the Creative Commons Attribution 4.0 License.
the Creative Commons Attribution 4.0 License.
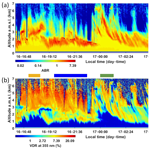
Raman lidar-derived optical and microphysical properties of ice crystals within thin Arctic clouds during PARCS campaign
Patrick Chazette
Jean-Christophe Raut
Cloud observations in the Arctic are still rare, which requires innovative observation techniques to assess ice crystal properties. We present an original approach using the Raman lidar measurements applied to a case study in northern Scandinavia. The vertical profiles of the optical properties, the effective radius of ice crystals and ice water content (IWC) in Arctic semi-transparent clouds were assessed using quantitative ground-based lidar measurements at 355 nm performed from 13 to 26 May 2016 in Hammerfest (north of Norway, 70∘39′48′′ N, 23∘41′00′′ E). The field campaign was part of the Pollution in the ARCtic System (PARCS) project of the French Arctic Initiative. The presence of low-level semi-transparent clouds was noted on 16 and 17 May. The cloud base was located just above the atmospheric boundary layer where the 0 ∘C isotherm reached around 800 m above the mean sea level (a.m.s.l.). To ensure the best penetration of the laser beam into the cloud, we selected case studies with cloud optical thickness (COT) lower than 2 and out of supercooled liquid pockets. Lidar-derived multiple scattering coefficients were found to be close to 1 and ice crystal depolarization around 10 %, suggesting that ice crystals were small and had a rather spherical shape. Using Mie computations, we determine effective radii between ∼7 and 25 µm in the clouds for ice water contents between 1 and 8 mg m−3, respectively. The uncertainties regarding the effective radii and ice water content are on average 2 µm and 0.65 mg m−3, respectively.
- Article
(11780 KB) - Full-text XML
- BibTeX
- EndNote
Cloud radiative effects significantly influence the radiative budget of the planet at high latitudes (Kay and Gettelman, 2009; Schweiger et al., 2008; Morrison et al., 2012), particularly beyond the Arctic Circle, and contribute to the well-known “Arctic amplification” phenomenon (Serreze and Barry, 2011; Liu and Key, 2014; Wendisch et al., 2019). In particular, clouds have impacted sea ice and glaciers which have been melting for several years in connection with climate change (Serreze et al., 2009; Hansen et al., 2010; Koenigk et al., 2013).
Arctic clouds exhibit a robust annual cycle with maximum cloudiness in fall and minimum cloudiness in winter (Yu et al., 2019). The annual cloud fraction amounts to about 80 %, with predominant low-level clouds up to 70 % of the time from spring to autumn (Curry and Ebert, 1992). Based on observations from spaceborne LITE (Lidar In-space Technology Experiment), GLAS (Geoscience Laser Altimeter System), and CALIOP (Cloud-Aerosol LIdar with Orthogonal Polarization) lidar, Berthier et al. (2008) showed that those low-level stratiform clouds represent between 30 % and 40 % of the cloud cover. As they are located in air masses below the 0 ∘C isotherm, these clouds are very often composed of ice crystals. The presence of supercooled liquid water droplets at the top of these clouds has nevertheless often been reported (Morrison et al., 2012; Shupe et al., 2008). Their subgrid-scale treatment is usually underestimated by physical parameterizations used in the climate models (Klaus et al., 2016; Taylor et al., 2019). This leads to a wrong representation of surface radiative fluxes, both in the solar and infrared spectra (Harrington et al., 1999; Klein et al., 2009; Maillard et al., 2021; Koenigk et al., 2013; Di Biagio et al., 2021). Reliable observations of microphysical and optical properties of ice particles, in particular of their ice crystal effective sizes, are critical for the evaluation of cloud parameterizations and for determining how clouds impact radiation (Zhang et al., 1996). Harrington and Olsson (2001) showed changes of up to 80 W m−2 due to a variation in the effective radius of ice particles. Accurately representing ice clouds in models is therefore necessary to realistically simulate the evolution of the Arctic surface energy budget.
The accuracy of cloud observations has been greatly improved in recent decades by the widespread use of lidar observations so as to better understand and predict the earth system climate (e.g., Hoffmann et al., 2009). Since the precursory work of Platt (1977), ground-based lidar observations have made it possible to better characterize the vertical distribution of cloud structures (Sassen, 1991). Coupled with infrared radiometric observations and even radar measurements, microphysical properties, such as the effective radius of ice crystals, are now accessible (Kalesse et al., 2016). With aircraft and satellite-based instrumentation, microphysical properties are now rendered at a larger spatial scale (Delanoë and Hogan, 2010; Chazette et al., 2022; Liu et al., 2012; Lampert et al., 2009). However, the velocity of those instrumented platforms, along with their temporal resolution, prevent us from sampling clouds at a spatial resolution which would be adequate to properly highlight their internal structure. Indeed, the resulting data provide cloud properties typically averaged over 5 km for satellites and over 1 km for airborne platforms, which may be insufficient to study cloud processes at a microphysical scale. Examining cloud structures with a high horizontal and vertical resolutions is, however, possible via ground-based lidars. Such instruments are unable to match the level of detail from aircraft in situ measurements or the spatial coverage of satellites, but they are fittingly positioned to capture the diurnal variability with high temporal and vertical resolutions (Shupe et al., 2011). This remains a challenge, as it requires that lidar measurements penetrate through the cloud with low attenuation. Semi-transparent clouds with small optical thicknesses (≲2) are therefore accessible to lidar measurements.
Due to the growing interest of the climate science community in clouds over the Arctic region, numerous experiments have been set up recently, for instance, the ACLOUD (Arctic Cloud Observations Using airborne measurements during polar Day (Ehrlich et al., 2019)) and the PASCAL (Physical feedback of Arctic PBL, Sea ice, Cloud And AerosoL (Wendisch et al., 2019)), both in 2017; AFLUX (Airborne measurements of radiative and turbulent FLUXes of energy and momentum in the Arctic boundary layer (Mech et al., 2022)) in 2019; or MOSAiC (Multidisciplinary Drifting Observatory for the Study of Arctic Climate (Shupe et al., 2022)) in 2019–2020. In this respect, we conducted Raman lidar observations at 355 nm during the PARCS (Pollution in the ARCtic System) field experiment (Chazette et al., 2018; Totems et al., 2019) in May 2016 at Hammerfest (Norway, over 70∘ N) in order to better constrain the estimate of ice crystal properties in thin cloud vertical structures. The high vertical resolution of the lidar allowed us to highlight the presence of supercooled water pockets embedded into ice clouds and to trace the vertical profiles of the optical properties of semi-transparent clouds. It was then possible to derive the hydrometeor size profiles taking into account the multiple scattering effects.
The lidar system and associated theory are presented in Sect. 2. Section 3 describes the methodology used to retrieve the effective radii of ice crystals and ice water content from the lidar measurements. The optical properties of the ice crystals accessible to the Raman lidar measurements are given in Sect. 4 and their associated effective radius in Sect. 5. Finally, the conclusions summarizing the findings are provided in Sect. 6.
2.1 Instrument
The ground-based WALI (Water vapor and Aerosol Lidar) was the Raman lidar operated during the PARCS campaign (Totems et al., 2019; Chazette et al., 2018). It uses an emitted wavelength of 354.7 nm and is designed to fulfill eye-safety conditions. During the field experiment, the acquisition was performed continuously with a vertical resolution of 15 m for mean profiles of 1000 laser shots, leading to a temporal sampling close to 1 min. The overlap function of this lidar is ∼200 m and can be assessed experimentally for lidar signal correction in the lowest atmospheric layers.
Three different channels have been used to study ice-clouds: the first one to detect the total (co-polarized and cross-polarized with respect to the polarized laser emission) backscatter coefficients, the second to detect solely the cross-polarized backscatter coefficients, and the third to detect the vibrational Raman scattering on nitrogen. The field of view of the lidar is less than 2 mrad to minimize the effect of multiple scattering within the troposphere.
In the following, the lidar equation is applied to the specific case of thin ice clouds.
