: Cloud droplet size and liquid water path retrievals from zenith radiance measurements: examples from the Atmospheric Radiation Measurement Program and the Aerosol Robotic Network, Atmos. Chem. Phys., 12, 10313–10329, https://doi.org/10.5194/acp-12-10313-2012, 2012.
Cromwell, E., Tomlinson, J., and Pekour, M.: Cloud and Aerosol Spectrometer aboard aircraft (AAFCAS), Atmospheric Radiation Measurement (ARM) User Facility [data set], https://doi.org/10.5439/1438488, 2023.
Dong, X., Ackerman, T. P., and Clothiaux, E. E. 1998: Parameterizations of the microphysical and shortwave radiative properties of boundary layer stratus from ground-based measurements, J. Geophys. Res.-Atmos., 103, 31681–31693, https://doi.org/10.1029/1998JD200047, 1998.
Donovan, D. P., Klein Baltink, H., Henzing, J. S., de Roode, S. R., and Siebesma, A. P.: A depolarisation lidar-based method for the determination of liquid-cloud microphysical properties, Atmos. Meas. Tech., 8, 237–266, https://doi.org/10.5194/amt-8-237-2015, 2015.
Fairless, T., Jensen, M., Zhou, A, and Giangrande, S. E: Interpolated Sounding and Gridded Sounding Value-Added Products, ARM Technical Report DOE/SC-ARM-TR-183, DOE Office of Science Atmospheric Radiation Measurement (ARM) Program, https://doi.org/10.2172/1248938, 2021.
Fan, J., Wang, Y., Rosenfeld, D., and Liu, X.: Review of Aerosol–Cloud Interactions: Mechanisms, Significance, and Challenges, J. Atmos. Sci., 73, 4221–4252, https://doi.org/10.1175/JAS-D-16-0037.1, 2016.
Fernald, F. G.: Analysis of atmospheric lidar observations: some comments, Appl. Optics, 23, 652–653, 1984.
Grosvenor, D. P., Sourdeval, O., Zuidema, P., Ackerman, A., Alexandrov, M. D., Bennartz, R., Boers, R., Cairns, B., Chiu, C., Christensen, M., Deneke, H., Diamond, M., Feingold, G., Fridlind, A., Hünerbein, A., Knist, C., Kollias, P., Marshak, A., McCoy, D., Merk, D., Painemal, D., Rausch, J., Rosenfeld, D., Russchenberg, H., Seifert, P., Sinclair, K., Stier, P., B. van D., Wendisch, M., Werner, F., Wood, R., Zhang, Z., and Quaas, J.: Remote sensing of cloud droplet number concentration in warm clouds: A review of the current state of knowledge and perspectives, Rev. Geophys., 56, 409–453, https://doi.org/10.1029/2017RG000593, 2018.
Gryspeerdt, E., Quaas, J., Ferrachat, S., Gettelman, A., Ghan, S., Lohmann, U., Morrison, H., Neubauer, D., Partridge, D. G., Stier, P., Takemura, T., Wang, H., Wang, M., and Zhang, K.: Constraining the instantaneous aerosol influence on cloud albedo, P. Natl. Acad. Sci. USA, 114, 4899–4904, https://doi.org/10.1073/pnas.1617765114, 2017.
Hodges, G. B. and Michalsky, J. J.: Multifilter Rotating Shadowband Radiometer (MFRSR) Handbook With subsections for derivative instruments: Multifilter Radiometer (MFR) Normal Incidence Multifilter Radiometer (NIMFR), ARM Technical Report DOE/SC-ARM-TR-144, DOE Office of Science Atmospheric Radiation Measurement (ARM) Program, https://doi.org/10.2172/1251387, 2016.
Hogan, R. J.: Fast lidar and radar multiple-scattering models. Part I: Small-angle scattering using the photon variance–covariance method, J. Atmos. Sci., 65, 3621–3635, https://doi.org/10.1175/2008JAS2642.1, 2008.
