the Creative Commons Attribution 4.0 License.
the Creative Commons Attribution 4.0 License.
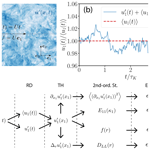
Estimating the turbulent kinetic energy dissipation rate from one-dimensional velocity measurements in time
Marcel Schröder
Tobias Bätge
Eberhard Bodenschatz
Michael Wilczek
Gholamhossein Bagheri
The turbulent kinetic energy dissipation rate is one of the most important quantities characterizing turbulence. Experimental studies of a turbulent flow in terms of the energy dissipation rate often rely on one-dimensional measurements of the flow velocity fluctuations in time. In this work, we first use direct numerical simulation of stationary homogeneous isotropic turbulence at Taylor-scale Reynolds numbers to evaluate different methods for inferring the energy dissipation rate from one-dimensional velocity time records. We systematically investigate the influence of the finite turbulence intensity and the misalignment between the mean flow direction and the measurement probe, and we derive analytical expressions for the errors associated with these parameters. We further investigate how statistical averaging for different time windows affects the results as a function of Rλ. The results are then combined with Max Planck Variable Density Turbulence Tunnel hot-wire measurements at to investigate flow conditions similar to those in the atmospheric boundary layer. Finally, practical guidelines for estimating the energy dissipation rate from one-dimensional atmospheric velocity records are given.
- Article
(7084 KB) - Full-text XML
- BibTeX
- EndNote
Turbulence is fundamental to many natural and engineering processes, such as transport of heat and moisture in the Earth's atmosphere (e.g., Wyngaard, 1992; Garratt, 1994; Muschinski and Lenschow, 2001; Fairall and Larsen, 1986; Hsieh and Katul, 1997), wind energy conversion (Smalikho et al., 2013), entrainment and mixing (e.g., Warhaft, 2000; Sreenivasan, 2004; Deshpande et al., 2009; Gerber et al., 2008, 2013; Siebert et al., 2013; Fodor and Mellado, 2020), and warm rain initiation (e.g., Shaw, 2003; Devenish et al., 2012; Pumir and Wilkinson, 2016; Li et al., 2020), to name just a few. In three-dimensional turbulence, the kinetic energy is typically injected into the flow at the largest scales and is successively transferred to smaller eddies by means of the direct energy cascade. At the smallest scales characterized by the Kolmogorov length scale (or the dissipation scale) ηK, kinetic energy is dissipated by viscous effects at the energy dissipation rate (a list of all the parameters and symbols used in this study can be found in Tables A1 and A2). The energy dissipation rate is one of the most fundamental quantities in turbulence and is used to estimate many relevant features of a turbulent flow, such as the Kolmogorov length scale ηK; the Taylor microscale λ; the Taylor-scale Reynolds number Rλ; and, by means of dimensional estimates, the energy injection scale.
The instantaneous energy dissipation field ϵ0(x,t), which is a function of the fluid kinematic viscosity ν and the velocity gradient tensor, is highly intermittent with strong small-scale fluctuations (Pope, 2000; Davidson, 2015, and references therein), which are at the core of the intermittency problem in turbulence (Sreenivasan and Antonia, 1997; Muschinski et al., 2004; Buaria et al., 2019). By “instantaneous” we want to emphasize here that ϵ0 is the energy dissipation rate at one point in space and time within the flow. It also plays an important role in turbulent mixing in reacting flows (e.g., Sreenivasan, 2004; Hamlington et al., 2012; Sreenivasan, 2019) or turbulence-induced rain initiation in warm clouds (Devenish et al., 2012). ϵ0(x,t), however, is extremely difficult to measure experimentally because it requires complete knowledge of the three-dimensional velocity field with spatial and temporal resolution that can resolve scales smaller than or at least comparable to the Kolmogorov scales.
Apart from the instantaneous dissipation field ϵ0(x,t), the energy dissipation in a turbulent flow can be statistically described by either the local or global mean energy dissipation rate, which are both important. Local volume averages of the instantaneous dissipation field 〈ϵ0〉R and related surrogates, e.g., diagonal or off-diagonal components of the velocity gradient, can capture intermittency of turbulence (Lefeuvre et al., 2014; Almalkie and de Bruyn Kops, 2012, and references therein). The local volume averages of the dissipation field converge to the global mean energy dissipation rate 〈ϵ〉 for statistically converged sampling. The mean dissipation rate 〈ϵ〉 can be used to parameterize the statistics of statistically homogeneous and locally isotropic turbulence based on Kolmogorov's phenomenology (K41) (Kolmogorov, 1941). Note that even if the global mean energy dissipation rate 〈ϵ〉 is known, it is also of high interest to know how locally averaged dissipation rates 〈ϵ0〉R differ from the global mean energy dissipation rate 〈ϵ〉. For example, the local dissipation rate determines whether droplets in a cloud behave as tracer or inertial particles, which in turn can affect the probability of collision and coalescence of the droplets and thus the likelihood of precipitation initiation (e.g., see Shaw, 2003).
For a statistically stationary homogeneous isotropic (SHI) turbulent flow, 〈ϵ〉 can be estimated from time-dependent single-point one-dimensional velocity measurements through different methods, such as longitudinal or transverse velocity gradients (Wyngaard and Clifford, 1977; Elsner and Elsner, 1996; Antonia, 2003; Siebert et al., 2006, among others), inertial-range scaling laws comprising the famous law (Kolmogorov, 1941, 1991; Muschinski et al., 2001), counting zero crossings of the velocity fluctuation time series (Sreenivasan et al., 1983; Wacławczyk et al., 2017), or dimensional arguments (e.g., Taylor, 1935; McComb et al., 2010; Vassilicos, 2015). These methods usually invoke Taylor's hypothesis to map temporal signals onto spatial signals, which requires a sufficiently small turbulence intensity. The turbulence intensity is defined as the ratio of the root mean square velocity fluctuations to the mean velocity U. When all of these criteria are met, single-point velocity measurements with hot-wire anemometers at a high temporal resolution have been shown to be suitable for accurately estimating the global energy dissipation rate (see also below) (Lewis et al., 2021; Sinhuber, 2015; Elsner and Elsner, 1996; Antonia, 2003; Frehlich et al., 2003). However, in atmospheric flows, the assumption of ideal stationary homogeneous isotropic turbulence needs to be considered very carefully, as, for example, thermals change in local weather conditions, and of course the diurnal cycle may lead to non-stationarity and inhomogeneity.
Then the global mean energy dissipation rate 〈ϵ〉 is not representative, as the turbulence can be highly time- and space-dependent even at the energy injection scales. As a result, one needs to calculate a local 〈ϵ0〉τ and 〈ϵ0〉R, respectively, based on velocity statistics for a properly chosen averaging window τ in time and R in space, which is short enough for resolving the temporal or spatial variations but also long enough to obtain statistically representative values with acceptable systematic and/or random errors (e.g., Wyngaard, 1992; Lenschow et al., 1994). Therefore, a conflict arises with respect to the averaging time between resolving small-scale features of a turbulent flow and statistical convergence under non-stationary and inhomogeneous conditions.
In the case of atmospheric flows, in situ measurements made via airborne (e.g., Malinowski et al., 2013; Siebert et al., 2006, 2013; Muschinski et al., 2004; Frehlich et al., 2004; Nowak et al., 2021; Dodson and Small Griswold, 2021) as well as ground-based (e.g., Chamecki and Dias, 2004; O'Connor et al., 2010; Risius et al., 2015; Siebert et al., 2015) platforms can typically only resolve the coarse-grained time series of the local mean energy dissipation rate 〈ϵ0〉τ. However, it remains unclear how large the errors in estimating the coarse-grained time series of the local mean energy dissipation rate are due to individual choices of the averaging window, since there is currently no high-resolution three-dimensional velocity measurement available during in situ measurements to serve as the ground truth. In the absence of a ground-truth reference, the comparison between different methods was used as the next best benchmark for validity of a given method (Siebert et al., 2010; Wacławczyk et al., 2020; Siebert et al., 2006; Risius et al., 2015; Wacławczyk et al., 2017), which in some cases makes the interpretation of the data difficult due to the large discrepancies between the estimates obtained by different methods. As an example, Wacławczyk et al. (2020) found deviations of about 5 %–50 % with respect to estimating the mean energy dissipation rate depending on the method and averaging windows using synthetic data modeled via a von Kármán spectrum. Another example is the work of Akinlabi et al. (2019), who found that estimates of the mean energy dissipation rate by one-dimensional longitudinal velocity can differ by a factor of 2 to 3 from those calculated using direct numerical simulations (DNSs), depending on the method used.
Our literature review indicates that a systematic investigation is still needed to fully understand how the choice of averaging window, analysis methods, turbulence intensity and large-scale random flow velocities can influence estimating the mean energy dissipation rate and its deviations from the instantaneous energy dissipation rate. To this end, we systematically benchmark different techniques available in the literature using fully resolved DNS of statistically stationary, homogeneous, isotropic turbulence. Since the full dissipation field is available from DNS, this approach provides a ground-truth reference for comparisons with various estimation techniques. To bridge the gap between typical Rλ of DNS and atmospheric flows, we use high-resolution measurements of the longitudinal velocity components of the Variable Density Turbulence Tunnel (VDTT) (Bodenschatz et al., 2014; Sinhuber, 2015; Küchler et al., 2019) at various Taylor-scale Reynolds numbers Rλ between 140 and 6000. The impact of turbulence intensity, large-scale random-sweeping velocities, the size of the averaging window, the Reynolds number and also possible experimental imperfections such as anemometer misalignment are investigated in detail. Our work aims to be a step towards the goal of extracting the time-dependent energy dissipation rate from non-ideal naturally occurring turbulent flows, mitigating the impact of non-ideal features of the flow, e.g., anisotropy or inhomogeneity. In Sect. 2, we first define the central statistical quantities and the individual methods for estimating the energy dissipation rate in detail. An analysis of the individual methods including discrepancies, errors due to finite turbulence intensity and alignment errors are discussed in Sect. 3 followed by a summary of our findings.
Suppose denotes the three-dimensional velocity vector of the turbulent flow, where is the position and t is the time. We assume that the streamwise direction of the global mean flow U is in the direction of e1 such that U=Ue1 is (by definition) constant in space and time. We refer to e1 as the longitudinal direction and the components normal to that, i.e., e2 and e3, as the transverse directions of the flow. As mentioned earlier, many experimental setups record only a one-dimensional flow velocity at one location and as a function of time. We consider this one-dimensional velocity time record to be in the longitudinal flow direction unless otherwise stated, e.g., when the probe misalignment is investigated. In the following, we first introduce different averaging principles that can be used to analyze turbulence statistics and Taylor's frozen hypothesis, and then we present the commonly used methods for extracting the energy dissipation rate. An introduction of the basic statistical description of turbulent flows is provided in the Appendix (Appendix A) for the sake of completeness.
2.1 On averaging, Reynolds decomposition and Taylor's hypothesis
Most methods used to retrieve the dissipation rate require spatially resolved velocity statistics, although the velocity is recorded only at a single point and as a function of time in many experiments. Therefore, prior to estimating the energy dissipation rate, the one-dimensional velocity time record should first be mapped onto a spatially resolved velocity field. This is achieved by invoking Taylor's hypothesis (Taylor, 1938), which requires a Reynolds decomposition of the velocity time record by separating the velocity fluctuations from the mean velocity. To perform the Reynolds decomposition, we first have to clarify what is meant by the mean velocity.
Generally, we have to distinguish between the global mean velocity , the volume-averaged velocity 〈u(x,t)〉R over a sphere of radius R (for one-dimensional data 〈⋅〉R denotes spatial averages over a window of size R), the time-averaged velocity 〈u(x,t)〉τ over a time interval τ and the ensemble-averaged velocity 〈u(x,t)〉N over N realizations (Wyngaard, 2010; Pope, 2000, among others). In this work, 〈⋅〉 denotes the global mean, i.e., for infinitely large averaging windows in time or space. Thus, U is by definition independent of time and space, which in reality is valid only when u(x,t) is statistically stationary and homogeneous. Implicitly, and are, respectively, local volume and time averages, as both R and τ are typically finite. In the limit of R, τ→∞, 〈u(x,t)〉R and 〈u(x,t)〉τ tend toward U. For repeatable experiments where identical experimental conditions are guaranteed, 〈u(x,t)〉N tends toward U when N→∞.
The mean of a one-dimensional velocity time record in the longitudinal direction Uτ here is defined by
such that the global mean , where τ is the averaging window. It should be noted that the global mean of the transverse velocity will be equal to zero; i.e., when τ→∞, since here it is assumed that they are orthogonal to the mean flow direction. According to the Reynolds decomposition, the longitudinal velocity time record is composed of the mean velocity U and the random velocity fluctuation component so that the mean of the longitudinal velocity fluctuations .
In certain circumstances, it is possible to map from time to space coordinates by applying Taylor's (frozen-eddy) hypothesis (Taylor, 1938; Wyngaard, 2010), which relates temporal and spatial velocity statistics. Taylor (1938) argues that eddies can be regarded as frozen in time if they are passing the probing volume much faster than they evolve in time. This is the case if the turbulence intensity is much smaller than unity, i.e., I≪1, where is the root mean square (rms) velocity fluctuation. Then, the series of time lags relative to the start time t0 is mapped onto a distance vector with (Taylor, 1938), where x0 is the initial position at time t0. This approach is found to be reliable for I≲0.25 (Nobach and Tropea, 2012; Wilczek et al., 2014; Risius et al., 2015), while it has been shown to fail when I>0.5 (Willis and Deardorff, 1976). The application of Taylor's hypothesis is inaccurate in the case of large-scale variations in the velocity fluctuation field comparable with the mean velocity, which are known as “random-sweeping velocity” (Kraichnan, 1964; Tennekes, 1975). Complicating the estimation of the mean velocity, random-sweeping causes the mean energy dissipation rate to be consistently overestimated (Lumley, 1965; Wyngaard and Clifford, 1977).
One way to cope with non-stationary velocity time records is to evaluate the mean velocity for a subset of this signal. If the averaging time τ is finite, the time average Uτ may differ from the mean velocity U, causing a systematic bias in the subsequent data analysis. The estimation variance of the time average Uτ can be analytically expressed as (Wyngaard, 2010; Pope, 2000, among others)
where T is the integral timescale and the variance of the velocity time series. Notably, the size of the averaging window has to be large enough such that it fulfills to apply the Reynolds decomposition.
2.2 Estimating the energy dissipation rate
The energy dissipation rate can be derived from various statistical quantities. A non-exhaustive list of the most common methods applicable to single-point measurements is shown in Table 1. Details of selected methods considered in this study are presented in the following subsections. If not explicitly mentioned, the averages denoted with 〈⋅〉 are defined globally.