2.2 Approach
Using Raman lidar sampling of thin ice clouds, we aimed to retrieve the vertical profiles of the optical and structural properties of ice crystals. This type of data remains very sporadic and is sorely lacking for a realistic modeling of the climatic impact of ice clouds.
Our approach was the following: (1) isolate the cloud structures that are entirely sampled by the lidar laser beam; (2) assess the cloud optical thickness (COT) via the elastic and N2-Raman channels and check the consistency of the result; (3) compute the integrated apparent backscatter coefficient of the cloud to derive the effective lidar ratio (LR), product of the LR by the multiple scattering coefficient; (iv) compute the vertical profiles of the extinction and backscatter coefficients, and the ice crystal depolarization ratio (ICDR), in the cloud; and (v) invert Mie calculations to estimate the effective size of the ice crystal and ice water content of the selected cloud layers.
2.3 Theory
After the molecular transmission, background radiance and solid angle are corrected, and the elastic lidar signal in the form of apparent backscatter coefficient (βapp) at zenith viewing beyond the influence of the overlap factor (∼200 m) is written at the altitude z in the cloud as
with
and
where C is the lidar system constant, and βm and βa are the backscatter coefficients due to molecules and aerosols, respectively. The cloud backscatter coefficient is represented by βc. The sum of the backscatter coefficients is the total volume backscatter coefficient (β). The vertical profile of the COT is calculated by integrating the extinction coefficient of ice crystal (αc) between the cloud base (zb) and altitude z in the cloud. Equation (1) assumes that the extinction coefficient due to aerosols is negligible above zb, which is realistic during the PARCS campaign (Chazette et al., 2018). The aerosol optical thickness (AOT) is therefore calculated under the cloud base from lidar altitude zo to zb as the integral of the aerosol extinction coefficient (αa). The multiple scattering coefficient η as defined by Platt (1981) traces the optical path lengthening associated with the multiple scattering process by the hydrometeors. The system constant C is obtained in cloud-free condition via the synergy between the lidar elastic and Raman channels above the planetary boundary layer (PBL) top, where only molecular scattering occurs, with a resultant relative accuracy of 0.5 %.
For the N2-Raman channel, after correction for molecular transmission and normalization to atmospheric density, the apparent N2-Raman lidar signal is written in a proportionality relationship as
where Ac (Aa) is the Ångström exponent in the cloud (aerosol layer) between the wavelengths of 355 and 387 nm. Ångström exponents are supposed to be constant. It is reasonable to assume Ac to be close to 0. It is also assumed here that the multiple scattering coefficient in clouds is the same at 355 and 387 nm.
2.3.1 Cloud optical thickness
Using the elastic scattering channel, the ratio of βapp above the top (zt) and below the base (zb) of the cloud, where molecular diffusion is assumed to be the only contribution to the lidar signal (negligible AOT), can be written as
Hence, we can assess the COT between zb and zt by
The COT can also be determined directly from the N2-Raman channel by normalizing to the altitude at the cloud base zb for each altitude z:
Accurate determination of the COT requires knowing the multiple scattering coefficient η. At this stage, we do not know this value, so we can only calculate the effective COT () instead, which includes multiple scattering processes. Furthermore, it should be noted immediately that if independent determinations of the COTe by the elastic and N2-Raman channels match, this means that the assumption of free-aerosol altitude zones below and above the cloud is justified. Indeed, in this case, aerosols have negligible influence on the determination of COTe via the N2-Raman channel.
2.3.2 Vertical profiles of the extinction coefficient and lidar ratio
The simultaneous use of the N2-Raman and elastic channels makes it possible to find the vertical profiles of the extinction and backscatter coefficients associated with the clouds, and then the LR. The LR is a characteristic parameter of the scattering layers (Chazette et al., 2016).
Combining Eqs. (1) and (5), the cloud backscatter coefficient between zb and zt can be derived for each z as
The AOT derived from Raman lidar below the cloud is lower than 0.04 in the PBL as in Chazette et al. (2018) just before the arrival of clouds.
In fact, COTe(zb,z) is the effective cumulative optical thickness profile that tends towards the COTe of the cloud when z is close to zt. Its derivative with respect to z gives the effective extinction coefficient from which we obtain the vertical profile of the effective LR (LRe), which is the ratio of to βc:
It is worth noting that given the degrees of freedom of the equation system, only the effective values are assessed.
The effective LR ratio equivalent to the cloud layer () can be directly evaluated by weighting LRe by the cloud backscatter coefficient:
2.3.3 Ice crystal linear depolarization ratio
The linear volume depolarization ratio (VDR) is calculated via the ratio of perpendicularly and parallelly polarized channels defined with respect to the laser emission as in Chazette et al. (2012) where the different sources of uncertainty are discussed. The ICDR is calculated using a similar relationship to that used for aerosols (Chazette et al., 2012), from VDR and βc:
where the molecular linear volume depolarization ratio (VDRm) has been taken as equal to 0.3945 % at 355 nm following Collis and Russel (1976) for Cabannes scattering.
2.3.4 Multiple scattering coefficient
To evaluate η, we used a Monte Carlo radiative transfer model specially developed for lidar measurements. This model was used to analyze the LITE (Lidar In-Space Technology Experiment) measurements by Berthier et al. (2006). It has also been used to estimate multiple scattering through forest canopy (Shang and Chazette, 2015) for airborne and spaceborne lidar measurements. The outputs of the model were successfully compared with the results of Wiegner et al. (1997) and again compared with simulations performed using the photon variance–covariance (PVC) method for quasi-small-angle multiple scattering (Hogan, 2006). The PVC algorithm can represent anisotropic phase function in the near 180∘ direction. As we noticed a very small difference in η (<5 %) between the two modeling approaches, we can infer that both approaches validate each other. The Monte Carlo model was initialized with the ice crystal phase functions determined by the method presented below, themselves constrained by the lidar measurements.
In order to assess the vertical profiles of the ice crystal effective radius (reff) and ice water content (IWC), we use a Mie code assuming ice particles are all spherical (Sect. 4.3). Ice crystals are generally not spherical. Nevertheless, for the clouds sampled in this study, the ICDR is ∼10 % (Sect. 4.3), far from the values associated with highly non-spherical crystals whose ICDR is between 30 % and 50 %. The complex index of refraction of ice is taken from the database established by Warren and Brandt (2008) from wavelengths between 44 nm and 2 µm at 266 K. The refractive index of ice crystals has been interpolated against the logarithm of the wavelength and assessed to be equal to 1.324 at the wavelength of the lidar (355 nm), with a negligible imaginary part.
Cloud ice crystal size distributions are commonly represented by generalized Gamma distribution (Stephens et al., 1990) as in microphysical schemes most widely used (Milbrandt and Yau, 2005; Morrison and Gettelman, 2008; Thompson et al., 2008):
where r is the radius of the ice crystal and dN the number concentration of ice crystals between r and r+dr. The intercept, slope, and shape parameters of the size distribution are N0i, λi, and μi, respectively. The normalized size distribution n(r) of the ice crystals can also be written equivalently as a function of r and the effective radius reff (Hansen and Travis, 1974):
where νeff is the effective variance of the distribution and Γ the Euler gamma function. The total number of ice crystals is symbolized by Nt.
Here, ice crystals are assumed to be spheres. In this paper, to evaluate the sensitivity of the effective variance νeff of ice crystal size distribution on cloud optical properties, we test two different values. First, the shape parameter μi is assumed to be zero (Marshall–Palmer distribution) as in many well-used two-moment microphysical schemes (Morrison et al., 2005; Thompson et al., 2008). The effective variance νeff is therefore . Second, we consider a smaller value of the effective variance (νeff=0.2), corresponding to a larger shape parameter (), which reduces the backscattering, then the LR, for the smallest particles.
The flow chart in Fig. 1 shows the process used to assess reff and IWC. Lidar-derived LR and IWC are compared with those calculated by a Mie code to retrieve the microphysical parameters of the ice crystals. Naturally, this calculation is carried out in altitude ranges where only ice crystals are present, i.e., with significant VDRs (>2), avoiding pockets of liquid water.