Holdridge, D.: Balloon-Borne Sounding System (SONDE) Instrument Handbook, ARM Technical Report DOE/SC-ARM-TR-029, DOE Office of Science Atmospheric Radiation Measurement (ARM) Program, https://doi.org/10.2172/1020712, 2020.
Illingworth, A. J., Hogan, R. J., O'Connor, E. J., Bouniol, D., Brooks, M. E., Delanoe, J., Donovan, D. P., Eastment, J. D., Gaussiat, N., Goddard, J. W. F., Haeffelin, M., Klein Baltink, H., Krasnov, O. A., Pelon, J., Piriou, J.-M., Protat, A., Russchenberg, H. W. J., Seifert, A., Tompkins, A. M., van Zadelhoff, G.-J., Vinit, F., Willen, U., Wilson, D. R., and Wrench, C. L.: Cloudnet – continuous evaluation of cloud profiles in seven operational models using ground-based observations, B. Am. Meteorol. Soc., 88, 883–898, 2007.
IPCC: Climate Change 2021: The Physical Science Basis. Contribution of Working Group I to the Sixth Assessment Report of the Intergovernmental Panel on Climate Change, edited by: Masson-Delmotte, V., Zhai, P., Pirani, A., Connors, S. L., Péan, C., Berger, S., Caud, N., Chen, Y., Goldfarb, L., Gomis, M. I., Huang, M., Leitzell, K., Lonnoy, E., Matthews, J. B. R., Maycock, T. K., Waterfield, T., Yelekçi, O., Yu, R., and Zhou, B., Cambridge University Press, Cambridge, United Kingdom and New York, NY, USA, 2391 pp., https://doi.org/10.1017/9781009157896, 2021.
Johnson, K. L., Giangrande, S. E., and Zhou, A.: Ka-Band ARM Zenith Radar (KAZR) Active Remote Sensing of Clouds (ARSCL) CloudSat Calibration (KAZRARSCL- CLOUDSAT) (Value-Added Product Report), ARM Technical Report DOE/SC-ARM-TR-279, DOE Office of Science Atmospheric Radiation Measurement (ARM) Program, https://doi.org/10.2172/1847644, 2022.
Klett, J. D.: Stable analytical inversion solution for processing lidar returns, Appl. Optics, 20, 211–220, https://doi.org/10.1364/AO.20.000211, 1981.
Kollias, P., Rémillard, J., Luke, E., and Szyrmer, W.: Cloud radar Doppler spectra in drizzling stratiform clouds: 1. Forward modeling and remote sensing applications, J. Geophys. Res., 116, D13201, https://doi.org/10.1029/2010JD015237, 2011.
Lim, K.-S. S., Riihimaki, L., Comstock, J. M., Schmid, B., Sivaraman, C., Shi, Y., and McFarquhar, G. M.: Evaluation of long-term surface-retrieved cloud droplet number concentration with in situ aircraft observations, J. Geophys. Res.-Atmos., 121, 2318–2331, https://doi.org/10.1002/2015JD024082, 2016.
Luke, E. and Kollias, P.: Separating Cloud and Drizzle Radar Moments during Precipitation Onset Using Doppler Spectra, J. Atmos. Ocean. Tech., 26, 167–179, 1656–1671, https://doi.org/10.1175/JTECH-D-11-00195.1, 2013.
Mace, G. G. and Sassen, K.: A constrained algorithm for retrieval of stratocumulus cloud properties using solar radiation, microwave radiometer, and millimeter cloud radar data, J. Geophys. Res., 105, 29099– 29108, https://doi.org/10.1029/2000JD900403, 2000.
Marais, W. J., Holz, R. E., Hu, Y. H., Kuehn, R. E., Eloranta, E. E., and Willett, R. M.: Approach to simultaneously denoise and invert backscatter and extinction from photon-limited atmospheric lidar observations, Appl. Optics, 55, 8316–8334, https://doi.org/10.1364/AO.55.008316, 2016.