Table 1Various definitions of the energy dissipation rate from the dissipative and inertial sub-range to the energy injection range. Here, the definitions for various dissipation estimates are given in the space or wavenumber domain, where ν is the viscosity, sij is the velocity fluctuation strain-rate tensor, R is the radius of the averaging volume 𝒱(R) (window size for one-dimensional data), is the longitudinal velocity fluctuation field along e1, DL…L(r) is the nth-order longitudinal structure function (SF) for distance r, is the variance of , is the standard deviation of , NL is the number of zero crossings of a velocity fluctuation signal per unit length, C2≈2, E11(κ1) is the one-dimensional energy spectrum with wavenumber κ1, CK≈1.5, is the variance of a band-pass-filtered signal for wavenumbers , Cϵ is the dissipation constant, L11 is the longitudinal integral scale and ηK is the Kolmogorov length scale. Dissipation estimates indicated with * are not considered in detail in this work. The assumptions of stationarity (S), homogeneity (H), local isotropy (I) and Kolmogorov's second similarity hypothesis from 1941 (K41) are represented by their individual abbreviations. References are given in the corresponding sections in the main text.
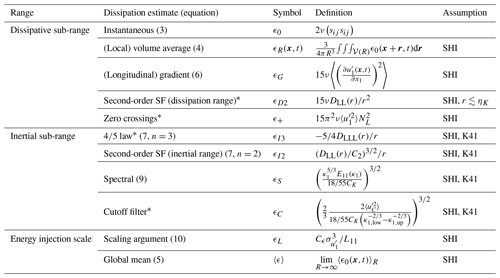
2.2.1 Dissipative sub-range
Proceeding from the Navier–Stokes equations for an incompressible Newtonian fluid, the instantaneous energy dissipation rate is given by 2ν(SijSij) (e.g., Pope, 2000; Davidson, 2015). As the velocity gradients are dominated by small-scale fluctuations, turbulent kinetic energy is dissipated into heat at small scales. Therefore, the contribution of large-scale variations in the velocity is small compared with the contribution of the small scales (Pope, 2000; Elsner and Elsner, 1996). Hence, the instantaneous energy dissipation rate can be defined in terms of the velocity fluctuations only, i.e., replacing Sij with the fluctuation strain-rate tensor (Pope, 2000):
Averaged over a sphere with radius R and volume 𝒱(R), the (local) volume average of the instantaneous energy dissipation rate is (Pope, 2000)
The local volume average ϵR(x,t) converges to the global mean energy dissipation rate if R tends toward infinity (Pope, 2000):
In experiments, it is often not possible to measure ϵ0(x,t). Under the assumption of statistically homogeneous and isotropic turbulence, the volume- and time-averaged energy dissipation rate are typically inferred from one-dimensional surrogates (Taylor, 1935; Elsner and Elsner, 1996; Siebert et al., 2006; Almalkie and de Bruyn Kops, 2012; Champagne, 1978; Donzis et al., 2008, among others), such as from the longitudinal velocity gradient (hence, the subscript G):
where the mapping between space and time domains is possible by applying Taylor's hypothesis if and R11 is the longitudinal component of the velocity covariance tensor defined in Eq. (A1) (Siebert et al., 2006; Muschinski et al., 2004). The relationship shown in Eq. (6) is often called the “direct” method in the literature (e.g., Muschinski et al., 2004; Siebert et al., 2006) and requires a spatial resolution higher than the Kolmogorov length scale ηK to be accurate within ∼10 % (see Fig. A8).
2.2.2 Inertial sub-range: structure functions
Kolmogorov's second similarity hypothesis from 1941 (Kolmogorov, 1941) provides another method for estimating the energy dissipation rate in the inertial range. Based on the inertial-range scaling of the nth-order longitudinal structure function, the mean energy dissipation rate can be calculated by (Pope, 2000)
where Cn is a constant, e.g., C2≈2 (Pope, 2000), and according to K41 by dimensional analysis. In practice, ϵI2 (Table 1) is retrieved by fitting either a constant to the compensated longitudinal second-order structure function DLL(r), n=2 in Eq. (7), or a power law () to the inertial range of DLL, defined in Eq. (A3), if the inertial range is pronounced over at least a decade. Accounting for intermittency, the scaling exponent of the nth-order structure function is modified to , where μ is the internal intermittency exponent (Kolmogorov, 1962; Obukhov, 1962; Pope, 2000). The inertial range is bounded by the energy injection scale L at large scales and by the dissipation range at small scales. That is why the fit range has to be chosen such that . If the inertial range is not sufficiently pronounced, the extended self-similarity may be used to extend the inertial range (Benzi et al., 1993b, a). Otherwise, ϵI2 can also be approximated by the maximum of Eq. (7) (for n=2) within the same range as before. This is possible because the maximum lies on the plateau in the case of a perfect K41 inertial-range scaling.
In the inertial range, the transverse second-order structure function DNN(r) is equal to in a coordinate system where r=re1 is parallel to the longitudinal flow direction (Pope, 2000), highlighting the importance of the measurement direction.
2.2.3 Inertial sub-range: spectral method
According to K41 (Kolmogorov, 1941), the inertial sub-range of the energy spectrum function scales as with the wavenumber κ by dimensional analysis. In isotropic turbulence, the energy spectrum function can be converted into a one-dimensional energy spectrum E11(κ1); see Eq. (A7). The wavenumber space is not directly accessible from one-dimensional velocity time records. Relying on Taylor's hypothesis, the one-dimensional energy spectrum E11(κ1) transforms into the frequency domain with , where (e.g., Wyngaard and Clifford, 1977; Oncley et al., 1996), yielding
which yields
with the Kolmogorov constant CK=1.5 (Sreenivasan, 1995; Pope, 2000). Applying Taylor's hypothesis to a flow with a randomly sweeping mean velocity causes the Kolmogorov constant to be systematically overestimated, whereas the scaling of power-law spectra remains unaffected (Wyngaard and Clifford, 1977; Wilczek and Narita, 2012; Wilczek et al., 2014). Hence, Eq. (9) is still valid for a randomly sweeping mean velocity, although ϵS is overestimated if CK is not corrected for random sweeping.
F11 has the units of a power spectral density of square meters per second (m2 s−1), and . Under the assumption of Kolmogorov scaling in the inertial sub-range, this identity can be adopted to estimate the mean energy dissipation rate from low- and moderate-resolution velocity measurements of a finite averaging window (Fairall et al., 1980; Siebert et al., 2006; O'Connor et al., 2010; Wacławczyk et al., 2017).
2.2.4 Energy injection scale
In equilibrium turbulence, the rate at which turbulent kinetic energy is transported across eddies of a given size is constant in the inertial range assuming high enough Reynolds numbers (e.g., Lumley, 1992). In a dimensional argument, this rate is proportional to , where u(l) is the characteristic velocity scale of eddies of length l. Considering the integral scale L11 and its characteristic velocity scale u(L11), namely the rms velocity fluctuation , the mean energy dissipation rate can be calculated by (Taylor, 1935)
where Cϵ is the dissipation constant, and for time- and space-varying turbulence, it depends on both initial and boundary conditions as well as the large-scale structure of the flow (Sreenivasan, 1998; Sreenivasan et al., 1995; Burattini et al., 2005; Vassilicos, 2015). Cϵ is found to be about 0.5 for shear turbulence (Sreenivasan, 1998; Pearson et al., 2002) and 1.0 (Sreenivasan, 1984; Sreenivasan et al., 1995) or 0.73 (Sreenivasan, 1998) for grid turbulence. In this work, Cϵ is assumed to be 0.5, which holds approximately in a variety of flows (Risius et al., 2015; Sreenivasan, 1995, and references therein).
Usually, the longitudinal integral length scale L11 is defined as (Pope, 2000)
where is the longitudinal autocorrelation function (see also Eq. A1 and Table A1). However, due to experimental limitations, r0 is often given by the first zero crossing of f(r) in both laboratory and in situ measurements (e.g., Risius et al., 2015) or, alternatively, by the position where e (Tritton, 1977; Bewley et al., 2012). Griffin et al. (2019) carried out an integration for r→∞ by performing an exponential fit in the vicinity of e. Notably, so that the estimation of L11 from the power spectrum is only recommended if (Pope, 2000) is accurately determined like in DNS. This approach requires not only a fully resolved velocity measurement but also a well-converged E11(κ1) as the conversion is highly sensitive to statistical scatter. Ultimately, the choice of L11 strongly affects ϵL. In this work, we integrate f(r) to the first zero crossing because it does not depend on assumptions on the decay of f(r) and the choice of the fit range.
2.3 Simulations of homogeneous isotropic turbulence
In this study, the direct numerical simulations of statistically homogeneous isotropic turbulent flow with are used as the basis for evaluating the different methods for determining the dissipation rate (see Table 2). Thereby, the performance of the different methods in estimating the energy dissipation rate is not affected by violating fundamental assumptions, e.g., isotropy or homogeneity. The simulations are carried out with the parallel solver TurTLE (Lalescu et al., 2022), which solves the Navier–Stokes equations on a periodic domain using a pseudo-spectral method with a third-order Runge–Kutta time stepping. Here, we use a forcing scheme with a fixed energy injection rate on large scales.
To mimic an ensemble of single-point measurements, we introduced 1000 virtual probes into the flow (one-way coupled, i.e., without back reaction on the flow), which move with a given constant speed in randomly directed straight paths to record the local flow velocity. Since the trajectories of the virtual probes are randomly oriented and the probability that they are exactly aligned with the simulation boundaries is low, the effect of periodic boundaries on the recorded velocity signal is expected to be small. We assume that the virtual probe records idealized velocity time series, neglecting the effect of transfer function associated with the anemometer (e.g., Horst and Oncley, 2006; Freire et al., 2019) or noise (Lenschow and Kristensen, 1985; Antonia, 2003; Lewis et al., 2021). While the root mean square velocity fluctuation is determined by the Navier–Stokes simulation, we can control the mean flow speed through the speed of the virtual probe. The range of constant speeds used corresponds to turbulence intensities of 1 %–50 %. Along the trajectories, we then sample the local three-dimensional velocity field (see Fig. 1) as well as the velocity gradient field, where we use spline interpolation to determine values in between grid points (Lalescu et al., 2010, 2022). By projecting the velocity vector onto the direction of the trajectory, e1, and the orthogonal directions, e2 and e3, we split the velocity field into longitudinal and transverse components, respectively. From the sampled velocity gradient tensor, we compute the local instantaneous dissipation ϵ0. The time step is limited either by the stability requirements of the flow solver or, for smaller turbulence intensities, by the sampling frequency required to capture the underlying flow. Here, we choose the time step such that the distance traveled by the probe within one step is around 110 of the grid spacing, UΔt≈0.1Δx. The grid spacing Δx is chosen such that the highest resolved wavenumber kmax satisfies .
Using Taylor's hypothesis, the longitudinal velocity time series correspond to at least 13L11 (for more details, see Table 2) so that second- and third-order moments of both longitudinal velocity fluctuations and increments are reasonably converged (see Fig. A3). To estimate ϵI3, ϵI2 and ϵS, the longitudinal structure functions are evaluated for scales or in the frequency domain for . The ground-truth reference for the mean energy dissipation rate per virtual probe is given by 〈ϵ0(x,t)〉VP, i.e., the average of the dissipation field along the trajectory of each virtual probe. The global mean energy dissipation rate can be approximated by the ensemble average of all 〈ϵ0(x,t)〉VP from all virtual probes, i.e., 〈〈ϵ0(x,t)〉VP〉N.
Table 2Parameter overview for each DNS. Rλ is the Taylor-scale Reynolds number, ηK is the Kolmogorov length scale, is the turbulence intensity, L11 is the longitudinal integral length scale derived from E(κ), ℒ is the average probe track distance and Np is the number of virtual probes. The turbulence intensity I is controlled by setting the probe mean velocity where is the root mean square longitudinal velocity fluctuation. For all cases , with kmax being the largest resolved wavenumber. For DNS 1.x and 2.x, the energy injection rate in code units is 0.4, while for DNS 3.x it is set to 0.5. The number of virtual probes Np for DNS 1.x is 10 000, whereas for DNS 2.x and DNS 3.x Np=1000.
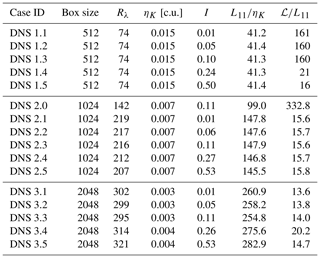
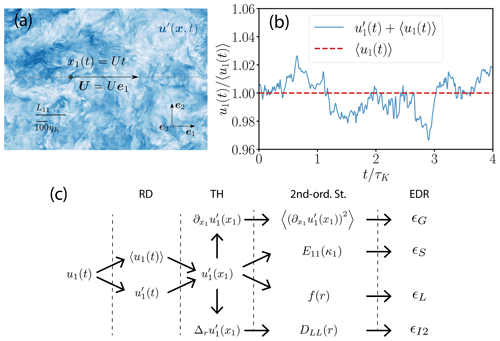
Figure 1Schematic representation of the procedure for calculating energy dissipation rates from single-point velocity time records. (a) Virtual probe sampling the three-dimensional velocity field of the DNS 3.1 (see Table 2) in time and space at a mean velocity U along its e1 direction corresponding to a turbulence intensity of 1 %. (b) One-dimensional velocity time series u1(t) (blue solid) with its corresponding time average U=〈u1(t)〉 (dashed red line, i.e., Eq. (1)) of the same DNS 3.1, where u1(t) is re-scaled by 〈u1(t)〉. (c) Visualization of the workflow from the one-dimensional velocity time record u1(t) to the energy dissipation rate via different methods. First, u1(t) is decomposed in its mean and fluctuating part according to Reynolds decomposition (RD). Then, the velocity time series is converted into a one-dimensional velocity field by invoking Taylor's hypothesis (TH). Subsequently, second-order statistics (2nd-ord. St.) of the longitudinal velocity fluctuations, their increments and their first spatial derivative are inferred, from which the energy dissipation rate is estimated with the help of different methods.
2.4 Variable Density Turbulence Tunnel (VDTT)
To evaluate the performance of different methods at Reynolds numbers applicable to atmospheric flows, we use the high-resolution hot-wire measurements of the longitudinal velocity components in the MPIDS VDTT (Bodenschatz et al., 2014). The VDTT datasets used here are associated with , which enables us to bridge the gap between DNS () and atmospheric Rλ∼𝒪(103) (Risius et al., 2015).
The VDTT is a recirculating wind tunnel where the working gas SF6 is pressurized up to 15 bar to increase its density, thereby enhancing the Taylor-scale Reynolds number. To achieve the same density in air, the pressure would have had to be about 100 bar, which would have required a much thicker tunnel wall and more expensive construction. The VDTT has a horizontal length of 11.68 m and an inner diameter of 1.52 m where the rotation frequency of the fan sets the mean flow velocity ranging from 0.5 to 5.5 m s−1 (Bodenschatz et al., 2014). Long-range correlations of the turbulent flow determine its anisotropy. These long-range correlations are shaped with the help of an active grid consisting of 111 independently rotating winglets (Küchler et al., 2019; Küchler, 2021).