Effective radius. The extinction and backscattering cross-sections are calculated using a Mie code as a function of the ice crystal radius in the range 1–200 µm. For a series of reff values ranging from 5 to 40 µm, the extinction and backscatter coefficients are then derived by integrating their respective cross sections over the size distribution n(r), enabling the retrieval of LR for each value of reff. By definition, LR is independent of the assumed value of the total number of ice crystals Nt. Figure 2 shows the variation of LR as the function of reff following Mie calculation for νeff equal to 0.20 and 0.33. LR turns out to be a monotonically decreasing function of reff. By minimizing the discrepancies between the theoretical and measured values of LR, the effective radius of ice crystals can then unequivocally be determined for the considered size distribution range.
The parameters of the size distribution are thus derived as
where Nt is the total number of ice crystals obtained such that the theoretical extinction coefficient matches its observed counterpart.
Ice water content. After assessing the concentration of ice crystals by comparing the cloud extinction coefficients derived from the lidar measurement and the Mie code, the IWC is obtained using
where ρi=920 kg m−3 is the density of pure ice.
The procedure is repeated at each altitude sampled, enabling us to derive vertical profiles of reff and IWC for each case study.
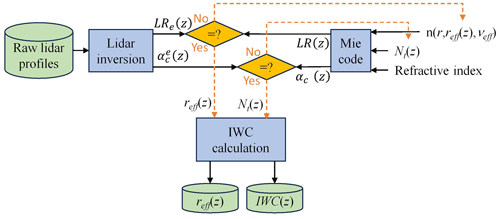
Figure 1Flow chart of the process used to assess the effective radius reff and the ice water content (IWC) at each altitude z by comparing the lidar ratio (LR) and the cloud extinction coefficient derived from lidar inversion and those calculated by a Mie code. The Mie code is initialized by the normalized size number distribution n(r) calculated for each radius r, the total number Nt and the refractive index of the ice crystals. Multiple scattering is assumed to be negligible.
4.1 Site and meteorological synoptic conditions
The lidar measurements were obtained near Hammerfest Airport on the island of Kvaløya, which lies in a southwest/northeast trough at an altitude of ∼90 m a.m.s.l. The site is bounded by the Norwegian Sea to the west and the Barents Sea to the north. It is bordered by relief peaking at around 360 m a.m.s.l. in the northwest and relief reaching up to 1045 m a.m.s.l. in the southeast. These reliefs can therefore significantly influence flows over the site by generating wind shears and vertical mixing.
From 14 to 16 May 2016, two ridges blocked a low-pressure system between the Barents and Norwegian seas. This weather situation is illustrated by the geopotential height at 850 hPa (∼1.5 km a.m.s.l.) in Fig. 3a, where the wind field is superimposed. The first ridge is located over Greenland and extends from the mid-latitudes to the Pole, while the second ridge is located between 55 and 90∘ E and passes over the Novaya Zemlya archipelago. The latter weakened from 16 to 17 May and thus favored the development of a low-pressure system around the Svalbard archipelago. This low then spread to the south of the Norwegian Sea. In the afternoon of 16 May, Hammerfest was on the edge of the low (Fig. 3b) with stratified cloud structures before it spread to cover the town completely by the late morning of 17 May (Fig. 3c). This led to a significant evolution of the cloud cover towards denser and precipitating clouds which appeared on the afternoon of 17 May over Hammerfest. The retrieval of the optical parameters of ice crystal relies on the lidar observations of semi-transparent frozen clouds before the arrival of denser precipitating clouds. The meteorological situation corresponds to a warm southwestern regime as named by Mioche et al. (2017).
4.2 Description of cloud structures
Just before the arrival of clouds over the measurement site, ultralight aircraft flights were made with a payload including (i) a meteorological probe to measure temperature, relative humidity, and pressure; and (ii) the Lidar for Automatic Atmospheric Survey Using Raman Scattering (LAASURS) for the retrieval of the aerosol extinction coefficient. This payload is described in Chazette et al. (2018). The vertical profiles of the temperature, relative humidity, and aerosol extinction coefficient derived from the ultralight payload on 16 May 2016, 11:30 local time (LT), are given in Fig. 4. That latter is negligible over 1.2 km above mean sea level (a.m.s.l.) (Chazette et al., 2018). The 0 ∘C isotherm is reached at an altitude of 0.8 km a.m.s.l. with relative humidity increasing with altitude. With the advection of moist air masses over the site, the presence of cold clouds is therefore probable above 0.8 km a.m.s.l. This value is within the range of the bottom height of boundary layer mixed-phase clouds in the western Arctic (McFarquhar et al., 2007; Maillard et al., 2021). At temperatures close to those encountered here, i.e., slightly below 0 ∘C, Luke et al. (2021) have recently shown that secondary ice formation can significantly increase the amount of ice crystals in clouds in the Arctic.
In the early afternoon of 16 May, the sky became overcast, leading to stratiform and cirrus clouds around 16:00 LT. These clouds were sampled between 16 and 17 May by the ground-based lidar. The apparent backscatter ratio (), including the attenuation due to aerosols and clouds, and VDR time series are given in Fig. 5a. The clouds extend between ∼0.8 and 6 km a.m.s.l. and display complex structures highlighting their great heterogeneity. Some stratiform clouds may occur at 2 km altitude on 16 May and in the range 2–3 km altitude on 17 May and can be detected by a strong attenuation of the lidar signal at their tops. This might indicate the presence of supercooled liquid droplets at the top of mixed-phase clouds as often reported in the Arctic region (Mioche et al., 2017; McFarquhar et al., 2017). Higher clouds (2–6 km altitude) are also detected by the lidar. The sedimentation of ice crystals from those higher ice clouds leads to a glaciation of most of the lower liquid cloud layers (i.e., the seeder–feeder effect; Fernández-González et al., 2015).
The VDR is also highly variable temporally and vertically (Fig. 5b). The higher values (>8 %) indicate the location of frozen hydrometeors, while the lower values inside clouds (<1 %) may indicate the presence of supercooled water liquid droplets or molecular holes in the cloud structure. It is worth noting that, as the cloud-related scattering coefficient is very large compared with that of the molecules and aerosols, the VDR should not be very far from the PDR. Our observations with a vertical resolution of 15 m of liquid structures embedded in ice clouds are in agreement with previous field measurements (Rangno and Hobbs, 2001; Korolev and Isaac, 2003) which suggested that different pockets of solely water or ice in mixed-phase regions coexist with a typical scale of tens of meters. In contrast, large-scale models erroneously assume that liquid and ice phases are uniformly mixed within each model grid box (Tan and Storelvmo, 2016), with implications on the efficiency of the Wegener–Bergeron–Findeisen effect (Beesley and Moritz, 1999; Tan and Storelvmo, 2019).
The clouds are not formed over the site but over the ocean. They are then transported with a vertical gradient of the horizontal wind that generates some of the noticeable structures, such as the comma-like configuration between 00:00 and 04:00 on 17 May (Fig. 5). Between 05:00 and 07:00 LT, a cloud layer composed of supercooled droplets can be observed between 2 and 2.5 km a.m.s.l. In this case, βapp is higher than 10 with a very low VDR. We also note the probable presence of supercooled liquid droplets on 16 May between 18:30 and 19:30 LT around 2.5 km a.m.s.l. Estimating the properties of ice crystals therefore requires selecting profiles outside supercooled water pockets.
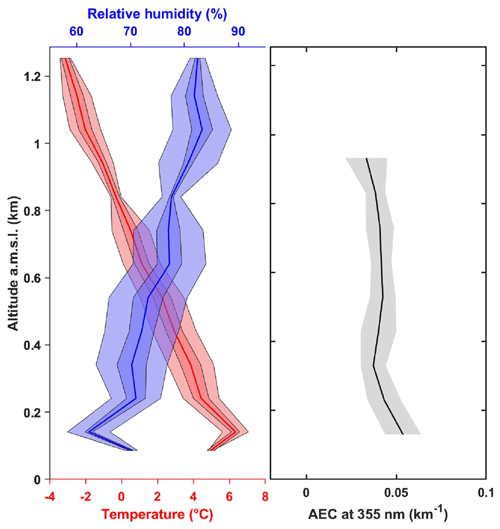
Figure 4Vertical profiles of the temperature, relative humidity, and aerosol extinction coefficient (AEC) derived from the ultralight on 16 May 2016, 11:30 LT. The lighter-colored areas (red and blue) give the data variability in 100 m-thick atmospheric layers. The darker-colored areas give the error on temperature (red) and relative humidity (blue). The gray shaded area is the error on the AEC.