Martin, G. M., Johnson, D. W., and Spice, A.: The Measurement and Parameterization of Effective Radius of Droplets in Warm Stratocumulus Clouds, J. Atmos. Sci., 51, 1823–1842, https://doi.org/10.1175/1520-0469(1994)051<1823:TMAPOE>2.0.CO;2., 1994.
Martucci, G. and O'Dowd, C. D.: Ground-based retrieval of continental and marine warm cloud microphysics, Atmos. Meas. Tech., 4, 2749–2765, https://doi.org/10.5194/amt-4-2749-2011, 2011.
McComiskey, A., Feingold, G., Frisch, A. S., Turner, D. D., Miller, M. A., Chiu, J. C., Min, Q., and Ogren, J. A.: An assessment of aerosol-cloud interactions in marine stratus clouds based on surface remote sensing, J. Geophys. Res.-Atmos., 114, D09203, https://doi.org/10.1029/2008JD011006, 2009.
McCoy, I. L., McCoy, D. T., Wood, R., Regayre, L., Watson-Parris, D., Grosvenor, D. P., Mulcahy, J. P., Hu, Y., Bender, F. A.-M., Field, P. R., Carslaw, K. S., and Gordon, H.: The hemispheric contrast in cloud microphysical properties constrains aerosol forcing, P. Natl. Acad. Sci. USA, 117, 18998–19006, https://doi.org/10.1073/pnas.1922502117,2020.
Mei, F., Burk, K., Ermold, B., and Zhang, D.: Fast Cloud Droplet Probe aboard aircraft (AAFFCDP), Atmospheric Radiation Measurement (ARM) User Facility [data set], https://doi.org/10.5439/1417472, 2023.
Merk, D., Deneke, H., Pospichal, B., and Seifert, P.: Investigation of the adiabatic assumption for estimating cloud micro- and macrophysical properties from satellite and ground observations, Atmos. Chem. Phys., 16, 933–952, https://doi.org/10.5194/acp-16-933-2016, 2016.
Miles, N. L., Verlinde, J., and Clothiaux, E. E.: Cloud Droplet Size Distributions in Low-Level Stratiform Clouds, J. Atmos. Sci., 57, 295–311, https://doi.org/10.1175/1520-0469(2000)057<0295:CDSDIL>2.0.CO;2, 2000.
Min, Q. and Harrison, L. C.: Cloud properties derived from surface MFRSR measurements and comparison with GOES results at the ARM SGP site, Geophys. Res. Lett., 23, 1641–1644, https://doi.org/10.1029/96GL01488, 1996.
Moore, R. H., Karydis, V. A., Capps, S. L., Lathem, T. L., and Nenes, A.: Droplet number uncertainties associated with CCN: an assessment using observations and a global model adjoint, Atmos. Chem. Phys., 13, 4235–4251, https://doi.org/10.5194/acp-13-4235-2013, 2013.
Muradyan, P. and Coulter, R.: Micropulse Lidar (MPL) Handbook, ARM Technical Report DOE/SC-ARM-TR-019, DOE Office of Science Atmospheric Radiation Measurement (ARM) Program, https://doi.org/10.2172/1020714, 2020.
Newsom, R. K., Bambha, R., and Chand, D.: Raman Lidar (RL) Instrument Handbook, ARM Technical Report DOE/SC-ARM-TR-038, DOE Office of Science Atmospheric Radiation Measurement (ARM) Program, https://doi.org/10.2172/1020561, 2022.
O'Connor, E. J., Illingworth, A. J., and Hogan, R. J.: A Technique for Autocalibration of Cloud Lidar, J. Atmos. Ocean. Tech., 21, 777–786, https://doi.org/10.1175/1520-0426(2004)021<0777:ATFAOC>2.0.CO;2, 2004.
Painemal, D. and Zuidema, P.: Microphysical variability in southeast Pacific Stratocumulus clouds: synoptic conditions and radiative response, Atmos. Chem. Phys., 10, 6255–6269, https://doi.org/10.5194/acp-10-6255-2010, 2010.