Longitudinal velocity fluctuations are temporally recorded with 30 to 60 µm long nanoscale thermal anemometry probes (NSTAPs; Bailey et al., 2010; Vallikivi et al., 2011, among others) or a 450 µm long conventional hot wire from Dantec (Jørgensen, 2001) corresponding to a resolution of <3ηK and <5ηK, respectively (Küchler et al., 2019), at variable distances from the active grid ranging from ≈ 6–9 m. The velocity measurements have been extensively characterized in terms of the mean flow profiles (Küchler, 2021) as well as the decay of turbulent kinetic energy (Sinhuber et al., 2015; Sinhuber, 2015), exposing velocity probability distribution functions (PDFs) as being flatter than Gaussian (Küchler, 2021). The inertial-range scaling exponent ζ2 of the longitudinal second-order structure function is in agreement with Kolmogorov's revised phenomenology from 1962 ( for Rλ>2000) for a large variety of wake generation schemes (Küchler et al., 2020). In the case of hot-wire measurements in the VDTT, the ground-truth energy dissipation rate for a given averaging window R is given by the gradient method 〈ϵG〉R, which converges the fastest, as shown in Sect. 3.5.
2.5 Strategies for the evaluation of systematic and random errors
The virtual probes record one-dimensional time records of the DNS longitudinal velocity component, from which the mean energy dissipation rate can be estimated by various methods and compared with the energy dissipation rate obtained directly from the DNS dissipation field. Generally, there are two different errors when estimating the mean energy dissipation rate, namely the systematic errors and random errors. The latter is related to the estimation variance of the mean energy dissipation rate, i.e., the statistical scatter of the 〈ϵ〉R estimates around the ground truth of the local mean energy dissipation rate defined in Eq. (4). The systematic error in the mean energy dissipation rate estimates expresses itself in a non-vanishing ensemble average of the deviations from the ground truth, i.e., the global volume average defined in Eq. (5).
Systematic errors are an inherent feature of the methods used for estimating the dissipation rate but are also affected by experimental limitations and imperfections such as averaging windows and finite turbulence intensity parameterized by R and I, respectively. One way to estimate these errors is to compare the estimated mean energy dissipation rate for a given averaging window R with the ground truth of the DNS defined by the mean energy dissipation rate per virtual probe track, i.e., . Another possibility is to compare the estimates with the ensemble average of the mean energy dissipation rate from all virtual probes, i.e., , where N=1000 is the total number of virtual probes. Either of these possibilities is valid and would be interesting to understand. However, our analysis (not presented here) shows that the second approach is associated with a slightly higher value for the systematic error and a slightly higher standard deviation. For that reason, we have chosen the second approach to make a conservative assessment of the systematic errors; i.e., we compare the estimates of each method against by
where and 〈ϵi〉R are the estimates of the energy dissipation rate via method i under the experimental limitation and imperfection such as the size of the averaging window or finite turbulence intensity. To distinguish between the different error terms in this paper, we refer to β as a “reference-compared” systematic error.
In addition, the systematic error can be evaluated by comparing the estimates of the energy dissipation rate obtained by a method with imperfect data against the estimates obtained by the same method with optimal data. We denote these types of errors with δ and refer to them as “self-compared” errors. An experimental imperfection we considered here is the sensor misalignment: this is a non-zero angle of incidence θ between the true longitudinal flow direction along U and the one expected based on the sensor orientation. To investigate the isolated effect of sensor misalignment, we consider a specific set of DNSs with constant turbulence intensity (I=1 %) and the entire track length for each virtual probe. The self-compared systematic error in each method due to misalignment is defined as
where ϵi(θ) is the estimate of the energy dissipation rate via method from data with misalignment θ and ϵi(0) is the estimated dissipation rate from the same method and flow conditions but with an aligned sensor; i.e., θ=0.
Estimates of the mean energy dissipation rate are susceptible not only to systematic errors, but also to random errors due to statistical uncertainty. For the averaging window, errors given by Eq. (12) would be the best indicator of systematic errors. However, random errors due to the size of the averaging window can also be significant. When the spatial averaging window R (or temporal averaging window τ) is finite, we capture the self-compared random error for each individual method by
where 〈ϵi〉R is the local mean energy dissipation rate based on the averaging window R normalized by its ensemble average, i.e., 〈〈ϵi〉R〉N. Equation (14) indeed calculates the standard deviation of the normalized 〈ϵi〉R, which is used here as a proxy for the random error. Table 3 provides an overview of the different error types and terminologies used here.
Table 3Different types of errors investigated in this study and their definitions. Subscript , where G stands for the gradient method, I3 for the 4/5 law, I2 for the second-order structure function in the inertial range, S for the spectral method and L for the scaling argument. The averaging window is denoted spatially by R and temporally by τ. The misalignment angle is represented by θ.
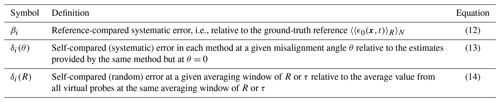
In the following, we first focus on the DNS data to calculate ϵG, ϵI3, ϵI2, ϵS and ϵL from the entire longitudinal velocity time records of all virtual probes and compare these estimates against the ground-truth reference. Then, we systematically investigate the impact of the turbulence intensity, (virtual) probe orientation and averaging window size for all methods of interest. The influence of the flow Reynolds number on the presented results is then discussed by taking into account the VDTT data together with the DNS data. Finally, we provide a proof of concept for a time-dependent dissipation rate calculation by comparing the dissipation time series measured by ϵG, ϵI2 and ϵL and its coarse-grained surrogate. In the following, we use the definitions of systematic and random errors as mentioned in Sect. 2.5 and Table 3.
3.1 Verification of the analytical methods and a first insight into their performance under ideal conditions
To verify the implementation of our methods, only data from cases with a low turbulence intensity of 0.01 and an averaging window covering the entire size of the probe track are used in this section. Furthermore, ϵI2 and ϵI3 are obtained by a fit according to Eq. (7) with n=2 and n=3, respectively, in the inertial range with for DNS 2.1 and 3.1. Analogously, ϵS is inferred from the inertial-range fit in Eq. (9) in the range . For DNS 1.1 with Rλ=74, due to the absence of an inertial range for low Taylor-scale Reynolds numbers (see Fig. A7), the maximum of Eq. (7) is used to infer ϵI2 and ϵI3 instead of fitting the inertial range.
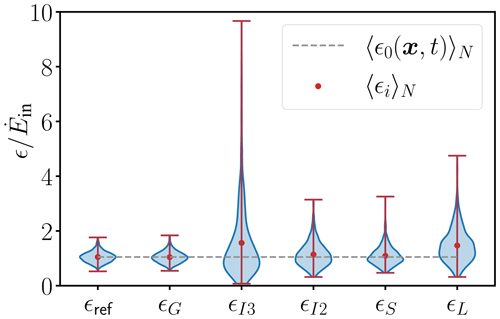
Figure 2Validation of estimating the energy dissipation rate from and ϵL re-scaled by the energy injection rate . The data are taken from DNS 3.1 with 1000 probes, Rλ=302, I=1 %, θ=0∘ and the maximal available averaging window (R≈3550ηK). The ensemble mean of each method 〈ϵi〉N is denoted by red dots where the whiskers extend from the minimal to maximal estimate of ϵi where . The reference mean energy dissipation rate for each probe is given by ϵref. The dashed line represents the re-scaled global mean energy dissipation rate of DNS 3.1, which is approximated by the ensemble average of the true mean energy dissipation rate along the trajectory of each virtual probe.
Table 4Statistics (mean, median, standard deviation (SD) and range) of the reference-compared systematic errors for different methods and case DNS 3.1 with Rλ=302. Here βref is defined as , which shows the actual variability in the dissipation rate field. The last column shows the range of the minimum to maximum percentage deviation from the mean.
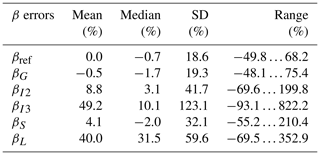
The distribution of the mean energy dissipation rate estimated by and ϵL for each probe at Rλ=302 is shown in Fig. 2 and Table 4. Estimations for other Rλ values are shown in Fig. A1. The best-performing method is the gradient method ϵG, with the range also being very close to the range of βref, whereas ϵI3 is associated with the mean highest deviation. The superior performance of ϵG compared with the others is mainly due to the fact that it relies on (dissipation-range) second-order statistics that can be captured with fast statistical convergence within a short sampling interval. Hence, the distributions of ϵG and ϵref are similar. ϵI3, on the other hand, relies on third-order moments of the velocity increments of inertial scales associated with slower statistical convergence compared to ϵG. Therefore, ϵI3 requires longer velocity records than ϵG to converge under stationary conditions. For this reason, the third-order structure function is not considered further in this study, as one of the main objectives of this study is to evaluate different methods suitable for extracting the time-dependent energy dissipation rate.
Figure 2 and Table 4 also show that the estimates of the energy dissipation rate provided by DLL(r) and E11(κ1) are close to each other, which can be explained by the fact that they are both second-order quantities (in real and Fourier space, respectively) connected by f(r). Unlike ϵG, both ϵI2 and, to a lesser extent, ϵS tend to overestimate the energy dissipation rate. However, ϵS more strongly depends on properly setting the fit range than ϵI2 (Fig. A2). The spectral method ϵS can differ by a factor of 2 from ϵI2 depending on the high-frequency limit. This factor of 2 is in accordance with a comparison of ϵI2 and ϵS by a linear fit, resulting in a slope close to 0.5 (Akinlabi et al., 2019). In the DNS, the power spectrum is subject to strong statistical uncertainty at high frequencies without ensemble averaging the spectra of each virtual probe or longer DNS runtimes. As the high-frequency limit of the inertial range of the spectrum is hardly distinguishable from its dissipation range, the choice of the fit range for ϵS is related to the fit range of the longitudinal second-order structure function by as mentioned above. Wacławczyk et al. (2020) found that the estimation of the energy dissipation rate from the power spectral density is generally robust at small wavenumbers (i.e., larger length scales), whereas the second-order structure function performs better at small length scales (i.e., larger wavenumbers). With our choice of the fit range for the DNS 3.1 dataset shown in Fig. 2, we confirm that ϵI2 is already reliable at the lower end of the inertial range where dissipative effects are negligible.
Lastly, ϵL overestimates by 40 % on average. This systematic overestimation might be due to the difficulty in determining L11, as different methods for estimating the integral length L11 can contribute to the systematic bias in ϵL. As mentioned above, we infer the longitudinal integral length from fitting f(r) to the first zero crossing which yields, at least in the DNS of this work, a systematic underestimation due to the scatter in both and L11, as illustrated in Figs. A3 and A4. However, the accuracy of the dissipation constant Cϵ, which is a function of large-scale forcing and initial conditions (Vassilicos, 2015; Sreenivasan, 1998; Sreenivasan et al., 1995; Burattini et al., 2005), can potentially cause larger mean deviations. Advantageously, the large-scale estimate ϵL is applicable to low-resolution measurement. Figure A1 and Table A3 give an overview of the systematic errors in the different methods at different Reynolds numbers, showing that the above conclusions are also valid for lower Rλ.
3.2 The validity of Taylor's hypothesis and the impact of random-sweeping effects
For a large turbulence intensity the local speed and direction of the flow vary significantly in time and space, which hinders the applicability of Taylor's hypothesis. Here, we quantify the impact of random sweeping on the accuracy of determining the mean energy dissipation rate. Therefore, we set the mean speed of the virtual probes in each DNS so that the turbulence intensity, and consequently the random sweeping, is a control parameter.
Figure 3 shows the systematic error βi for ϵG, ϵI2, ϵS and ϵL at different turbulence intensities for DNS 3.1–3.5. For each virtual probe taken into account in Fig. 3, we used the entire time series so that the size of the averaging window is maximal. While each method has a different systematic error and scatter, Fig. 3 indicates that the mean relative deviation of each estimate from the global mean 〈〈ϵ0(x,t)〉VP〉N increases with turbulence intensity. This is particularly strong for the gradient method. For I=1 % and I=10 %, the gradient method has the lowest scatter in terms of the standard deviation (19.3 % and 27.3 %) and the lowest systematic error in terms of 〈βG〉N (−0.5 % and 6.1 %), respectively. At higher turbulence intensities, ϵI2 is the least affected method, with 〈βI2〉N=6.5 % and % for I=25 % as well as 〈βI2〉N=24.5 % and % for I=50 %. At the highest turbulence intensities, both ϵL and ϵS are associated with lower mean β than that of ϵG.
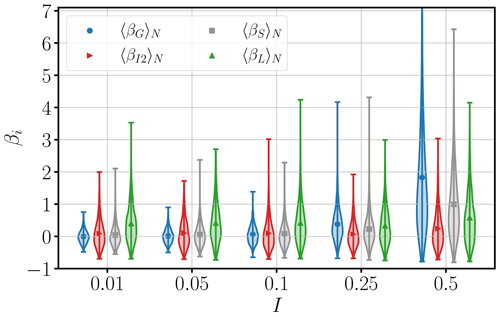
Figure 3Systematic error βi in Eq. (12) as a function of turbulence intensity for ϵG (•), ϵI2 (▸), ϵS (▪) and ϵL (▴). The energy dissipation rates are estimated from each longitudinal velocity time series of DNS 3.1–3.5 with ideal alignment (θ=0∘) where the maximal available window size was used. The fit range for the inertial range of the power spectral density is chosen to be within , where ηK is the Kolmogorov length scale, and, equivalently in the space domain, for the longitudinal second-order structure function. The upper limit of the y axis is chosen to be 7.1 in order to improve the plot visibility (upper whiskers of ϵG for I=50 % are not shown for better visibility of other cases).
The fraction of track samples that can lead to a deviation of larger than 100 % increases from 0 % to ∼60 % for ϵG as the turbulence intensity increases from 1 % to 50 %. We hypothesize that these deviations of the mean are the result of random-sweeping effects, which limit the applicability of Taylor's hypothesis. In frequency space, Taylor's hypothesis (Taylor, 1938) establishes a one-to-one mapping between the frequency and the streamwise wavenumber; i.e., ω=κ1U. As the turbulence intensity grows, a randomly sweeping mean velocity smears out this correspondence between frequencies and wavenumbers. For the spectrum, this smearing out effectively moves energy from larger scales to smaller and less energetic ones (Lumley, 1965; Tennekes, 1975). Therefore, it leads to an overestimation in the inertial and dissipation range of the spectrum, thus affecting the inertial range and gradient method. To visualize this overestimation, we evaluate the effect of random sweeping on the spectrum (Eq. B2) numerically for different turbulence intensities at the example of a model spectrum (Eq. B4). The result is shown in Fig. 4, where the spectrum is premultiplied by to later highlight the effect on the gradient method. Here, the overestimation is most pronounced in the dissipative range.
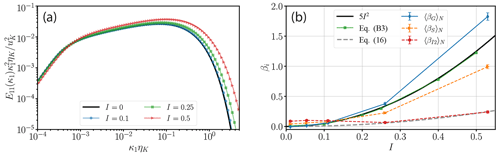
Figure 4The effects of random sweeping on the energy dissipation estimates. (a) Premultiplied model energy spectrum with random-sweeping effects in Eq. (B2) for turbulence intensities , where the original energy spectrum corresponds to I=0. is the Kolmogorov velocity scale. (b) Systematic overprediction illustrated by the relative error βi in Eq. (12) at different turbulence intensities. The systematic overprediction by Lumley (1965) (solid black) matches the numerically obtained systematic error βG for the gradient method relative to the ground-truth reference 〈ϵ〉 by using the model spectrum and numerically evaluating the integral in Eq. (B3) (green squares). Both estimate the data obtained from DNS 3.1–3.5 (blue diamonds) up to a turbulence intensity of I=25 % reasonably well. Moreover, we show the systematic overprediction of inertial-sub-range methods (βS: orange triangles and βI2: red circles, both from Eq. 12) compared with the analytically derived error obtained by the random-sweeping model (βI2,S, Eq. 16, grey dashed).