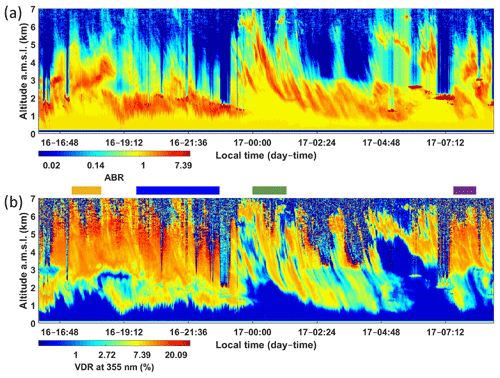
Figure 5Time series of the (a) apparent backscatter ratio (ABR) and (b) linear volume depolarization ratio (VDR) derived from the ground-based lidar from 16 to 17 May 2016. Cases studied specifically are highlighted by the color bar above each period concerned: case 1 in orange, case 2 in blue, case 3 in green, and case 4 in violet.
4.3 Lidar-derived optical properties
We selected four cases indicated by the horizontal-colored bands in Fig. 5, when the laser beam passed through ice clouds. For each selected period we averaged the profiles over the previous time intervals to increase the signal-to-noise ratio of the lidar measurements. The lidar-derived integrated optical parameters of sampled clouds are given in Table 1 for each case. The optical properties assume a contribution from multiple scattering which is evaluated later. The consistency of the retrievals can be assessed by comparing COTe derived from both elastic and N2-Raman channels, which are two independent measurements. This ensures that the assumptions for molecular scattering before and after the cloud are reasonable. The difference may be ascribed to mainly two reasons: (i) the level of noise above the cloud layer that affects the boundary condition or (ii) the smaller range of the N2-Raman channel that therefore integrates less of the upper part of the clouds. In Table 1, the “Klett” columns give the result of the Klett (1981) inversion using calculated via the N2-Raman (Eq. 10) channel. The differences observed with the Klett inversion remain lower than 3 %. Note that the stable Klett inversion significantly reduces the errors as one moves away from the top of the cloud layer. The value of derived from the N2-Raman channel is shown to be very stable from one case to another (between 21 and 25 sr).
In the following, we therefore use the coupling between the elastic and N2-Raman channels to determine the vertical profiles of the LR and the vertical profiles of the extinction coefficient of ice crystals. For the four cases, the vertical profiles derived from the lidar measurements are shown in Fig. 6. are mainly between 10 and 30 sr for the cloudy structures. The higher values observed in the lowest layers are related to the transition with the atmospheric boundary layer where there can be a contribution of aerosols when the cloud structures have a very low density. ICDR is retrieved between 5 % and 15 % in the clouds, mostly below 10 %, as shown in Fig. 7 for the four cases. Such values are weak for large non-spherical ice crystals. We can therefore infer that the clouds observed are composed of small ice crystals with a rather spherical shape. It is worth noting that Nakoudi et al. (2021) found ICDR values between 10 % and 35 % for cirrus clouds over Svalbard.
Assuming that the retrieved optical properties are slightly influenced by multiple scattering and considering the processes presented in Sect. 3, we can constrain the size distribution of the ice crystals. The phase functions calculated using a Mie model coupled to the extinction profile then allow us to evaluate the importance of multiple scattering using a Monte Carlo model. The retrieved values of η remain above 0.95 for all cases and mainly influence the top part of the clouds. We can therefore make the reasonable assumption that multiple scattering has negligible influence on our results. Note that the derived values are close to those previously determined from ground-based lidar measurements for the same type of clouds in the Arctic region () by Mariage et al. (2017).
Table 1Equivalent integrated optical parameters at 355 nm and their uncertainties for four case studies of cloud layers: effective optical thickness (COTe) from the elastic and N2-Raman channels, and equivalent effective lidar ratio (). The “Klett” column shows results using the Klett (1981) inversion. Altitude ranges where calculations are made are given in parentheses.
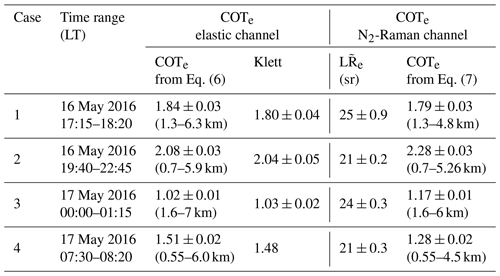
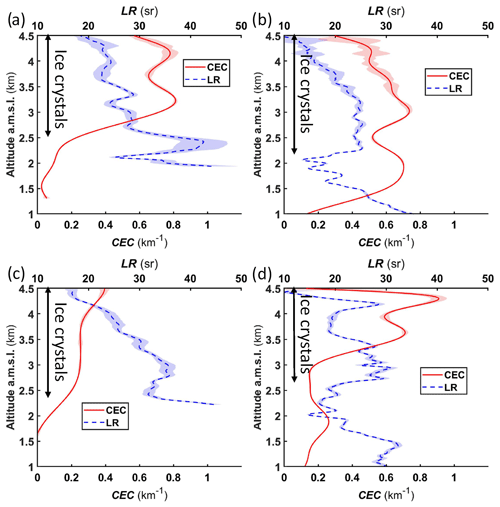
Figure 6Vertical profiles of the lidar-derived cloud extinction coefficient (CEC) and lidar ratio (LR) on (a) 16 May 2016, 17:15–18:20 local time (LT) (case 1), (b) 16 May 2016, 19:40–22:45 LT (case 2), (c) 17 May 2016, 00:00–01:15 LT (case 3), and (d) 17 May 2016, 07:30–08:20 LT (case 4). The altitude location of ice crystals is indicated. The colored areas around the curves represent the data uncertainties.
The vertical profiles of the effective radius reff and ice water content calculated as in Sect. 3 are shown in Fig. 8. Two values of νeff are considered (0.33 and 0.20) to evaluate potential ranges of reff and IWC (Fig. 8a, c, e, g). The effective radius reff of ice crystals presents rather small values, between 7 and 25 µm. The corresponding IWC is lower than 8 mg m−3, as it corresponds to small ice crystals and semi-transparent clouds. It can be noted that the value of νeff has little influence on reff and IWC because the backscatter phase functions do not change significantly (Fig. 2). The uncertainties on reff and IWC (Fig. 8b, d, f, h) are mainly due to the shot noise of the detection and have been calculated using a statistical error propagation. They are at most 2 µm and 0.65 mg m−3, respectively. The highest uncertainties are generally found at higher altitudes, where the lidar signal is most attenuated.
The values of reff are in the lowest range of those reported in the literature for the Arctic region by Mioche et al. (2017). They have analyzed the vertical distribution of microphysical properties, in most cases stratiform mixed-phase clouds in the boundary layer, using in situ measurements from four airborne spring campaigns in the European Arctic between 2004 and 2010. For these clouds of different nature in the southwestern regime, they showed that the ice phase dominates the microphysical properties with mean values of ∼25 µm and less than 25 mg m−3 for effective radius and IWC, respectively. Their analysis also revealed that large values of the liquid water content and high concentrations of small droplets may be linked to polluted situations and air mass origins from the south, leading to the lower values of ice crystal size and IWC ∼10 mg m−3. Our results are therefore consistent with airborne measurements on the same cloud types. Note that during PARCS, measurements were carried out over the coast of northern Norway, where the influence of local sources of pollution was not negligible (gas flaring from the Melkoya processing facility, presence of shipping activities close to Hammerfest, and transport of anthropogenic pollution from Russia) and when a plume containing biomass burning aerosols from huge forest fires in Canada reached Scandinavia (Chazette et al., 2018). The small values of ice crystal sizes and IWC found in our study may be explained by such polluted situations in comparison with clouds sampled in more pristine conditions in the high Arctic.
