the Creative Commons Attribution 4.0 License.
the Creative Commons Attribution 4.0 License.
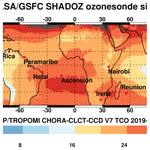
Improved convective cloud differential (CCD) tropospheric ozone from S5P-TROPOMI satellite data using local cloud fields
Swathi Maratt Satheesan
Kai-Uwe Eichmann
John P. Burrows
Mark Weber
Ryan Stauffer
Anne M. Thompson
Debra Kollonige
We present the CHORA (Cloud Height Ozone Reference Algorithm) for retrieving tropospheric-ozone columns from S5P-TROPOMI (Sentinel-5 Precursor–TROPOspheric Monitoring Instrument). The method uses a local-cloud reference sector (CLC – CHORA Local Cloud) to determine the stratospheric (above-cloud) column, which is subtracted from the total column in clear-sky scenes in the same zonal band to retrieve the tropospheric column. The standard CCD (convective cloud differential) approach uses cloud data from the Pacific region (CPC – CHORA Pacific Cloud) instead. An important assumption for the standard method is the zonal invariance of stratospheric ozone. The local-cloud approach is the first step to diminish this constraint in order to extend the CCD method to mid-latitudes, where stratospheric-ozone variability is larger. An iterative approach has been developed for the automatic selection of an optimal local-cloud reference sector around each retrieval grid box varying latitudinally by ± 1° and longitudinally between ± 5 and ± 50°. The optimised CLCT (CHORA Local Cloud Theil–Sen) algorithm, a follow-up from the CLC, employs a homogeneity criterion for total ozone from the cloud reference sector in order to overcome the inhomogeneities in stratospheric ozone. It directly estimates the above-cloud column ozone for a common reference altitude of 270 hPa using the Theil–Sen regression. The latter allows for the combination of the CCD method with the cloud-slicing algorithm that retrieves upper-tropospheric ozone volume mixing ratios. Monthly averaged tropospheric-column ozone (TCO) using the Pacific cloud reference sector (CPC) and the local-cloud reference sector (CLC, CLCT) has been determined over the tropics and subtropics (26° S–22° N) using TROPOMI for the time period from 2018 to 2022. The accuracy of the various methods was investigated by means of comparisons with spatially collocated NASA/GSFC SHADOZ (Southern Hemisphere Additional Ozonesondes) measurements and the ESA TROPOMI level-2 tropospheric-ozone product. At eight out of nine tropical stations, tropospheric-ozone columns using the CLCT yield better agreement with ozonesondes than the CPC. In the tropical region (20° S–20° N), the CLCT shows a significantly lower overall mean bias and dispersion of 1 ± 7 %, outperforming both the CPC (12 ± 10 %) and CCD-ESA (22 ± 10 %). The CLCT surpasses the ESA operational product, providing more accurate tropospheric-ozone retrievals at eight out of nine stations in the tropics. For the Hilo station, with a larger stratospheric-ozone variability due to its proximity to the subtropics, the bias of +30 % (CPC) is effectively reduced to −5 % (CLCT). Similarly, in the subtropics (Reunion, Irene, Hanoi, and King's Park), the CLCT algorithm provides an overall bias and scatter of −11 ± 9 % with respect to sondes. The CLCT effectively reduces the impact of stratospheric-ozone inhomogeneity, typically at higher latitudes. These results demonstrate the advantage of the local-cloud reference sector in the subtropics. The algorithm is therefore an important basis for subsequent systematic applications in current and future missions of geostationary satellites, like GEMS (Geostationary Environment Monitoring Spectrometer, Korea), ESA Sentinel-4, and NASA TEMPO (Tropospheric Emissions: Monitoring of POllution), predominantly covering the middle latitudes.