To quantify the impact of random sweeping on estimates of 〈ϵ〉, we first consider the influence of random sweeping on the gradient method. For the gradient method, Lumley (1965) and Wyngaard and Clifford (1977) have shown that in isotropic turbulence, random sweeping leads to an relative deviation of the volume-averaged mean energy dissipation rate by
We illustrate this result in Appendix B, where we consider a model wave-number–frequency spectrum (Wilczek and Narita, 2012; Wilczek et al., 2014), which is based on the same modeling assumptions used in Wyngaard and Clifford (1977). Due to the weighting of the gradient method, the mean dissipation rate estimate is highly sensitive to the viscous cutoff of the energy spectrum, which is overestimated by random-sweeping effects (see Fig. 4). As a consequence, deviations in the estimated dissipation rate grow rapidly with turbulence intensity. In the right panel of Fig. 4, we compare the effect of random sweeping on the gradient method obtained with a model spectrum, the one computed by Lumley (1965) and the observed deviations by measurements of the virtual probes in a DNS flow; shown here are the DNS 3.1–3.5 cases. In fact, the estimate from Lumley (1965) can explain the magnitude of deviations observed by the virtual probes in the case of ϵG up to I=25 %. The strong deviation in βG at I=50 % is likely due to the sensitivity of the gradients to the space-to-time conversion via Taylor's hypothesis: at high turbulence intensities, the mean velocity becomes smaller compared with the fluctuations. Therefore, the error in estimating the mean velocity due to the finite averaging window (Eq. 2) increases relative to the mean velocity. Larger relative errors in the estimated mean velocity lead – applying Taylor's hypothesis – to both under- and overestimated spatial gradients for the individual averaging windows, in addition to the effect of random sweeping. Similarly this results in an additional overestimation of the dissipation rate. These deviations do not appear in evaluating random-sweeping effects based on a model spectrum, as there the mean velocity is a parameter we choose.
Now let us consider the two inertial-sub-range methods. Here, as one can see in Figs. 3 and 4, the increase in the mean relative deviation, βi, is less pronounced. In the inertial sub-range, random sweeping causes an overestimation of the spectrum of merely several percent, while the inertial-range scaling is preserved as shown in Wyngaard and Clifford (1977) and Wilczek et al. (2014). As both the second-order structure function and the spectral method are based on the inertial sub-range of the energy spectrum, the effect of a randomly sweeping mean velocity on ϵI2 and ϵS is expected to be small. Here, the overestimation of the spectrum can be used to express the relative systematic deviation in both ϵI2 and ϵS for different turbulence intensities analytically:
where CT(I) quantifies the spectral overestimation as a function of mean wind and fluctuations defined as in Wilczek et al. (2014). In Fig. 4b, we compare the observed deviations from the DNS to Eq. (16). This shows that Eq. (16) underestimates βI2 for (i.e., DNS 3.1, 3.2 and 3.3). The underestimation is most likely due to additional random errors associated with finite averaging window lengths. It is obvious from Table 2 that DNS 3.3 statistically has the shortest probe tracks of ∼3440ηK (DNS 3.1: ∼3550ηK, DNS 3.2: ∼3560ηK). Nonetheless, βI2 matches the prediction of Eq. (16) for where the corresponding probe tracks statistically amount to ∼5570ηK and ∼4260ηK, respectively. The effect of the averaging window size on ϵI2 is explored in Sect. 3.4. We conclude that Eq. (16) can be used to estimate the error introduced by random sweeping of ϵI2.
For the spectral method, Eq. (16) underestimates the relative error βS for all turbulence intensities. This may be due to the strong dependence of ϵS on the U-based fitting range (see Fig. A2), i.e., , which can differ significantly between virtual probes at high turbulence intensities. Further work is needed to assess the dependence of the spectral method on the choice of the fit range for finite turbulence intensities.
Overall, random-sweeping effects explain why the gradient method is more sensitive to turbulence intensity than inertial-range methods. Here, random sweeping accurately captures the deviations of the second-order structure function method as a function of turbulence intensity, whereas it can only partially account for the observed deviations for the spectral method.
3.3 Probe misalignment
In this section, we assess the influence of probe misalignment with respect to the mean flow direction on estimating the energy dissipation rate at the energy injection scale, the inertial range and the dissipation range. Here, we assume the angle θ between the (virtual) anemometer and the global mean wind direction to be constant throughout the sampling trajectory. As can be seen from Eq. (C6), ϵL depends on θ. Then, the analytically derived error for ϵL due to misalignment of the sensor and the longitudinal wind direction is given by
where ϵL(θ) represents the energy dissipation that is derived given an angle of incidence θ, and ϵL(0) is the reference value for perfect alignment of the mean flow direction and the probe, i.e., when θ=0.
Analogously, the second-order structure function tensor is affected by misalignment (cf. Appendix C). Thus, it can be shown that the analytically derived error δI2(θ) as a function of θ reads
where ϵI2(θ) represents the energy dissipation that is derived given an angle of incidence θ, and ϵI2(0) is the reference value for perfect alignment of the mean flow direction and the probe. As outlined in Appendix C and with Eq. (5), the analytically derived error in ϵG as a function of θ can be calculated to
where ϵG(θ) represents the energy dissipation that is derived given an angle of incidence θ, and ϵG(0) is the reference value for perfect alignment of the mean flow direction and the probe.
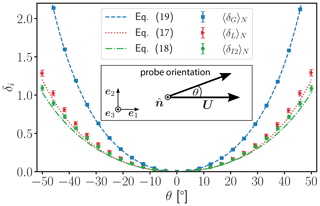
Figure 5Influence of misalignment between probe orientation and the mean flow direction U in terms of the average error in the energy dissipation rate δi(θ) as a function of the angle of attack θ. The energy dissipation rates are derived from DNS 3.1 with a turbulence intensity of 1 %, an Rλ=293 and the maximally available window size. The error bars are given by the standard error of the mean. The analytically derived errors δL(θ), δI2(θ) and δG(θ) are given by Eqs. (17), (18) and (19), respectively. The ordinate is limited from 0 to 2.2 to guarantee better visibility for δL(θ) and δI2(θ). The inset visualizes the misalignment angle θ between the probe orientation and the mean flow direction U. The rotation axis is denoted by . As mentioned above, the mean flow direction U is considered to be the longitudinal direction of the flow.
To compare the analytical expressions with DNS results, the sensing orientation of the virtual probes is rotated around the e3 axis in the coordinate system of each virtual probe by an angle θ relative to their direction of motion, i.e., the e1 axis. Then, ϵL(θ), ϵI2(θ) and ϵG(θ) are inferred from the new longitudinal velocity component. The ensemble-averaged relative errors in the estimated energy dissipation rates δ(θ) due to misalignment are shown as a function of θ in Fig. 5 in the range of ±50∘ both for DNS and for the analytically derived Eqs. (17)–(19). In general, the ensemble-averaged systematic errors follow the analytically derived errors reliably in terms of the limits of accuracy for all Rλ values at turbulence intensity I=1 %. The longitudinal second-order structure function is the best-performing method, with a systematic error 〈δI2〉N of lower than 20 % for , which increases to 100 % at ∘. 〈δL〉N is similarly affected by misalignment but is slightly larger than 〈δI2〉N. Despite its rapid statistical convergence, ϵG is the method most vulnerable to misalignment compared with the other two methods.
In experiments where the sensor can be aligned to the mean wind direction within over the entire record time, δi(θ) is expected to be small. Further work is needed to evaluate the impact of a time-dependent misalignment angle θ(t). We suppose that keeping the angle of attack θ fixed over the entire averaging window, here the entire time record of each probe, potentially leads to overestimation of δi(θ), with θ being a function of time in practice.
3.4 Systematic errors due to the finite averaging window of size R
Here, our goal is to investigate how the accuracy of estimating the global mean energy dissipation rate depends on the averaging window size by investigating the associated systematic and random errors individually. To do this, we select an averaging window of size R from the beginning of each track of virtual probes for the DNS 3.1 case. In this way, we obtain one subrecord for each virtual probe, which amounts to a total of 1000 subrecords for each averaging window R. From each of these subrecords, mean values of ϵ0 (i.e., ), 〈ϵG〉R, 〈ϵL〉R and 〈ϵI2〉R are then evaluated. The smallest R considered for these analyses is 501ηK, which is limited by the upper bound of the fitting range for estimating ϵI2. The largest window size considered in this section is 3000ηK, which is limited by the total length of the virtual probe track (Table 2).
Before comparing estimates of the energy dissipation rate using different methods, let us first compare the locally averaged energy dissipation rate with the instantaneous energy dissipation rate, which is shown in Fig. 6a. All averaging window sizes create PDFs with similar shapes but significantly different from the shape of the instantaneous field. The larger the volume over which the dissipation field is averaged, the more the PDF converges to a peak at the global mean energy dissipation rate normalized by , i.e., .
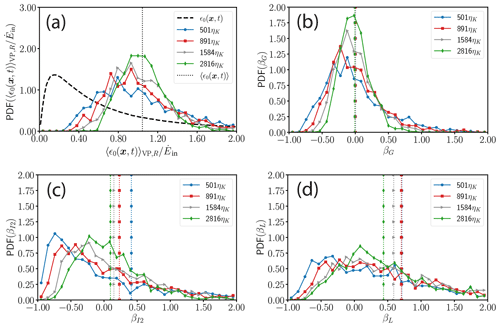
Figure 6The effect of the averaging window size R (a) on the distribution of and on the accuracy of estimates obtained via (b) 〈ϵG〉R, (c) 〈ϵI2〉R and (d) 〈ϵL〉R in terms of the systematic errors βG, βI2 and βL, respectively, from the ground-truth reference as given by Eq. (12). The velocity time records of the longitudinal component are taken from DNS 3.1 (Rλ=302, I=1 %, θ=0∘). In panel (a), the distribution of the instantaneous dissipation rate sampled by all virtual probes is shown by the dashed line, and the global average energy dissipation rate normalized by is shown by the vertical dotted line. The other PDFs in (a) are from the local average of the energy dissipation rate obtained from a window of size R at the beginning of each virtual probe, i.e., 1000 averaged values for a given R. In panels (b), (c) and (d) the vertical dotted lines correspond to the ensemble averages of the systematic errors βi. The ensemble average of βG decreases slightly from 0.4 % for R=501ηK to −0.7 % for R=2815ηK where the standard deviation of βG decreases from 50 % to 22 %. The ensemble average of βI2 decreases from 41 % to 10 % and the standard deviation from 185 % to 5 %. βL exhibits stronger deviations (mean βL of ∼44 % and standard deviation of ∼67 % for R=2816ηK).
We can further explore the influence of the averaging window R for each method by examining the distribution of systematic errors, i.e., βi, as shown in Fig. 6b–d. The first main point to note is the fact that at small R all methods tend to peak at a dissipation rate lower than the global. Hence, the mean energy dissipation rate is most likely underestimated. All PDF(βi(R)) values become narrower, and the mean relative error βi(R) converges to zero as R increases. The second main point to consider is the statistical uncertainty, causing a random error in estimating the local mean energy dissipation rate . As can be seen in Fig. 6b–d, the width of the distribution is wide with asymmetric long tails, especially for βI2 and βL. This is an indication that high random errors are to be expected in the estimation of the mean energy distribution rate.
3.5 Random errors due to the finite averaging window of size R
We now analytically focus on random errors associated with ϵG, ϵL and ϵI2. We denote 〈ϵG〉R, 〈ϵL〉R and 〈ϵI2〉R as the energy dissipation rates that are estimated for a longitudinal velocity time record for a window of size R. For the calculation of random errors caused by the choice of the size of the averaging window, we consider DNS 1.3, 2.3 and 3.3, as well as wind tunnel experiments that all have a comparable turbulence intensity of I≈10 %.
Both the second-order structure function in Eq. (A3) and the scaling argument in Eq. (10) depend on the variance of the longitudinal velocity time record. ϵG is also related to through Eqs. (6) and (A1). The variance itself is subject to both systematic and random errors in the case of a finite averaging window R<∞. Assuming an ergodic and hence stationary velocity fluctuation time record with a vanishing mean, the systematic error in estimating the variance over an averaging window of size R is given by (following Lenschow et al., 1994, while applying Taylor's hypothesis)
where is the estimated variance based on the (finite) averaging window R, is the true variance and it is assumed R≫L11. The always negative error predicted by Eq. (20) indicates that, for finite averaging window sizes, the variance is always statistically underestimated, which agrees with Fig. A3a. Equation (20) furthermore indicates that the systematic error in the variance estimates can be neglected for sufficiently long averaging windows R≫L11.
The variance estimates are also subject to statistical uncertainty, which is also known as the random error in variance estimation (Lenschow et al., 1994). Assuming that , which has a zero mean, can be modeled by a stationary Gaussian process and that its autocorrelation function is sufficiently well represented by an exponential, the random error in estimating the variance can be expressed as (following Lenschow et al., 1994, while applying Taylor's hypothesis)
where it is assumed R≫L11 such that the systematic error can be neglected and, hence, . Here, is the ensemble average of the variance estimates for an averaging window R. It can be seen that erand is larger than the systematic error in Eq. (20) when R>L11.
Consequently, the estimation of the mean energy dissipation rate by the scaling argument in Eq. (10) is affected by the (absolute) random error in the variance estimation given by the product of erand and . Invoking the Gaussian error propagation, the analytically estimated error reads
where erand is the relative random error in the variance estimate of the velocity fluctuations defined in Eq. (21), and is the variance estimate of based on the averaging window R. Then, the absolute random error in the variance estimate of the velocity fluctuations is given by . δL(R) is a relative error itself, hence the prefactor . Notably, δL(R) scales as .
Similarly, the longitudinal second-order structure function is also affected by the estimation variance ,
where DLL(r;R) is the longitudinal second-order structure function evaluated over an averaging window of size R and under the assumption that the longitudinal autocorrelation function f(r) is sufficiently converged over the range of the averaging window. This is a simplistic assumption that may be questionable in some cases, but a more robust evaluation of the validity of the assumption is complex and beyond the scope of this study.
Thus, the uncertainty in estimating the variance propagates to 〈ϵI2〉R relying on DLL(r;R) (Eq. 7 for n=2). The random error δI2(R) can be analytically inferred from the random error in the second-order structure function by Gaussian error propagation, yielding
which shows that δI2(R) scales as similar to δL(R). Considering Eqs. (6) and (A1), the gradient method can also be expressed as a function of the variance . Hence, Gaussian error propagation yields
assuming R≫L11 such that the systematic error is negligible so that .
Equations (22), (24) and (25) are expressed as a function of R and L11, which do not reveal the dependency of random errors on the Reynolds number. In addition, this expression relies on large scales that depend on the scale of the energy injection, which makes it difficult to fairly compare the errors between different flows as it is not a universal feature. Therefore, we want to link the averaging window to the Kolmogorov length scale ηK, which only depends on the viscosity and the mean energy dissipation rate. We can rewrite these equations in terms of ηK, R and Rλ as follows:
where we have invoked , which is valid at sufficiently high Rλ, and have used the relationship (Pope, 2000). Following the intuition, the longer the averaging window, the smaller the random error in each method.