Similarly, McFarquhar et al. (2007) investigated the microphysical properties of single-layer stratus clouds over Barrow and Oliktok points in Alaska as part of the M-PACE (Mixed-Phase Arctic Cloud Experiment (Verlinde et al., 2007) campaign in fall 2004. They found that the effective radius of ice crystals was 25.2±3.9 µm and nearly independent of the normalized cloud altitude. Korolev and Isaac (2003) investigated mixed-phase clouds associated with frontal systems. They found that the mean volume radius of particles in ice clouds varied between 10 and 17.5 µm. Between −50 and −30 ∘C where all cloud particles were presumably ice, the effective radius was found to be about 7 µm which is of similar magnitude to our retrievals despite higher temperatures. They argued that IWC in glaciated clouds decreased with decreasing temperature, from about 100 mg m−3 at −5 ∘C to 20 mg m−3 at −35 ∘C. Our observations shown in Fig. 7 do not show any evidence of such behavior for clouds encountered during PARCS. The vertical profile of IWC cannot be explained solely by the temperature values but may also be ascribed to the history of the air masses.
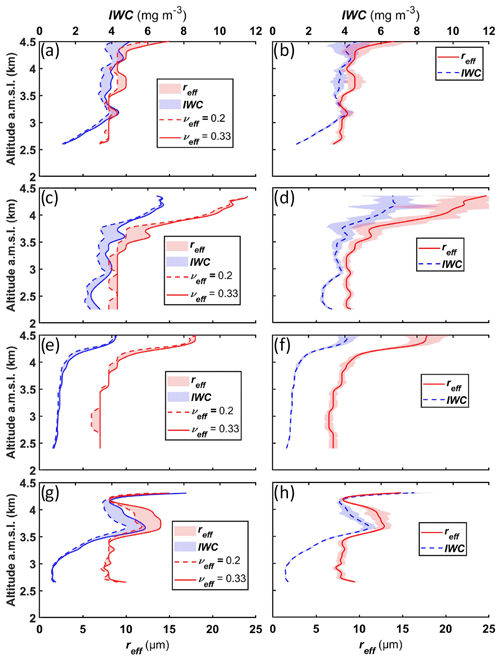
Figure 8Vertical profiles of effective radius (reff) of ice crystal and ice water content (IWC) on (a, b) 16 May 2016, 17:15–18:20 LT (case 1), (c, d) 16 May 2016, 19:40–22:45 LT (case 2), (e, f) 17 May 2016, 00:00–01:15 LT (case 3), and (g, h) 17 May 2016, 07:30–08:20 LT (case 4). The left panels (a, c, e, g) represent the bias that may be linked to the choice of the νeff value, and the right panels (b, d, f, h) represent the shaded areas associated with the lidar measurements assuming a νeff value between 0.2 and 0.33.
Thin ice clouds were sampled by ground-based lidar in late spring 2016 over the Hammerfest area in northern Norway. In the presence of semi-transparent clouds with an optical thickness lower than 2 at the wavelength of 355 nm, ground-based lidar measurements allow us to differentiate the contributions of ice crystals and liquid water pockets embedded in the cloud. The clouds are located just above the atmospheric boundary layer, with a cloud base between 0.8 and 1.2 km a.m.s.l. where the temperature is below the 0 ∘C isotherm. The inversion of the lidar profiles shows a modest level of depolarization, of the order of 10 %, with a negligible multiple scattering coefficient (<0.95 at the cloud top), suggesting that sampled ice crystals are small and of rather spherical shape. This agrees with Mie computations determining effective radii between ∼7 and 25 µm. The ice water contents are found to be lower than 8 mg m−3. Such small values may be ascribed to more polluted situations compared with pristine conditions in the high Arctic. It is worth noting that the uncertainties on the effective radius and ice water content are on average of 2 µm and 0.65 mg m−3, respectively, allowing us to follow their evolution in the cloud, as long as the lidar signal is not too attenuated.
The use of vibrational Raman measurements to constrain the elastic lidar equation allows us to remove ambiguities on the restitution of optical properties of semi-transparent ice clouds. It is an alternative approach to airborne measurements limited by the ability to sample the clouds over long periods of time. It is complementary to approaches proposing the coupling of lidar and radar measurements at 95 GHz. Its limitation is mainly on the ability of the lidar to sample the cloud layer along the line of sight and on the assumptions of sphericity of ice crystals, which have been justified by the observed values of the depolarization ratio. Our approach can nevertheless be easily extended to clouds containing non-spherical ice crystals if we consider the appropriate phase functions, which can be obtained, for instance, using T-matrix approaches applied on specific shape functions.
Data from the PARCS Hammerfest campaign can be downloaded from the https://www4.obs-mip.fr/parcs/database/ (Raut and Chazette, 2023) database upon request to the first author of the paper.
Both authors conceived and participated in the experiment, and contributed to the analysis of the lidar data, the conception of the study, and the writing of the manuscript.
The contact author has declared that neither of the authors has any competing interests.
Publisher's note: Copernicus Publications remains neutral with regard to jurisdictional claims made in the text, published maps, institutional affiliations, or any other geographical representation in this paper. While Copernicus Publications makes every effort to include appropriate place names, the final responsibility lies with the authors.
We thank Xiaoxia Shang, Julien Totems, Yoann Chazette, Nathalie Toussaint, and Sébastien Blanchon for their help during the field experiment. The ULA flights were performed by Franck Toussaint. The Avinor crew of Hammerfest Airport, represented by Hans-Petter Nergård, and the Air Création company are acknowledged for their hospitality. Kathy Law is acknowledged for securing the funding of the Pollution in the ARCtic System campaign. Computer analyses benefited from access to IDRIS HPC resources (GENCI allocations A011017141 and A013017141) and the IPSL mesoscale computing center.
This work was supported by the Institut National des Sciences de l'Univers (INSU) of the Centre National de la Recherche Scientifique (CNRS) via the French Arctic Initiative and the Commissariat à l'Énergie Atomique et aux Énergies Alternatives (CEA).
This paper was edited by Linlu Mei and reviewed by two anonymous referees.
Beesley, J. A. and Moritz, R. E.: Toward an explanation of the annual cycle of cloudiness over the Arctic Ocean, J. Climate, 12, 395–415, https://doi.org/10.1175/1520-0442(1999)012<0395:TAEOTA>2.0.CO;2, 1999.
Berthier, S., Chazette, P., Couvert, P., Pelon, J., Dulac, F., Thieuleux, F., Moulin, C., and Pain, T.: Desert dust aerosol columnar properties over ocean and continental Africa from Lidar in-Space Technology Experiment (LITE) and Meteosat synergy, J. Geophys. Res., 111, D21202, https://doi.org/10.1029/2005JD006999, 2006.
Berthier, S., Chazette, P., Pelon, J., and Baum, B.: Comparison of cloud statistics from spaceborne lidar systems, Atmos. Chem. Phys., 8, 6965–6977, https://doi.org/10.5194/acp-8-6965-2008, 2008.
Chazette, P., Bocquet, M., Royer, P., Winiarek, V., Raut, J.-C., Labazuy, P., Gouhier, M., Lardier, M., and Cariou, J.-P.: Eyjafjallajökull ash concentrations derived from both lidar and modeling, J. Geophys. Res.-Atmos., 117, D00U14, https://doi.org/10.1029/2011JD015755, 2012.
Chazette, P., Totems, J., Ancellet, G., Pelon, J., and Sicard, M.: Temporal consistency of lidar observations during aerosol transport events in the framework of the ChArMEx/ADRIMED campaign at Minorca in June 2013, Atmos. Chem. Phys., 16, 2863–2875, https://doi.org/10.5194/acp-16-2863-2016, 2016.