- Article
(9914 KB) - Full-text XML
-
Supplement
(1219 KB) - BibTeX
- EndNote
Tropospheric ozone is one of the most important pollutants and greenhouse gases in the Earth’s atmosphere. At the top of the troposphere, it acts as a greenhouse gas and contributes to global warming. When it appears closer to the Earth’s surface, it adversely affects the air quality and is hazardous to the health of humans, animals, and vegetation (Crutzen, 2016; Iriti and Faoro, 2008; Fleming et al., 2018; Mills et al., 2018; Gaudel et al., 2018; Szopa et al., 2021). Tropospheric ozone is a short-lived secondary gas with an atmospheric lifetime of hours to weeks, with no direct emission sources. The downward transport of ozone from the stratosphere and the emission of nitrogen oxides and hydrocarbons by the chemical reaction cycles forced by solar radiation contribute to ozone abundance in the troposphere and, in turn, result in a degradation of air quality on a global scale (Monks et al., 2015; Škerlak et al., 2014). Since the 1960s, ozonesonde records have revealed that tropospheric-ozone trends are mainly positive in the northern mid-latitudes and tropics, except over the Arctic and mid-latitude regions of Canada (Cooper et al., 2020; Oltmans et al., 2013). Since 1998, free-tropospheric trends in the tropical regions observed by the Southern Hemisphere Additional Ozonesondes (SHADOZ) network have shown more moderate variations, which vary with the seasons and across different regions (Thompson et al., 2021). The relatively short atmospheric lifetime of tropospheric ozone and its strong warming potential imply that proposed strategies to reduce such emissions can contribute to climate and health benefits within a few decades. Therefore, the precise quantitative analysis of the tropospheric-ozone levels is crucial for assisting policymakers in developing effective prevention strategies (Jaffe et al., 2018).
Even though ground-based techniques, ozonesondes, and lidars (light detection and ranging) are considered to be the most common and accurate methods to measure vertical ozone profiles, only satellite observations ensure the continuous global monitoring of ozone with sufficient spatial and temporal coverage. However, due to its short lifetime and dependence on sunlight and precursor emissions from natural and anthropogenic sources, tropospheric ozone exhibits a high spatio-temporal variability on seasonal, interannual, and decadal timescales (Cooper et al., 2014; Putero et al., 2023; Seguel et al., 2024), which, in turn, poses a clear challenge to the satellite observing system. The proper implementation of ozone retrieval algorithms in the satellite sensors helps to overcome this issue to a certain degree by improving the accuracy of measurements. The convective cloud differential (CCD) (Ziemke et al., 1998) and cloud-slicing algorithms (CSAs) (Ziemke et al., 2005) are two standard tropospheric-ozone retrieval methods limited to the tropical band (20° S–20° N). In particular, the CCD approach has been successfully applied to currently operating satellite sensors such as Aura OMI, MetOp GOME-2, and Sentinel-5 Precursor TROPOMI (TROPOspheric Monitoring Instrument) to derive tropospheric-ozone columns (Valks et al., 2003, 2014; Ziemke et al., 2010; Heue et al., 2016; Leventidou et al., 2016, 2018; Hubert et al., 2021).
The Sentinel-5 Precursor (S5P) satellite was launched in October 2017 to bridge the gap between Heritage and Sentinel-5 satellite series, providing information on and services for air quality, climate forcing, and the ozone layer in the time frame after 2018. The TROPOspheric Monitoring Instrument (TROPOMI) on board the Copernicus S5P satellite provides highly spatially resolved observations of key atmospheric constituents, including total ozone and cloud properties, which are needed to derive tropospheric ozone using the CCD method (Veefkind et al., 2012).
This study introduces the CHORA-CCD (Cloud Height induced Ozone Reference Algorithm) for retrieving tropospheric-ozone columns. It takes advantage of the high-resolution TROPOMI ozone and cloud data (minimum of 3.5 × 5.5 km2 in nadir geometry). The new method uses the local-cloud reference sector (CLC) instead of the standard approach using that of the Pacific region (CPC) to calculate the stratospheric- and/or above-cloud column ozone. The CLC algorithm is further optimised by introducing a homogeneity criterion and an alternative method to directly estimate the above-cloud column by using the Theil–Sen regression (CLCT) in order to interpolate to the tropospheric column from the ground to the reference altitude at 270 hPa. This local-cloud approach is the first step towards extending the CCD method to middle latitudes.