Furthermore, Eqs. (26) and (27) provide means to choose a suitable averaging window size to achieve a given random-error threshold a. Let Ra be the averaging window of size R such that δi(R)<a. Then, the required averaging window Ra for ϵI2 and ϵL is
where the required averaging window size Ra scales with . Similarly, the required averaging window for ϵG is
For example, for the random errors of ϵI2 and ϵL to be less than 10 % at Rλ=1000, the averaging window should be , while for ϵG the required averaging window is .
Figure 7 shows the empirical random errors δG(R) (Fig. 7a) and δI2(R) (Fig. 7b) as a function of the averaging window size for various Rλ values based on VDTT data (for ϵL, see Fig. A5). To do this, we select an averaging window of size R, where , from the beginning of each 30 s time segment of the VDTT longitudinal velocity records (a total of 47 to 597 time segments depending on Rλ).
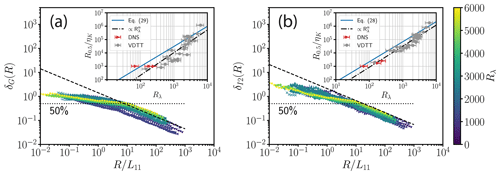
Figure 7Random errors δG(R) (a) and δI2(R) (b) as a function of the re-scaled averaging window size obtained from VDTT data at various Rλ values shown by the color bar. The analytical results for δG(R) (a, Eq. 25) and δI2(R) (b, Eq. 24) are shown by the dashed black lines. The dotted black line annotated with 50 % in each subplot corresponds to a 50 % error threshold. The insets show the sizes of the averaging windows in terms of ηK when as a function of the Taylor-microscale Reynolds number Rλ. The inset plots include data from both the DNS (red triangles) and the VDTT (grey circles). DNS data used for the inset plots are from cases 1.3, 2.3 and 3.3 with I=10 % and θ=0∘. The solid blue lines show the prediction of the required averaging window according to Eq. (29) (a – inset) and Eq. (28) (b – inset). The black dash-dotted line in the inset plots is a fit to the data: , yielding and (a – inset); , yielding and (b – inset).
The scaling of δG(R) and δI2(R) is predicted well for R≳10L11 as expected from Eqs. (25) and (24) and the assumptions we made to derive them. However, for smaller R a statistical convergence of ϵG, ϵI2 or ϵL against the mean energy dissipation rate cannot be expected, in particular if .
Furthermore, it is evident from Fig. 7 that the random errors do not fully collapse on each other for different Reynolds numbers and at a given . Moving horizontally on a line of constant random error, e.g., the dashed line of 50 % error, the required window size increases with Rλ, as shown in the insets of Fig. 7a and b. Predictions of Eqs. (28) and (29) are also shown in these plots via solid blue lines.
For both ϵG and ϵI2, the theoretical expectation for Ra tends to overestimate the actual averaging window size at which a random error of 50 % is achieved. This overestimation is expected as the theoretical expectation for Ra in Eqs. (28) and (29) is derived assuming that large-scale quantities such as f(r) and L11 are fully converged. However, ϵG is technically relying on small scales. ϵG depends on velocity fluctuation gradients, which are numerically obtained by central differences. Hence, each increment in the velocity record contributes to the average in the gradient method (Eq. 6). In the case of ϵI2, the number of possible increments reduces for larger separations for a finite averaging window. By definition, the exact computation of L11 even requires a fully converged f(r) for all r values.
However, VDTT experiments with Rλ>3000 underestimate the prediction of Eq. (20) by about a factor of 2. This is particularly clear for ϵL shown in Fig. A5. This deviation at high Rλ can be explained, at least in part, by the strong assumptions made for the derivation of the random errors, i.e., Eqs. (24), (22) and (25). In particular, for experiments with high Re in VDTT, the assumption of Gaussian velocity fluctuations with zero skewness is questionable, as shown in Fig. A6. Lenschow et al. (1994) have already established that the size of the averaging window for a skewed Gaussian process (see Eq. 19 in Lenschow et al., 1994) must be twice as large as for a Gaussian process with vanishing skewness. However, further work is needed to investigate these deviations and improve the theoretical prediction.
3.6 Estimating the transient energy dissipation rate
As has been shown previously in Figs. 6 and 7, both systematic and random errors decrease with the size of the averaging window. For a correct estimate of the magnitude, it is therefore advantageous to choose the averaging window as large as possible, but the price of this is that the transient trend smaller than the selected window size cannot be reproduced. In addition, it is also important to know to what extent statistical uncertainties originating from the estimation methods themselves disguise the true trends of the underlying turbulent flow. Given a certain averaging window size R, here, we empirically evaluate if trends in the coarse-grained time series are physical or rather statistical. In other words, we ask the question whether local estimates of the mean energy dissipation rate follow the ground-truth reference or not. Respecting the intermittent nature of turbulence and energy dissipation, the standard deviation of is a first proxy for the variability of the trend in . Hence, detecting the true trend requires that βi and δi(R) are smaller than the standard deviation of .
It can already be concluded from Figs. 2, 7, A1 and A5 that ϵG is the most promising candidate to capture the true trend. However, to fully answer the above questions, we need to conduct a more in-depth analysis. The upper plot in Fig. 8 shows the re-scaled and coarse-grained dissipation field for a sliding window of size R≈5500ηK and a turbulence intensity I=10 % obtained from the time series of one virtual probe for case DNS 2.0 (“probe 0”). Consistent with results shown earlier, 〈ϵG〉R follows best in comparison with 〈ϵI2〉R and 〈ϵL〉R. Both 〈ϵI2〉R and 〈ϵL〉R are associated with substantial scatter, although 〈ϵI2〉R has smaller deviations from the ground truth overall. Other probe tracks sample different portions of the flow, which is why a quantitative conclusion is not possible from one single probe. A more comprehensive evaluation of which method is able to capture the true trend is conducted below.
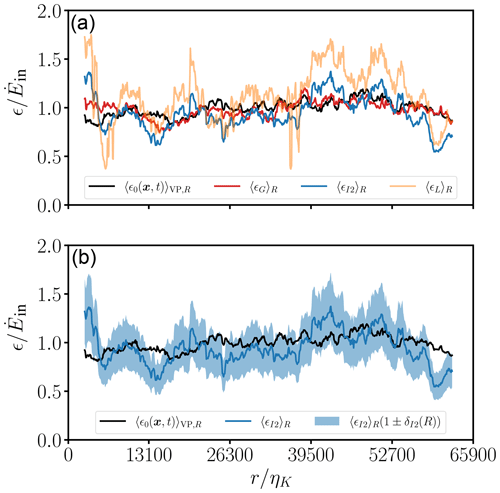
Figure 8(a) Proof of concept for estimating the coarse-grained energy dissipation rate re-scaled by the energy injection rate via the one-dimensional surrogates 〈ϵG〉R, 〈ϵI2〉R and 〈ϵL〉R for Rλ=142, , θ=0∘ and a turbulence intensity I=10 % (DNS 2.0). All estimates are re-scaled by the energy injection rate , too. We narrowed the fit range to , ensuring optimal fit results. (b) Comparison between with the estimated random error according to Eq. (24) for the averaging window R and .
The lower plot in Fig. 8 shows 〈ϵI2〉R together with the random error of ϵI2 as defined by Eq. (24). Despite the strong scatter, the ground-truth reference is nearly always within the error bar of ϵI2, with some exceptions, e.g., or 60 000. It can also be seen that 〈ϵI2〉R is, if at all, only weakly correlated with the ground-truth reference for a window size of . This shows that it is extremely difficult, if at all possible, to track the true trend with low-resolution time records.
To assess this correlation more quantitatively, we evaluate the Pearson correlation coefficient between the ground-truth reference and ϵG, ϵI2 as well as ϵL, respectively, as a function of the re-scaled averaging window size for all virtual probes of case DNS 2.0. As an example, the Pearson correlation coefficient between ϵ0(x,t)〉R and ϵI2 is 0.33 in Fig. 8 (upper plot). Figure 9a shows the ensemble averages of the Pearson correlation coefficient together with the standard error (shaded area). While 〈ϵG〉R has a pronounced correlation with the ground-truth reference , both 〈ϵI2〉R and 〈ϵL〉R are only very weakly correlated with 〈ϵG〉R.
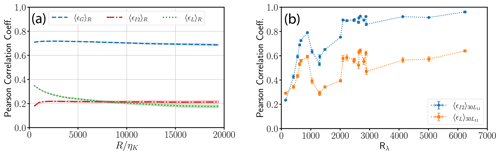
Figure 9(a) Dependence of the Pearson correlation coefficient between 〈ϵi〉R and as a function of the re-scaled averaging window where . Time records of the longitudinal velocity by all virtual probes and are taken from DNS 2.0 with Rλ=142, turbulence intensity I=10 % and perfect alignment (θ=0∘). The shaded region is given by the standard error. (b) Dependence of the Pearson correlation coefficient between 〈ϵI2,L〉R and 〈ϵG〉R as a function Rλ for a fixed re-scaled averaging window R=30L11. The error bars of the ensemble-averaged coefficients are given by the standard error.
The effect of Rλ on the Pearson correlation coefficient is also shown in Fig. 9b for the VDTT experiments at various Rλ values. Here, we compare ϵI2 and ϵL to ϵG in the absence of a ground-truth reference. To ensure a negligible systematic error, we chose a fixed averaging window of R=30L11 for each Rλ. Figure 9b shows that the correlation for ϵI2 is always higher than that of ϵL, except for very low Rλ. There is a non-monotonic behavior in the correlation coefficients in Fig. 9b that seems to be related to the skewness values shown in Fig. A6. Nonetheless, there is a clear increase in correlation coefficients with Rλ. Firstly, the random error in δI2(R) ranges from 20 % to 40 % at R=30L11. Secondly, the kurtosis of the instantaneous energy dissipation field scales with (Pope, 2000), which is why the variability in the instantaneous energy dissipation field increases with Rλ. Hence, at small and R=30L11, scatters only randomly around the global mean energy dissipation rate (with a 3 % standard deviation of ), which is why the correlation coefficient is low. In contrast, at large Rλ and R=30L11, the locally averaged mean energy dissipation rate fluctuates more strongly (≈30 % standard deviation of ) where δI2(R) is already comparable.
Up to this point, we have presented the results largely as is so that one can interpret them with minimal bias. However, the amount of data and details given may make the use of the results in practice difficult. Therefore, we propose here practical guidelines for measuring the energy dissipation rate from one-dimensional velocity records in atmospheric flows.
The gradient method should be preferred over other methods for conditions where the turbulence intensity is low and where the probe could be perfectly aligned in the direction of the mean wind. In particular, the gradient method is more sensitive to turbulence intensity than inertial-range methods due to random-sweeping effects. Low values of turbulence intensity and ideal alignment of probes can be best controlled in ground-based measurements. Measurements aboard research aircraft traveling at a true speed of 100 m s−1 can also satisfy these conditions, but the spatial and temporal resolution required to measure the velocity gradients (see Fig. A8) is challenging to achieve; i.e., a robust probe with a wire length ideally smaller than 1 mm (or of the same order as ηK) and a true response frequency of 105 Hz are needed. Other airborne platforms, such as helicopters, balloons and kites, would encounter higher turbulence intensities, making Taylor's frozen flow assumption more difficult to satisfy. Most importantly, however, they all suffer from probe alignment into the local wind at the scale of the measurement platform.
Other estimates based on inertial-sub-range methods (cf. Table 1) are less sensitive to a high turbulence intensity and probe alignment. Regarding the impact of a high turbulence intensity, we find the most reliable method for most field applications to be the second-order structure function. However the accuracy is at least 10 %, even in most ideal conditions considered here (Table 4). Considering experimental imperfections, the actual deviation is expected to be of the order of 100 % (Figs. 3 and 5).
Given atmospheric turbulence with ηK∼1 mm and L11∼100 m, the inertial range of the second-order structure function extends from about 60ηK (Pope, 2000) to at most the integral scale, if at all. However, for applying the second-order SF method (inertial range), at least 1 to 2 decades of the inertial range are needed. Assuming that the fitting range is chosen from 0.1 to 10 m, a measurement platform with a true air speed of 10–100 m s−1 requires an anemometer with a sampling frequency of 100–1000 Hz in order to provide about 2 decades of data within the inertial range.
Our analysis further shows that estimating the transient energy dissipation in atmospheric clouds (L11∼100 m, 𝒪(Rλ)∼103–104) via the second-order structure function (inertial range) with an averaging window of R=100 m is prone to random errors of the order of 100 % (Fig. 7b). Shorter averaging windows involve even higher random errors. It is therefore recommended to choose the averaging window as large as possible but still smaller than length and timescales on which the atmosphere remains homogeneous and stationary, respectively. This recommendation is not easy to achieve in an atmosphere. For example, measurements in the marine boundary layer with intermittent shallow cumulus clouds require a careful consideration in regard to stationary conditions. Then, two approaches can be recommended. On the one hand, one can choose a very long averaging window to average over many individual clouds associated with a small random error but potentially violating the stationary and homogeneity condition. On the other hand, one could investigate the transient mean energy dissipation rate (as a proxy for the local mean energy dissipation rate per cloud), while accepting a large random error.
The large random errors also preclude the possibility of interpreting trends in the measured energy dissipation rate via the second-order structure function if we consider the results shown in Figs. 8 and 9a. Even if we consider the promising correlations shown in Fig. 9b at high Reynolds numbers, interpreting trends and patterns in atmospheric data from one-dimensional time records remains a challenge, especially in an atmosphere with intermittent cloudiness. As an example, in a cloud with a horizontal extent of 1 km, one can obtain 10 non-overlapping data blocks with a window length of 100 m, which corresponds to a random error of about 100 % to be combined with a systematic error of the same order of magnitude. With 10 data points combined with such large uncertainties, it would be extremely difficult to interpret the change in the dissipation rate in this cloud. Interpretations of trends in the energy dissipation rate would be particularly flawed if the size of the averaging window is <10L11. At best, one average value can be determined for such small clouds, which would still deviate from the global mean by at least 50 %–100 % if (and this is a big if) the cloud is sufficiently stationary and homogeneous for averaging. However, the limitations imposed by the validity of the Taylor hypothesis can be mitigated by using an array of high-resolution anemometers. The array should be oriented so that the anemometers point in the mean local wind direction. The calculation of transverse velocity gradients or second-order structure functions would no longer depend on the applicability of the Taylor hypothesis, since the distances between the sensors are predefined by the design.
We have presented an extensive review on the analysis procedure for estimating the energy dissipation rate from single-point one-dimensional velocity time records along with an overview of the advantages and disadvantages (see Table 1). To conclude, this paper provides means to estimate errors in the global and local mean energy dissipation rates due to experimental imperfections and limitations. These estimated errors can be used to assess the quality and accuracy of the measurement. Furthermore, error estimates of the global and local mean energy dissipation rates can be used to assess the errors in other turbulence and cloud droplet parameters, e.g., various turbulence length scales and the cloud droplet Stokes number, with the help of Gaussian error propagation. A set of practical guidelines for measurement and analysis strategies has been provided in the previous section, and the following presents a technical summary of the main results.