Chazette, P., Raut, J.-C., and Totems, J.: Springtime aerosol load as observed from ground-based and airborne lidars over northern Norway, Atmos. Chem. Phys., 18, 13075–13095, https://doi.org/10.5194/acp-18-13075-2018, 2018.
Chazette, P., Baron, A., and Flamant, C.: Mesoscale spatio-temporal variability of airborne lidar-derived aerosol properties in the Barbados region during EUREC4A, Atmos. Chem. Phys., 22, 1271–1292, https://doi.org/10.5194/acp-22-1271-2022, 2022.
Collis, R. T. H. and Russel, P. B.: Lidar measurement of particles and gases by elastic backscattering and differential absorption in Laser Monitoring of the Atmosphere, edited by: Hinkley, E. D., Springer Berlin Heidelberg, Berlin, Heidelberg, 71–152 pp., https://doi.org/10.1007/3-540-07743-X, 1976.
Curry, J. A. and Ebert, E. E.: Annual cycle of radiation fluxes over the Arctic Ocean: sensitivity to cloud optical properties, J. Climate, 5, 1267–1280, https://doi.org/10.1175/1520-0442(1992)005<1267:ACORFO>2.0.CO;2, 1992.
Delanoë, J. and Hogan, R. J.: Combined CloudSat-CALIPSO-MODIS retrievals of the properties of ice clouds, J. Geophys. Res.-Atmos., 115, 1–17, https://doi.org/10.1029/2009JD012346, 2010.
Di Biagio, C., Pelon, J., Blanchard, Y., Loyer, L., Hudson, S. R., Walden, V. P., Raut, J. C., Kato, S., Mariage, V., and Granskog, M. A.: Toward a Better Surface Radiation Budget Analysis Over Sea Ice in the High Arctic Ocean: A Comparative Study Between Satellite, Reanalysis, and local-scale Observations, J. Geophys. Res.-Atmos., 126, e2020JD032555, https://doi.org/10.1029/2020JD032555, 2021.
Ehrlich, A., Wendisch, M., Lüpkes, C., Buschmann, M., Bozem, H., Chechin, D., Clemen, H.-C., Dupuy, R., Eppers, O., Hartmann, J., Herber, A., Jäkel, E., Järvinen, E., Jourdan, O., Kästner, U., Kliesch, L.-L., Köllner, F., Mech, M., Mertes, S., Neuber, R., Ruiz-Donoso, E., Schnaiter, M., Schneider, J., Stapf, J., and Zanatta, M.: A comprehensive in situ and remote sensing data set from the Arctic CLoud Observations Using airborne measurements during polar Day (ACLOUD) campaign, Earth Syst. Sci. Data, 11, 1853–1881, https://doi.org/10.5194/essd-11-1853-2019, 2019.
Fernández-González, S., Valero, F., Sánchez, J. L., Gascón, E., López, L., García-Ortega, E., and Merino, A.: Analysis of a seeder-feeder and freezing drizzle event, J. Geophys. Res., 120, 3984–3999, https://doi.org/10.1002/2014JD022916, 2015.
Hansen, J., Ruedy, R., Sato, M., and Lo, K.: Global surface temperature change, Rev. Geophys., 48, RG4004, https://doi.org/10.1029/2010RG000345, 2010.
Hansen, J. E. and Travis, L. D.: Light scattering in planetary atmospheres, Space Sci. Rev., 16, 527–610, https://doi.org/10.1007/BF00168069, 1974.
Harrington, J. Y. and Olsson, P. Q.: A method for the parameterization of cloud optical properites in bulk and bin microphysical models. Implications for arctic cloudy boundary layers, Atmos. Res., 57, 51–80, https://doi.org/10.1016/S0169-8095(00)00068-5, 2001.
Harrington, J. Y., Reisin, T., Cotton, W. R., and Kreidenweis, S. M.: Cloud resolving simulations of Arctic stratus part II: Transition-season clouds, Atmos. Res., 51, 45–75, https://doi.org/10.1016/S0169-8095(98)00098-2, 1999.
Hoffmann, A., Ritter, C., Stock, M., Shiobara, M., Lampert, A., Maturilli, M., Orgis, T., Neuber, R., and Herber, A.: Ground-based lidar measurements from Ny-Ålesund during ASTAR 2007, Atmos. Chem. Phys., 9, 9059–9081, https://doi.org/10.5194/acp-9-9059-2009, 2009.
Hogan, R. J.: Fast approximate calculation of multiply scattered lidar returns, Appl. Optics, 45, 5984–5992, https://doi.org/10.1364/AO.45.005984, 2006.
Kalesse, H., de Boer, G., Solomon, A., Oue, M., Ahlgrimm, M., Zhang, D., Shupe, M. D., Luke, E., and Protat, A.: Understanding rapid changes in phase partitioning between cloud liquid and ice in stratiform mixed-phase clouds: An arctic case study, Mon. Weather Rev., 144, 4805–4826, https://doi.org/10.1175/MWR-D-16-0155.1, 2016.
Kay, J. E. and Gettelman, A.: Cloud influence on and response to seasonal Arctic sea ice loss, J. Geophys. Res.-Atmos., 114, D18204, https://doi.org/10.1029/2009JD011773, 2009.
Klaus, D., Dethloff, K., Dorn, W., Rinke, A., and Wu, D. L.: New insight of Arctic cloud parameterization from regional climate model simulations, satellite-based, and drifting station data, Geophys. Res. Lett., 43, 5450–5459, https://doi.org/10.1002/2015GL067530, 2016.
Klein, S. A., McCoy, R. B., Morrison, H., Ackerman, A. S., Avramov, A., Boer, G. de, Chen, M., Cole, J. N. S., Del Genio, A. D., Falk, M., Foster, M. J., Fridlind, A., Golaz, J.-C., Hashino, T., Harrington, J. Y., Hoose, C., Khairoutdinov, M. F., Larson, V. E., Liu, X., Luo, Y., McFarquhar, G. M., Menon, S., Neggers, R. A. J., Park, S., Poellot, M. R., Schmidt, J. M., Sednev, I., Shipway, B. J., Shupe, M. D., Spangenberg, D. A., Sud, Y. C., Turner, D. D., Veron, D. E., Salzen, K. von, Walker, G. K., Wang, Z., Wolf, A. B., Xie, S., Xu, K.-M., Yang, F., and Zhang, G.: Intercomparison of model simulations of mixed-phase clouds observed during the ARM Mixed-Phase Arctic Cloud Experiment. I: single-layer cloud, Q. J. Roy. Meteor. Soc., 135, 979–1002, https://doi.org/10.1002/qj.416, 2009.
Klett, J. D.: Stable analytical inversion solution for processing lidar returns, Appl. Optics, 20, 211–220, https://doi.org/10.1364/AO.20.000211, 1981.
Koenigk, T., Brodeau, L., Graversen, R. G., Karlsson, J., Svensson, G., Tjernström, M., Willén, U., and Wyser, K.: Arctic climate change in 21st century CMIP5 simulations with EC-Earth, Clim. Dynam., 40, 2719–2743, https://doi.org/10.1007/s00382-012-1505-y, 2013.
Korolev, A. and Isaac, G.: Phase transformation of mixed-phase clouds, Q. J. Roy. Meteor. Soc., 129, 19–38, https://doi.org/10.1256/qj.01.203, 2003.
Lampert, A., Ehrlich, A., Dörnbrack, A., Jourdan, O., Gayet, J.-F., Mioche, G., Shcherbakov, V., Ritter, C., and Wendisch, M.: Microphysical and radiative characterization of a subvisible midlevel Arctic ice cloud by airborne observations – a case study, Atmos. Chem. Phys., 9, 2647–2661, https://doi.org/10.5194/acp-9-2647-2009, 2009.
Liu, Y. and Key, J. R.: Less winter cloud aids summer 2013 Arctic sea ice return from 2012 minimum, Environ. Res. Lett., 9, 044002, https://doi.org/10.1088/1748-9326/9/4/044002, 2014.
Liu, Y., Key, J. R., Ackerman, S. A., Mace, G. G., and Zhang, Q.: Arctic cloud macrophysical characteristics from CloudSat and CALIPSO, Remote Sens. Environ., 124, 159–173, https://doi.org/10.1016/j.rse.2012.05.006, 2012.