Pawlowska, H., Grabowski, W. W., and Brenguier, J.-L.: Observations of the width of cloud droplet spectra in stratocumulus, Geophys. Res. Lett., 33, L19810, https://doi.org/10.1029/2006GL026841, 2006.
Regayre, L. A., Pringle, K. J., B. Booth, B. B., Lee, L. A., Mann, G. W., Browse, J., Woodhouse, M. T., Rap, A., Reddington, C. L., and Carslaw, K. S.: Uncertainty in the magnitude of aerosol-cloud radiative forcing over recent decades, Geophys. Res. Lett., 41, 9040–9049, https://doi.org/10.1002/2014GL062029, 2014.
Riihimaki, L., McFarlane, S., and Sivaraman, C.: Droplet Number Concentration Value-Added Product, ARM Technical Report DOE/SC-ARM-TR-140, DOE Office of Science Atmospheric Radiation Measurement (ARM) Program, https://doi.org/10.2172/1237963, 2021.
Riihimaki, L., McFarlane, S., Sivaraman, C., and Zhang, D.: Droplet number concentration (NDROPMFRSR), Atmospheric Radiation Measurement (ARM) User Facility [data set], https://doi.org/10.5439/1131339, 2023.
Rosenfeld, D., Zhu, Y., Wang, M., Zheng, Y., Goren, T., and Yu, S.: Aerosol-driven droplet concentrations dominate coverage and water of oceanic low-level clouds, Science, 363, eaav0566, https://doi.org/10.1126/science.aav0566, 2019.
Sarna, K., Donovan, D. P., and Russchenberg, H. W. J.: Estimating the optical extinction of liquid water clouds in the cloud base region, Atmos. Meas. Tech., 14, 4959–4970, https://doi.org/10.5194/amt-14-4959-2021, 2021.
Schmidt, J., Wandinger, U., and Malinka, A.: Dual-field-of-view Raman lidar measurements for the retrieval of cloud microphysical properties, Appl. Optics, 52, 2235, https://doi.org/10.1364/AO.52.002235, 2013.
Snider, J. R., Leon, D., and Wang, Z.: Droplet Concentration and Spectral Broadening in Southeast Pacific Stratocumulus Clouds, J. Atmos. Sci., 74, 719–749, https://doi.org/10.1175/JAS-D-16-0043.1, 2017.
Stephens, G. L., Li, J., Wild, M., Clayson, C. A., Loeb, N., Kato, S., L'Ecuyer, T., Stackhouse Jr., P. W., Lebsock, M., and Andrews, T.: An update on Earth's energy balance in light of the latest global observations, Nat. Geosci., 5, 691–696, https://doi.org/10.1038/ngeo1580, 2012.
Storelvmo, T., Kristjánsson, J. E., Ghan, S. J., Kirkevåg, A., Seland, Ø., and Iversen, T.: Predicting cloud droplet number concentration in Community Atmosphere Model (CAM)-Oslo, J. Geophys. Res., 111, D24208, https://doi.org/10.1029/2005JD006300, 2006.
Thorsen, T. J. and Fu, Q.: Automated Retrieval of Cloud and Aerosol Properties from the ARM Raman Lidar. Part II: Extinction, J. Atmos. Ocean. Tech., 32, 1999–2023, https://doi.org/10.1175/JTECH-D-14-00178.1, 2015.
Thorsen, T. J., Fu, Q., Newsom, R. K., Turner, D. D., and Comstock, J. M.: Automated Retrieval of Cloud and Aerosol Properties from the ARM Raman Lidar. Part I: Feature Detection, J. Atmos. Ocean. Tech., 32, 1977–1998, https://doi.org/10.1175/JTECH-D-14-00150.1, 2015.
Turner, D. D., Clough, S. A., Liljegren, J. C., Clothiaux, E. E., Cady-Pereira, K. E., and Gaustad, K. L.: Retrieving liquid water path and precipitable water vapor from the Atmospheric Radiation Measurement (ARM) microwave radiometers, IEEE T. Geosci. Remote, 45, 3680–3690, 2007.