The main methods considered in this study are the gradient method ϵG, the second-order SF (inertial-range) method ϵI2, the spectral method ϵS and the scaling argument ϵL. We have provided a systematic assessment of the accuracy of inferring the energy dissipation rate from such one-dimensional velocity time series as a function of turbulence intensity, probe orientation with respect to the longitudinal direction and the effect of a finite averaging window size. We used DNS data with Reynolds numbers in the range as well as experimental data from high-resolution one-dimensional wind tunnel measurements with Reynolds numbers in the range to evaluate the performance of different methods against robust benchmark values. The results presented in this study help to assess the accuracy of the energy-dissipation-rate estimates as a function of several parameters, such as finite turbulence intensity, misalignment between sensor and longitudinal flow direction, and finite size of the averaging window. The main results are as follows:
-
Each method could reproduce the ground-truth reference 〈ϵ(x,t)〉 to within less than 10 % for well-converged statistics and at low turbulence intensity. The most accurate method is the gradient method (ϵG), and the least accurate method is the one based on the law (ϵI3) (see Fig. 2). The reference-compared systematic error tends to be overestimated due to the global choice of the fit range; e.g., lower systematic errors for ϵI2 can be obtained by choosing a fit range for each DNS dataset that is in a range where the scaling of the structure function is closest to the expected scaling.
-
In the case of finite turbulence intensities, ϵG, ϵS and ϵI2 systematically overestimate the ground-truth energy dissipation rate. The gradient method (ϵG) is the most affected by a finite turbulence intensity I, whereas ϵI2 is the least affected (see Figs. 3 and 4b). The overestimation can be captured by a random-advection model (Fig. 4). Regarding the small-scale estimate ϵG, the error formula provided by Lumley (1965) (βG∝5I2) captures the effect of random advection.
-
Considering the probe orientation, the gradient method (ϵG) is the most affected by misalignment between the probe orientation and the longitudinal flow direction, whereas ϵI2 is the least affected (Fig. 5) (compare Eqs. 19, 17 and 18).
-
We provide scaling arguments δi(R) to estimate the required averaging window size optimized for a desired random-error threshold for ϵG in Eq. (29), ϵI2 in Eq. (28) and ϵL in Eq. (28). With this, we can estimate a coarse-grained energy dissipation rate to within a predicted uncertainty as shown in Fig. 8. Systematic errors βi are smaller than random errors δi(R) for R>2L11.
-
The random error in the gradient method δG(R) converges at least 4–5 times faster than ϵI2 (compare Eqs. 28 and 29).
-
Only ϵG estimates the transient energy dissipation rate 〈ϵ0〉R reliably, although it is the most vulnerable to experimental imperfections/limitations.
As discussed in detail below, the mean energy dissipation rate can be related to second-order statistics of the velocity field, either in terms of velocity gradients or in terms of velocity increments. In any case, the two-point velocity covariance tensor turns out to be the central quantity of interest from which the second-order structure function tensor, the spectral energy tensor and the velocity gradient covariance tensor can be obtained.
In the following, we assume a zero mean SHI turbulence so that two-point quantities depend only on the separation vector r. All averages are invariant under rotations of the coordinate system, and the mean squared velocity fluctuation is identical for all velocity components; i.e., . We provide an overview of the most relevant definitions, their notations and their conventions. This section does not explicitly discuss the effect of the averaging window, but the definitions presented can be applied to windowed inputs with no or straightforward modifications.
Under the given assumptions, the two-point velocity covariance tensor takes the form (e.g., Pope, 2000; Robertson, 1940; Batchelor, 1953)
where and are the longitudinal and transverse autocorrelation functions, respectively, with . Notably, if one chooses r=re1, then and as well as all other components vanish (e.g., Pope, 2000). As a remarkable consequence, Rij(r) is uniquely defined by f(r) in isotropic turbulence. As mentioned below, the integral length scale as well as the Taylor microscale are determined by f(r) (Pope, 2000).
Analogously, a covariance tensor can be defined for velocity increments, i.e., the second-order velocity structure function tensor (Pope, 2000; Davidson, 2015)
The longitudinal second-order structure function D11(r) is related to f(r) by (e.g., Pope, 2000; Davidson, 2015)
As explained below, by measuring the longitudinal second-order structure function DLL(r), the mean energy dissipation rate can be inferred from the inertial-range scaling of the longitudinal structure function (cf. Eq. 7).
Furthermore, the velocity gradient covariance tensor can also be defined in terms of the velocity covariance tensor
Since the local and instantaneous energy dissipation rate (cf. Eq. 3) is defined in terms of the strain-rate tensor , the mean energy dissipation rate can be directly related to contractions of the velocity gradient covariance tensor evaluated at zero. Note that in a turbulent flow with a zero mean velocity, the strain-rate tensor Sik is equal to the fluctuation strain-rate tensor sik.
The two-point velocity covariance tensor can be expressed in Fourier space through the energy spectrum tensor (Pope, 2000)
where κ is the wave vector. For SHI turbulence, Φij(κ) takes the form
where E(κ) is the energy spectrum function.
Since access to the full energy spectrum function is not always available, one-dimensional spectra are of interest, too. The mean energy dissipation rate can be estimated from the inertial-range scaling of the longitudinal one-dimensional spectrum (as shown in Eq. 9), which can be calculated by both the energy spectrum function and the velocity covariance tensor (Pope, 2000)
with the wavenumber κ1 corresponding to the e1 direction and .
This concludes the second-order statistics in terms of the velocity that we consider in the main text to determine the mean energy dissipation rate.
Table A1Nomenclature for the turbulent flow. If our naming convention differs from the terminology in Pope (2000), we add the convention of Pope (2000) in parentheses. Equations are either directly given or referenced from definitions below.
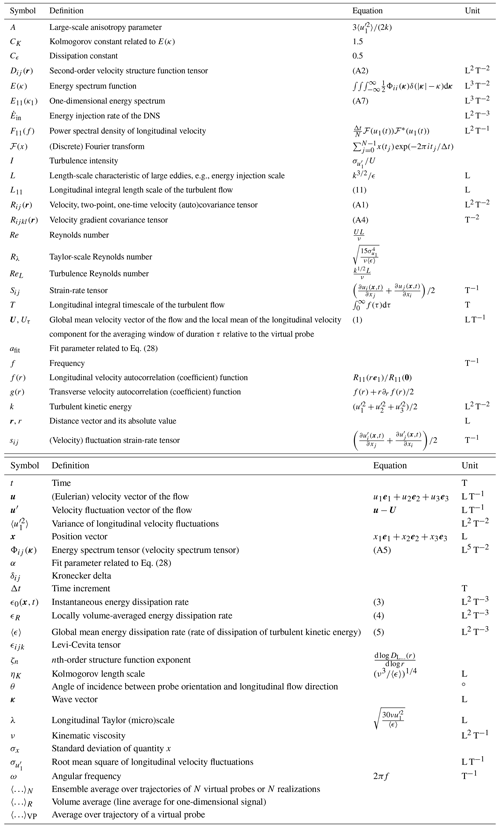
Table A3The systematic error in each method βi relative to the global mean energy dissipation rate in Eq. (12) of each DNS where . The error is given by the standard error, which is defined as the standard deviation divided by the square root of the number of samples. In both DNS 2.1 and DNS 3.1, ϵI2 and ϵI3 were obtained by fitting Eq. (7) for n=2 and n=3, respectively, in the range . This fit range is also used for calculating ϵS, and it was converted into the frequency domain by , where U is the mean velocity. In the case of DNS 1.1, the maximum of Eq. (7) was used to infer ϵI2 due to the absence of a pronounced inertial range. We used the maximum available window size R in all cases and fixed turbulence intensity I=1 % and considered perfect alignment; i.e., θ=0∘.

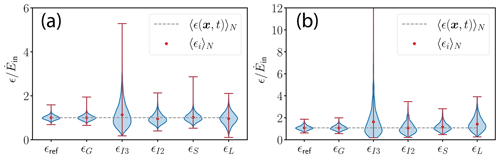
Figure A1Validation of estimating the energy dissipation rate from and ϵL. All estimates are re-scaled by the energy injection rate . The data are taken from DNS 1.1 (a) and 2.1 (b); turbulence intensity I=1 %. The ensemble mean of each method 〈ϵi〉N is denoted by red dots where the whiskers extend from the minimal to maximal estimate of ϵi where . As the inertial range of DNS 1.1 (I=1 %, θ=0∘ and maximal available averaging window) is not pronounced well due to the low Rλ∼74, we used the maximum of Eq. (7) in order to retrieve ϵI2,3. The dashed line represents the global mean energy dissipation rate of DNS 1.1 and 2.1 (Rλ=219, I=1 %, θ=0∘ and maximal available averaging window), respectively, which is approximated by the ensemble average of the true mean energy dissipation rate along the trajectory of each virtual probe. ϵref is the reference distribution of the ground-truth global mean energy dissipation field originating from the dissipation field along the trajectory of each virtual probe.
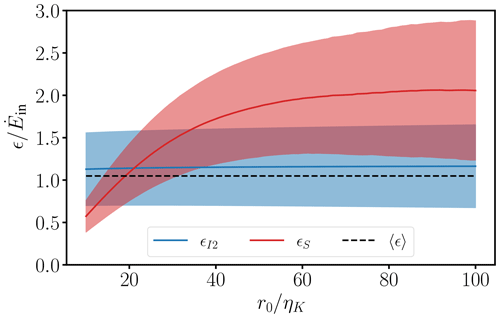
Figure A2Estimates of the mean energy dissipation rate as a function of the fit range for ϵI2 and ϵS for DNS 3.1 (1000 probes, Rλ=302, I=1 %, θ=0∘ and maximal available averaging window) re-scaled by the energy injection rate . The solid line represents the ensemble average, whereas the shaded region is given by the standard deviation. is the lower boundary of the fit range for ϵI2 where the upper boundary is fixed at r1=500ηK. For ϵS, the fit range is given by . The dashed line denotes the global mean energy dissipation rate.
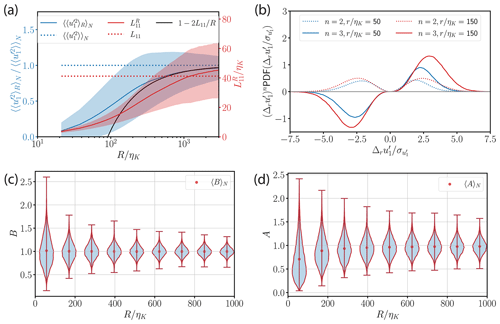
Figure A3Convergence of higher-order statistical quantities and longitudinal integral length scales as well as small- and large-scale anisotropy obtained from all virtual probes of DNS 1.1 (Rλ=74, I=1 %, θ=0∘). (a) The variance of the longitudinal velocity fluctuations and the longitudinal integral length scale as a function of the averaging window size R normalized by the Kolmogorov length scale ηK. is re-scaled by the ensemble-averaged variance of the longitudinal velocity fluctuations. For large R, converges to (blue-dotted line) and the systematic error in the variance (solid black line), Eq. (20), decays to zero. is the longitudinal integral length scale obtained from averaging windows of size R. For large R, should converge to L11 (red-dotted line), which is not fully achieved in this range of R. (b) Premultiplied PDFs of second- and third-order velocity increments over distances r=50ηK and r=150ηK. The tails of the premultiplied PDFs have decayed to zero for large (and re-scaled) increments so that they can globally be considered converged. (c) Small-scale anisotropy based on the ratio of longitudinal gradients to the instantaneous energy dissipation . In isotropic turbulence, B=1 on average. (d) Large-scale anisotropy parameter as a function of the averaging window R where k is the turbulent kinetic energy and the variance of the longitudinal velocity fluctuations. In isotropic turbulence, A=1 on average.
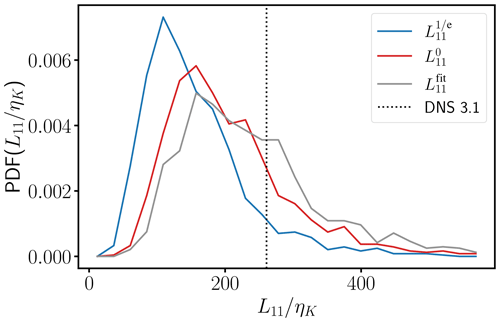
Figure A4Different estimates of the integral length from DNS 3.1 with Rλ=302, I=1 %, θ=0∘ and the maximal available averaging window. is inferred from integrating f(r) to its first zero, whereas refers to the integration of . extends f(r) with an exponential tail where the integration is performed up to infinity. The dotted black line is the reference from DNS 3.1 obtained by Eq. (11). All estimates are re-scaled by ηK.
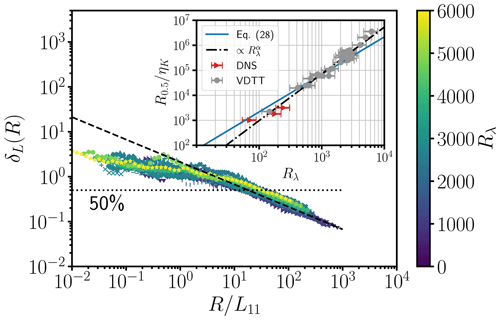
Figure A5Convergence of energy dissipation rate estimates for ϵL. The empirical random error δL(R) is plotted as a function of the re-scaled averaging window size from VDTT experiments at various Rλ values. The analytical result for the random error (Eq. 22) is shown by the dashed black line. Inset: the insets show the length of the averaging window in terms of ηK where δL(R) is less than 50 % as a function of the Taylor-microscale Reynolds number Rλ. The inset plot shows data from DNS 1.3, 2.3 and 3.3 (red triangles) and the VDTT (grey circle). The three red dots mark the experiments with the highest Rλ where the isotropy of the grid forcing in the VDTT is not guaranteed anymore. The solid blue line shows Eq. (28) resolved for . The double logarithmic fit () is performed for the scaling argument, resulting in and (dash-dotted black line).
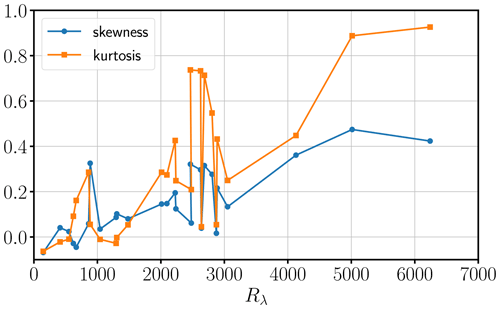
Figure A6Skewness and kurtosis of all VDTT experiments as a function of Rλ. The skewness vanishes for normally distributed velocity time records. Similarly, the kurtosis equals zero for normally distributed velocity time records, according to Fisher's convention, which is a measure for the excess kurtosis.