Luke, E. P., Yang, F., Kollias, P., Vogelmann, A. M., and Maahn, M.: New insights into ice multiplication using remote-sensing observations of slightly supercooled mixed-phase clouds in the Arctic, P. Natl. Acad. Sci. USA, 118, e2021387118, https://doi.org/10.1073/pnas.2021387118, 2021.
Maillard, J., Ravetta, F., Raut, J.-C., Mariage, V., and Pelon, J.: Characterisation and surface radiative impact of Arctic low clouds from the IAOOS field experiment, Atmos. Chem. Phys., 21, 4079–4101, https://doi.org/10.5194/acp-21-4079-2021, 2021.
Mariage, V., Pelon, J., Blouzon, F., Victori, S., Geyskens, N., Amarouche, N., Drezen, C., Guillot, A., Calzas, M., Garracio, M., Wegmuller, N., Sennéchael, N., and Provost, C.: IAOOS microlidar-on-buoy development and first atmospheric observations obtained during 2014 and 2015 arctic drifts, Opt. Express, 25, A73, https://doi.org/10.1364/oe.25.000a73, 2017.
McFarquhar, G. M., Zhang, G., Poellot, M. R., Kok, G. L., McCoy, R., Tooman, T., Fridlind, A., and Heymsfield, A. J.: Ice properties of single-layer stratocumulus during the Mixed-Phase Arctic Cloud Experiment: 1. Observations, J. Geophys. Res.-Atmos., 112, D24201, https://doi.org/10.1029/2007JD008633, 2007.
McFarquhar, G. M., Baumgardner, D., Bansemer, A., Abel, S. J., Crosier, J., French, J., Rosenberg, P., Korolev, A., Schwarzoenboeck, A., Leroy, D., Um, J., Wu, W., Heymsfield, A. J., Twohy, C., Detwiler, A., Field, P., Neumann, A., Cotton, R., Axisa, D., and Dong, J.: Processing of Ice Cloud In Situ Data Collected by Bulk Water, Scattering, and Imaging Probes: Fundamentals, Uncertainties, and Efforts toward Consistency, Meteorol. Monogr., 58, 11.1–11.33, https://doi.org/10.1175/amsmonographs-d-16-0007.1, 2017.
Mech, M., Ehrlich, A., Herber, A., Lüpkes, C., Wendisch, M., Becker, S., Boose, Y., Chechin, D., Crewell, S., Dupuy, R., Gourbeyre, C., Hartmann, J., Jäkel, E., Jourdan, O., Kliesch, L. L., Klingebiel, M., Kulla, B. S., Mioche, G., Moser, M., Risse, N., Ruiz-Donoso, E., Schäfer, M., Stapf, J., and Voigt, C.: MOSAiC-ACA and AFLUX – Arctic airborne campaigns characterizing the exit area of MOSAiC, Sci. Data, 9, 790, https://doi.org/10.1038/s41597-022-01900-7, 2022.
Milbrandt, J. A. and Yau, M. K.: A multimoment bulk microphysics parameterization. Part II: A proposed three-moment closure and scheme description, J. Atmos. Sci., 62, 3065–3081, https://doi.org/10.1175/JAS3535.1, 2005.
Mioche, G., Jourdan, O., Delanoë, J., Gourbeyre, C., Febvre, G., Dupuy, R., Monier, M., Szczap, F., Schwarzenboeck, A., and Gayet, J.-F.: Vertical distribution of microphysical properties of Arctic springtime low-level mixed-phase clouds over the Greenland and Norwegian seas, Atmos. Chem. Phys., 17, 12845–12869, https://doi.org/10.5194/acp-17-12845-2017, 2017.
Morrison, H. and Gettelman, A.: A new two-moment bulk stratiform cloud microphysics scheme in the community atmosphere model, version 3 (CAM3). Part I: Description and numerical tests, J. Climate, 21, 3642–3659, https://doi.org/10.1175/2008JCLI2105.1, 2008.
Morrison, H., Curry, J. A., and Khvorostyanov, V. I.: A new double-moment microphysics parameterization for application in cloud and climate models. Part I: Description, J. Atmos. Sci., 62, 1665–1677, https://doi.org/10.1175/JAS3446.1, 2005.
Morrison, H., De Boer, G., Feingold, G., Harrington, J., Shupe, M. D., and Sulia, K.: Resilience of persistent Arctic mixed-phase clouds, J. Climate, 21, 3642–3659, https://doi.org/10.1038/ngeo1332, 2012.
Nakoudi, K., Ritter, C., and Stachlewska, I. S.: Properties of cirrus clouds over the european arctic (Ny-Ålesund, svalbard), Remote Sens., 13, 4555, https://doi.org/10.3390/rs13224555, 2021.
Platt, C. M. R.: Lidar Observation of a Mixed-Phase Altostratus Cloud, J. Appl. Meteorol., 16, 339–345, https://doi.org/10.1175/1520-0450(1977)016<0339:looamp>2.0.co;2, 1977.
Platt, C. M. R.: Remote sounding of high clouds. III: Monte Carlo calculations of multiple-scattered lidar returns., J. Atmos. Sci., 38, 156–167, https://doi.org/10.1175/1520-0469(1981)038<0156:RSOHCI>2.0.CO;2, 1981.
Rangno, A. L. and Hobbs, P. V.: Ice particles in stratiform clouds in the Arctic and possible mechanisms for the production of high ice concentrations, J. Geophys. Res.-Atmos., 106, 15065–15075, https://doi.org/10.1029/2000JD900286, 2001.
Raut, J.-C. and Chazette, P.: PARCS, WALI lidar, PARCS [data set], https://www4.obs-mip.fr/parcs/, last access: 27 November 2023.
Sassen, K.: The polarization lidar technique for cloud research: a review and current assessment, B. Am. Meteorol. Soc., 72, 1848–1866, https://doi.org/10.1175/1520-0477(1991)072<1848:TPLTFC>2.0.CO;2, 1991.
Schweiger, A. J., Lindsay, R. W., Vavrus, S., and Francis, J. A.: Relationships between Arctic sea ice and clouds during autumn, J. Climate, 21, 4799–4810, https://doi.org/10.1175/2008JCLI2156.1, 2008.
Serreze, M. C. and Barry, R. G.: Processes and impacts of Arctic amplification: A research synthesis, Glob. Planet. Change, 77, 85–96, https://doi.org/10.1016/j.gloplacha.2011.03.004, 2011.
Serreze, M. C., Barrett, A. P., Stroeve, J. C., Kindig, D. N., and Holland, M. M.: The emergence of surface-based Arctic amplification, The Cryosphere, 3, 11–19, https://doi.org/10.5194/tc-3-11-2009, 2009.
Shang, X. and Chazette, P.: End-to-End Simulation for a Forest-Dedicated Full-Waveform Lidar onboard a Satellite Initialized from UV Airborne Lidar Experiments, Remote Sens., 7, 5222–5255, https://doi.org/10.3390/rs70505222, 2015.
Shupe, M. D., Kollias, P., Poellot, M., and Eloranta, E.: On deriving vertical air motions from cloud radar doppler spectra, J. Atmos. Ocean. Tech., 25, 547–557, https://doi.org/10.1175/2007JTECHA1007.1, 2008.
Shupe, M. D., Walden, V. P., Eloranta, E., Uttal, T., Campbell, J. R., Starkweather, S. M., and Shiobara, M.: Clouds at Arctic atmospheric observatories. Part I: Occurrence and macrophysical properties, J. Appl. Meteorol. Climatol., 50, 626–644, https://doi.org/10.1175/2010JAMC2467.1, 2011.