This paper is structured as follows. The measurement data taken from S5P-TROPOMI and used for deriving the tropospheric-ozone columns are described in Sect. 2. Spatially collocated ozonesonde measurements used as a reference for validation are described in Sect. 3. The standard tropospheric-ozone retrieval algorithm (CHORA) and the changes to the local-cloud algorithms (CLC, CLCT) are explained in Sect. 4. Section 5 compares tropospheric-ozone retrievals by all three algorithms (including the CPC) of CHORA with respect to ozonesondes at 13 ground stations and analyses the possible sources of biases and uncertainties. In Sect. 6, all major findings are recollected and discussed to derive conclusions on the CLCT algorithm's efficiency. Section 7 describes further modifications required to extend the applicability to mid-latitudes.
2.1 S5P-TROPOMI
The Copernicus Sentinel-5 Precursor (S5P) mission is the first Copernicus mission dedicated to monitoring the atmospheric composition (Ingmann et al., 2012). The TROPOspheric Monitoring Instrument (TROPOMI) on board the S5P satellite is a space-borne nadir-viewing imager with four separate spectrometers covering wavelength bands between the ultraviolet and the shortwave infrared (270–2385 nm). TROPOMI was launched on 13 October 2017 and flies in a near-polar, sun-synchronous orbit with an ascending node and Equator-crossing local time of 13:30 h. The instrument uses passive remote sensing techniques to measure the solar radiation reflected by and radiated from the Earth at the top of the atmosphere. By operating in a push-broom configuration with a wide swath of 108°, along with a near-polar orbit at 824 km altitude, TROPOMI achieves complete global surface coverage daily. The spatial resolution at nadir, originally 3.5 × 7 km2, was further refined to 3.5 × 5.5 km2 (across-track × along-track) on 6 August 2019. From the spectral and radiometric calibration of the Earth's radiance and solar irradiance data, it is possible to retrieve information on the total, tropospheric, and stratospheric total column densities of several atmospheric trace gases and surface reflectances (Kleipool et al., 2018; Ludewig et al., 2020). In particular, TROPOMI operational products include the vertical column amount of ozone and the cloud parameters required for the computation of tropospheric ozone by applying the CCD technique (Kleipool et al., 2018; Hubert et al., 2021).
A total of 5 years of TROPOMI total ozone column data (1 June 2018 to 31 December 2022), corresponding to the reprocessed/offline processor version 02.04.01, have been used in this study (ESA, 2022; Hubert et al., 2021). The ozone columns used for the tropospheric-ozone computations are retrieved by the GODFIT (GOme Direct FITting) algorithm version 4 based on ozone absorption in the UV Huggins bands (325–335 nm) (Van Roozendael et al., 2012; Lerot et al., 2010, 2014; Garane et al., 2019; Heue et al., 2022; ESA, 2022). This algorithm uses an iterative least-squares cost function minimisation approach based on the differences between satellite-measured and model-simulated radiances.
The cloud information used as input to the TROPOMI-S5P total ozone algorithm and later tropospheric-ozone computations are retrieved through a combination of the OCRA (Optical Cloud Recognition Algorithm) (Loyola and Ruppert, 1998) and ROCINN (Retrieval of Cloud Information using Neural Networks) algorithm (Rodriguez et al., 2007). The main product of the OCRA is the cloud fraction (between 0 and 1), determined through comparisons of broadband reflectance measurements in the UV–VIS–NIR spectral regions, with those from a global cloud-free composite data set containing minimum reflectance data (Loyola et al., 2018; Compernolle et al., 2021; ESA, 2021a). The ROCINN algorithm, using as input the OCRA-derived cloud fraction, retrieves cloud top height and cloud albedo as primary quantities, determined through a classical inversion method based on measurements in and around the O2 A-band. The ROCINN version 2.0 is based on the assumption that clouds are Lambertian reflecting surfaces. This is known as the “clouds-as-reflecting-boundaries” (ROCINN-CRB) model (Van Roozendael et al., 2006; Loyola et al., 2011). In this study, cloud fraction is obtained from the OCRA algorithm, and effective cloud top height is obtained from the ROCINN-CRB algorithm (ESA, 2021a). For simplicity, we will refer to the effective cloud top height as cloud top height.