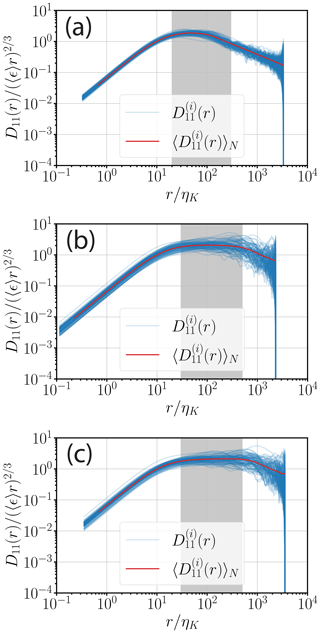
Figure A7Compensated longitudinal second-order structure functions for DNS 1.1 (a), DNS 2.1 (b) and DNS 3.1 (c). The grey shaded region represents the fit range (Eq. 7) for each DNS. The individual longitudinal second-order structure functions are calculated from the velocity time records along the e1 direction of each virtual probe (blue lines). The ensemble-averaged longitudinal second-order structure functions are shown in red.
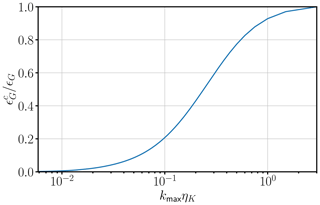
Figure A8Resolution effect on ϵG. refers to the coarse-grained velocity time record. Coarse graining is realized by taking only every nth value of the fully resolved velocity time record where , thereby controlling the resolution kmaxηK. Velocity data are taken from DNS 2.0 (Rλ=142, R≈32 000ηK, I=10 %, θ=0∘).
In the following, we illustrate how one obtains an expression for the impact of random-sweeping effects on the dissipation rate estimate in terms of the turbulence intensity using the gradient method (Lumley, 1965; Wyngaard and Clifford, 1977). We consider a model wave-number–frequency spectrum (Wilczek and Narita, 2012; Wilczek et al., 2014), which is based on the same modeling assumptions used in Wyngaard and Clifford (1977). It enables us to conduct a systematic assessment of the interplay between Taylor's hypothesis and the random-sweeping effects. The model wave-number–frequency spectrum tensor Φij(κ,ω) can be derived from an elementary linear random-advection model (Kraichnan, 1964; Wilczek and Narita, 2012; Wilczek et al., 2014), which in the case of SHI turbulence can be expressed in terms of the energy spectrum tensor Φij(κ):
Within the model, the wave-number–frequency spectrum Φij(κ,ω) consists of the energy spectrum tensor in wavenumber space Φij(κ) multiplied by a Gaussian frequency distribution. Φij(κ,ω) has a mean value determined by ω=κ1U, i.e., Taylor's hypothesis expressed in Fourier space, and a variance proportional to the turbulence intensity. When the turbulence intensity tends toward zero at a fixed mean velocity, the frequency distribution tends toward a delta function, re-establishing the one-to-one correspondence between the frequency and the wavenumber in the direction of the mean flow. To establish the connection to the different methods using longitudinal components and Taylor's hypothesis, we consider the component of Eq. (B1). One obtains the estimate for the longitudinal wavenumber spectrum based on Taylor's hypothesis, which includes the effect of random sweeping, by first integrating over the wave vector space. This leads to the frequency spectrum, which corresponds to the one obtained from temporal single-point measurements of the longitudinal velocity component. Then, one applies Taylor's hypothesis, corresponding to the substitution ω=κ1U, which leads to
Finally, this enables us to evaluate the influence of random sweeping on the gradient method since it is closely related to the wavenumber spectrum. Expressed in wavenumber space, the relation (Eq. 6) takes the following form, where we insert Eqs. (B1)–(B2) and solve the corresponding Gaussian integral over ω in the second step:
Hence, we recover, as expected, the result by Lumley (1965) and Wyngaard and Clifford (1977).
To numerically assess how random sweeping smears out the spectrum at finite turbulence intensities (see Fig. 4), we assumed a model wavenumber spectrum (Pope, 2000, Eq. 6.246 ff.):
where L is the energy injection scale and cL=6.78, p0=2 and β=2.094 are positive constants. Based on this model wavenumber spectrum, we first obtain E11(κ) through Eq. (A7). One can then evaluate Eq. (B2), resulting in the spectrum being smeared out by random sweeping.
Due to the misalignment, the probe frame of reference is rotated with respect to the frame given by the mean velocity. Without loss of generality, we assume that the misalignment is due to a rotation around the axis with the angle θ; see Fig. 5.
The misalignment has two consequences. First, the estimated mean velocity differs from the true mean velocity, which leads to errors when evaluating Taylor's hypothesis. Second, the measured velocity component is not the true velocity component but rather a combination of the longitudinal and transverse components.
In the probe frame of reference, we assume that we measure the longitudinal velocity field component, i.e., the component along the probe orientation . The mean of this component gives us the estimate of the mean velocity of the probe U′. Due to the misalignment between probe and true mean velocity, we underestimate the mean velocity compared to the true mean velocity U. As we apply Taylor's hypothesis to convert temporal increments into spatial distances, we therefore also underestimate the spatial distances:
Here denotes the estimated spatial distance and r the true distance that the probe moved.
The sampling direction of the probe, given by the direction of the mean velocity, e1, can be expressed in the probe frame of reference:
Combining these two aspects, the vectorial distance covered by the probe in terms of the estimated one, therefore, becomes
In the probe frame of reference, the covariance tensor is given by (see Eq. A1)
By application of Taylor's hypothesis, we can evaluate this tensor at . So the corresponding longitudinal component in terms of the estimated distance becomes
which, due to the misalignment, we interpret as the measured autocorrelation function. Then, the measured longitudinal integral length scale, Eq. (11), amounts to
where the integration of f(r) and g(r) is carried out in the last step (see Eq. 11) while considering the fact that for isotropic turbulence (Pope, 2000). Therefore, the energy injection scale estimate of the dissipation rate, Eq. (10), overestimates the dissipation rate due to misalignment as follows:
The same arguments that we applied to the covariance tensor, of course, also hold for the second-order structure function tensor, Eq. (A2). Due to the effects of misalignment, it takes the form
where in the inertial range of SHI turbulence the transverse second-order structure function can be expressed as (Pope, 2000). Therefore, the estimated dissipation rate using the second-order SF method (cf. Eq. 7) is affected by misalignment as
The misalignment error for the gradient method can be estimated analytically starting from the longitudinal component of the velocity gradient covariance tensor , Eq. (A4). Following similar arguments as above and starting from Eq. (A4), we obtain
where we expressed the estimated distance through the true one in the second line. Using (Pope, 2000), the velocity gradient covariance tensor reduces to
where (Pope, 2000) is used for the last step. The energy dissipation rate based on the gradient method, Eq. (6), is overestimated by the very same factor due to misalignment:
The code can be shared by the corresponding author upon request.
The data can be shared by the corresponding author upon request.
TB, MW and EB provided the velocity data. MS, EB, MW and GB conceptualized the study. MS developed, validated and ran the analysis code. The theoretical modeling was performed by MS, TB and MW. MS, TB, MW and GB analyzed and interpreted the data. MS, TB, MW and GB wrote the initial draft. MS, TB, EB, MW and GB proofread and edited the final paper.
The contact author has declared that none of the authors has any competing interests.
Publisher’s note: Copernicus Publications remains neutral with regard to jurisdictional claims in published maps and institutional affiliations.
We thank Christian Küchler and Gregory Bewley for providing us with VDTT data, and we thank David Kleinhans, Christian Küchler, Freja Nordsiek, Naseem Ali and Holger Nobach for helpful discussions. Furthermore, we thank Cristian C. Lalescu and Bérenger Bramas for their support and development of the TurTLE code used in this study. We are grateful for the support by the MPIDS High-Performance Computation (HPC) team for providing and maintaining computational resources.
This work was supported by the Fraunhofer–Max Planck cooperation program through the TWISTER project. Marcel Schröder was financially supported by the Konrad-Adenauer-Stiftung. Tobias Bätge was financially supported by a fellowship of the IMPRS for Physics of Biological and Complex Systems.
The article processing charges for this open-access publication were covered by the Max Planck Society.
This paper was edited by Luca Mortarini and reviewed by two anonymous referees.
Akinlabi, E. O., Wacławczyk, M., Mellado, J. P., and Malinowski, S. P.: Estimating turbulence kinetic energy dissipation rates in the numerically simulated stratocumulus cloud-top mixing layer: Evaluation of different methods, J. Atmos. Sci., 76, 1471–1488, 2019. a, b
Almalkie, S. and de Bruyn Kops, S. M.: Energy dissipation rate surrogates in incompressible Navier–Stokes turbulence, J. Fluid Mech., 697, 204–236, 2012. a, b
Antonia, R.: On estimating mean and instantaneous turbulent energy dissipation rates with hot wires, Exp. Therm. Fluid Sci., 27, 151–157, 2003. a, b, c
Bailey, S. C., Kunkel, G. J., Hultmark, M., Vallikivi, M., Hill, J. P., Meyer, K. A., Tsay, C., Arnold, C. B., and Smits, A. J.: Turbulence measurements using a nanoscale thermal anemometry probe, J. Fluid Mech., 663, 160–179, 2010. a
Batchelor, G. K.: The theory of homogeneous turbulence, Cambridge University Press, ISBN: 0521041171, 1953. a
Benzi, R., Ciliberto, S., Baudet, C., Chavarria, G. R., and Tripiccione, R.: Extended self-similarity in the dissipation range of fully developed turbulence, Europhys. Lett., 24, 275, https://doi.org/10.1209/0295-5075/24/4/007, 1993a. a
Benzi, R., Ciliberto, S., Tripiccione, R., Baudet, C., Massaioli, F., and Succi, S.: Extended self-similarity in turbulent flows, Phys. Rev. E, 48, R29, https://doi.org/10.1103/PhysRevE.48.R29, 1993b. a
Bewley, G. P., Chang, K., and Bodenschatz, E.: On integral length scales in anisotropic turbulence, Phys. Fluids, 24, 061702, https://doi.org/10.1063/1.4726077, 2012. a
Bodenschatz, E., Bewley, G. P., Nobach, H., Sinhuber, M., and Xu, H.: Variable density turbulence tunnel facility, Rev. Sci. Instrum., 85, 093908, https://doi.org/10.1063/1.4896138, 2014. a, b, c
Buaria, D., Pumir, A., Bodenschatz, E., and Yeung, P.-K.: Extreme velocity gradients in turbulent flows, New J. Phys., 21, 043004, https://doi.org/10.1088/1367-2630/ab0756, 2019. a
Burattini, P., Lavoie, P., and Antonia, R. A.: On the normalized turbulent energy dissipation rate, Phys. Fluids, 17, 098103, https://doi.org/10.1063/1.2055529, 2005. a, b
Chamecki, M. and Dias, N.: The local isotropy hypothesis and the turbulent kinetic energy dissipation rate in the atmospheric surface layer, Q. J. Roy. Meteor. Soc., 130, 2733–2752, 2004. a
Champagne, F.: The fine-scale structure of the turbulent velocity field, J. Fluid Mech., 86, 67–108, 1978. a
Cole, I. R.: Modelling CPV, PhD thesis, Loughborough University, https://hdl.handle.net/2134/18050 (last access: 30 March) 2015.
Davidson, P. A.: Turbulence: an introduction for scientists and engineers, Oxford University Press, ISBN 10019852949X, 2015. a, b, c, d
Deshpande, S. S., Sathe, M. J., and Joshi, J. B.: Evaluation of local turbulent energy dissipation rate using PIV in jet loop reactor, Ind. Eng. Chem. Res., 48, 5046–5057, 2009. a
Devenish, B., Bartello, P., Brenguier, J.-L., Collins, L., Grabowski, W. W., IJzermans, R., Malinowski, S. P., Reeks, M., Vassilicos, J., Wang, L.-P., and Warhaft, Z.: Droplet growth in warm turbulent clouds, Q. J. Roy. Meteor. Soc., 138, 1401–1429, 2012. a, b
Dodson, D. S. and Small Griswold, J. D.: Turbulent and boundary layer characteristics during VOCALS-REx, Atmos. Chem. Phys., 21, 1937–1961, https://doi.org/10.5194/acp-21-1937-2021, 2021. a
Donzis, D., Yeung, P., and Sreenivasan, K.: Dissipation and enstrophy in isotropic turbulence: resolution effects and scaling in direct numerical simulations, Phys. Fluids, 20, 045108, https://doi.org/10.1063/1.2907227, 2008. a
Elsner, J. and Elsner, W.: On the measurement of turbulence energy dissipation, Meas. Sci. Technol., 7, 1334, https://doi.org/10.1088/0957-0233/7/10/005, 1996. a, b, c, d
Fairall, C. and Larsen, S. E.: Inertial-dissipation methods and turbulent fluxes at the air-ocean interface, Bound.-Lay. Meteorol., 34, 287–301, 1986. a
Fairall, C., Markson, R., Schacher, G., and Davidson, K.: An aircraft study of turbulence dissipation rate and temperature structure function in the unstable marine atmospheric boundary layer, Bound.-Lay. Meteorol., 19, 453–469, 1980. a
Fodor, K. and Mellado, J. P.: New Insights into Wind Shear Effects on Entrainment in Convective Boundary Layers Using Conditional Analysis, J. Atmos. Sci., 77, 3227–3248, 2020. a
Frehlich, R., Meillier, Y., Jensen, M. L., and Balsley, B.: Turbulence measurements with the CIRES tethered lifting system during CASES-99: Calibration and spectral analysis of temperature and velocity, J. Atmos. Sci., 60, 2487–2495, 2003. a
Frehlich, R., Meillier, Y., Jensen, M. L., and Balsley, B.: A statistical description of small-scale turbulence in the low-level nocturnal jet, J. Atmos. Sci., 61, 1079–1085, 2004. a
Freire, L. S., Dias, N. L., and Chamecki, M.: Effects of path averaging in a sonic anemometer on the estimation of turbulence-kinetic-energy dissipation rates, Bound.-Lay. Meteorol., 173, 99–113, 2019. a
Garratt, J. R.: The atmospheric boundary layer, Earth-Sci. Rev., 37, 89–134, 1994. a
Gerber, H., Frick, G., Malinowski, S. P., Jonsson, H., Khelif, D., and Krueger, S. K.: Entrainment rates and microphysics in POST stratocumulus, J. Geophys. Res.-Atmos., 118, 12094–12109, https://doi.org/10.1002/jgrd.50878, 2013. a
Gerber, H. E., Frick, G. M., Jensen, J. B., and Hudson, J. G.: Entrainment, mixing, and microphysics in trade-wind cumulus, J. Meteorol. Soc. Jpn., Ser. II, 86, 87–106, 2008. a
Griffin, K. P., Wei, N. J., Bodenschatz, E., and Bewley, G. P.: Control of long-range correlations in turbulence, Exp. Fluids, 60, 55, https://doi.org/10.1007/s00348-019-2698-1, 2019. a
Hamlington, P. E., Poludnenko, A. Y., and Oran, E. S.: Intermittency in premixed turbulent reacting flows, Phys. Fluids, 24, 075111, https://doi.org/10.1063/1.4729615, 2012. a
Hanson, J.: Rotations in three, four, and five dimensions, arXiv [preprint], https://doi.org/10.48550/arXiv.1103.5263, 8 March 2011.