Turner, D. D., McFarlane, S. A., Riihimaki, L., Shi, Y., Lo, C., and Min, Q.: Cloud Optical Properties from the Multifilter Shadowband Radiometer (MFRSRCLDOD): An ARM Value- Added Product, ARM Technical Report DOE/SC-ARM-TR-139, DOE Office of Science Atmospheric Radiation Measurement (ARM) Program, https://doi.org/10.2172/1237958, 2014.
Twomey, S.: Influence of pollution on shortwave albedo of clouds, J. Atmos. Sci., 34, 1149–1152, https://doi.org/10.1175/1520-0469(1977)034<1149:TIOPOT>2.0.CO;2, 1977.
Vogelmann, A. M., McFarquhar, G. M., Ogren, J. A., Turner, D. D., Comstock, J. M., Feingold, G., Long, C. N., Jonsson, H. H., Bucholtz, A., Collins, D. R., Diskin, G. S., Gerber, H., Lawson, R. P., Woods, R. K., Andrews, E., Yang, H.-J., Chiu, J. C., Hartsock, D., Hubbe, J. M., Lo, C., Marshak, A., Monroe, J. W., McFarlane, S. A., Schmid, B., Tomlinson, J. M., and Toto, T.: Racoro Extended-Term Aircraft Observations of Boundary Layer Clouds, B. Am. Meteorol. Soc., 93, 861–878, https://doi.org/10.1175/BAMS-D-11-00189.1, 2012.
Wang, J., Wood, R., Jensen, M. P., Chiu, J. C., Liu, Y., Lamer, K., Desai, N., Giangrande, S. E., Knopf, D. A., Kollias, P., Laskin, A., Liu, X., Lu, C., Mechem, D., Mei, F., Starzec, M., Tomlinson, J., Wang, Y., Yum, S. S., Zheng, G., Aiken, A. C., Azevedo, E. B., Blanchard, Y., China, S., Dong, X., Gallo, F., Gao, S., Ghate, V. P., Glienke, S., Goldberger, L., Hardin, J. C., Kuang, C., Luke, E. P., Matthews, A. A., Miller, M. A., Moffet, R., Pekour, M., Schmid, B., Sedlacek, A. J., Shaw, R. A., Shilling, J. E., Sullivan, A., Suski, K., Veghte, D. P., Weber, R., Wyant, M., Yeom, J., Zawadowicz, M., and Zhang, Z.: Aerosol and Cloud Experiments in the Eastern North Atlantic (ACE-ENA), B. Am. Meteorol. Soc, 103, E619–E641, https://doi.org/10.1175/BAMS-D-19-0220.1, 2022.
Welton, E. J., Campbell, J. R., Spinhirne, J. D., and Scott, V. S.: Global monitoring of clouds and aerosols using a network of micro-pulse lidar systems, in: Lidar Remote Sensing for Industry and Environmental Monitoring, edited by: Singh, U. N., Itabe, T., and Sugimoto, N., Proc. SPIE, 4153, 151–158, https://doi.org/10.1117/12.417040, 2001.
Wood, R., Mechoso, C. R., Bretherton, C. S., Weller, R. A., Huebert, B., Straneo, F., Albrecht, B. A., Coe, H., Allen, G., Vaughan, G., Daum, P., Fairall, C., Chand, D., Gallardo Klenner, L., Garreaud, R., Grados, C., Covert, D. S., Bates, T. S., Krejci, R., Russell, L. M., de Szoeke, S., Brewer, A., Yuter, S. E., Springston, S. R., Chaigneau, A., Toniazzo, T., Minnis, P., Palikonda, R., Abel, S. J., Brown, W. O. J., Williams, S., Fochesatto, J., Brioude, J., and Bower, K. N.: The VAMOS Ocean-Cloud-Atmosphere-Land Study Regional Experiment (VOCALS-REx): goals, platforms, and field operations, Atmos. Chem. Phys., 11, 627–654, https://doi.org/10.5194/acp-11-627-2011, 2011.