Shupe, M. D., Rex, M., Blomquist, B., G. Persson, P. O., Schmale, J., Uttal, T., Althausen, D., Angot, H., Archer, S., Bariteau, L., Beck, I., Bilberry, J., Bucci, S., Buck, C., Boyer, M., Brasseur, Z., Brooks, I. M., Calmer, R., Cassano, J., Castro, V., Chu, D., Costa, D., Cox, C. J., Creamean, J., Crewell, S., Dahlke, S., Damm, E., de Boer, G., Deckelmann, H., Dethloff, K., Dütsch, M., Ebell, K., Ehrlich, A., Ellis, J., Engelmann, R., Fong, A. A., Frey, M. M., Gallagher, M. R., Ganzeveld, L., Gradinger, R., Graeser, J., Greenamyer, V., Griesche, H., Griffiths, S., Hamilton, J., Heinemann, G., Helmig, D., Herber, A., Heuzé, C., Hofer, J., Houchens, T., Howard, D., Inoue, J., Jacobi, H. W., Jaiser, R., Jokinen, T., Jourdan, O., Jozef, G., King, W., Kirchgaessner, A., Klingebiel, M., Krassovski, M., Krumpen, T., Lampert, A., Landing, W., Laurila, T., Lawrence, D., Lonardi, M., Loose, B., Lüpkes, C., Maahn, M., Macke, A., Maslowski, W., Marsay, C., Maturilli, M., Mech, M., Morris, S., Moser, M., Nicolaus, M., Ortega, P., Osborn, J., Pätzold, F., Perovich, D. K., Petäjä, T., Pilz, C., Pirazzini, R., Posman, K., Powers, H., Pratt, K. A., Preußer, A., Quéléver, L., Radenz, M., Rabe, B., Rinke, A., Sachs, T., Schulz, A., Siebert, H., Silva, T., Solomon, A., Sommerfeld, A., Spreen, G., Stephens, M., Stohl, A., Svensson, G., Uin, J., Viegas, J., Voigt, C., von der Gathen, P., Wehner, B., Welker, J.M. Wendisch, M. Werner, M. Xie, Z., and Yue, F.: Overview of the MOSAiC expedition: Atmosphere, Elementa: Science of the Anthropocene, 10, 00060, https://doi.org/10.1525/elementa.2021.00060, 2022.
Stephens, G. L., Tsay, S.-C., Stackhouse, P. W., and Flatau, P. J.: The Relevance of the Microphysical and Radiative Properties of Cirrus Clouds to Climate and Climatic Feedback, J. Atmos. Sci., 47, 1742–1753, https://doi.org/10.1175/1520-0469(1990)047<1742:TROTMA>2.0.CO;2, 1990.
Tan, I. and Storelvmo, T.: Sensitivity study on the influence of cloud microphysical parameters on mixed-phase cloud thermodynamic phase partitioning in CAM5, J. Atmos. Sci., 73, 709–728, https://doi.org/10.1175/JAS-D-15-0152.1, 2016.
Tan, I. and Storelvmo, T.: Evidence of Strong Contributions From Mixed-Phase Clouds to Arctic Climate Change, Geophys. Res. Lett., 46, 2894–2902, https://doi.org/10.1029/2018GL081871, 2019.
Taylor, P. C., Boeke, R. C., Li, Y., and Thompson, D. W. J.: Arctic cloud annual cycle biases in climate models, Atmos. Chem. Phys., 19, 8759–8782, https://doi.org/10.5194/acp-19-8759-2019, 2019.
Thompson, G., Field, P. R., Rasmussen, R. M., and Hall, W. D.: Explicit forecasts of winter precipitation using an improved bulk microphysics scheme. Part II: Implementation of a new snow parameterization, Mon. Weather Rev., 136, 5095–5115, https://doi.org/10.1175/2008MWR2387.1, 2008.
Totems, J., Chazette, P., and Raut, J. J.-C.: Accuracy of current Arctic springtime water vapour estimates, assessed by Raman lidar, Q. J. Roy. Meteor. Soc., 145, 1234–1249, https://doi.org/10.1002/qj.3492, 2019.
Verlinde, J., Harrington, J. Y., McFarquhar, G. M., Yannuzzi, V. T., Avramov, A., Greenberg, S., Johnson, N., Zhang, G., Poellot, M. R., Mather, J. H., Turner, D. D., Eloranta, E. W., Zak, B. D., Prenni, A. J., Daniel, J. S., Kok, G. L., Tobin, D. C., Holz, R., Sassen, K., Spangenberg, D., Minnis, P., Tooman, T. P., Ivey, M. D., Richardson, S. J., Bahrmann, C. P., Shupe, M., DeMott, P. J., Heymsfield, A. J., and Schofield, R.: The mixed-phase arctic cloud experiment, B. Am. Meteorol. Soc., 88, 205–221, https://doi.org/10.1175/BAMS-88-2-205, 2007.
Warren, S. G. and Brandt, R. E.: Optical constants of ice from the ultraviolet to the microwave: A revised compilation, J. Geophys. Res.-Atmos., 113, D14220, https://doi.org/10.1029/2007JD009744, 2008.
Wendisch, M., MacKe, A., Ehrlich, A., LüPkes, C., Mech, M., Chechin, D., Dethloff, K., Velasco, C. B., Bozem, H., BrüCkner, M., Clemen, H. C., Crewell, S., Donth, T., Dupuy, R., Ebell, K., Egerer, U., Engelmann, R., Engler, C., Eppers, O., Gehrmann, M., Gong, X., Gottschalk, M., Gourbeyre, C., Griesche, H., Hartmann, J., Hartmann, M., Heinold, B., Herber, A., Herrmann, H., Heygster, G., Hoor, P., Jafariserajehlou, S., JäKel, E., JäRvinen, E., Jourdan, O., KäStner, U., Kecorius, S., Knudsen, E. M., KöLlner, F., Kretzschmar, J., Lelli, L., Leroy, D., Maturilli, M., Mei, L., Mertes, S., Mioche, G., Neuber, R., Nicolaus, M., Nomokonova, T., Notholt, J., Palm, M., Van Pinxteren, M., Quaas, J., Richter, P., Ruiz-Donoso, E., SchäFer, M., Schmieder, K., Schnaiter, M., Schneider, J., SchwarzenböCk, A., Seifert, P., Shupe, M. D., Siebert, H., Spreen, G., Stapf, J., Stratmann, F., Vogl, T., Welti, A., Wex, H., Wiedensohler, A., Zanatta, M., and Zeppenfeld, A. S.: The arctic cloud puzzle using acloud/pascal multiplatform observations to unravel the role of clouds and aerosol particles in arctic amplification, B. Am. Meteorol. Soc., 100, 841–871, https://doi.org/10.1175/BAMS-D-18-0072.1, 2019.
Wiegner, M., Oppel, U., Krasting, H., Renger, W., Kiemle, C., and Wirth, M.: Cirrus Measurements from a Spaceborne Lidar: Influence of Multiple Scattering, in: Advances in Atmospheric Remote Sensing with Lidar, Springer, Berlin, Heidelberg, 189–192, https://doi.org/10.1007/978-3-642-60612-0_47, 1997.
Yu, Y., Taylor, P. C., and Cai, M.: Seasonal Variations of Arctic Low-Level Clouds and Its Linkage to Sea Ice Seasonal Variations, J. Geophys. Res.-Atmos., 124, 12206–12226, https://doi.org/10.1029/2019JD031014, 2019.
Zhang, M. H., Cess, R. D., and Xie, S. C.: Relationship between cloud radiative forcing and sea surface temperatures over the entire tropical oceans, J. Climate, 9, 1374–1384, https://doi.org/10.1175/1520-0442(1996)009<1374:RBCRFA>2.0.CO;2, 1996.
- Abstract
- Introduction
- Ground-based lidar observations
- Method for the determination of ice water content and ice crystal effective radius
- Lidar sampling
- Vertical profile of cloud microphysical properties
- Conclusion
- Data availability
- Author contributions
- Competing interests
- Disclaimer
- Acknowledgements
- Financial support
- Review statement
- References
- Abstract
- Introduction
- Ground-based lidar observations
- Method for the determination of ice water content and ice crystal effective radius
- Lidar sampling
- Vertical profile of cloud microphysical properties
- Conclusion
- Data availability
- Author contributions
- Competing interests
- Disclaimer
- Acknowledgements
- Financial support
- Review statement
- References