Horst, T. and Oncley, S.: Corrections to inertial-range power spectra measured by CSAT3 and Solent sonic anemometers, 1. Path-averaging errors, Bound.-Lay. Meteorol., 119, 375–395, 2006. a
Hsieh, C.-I. and Katul, G. G.: Dissipation methods, Taylor's hypothesis, and stability correction functions in the atmospheric surface layer, J. Geophys. Res.-Atmos., 102, 16391–16405, 1997. a
Jørgensen, F. E.: How to measure turbulence with hot-wire anemometers: a practical guide, Dantec Dynamics, 2001. a
Kolmogorov, A. N.: The local structure of turbulence in incompressible viscous fluid for very large Reynolds numbers, Dokl. Akad. Nauk SSSR, 30, 301–305, 1941 (in Russian); Proc. R. Soc. A, 434, 9–13 (English transl.), 1991. a, b, c, d
Kolmogorov, A. N.: A refinement of previous hypotheses concerning the local structure of turbulence in a viscous incompressible fluid at high Reynolds number, J. Fluid Mech., 13, 82–85, 1962. a
Kolmogorov, A. N.: Dissipation of energy in the locally isotropic turbulence, Dokl. Akad. Nauk SSSR, 32, 16–18, 1941 (in Russian); Proc. R. Soc. A, 434, 15–17, 1991 (English transl.). a
Kraichnan, R. H.: Kolmogorov's hypotheses and Eulerian turbulence theory, Phys. Fluids, 7, 1723–1734, 1964. a, b
Küchler, C.: Measurements of Turbulence at High Reynolds Numbers, PhD thesis, Georg-August-Universität Göttingen, https://doi.org/10.53846/goediss-8490, 2021. a, b, c
Küchler, C., Bewley, G., and Bodenschatz, E.: Experimental study of the bottleneck in fully developed turbulence, J. Stat. Phys., 175, 617–639, 2019. a, b, c
Küchler, C., Bodenschatz, E., and Bewley, G. P.: Scaling in Decaying Turbulence at High Reynolds Numbers, arXiv [preprint], https://doi.org/10.48550/arXiv.2006.10993, 19 June 2020. a
Lalescu, C., Teaca, B., and Carati, D.: Implementation of high order spline interpolations for tracking test particles in discretized fields, J. Comput. Phys., 229, 5862–5869, https://doi.org/10.1016/j.jcp.2009.10.046, 2010. a
Lalescu, C. C., Bramas, B., Rampp, M., and Wilczek, M.: An efficient particle tracking algorithm for large-scale parallel pseudo-spectral simulations of turbulence, Comput. Phys. Commun., 278, 108406, https://doi.org/10.1016/j.cpc.2022.108406, 2022. a, b
Lefeuvre, N., Thiesset, F., Djenidi, L., and Antonia, R.: Statistics of the turbulent kinetic energy dissipation rate and its surrogates in a square cylinder wake flow, Phys. Fluids, 26, 095104, https://doi.org/10.1063/1.4895637, 2014. a
Lenschow, D., Mann, J., and Kristensen, L.: How long is long enough when measuring fluxes and other turbulence statistics?, J. Atmos. Ocean. Tech., 11, 661–673, 1994. a, b, c, d, e, f
Lenschow, D. H. and Kristensen, L.: Uncorrelated noise in turbulence measurements, J. Atmos. Ocean. Tech., 2, 68–81, 1985. a
Lewis, J. M., Koster, T. W., and LaRue, J. C.: On the determination of the dissipation rate of turbulence kinetic energy, Exp. Fluids, 62, 1–13, 2021. a, b
Li, X.-Y., Brandenburg, A., Svensson, G., Haugen, N. E., Mehlig, B., and Rogachevskii, I.: Condensational and collisional growth of cloud droplets in a turbulent environment, J. Atmos. Sci., 77, 337–353, 2020. a
Lumley, J.: Interpretation of time spectra measured in high-intensity shear flows, Phys. Fluids, 8, 1056–1062, 1965. a, b, c, d, e, f, g, h, i
Lumley, J.: Some comments on turbulence, Phys. Fluids A-Fluid, 4, 203–211, 1992. a
Malinowski, S. P., Gerber, H., Jen-La Plante, I., Kopec, M. K., Kumala, W., Nurowska, K., Chuang, P. Y., Khelif, D., and Haman, K. E.: Physics of Stratocumulus Top (POST): turbulent mixing across capping inversion, Atmos. Chem. Phys., 13, 12171–12186, https://doi.org/10.5194/acp-13-12171-2013, 2013. a
McComb, W. D., Berera, A., Salewski, M., and Yoffe, S.: Taylor's (1935) dissipation surrogate reinterpreted, Phys. Fluids, 22, 061704, https://doi.org/10.1063/1.3450299, 2010. a
Muschinski, A. and Lenschow, D. H.: Future directions for research on meter-and submeter-scale atmospheric turbulence, B. Am. Meteorol. Soc., 82, 2831–2843, 2001. a
Muschinski, A., Frehich, R., Jensen, M., Hugo, R., Hoff, A., Eaton, F., and Balsley, B.: Fine-scale measurements of turbulence in the lower troposphere: An intercomparison between a kite-and balloon-borne, and a helicopter-borne measurement system, Bound.-Lay. Meteorol., 98, 219–250, 2001. a
Muschinski, A., Frehlich, R. G., and Balsley, B. B.: Small-scale and large-scale intermittency in the nocturnal boundary layer and the residual layer, J. Fluid Mech., 515, 319–351, https://doi.org/10.1017/S0022112004000412, 2004. a, b, c, d
Nobach, H. and Tropea, C.: A statistical method for transforming temporal correlation functions from one-point measurements into longitudinal spatial and spatio-temporal correlation functions, Exp. Fluids, 53, 1815–1821, 2012. a
Nowak, J. L., Siebert, H., Szodry, K.-E., and Malinowski, S. P.: Coupled and decoupled stratocumulus-topped boundary layers: turbulence properties, Atmos. Chem. Phys., 21, 10965–10991, https://doi.org/10.5194/acp-21-10965-2021, 2021. a
Obukhov, A.: Some specific features of atmospheric turbulence, J. Geophys. Res., 67, 3011–3014, 1962. a
O'Connor, E. J., Illingworth, A. J., Brooks, I. M., Westbrook, C. D., Hogan, R. J., Davies, F., and Brooks, B. J.: A method for estimating the turbulent kinetic energy dissipation rate from a vertically pointing Doppler lidar, and independent evaluation from balloon-borne in situ measurements, J. Atmos. Ocean. Tech., 27, 1652–1664, 2010. a, b
Oncley, S. P., Friehe, C. A., Larue, J. C., Businger, J. A., Itsweire, E. C., and Chang, S. S.: Surface-layer fluxes, profiles, and turbulence measurements over uniform terrain under near-neutral conditions, J. Atmos. Sci., 53, 1029–1044, 1996. a
Pearson, B., Krogstad, P.-Å., and Van De Water, W.: Measurements of the turbulent energy dissipation rate, Phys. Fluids, 14, 1288–1290, 2002. a
Pope, S. B.: Turbulent flows, Cambridge University Press, https://doi.org/10.1017/CBO9780511840531, 2000. a, b, c, d, e, f, g, h, i, j, k, l, m, n, o, p, q, r, s, t, u, v, w, x, y, z, aa, ab, ac, ad, ae, af
Pumir, A. and Wilkinson, M.: Collisional aggregation due to turbulence, Annu. Rev. Conden. Ma. P., 7, 141–170, 2016. a
Risius, S., Xu, H., Di Lorenzo, F., Xi, H., Siebert, H., Shaw, R. A., and Bodenschatz, E.: Schneefernerhaus as a mountain research station for clouds and turbulence, Atmos. Meas. Tech., 8, 3209–3218, https://doi.org/10.5194/amt-8-3209-2015, 2015. a, b, c, d, e, f
Robertson, H.: The invariant theory of isotropic turbulence, in: Mathematical Proceedings of the Cambridge Philosophical Society, Cambridge University Press, 36, 209–223, https://doi.org/10.1017/S0305004100017199, 1940. a
Shaw, R. A.: Particle-turbulence interactions in atmospheric clouds, Annu. Rev. Fluid Mech., 35, 183–227, 2003. a, b
Siebert, H., Lehmann, K., and Wendisch, M.: Observations of small-scale turbulence and energy dissipation rates in the cloudy boundary layer, J. Atmos. Sci., 63, 1451–1466, 2006. a, b, c, d, e, f, g
Siebert, H., Gerashchenko, S., Gylfason, A., Lehmann, K., Collins, L., Shaw, R., and Warhaft, Z.: Towards understanding the role of turbulence on droplets in clouds: in situ and laboratory measurements, Atmos. Res., 97, 426–437, 2010. a
Siebert, H., Beals, M., Bethke, J., Bierwirth, E., Conrath, T., Dieckmann, K., Ditas, F., Ehrlich, A., Farrell, D., Hartmann, S., Izaguirre, M. A., Katzwinkel, J., Nuijens, L., Roberts, G., Schäfer, M., Shaw, R. A., Schmeissner, T., Serikov, I., Stevens, B., Stratmann, F., Wehner, B., Wendisch, M., Werner, F., and Wex, H.: The fine-scale structure of the trade wind cumuli over Barbados – an introduction to the CARRIBA project, Atmos. Chem. Phys., 13, 10061–10077, https://doi.org/10.5194/acp-13-10061-2013, 2013. a, b
Siebert, H., Shaw, R. A., Ditas, J., Schmeissner, T., Malinowski, S. P., Bodenschatz, E., and Xu, H.: High-resolution measurement of cloud microphysics and turbulence at a mountaintop station, Atmos. Meas. Tech., 8, 3219–3228, https://doi.org/10.5194/amt-8-3219-2015, 2015. a
Sinhuber, M.: On the scales of turbulent motion at high Reynolds numbers, PhD thesis, Georg-August-Universität Göttingen, https://doi.org/10.53846/goediss-5420, 2015. a, b, c
Sinhuber, M., Bodenschatz, E., and Bewley, G. P.: Decay of turbulence at high Reynolds numbers, Phys. Rev. Lett., 114, 034501, https://doi.org/10.1103/PhysRevLett.114.034501, 2015. a
Smalikho, I., Banakh, V., Pichugina, Y., Brewer, W., Banta, R., Lundquist, J., and Kelley, N.: Lidar investigation of atmosphere effect on a wind turbine wake, J. Atmos. Ocean. Tech., 30, 2554–2570, 2013. a
Sreenivasan, K.: Possible effects of small-scale intermittency in turbulent reacting flows, Flow Turbul. Combust., 72, 115–131, 2004. a, b
Sreenivasan, K., Prabhu, A., and Narasimha, R.: Zero-crossings in turbulent signals, J. Fluid Mech., 137, 251–272, 1983. a
Sreenivasan, K., Deshpande, S., Prabhu, A., and Viswanath, P.: The energy dissipation in turbulent shear flows, in: Symposium on Developments in Fluid Dynamics and Aerospace Engineering, Viswanath, Bangalore, 9–10 December 1993, Interline Publishers, 159–190, 1995. a, b, c
Sreenivasan, K. R.: On the scaling of the turbulence energy dissipation rate, Phys. Fluids, 27, 1048–1051, 1984. a
Sreenivasan, K. R.: On the universality of the Kolmogorov constant, Phys. Fluids, 7, 2778–2784, 1995. a, b
Sreenivasan, K. R.: An update on the energy dissipation rate in isotropic turbulence, Phys. Fluids, 10, 528–529, 1998. a, b, c, d
Sreenivasan, K. R.: Turbulent mixing: A perspective, P. Natl. Acad. Sci. USA, 116, 18175–18183, 2019. a
Sreenivasan, K. R. and Antonia, R.: The phenomenology of small-scale turbulence, Annu. Rev. Fluid Mech., 29, 435–472, 1997. a
Taylor, G. I.: Statistical theory of turbulenc, P. Roy. Soc. Lond. A Mat., 151, 421–444, 1935. a, b, c
Taylor, G. I.: The spectrum of turbulence, P. Roy. Soc. Lond. A Mat., 164, 476–490, 1938. a, b, c, d, e
Tennekes, H.: Eulerian and Lagrangian time microscales in isotropic turbulence, J. Fluid Mech., 67, 561–567, 1975. a, b
Tritton, D.: Physical Fluid Dynamics, https://doi.org/10.1007/978-94-009-9992-3, 1977. a
Vallikivi, M., Hultmark, M., Bailey, S. C. C., and Smits, A.: Turbulence measurements in pipe flow using a nano-scale thermal anemometry probe, Exp. Fluids, 51, 1521–1527, 2011. a
Vassilicos, J. C.: Dissipation in turbulent flows, Annu. Rev. Fluid Mech., 47, 95–114, 2015. a, b, c
Wacławczyk, M., Ma, Y.-F., Kopeć, J. M., and Malinowski, S. P.: Novel approaches to estimating the turbulent kinetic energy dissipation rate from low- and moderate-resolution velocity fluctuation time series, Atmos. Meas. Tech., 10, 4573–4585, https://doi.org/10.5194/amt-10-4573-2017, 2017. a, b, c
Wacławczyk, M., Gozingan, A. S., Nzotungishaka, J., Mohammadi, M., and Malinowski, S. P.: Comparison of Different Techniques to Calculate Properties of Atmospheric Turbulence from Low-Resolution Data, Atmosphere, 11, 199, https://doi.org/10.3390/atmos11020199, 2020. a, b, c
Warhaft, Z.: Passive scalars in turbulent flows, Annu. Rev. Fluid Mech., 32, 203–240, 2000. a
Wilczek, M. and Narita, Y.: Wave-number–frequency spectrum for turbulence from a random sweeping hypothesis with mean flow, Phys. Rev. E, 86, 066308, https://doi.org/10.1103/PhysRevE.86.066308, 2012. a, b, c, d
Wilczek, M., Xu, H., and Narita, Y.: A note on Taylor's hypothesis under large-scale flow variation, Nonlin. Processes Geophys., 21, 645–649, https://doi.org/10.5194/npg-21-645-2014, 2014. a, b, c, d, e, f, g
Willis, G. and Deardorff, J.: On the use of Taylor's translation hypothesis for diffusion in the mixed layer, Q. J. Roy. Meteor. Soc., 102, 817–822, 1976. a
Wyngaard, J. and Clifford, S.: Taylor's hypothesis and high–frequency turbulence spectra, J. Atmos. Sci., 34, 922–929, 1977. a, b, c, d, e, f, g, h, i, j
Wyngaard, J. C.: Atmospheric turbulence, Annu. Rev. Fluid Mech., 24, 205–234, 1992. a, b
Wyngaard, J. C.: Turbulence in the Atmosphere, Cambridge University Press, https://doi.org/10.1017/CBO9780511840524, 2010. a, b, c
- Abstract
- Introduction
- Methods
- Results and discussion
- Practical guidelines
- Summary
- Appendix A: Preliminaries on second-order statistics
- Appendix B: Impact of random-sweeping effects on the gradient method
- Appendix C: Effect of probe misalignment
- Code availability
- Data availability
- Author contributions
- Competing interests
- Disclaimer
- Acknowledgements
- Financial support
- Review statement
- References
- Abstract
- Introduction
- Methods
- Results and discussion
- Practical guidelines
- Summary
- Appendix A: Preliminaries on second-order statistics
- Appendix B: Impact of random-sweeping effects on the gradient method
- Appendix C: Effect of probe misalignment
- Code availability
- Data availability
- Author contributions
- Competing interests
- Disclaimer
- Acknowledgements
- Financial support
- Review statement
- References