Wood, R., Wyant, M., Bretherton, C. S., Rémillard, J., Kollias, P., Fletcher, J., Stemmler, J., De Szoeke, S., Yuter, S., Miller, M., Mechem, D., Tselioudis, G., Chiu, J. C., Mann, J. A. L., O'Connor, E. J., Hogan, R. J., Dong, X., Miller, M., Ghate, V., Jefferson, A., Min, Q., Minnis, P., Palikonda, R., Albrecht, B., Luke, E., Hannay, C., and Lin, Y.: Clouds, aerosols, and precipitation in the marine boundary layer: An arm mobile facility deployment, B. Am. Meteorol. Soc., 96, 419–440, https://doi.org/10.1175/BAMS-D-13-00180.1, 2015.
Wu, P., Dong, X., Xi, B., Tian, J., and Ward, D. M.: Profiles of MBL Cloud and Drizzle Microphysical Properties Retrieved From Ground-Based Observations and Validated by Aircraft In Situ Measurements Over the Azores, J. Geophys. Res.-Atmos., 125, e2019JD032205, https://doi.org/10.1029/2019JD032205, 2020a.
Wu, P., Dong, X., and Xi, B.: A climatology of marine boundary layer cloud and drizzle properties derived from groundbased observations over the azores, J. Climate, 33, 10133–10148, https://doi.org/10.1175/JCLI-D-20-0272.1, 2020b.
Yeom, J. M., Yum, S. S., Shaw, R. A., La, I., Wang, J., Lu, C., Liu, Y., Mei, F., Schmid, B., and Matthews, A.: Vertical Variations of Cloud Microphysical Relationships in Marine Stratocumulus Clouds Observed During the ACE-ENA Campaign, J. Geophys. Res.-Atmos., 126, e2021JD034700, https://doi.org/10.1029/2021JD034700, 2021.
Zelinka, M. D., Randall, D. A., Webb, M. J., and Klein, S. A.: Clearing Clouds of Uncertainty, Nat. Clim. Change, 7, 674–678, https://doi.org/10.1038/nclimate3402, 2017.
Zhang, D.: Lidar-based cloud droplet number concentration retrievals at the ARM ENA observatory, Atmospheric Radiation Measurement (ARM) User Facility [data set], https://adc.arm.gov/discovery/#/results/s::droplet%20number%20concentration, last access: 5 December 2023.
Zhang, D., Vogelmann, A., Kollias, P., Luke, E., Yang, F., Lubin, D., and Wang, Z.: Comparison of Antarctic and Arctic single-layer stratiform mixed-phase cloud properties using ground-based remote sensing measurements. J. Geophys. Res.-Atmos., 124, 10186–10204, https://doi.org/10.1029/2019JD030673, 2019.
Zhang, Z., Song, Q., Mechem, D. B., Larson, V. E., Wang, J., Liu, Y., Witte, M. K., Dong, X., and Wu, P.: Vertical dependence of horizontal variation of cloud microphysics: observations from the ACE-ENA field campaign and implications for warm-rain simulation in climate models, Atmos. Chem. Phys., 21, 3103–3121, https://doi.org/10.5194/acp-21-3103-2021, 2021.
Zhao, C., Xie, S., Klein, S. A., Protat, A., Shupe, M. D., McFarlane, S. A., Comstock, J. M., Delanoë, J., Deng, M., Dunn, M., Hogan, R. J., Huang, D., Jensen, M. P., Mace, G. G., McCoy, R., O'Connor, E. J., Turner, D. D., and Wang, Z.: Toward understanding of differences in current cloud retrievals of ARM ground-based measurements, J. Geophys. Res.-Atmos., 117, D10206, https://doi.org/10.1029/2011JD016792, 2012.
Zhu, Z., Kollias, P., Luke, E., and Yang, F.: New insights on the prevalence of drizzle in marine stratocumulus clouds based on a machine learning algorithm applied to radar Doppler spectra, Atmos. Chem. Phys., 22, 7405–7416, https://doi.org/10.5194/acp-22-7405-2022, 2022.