3.1 Ozonesondes
Ozonesondes are balloon-borne instruments that provide accurate measurements of ozone and standard meteorological quantities such as pressure, temperature, and humidity as the balloon ascends through the atmosphere. They can reach up to altitudes of about 35 km before the balloon bursts. The vertical resolution is about 100–200 m. The main part of the ozonesonde is an electrochemical concentration cell (ECC) that senses the ozone concentration of the air sample by producing a corresponding weak electrical current. Due to the very high vertical resolution and high accuracy, ozonesondes are very well suited for validating satellite observations (Huang et al., 2017; Hubert et al., 2021; Tarasick et al., 2021).
The ozonesonde stations are widely distributed all over the world. In the tropics, they are regularly launched weekly or every other week (once to four times per month) from various stations associated with NASA’s Southern Hemisphere ADditional OZonesondes (SHADOZ) programme (Witte et al., 2017; Thompson et al., 2017, 2019; Sterling et al., 2018) (https://doi.org/10.57721/SHADOZ-V06). The screened ozonesonde volume mixing ratio profiles over each station are integrated from the first measurement level up to 270 hPa (≃ 10.5 km in the tropics) to obtain the tropospheric-ozone column to be compared with TROPOMI measurements. The conversion from ozone volume mixing ratio profiles to subcolumns (DU) is done using the formulae from the TEMIS (Tropospheric Emission Monitoring Internet Service) project (https://www.temis.nl/data/, last access: 24 May 2024) using pressure (hPa) and volume mixing ratio (ppmv) as input parameters. The sonde column is not processed when data gaps in the profiles are wider than 1.2 km or when the burst height of the sonde is below 15 km. The percentage of missing volume mixing ratio values in a profile is generally 0 % but can occasionally reach up to 2 %. Only for Ascension Island are up to 30 % missing data found for just a few days.
Ozonesonde profiles are considered to be spatially collocated in relation to the satellite data when the station is inside the grid box of the TROPOMI tropospheric-ozone data, here 0.5° × 0.5°.
In total, subcolumns from 13 ozonesonde stations (9 in the tropics and 4 in the subtropics) were compared with spatially collocated TROPOMI tropospheric-ozone columns for the time period from June 2018 to December 2022. The station locations are displayed in Fig. 1.
The convective cloud differential (CCD) method is a standard retrieval approach to derive tropospheric-column ozone (TCO) from satellite data using total columns of ozone and cloud information (Ziemke et al., 1998) (Fig. 2). The CCD technique only focuses on the tropics (20° S–20° N) where stratospheric ozone is assumed to be zonally invariant.
In the first step of the TCO calculation, ozone columns above deep convective clouds over the tropical eastern Indian and western Pacific oceans (20° S–20° N, 70° E–170°W) are retrieved since the occurrence rate of such clouds is lower outside this area. The clouds are defined by a high cloud fraction (≥ 0.8) and cloud height (≥ 7 km). When retrieving total ozone columns over clouds, a ghost column is added for the tropospheric part of ozone shielded by clouds. This ghost column is subtracted from the total ozone under partially cloudy scenes (CF ≥ 0.8) to obtain the above-cloud-column ozone (ACCO). Since cloud top height varies, the ACCO has to be referenced to 270 hPa by adding or subtracting ozone subcolumns between 270 hPa and the cloud top height. This means that, by using an ozone climatology, the missing (or additional) ozone column between the fixed reference level of 270 hPa and the measured cloud top pressure is added (or subtracted). The ACCO normalised to 270 hPa is then averaged in each corresponding latitude band of the Pacific sector. In the next step, total ozone columns under nearly clear-sky conditions (TOZ, CF ≤ 0.2) are averaged for all tropical grid boxes. In the final step, the tropospheric-ozone column in each grid box is derived by subtracting the Pacific ACCO from the clear-sky total ozone column in the same zonal band (Ziemke et al., 1998; Valks et al., 2003, 2014; Heue et al., 2016; Leventidou et al., 2016, 2018; Hubert et al., 2021).
4.1 CHORA Pacific Cloud (CPC) algorithm
The Cloud Height Ozone Reference Algorithm (CHORA) is an advanced version of the CCD method developed at the University of Bremen (Leventidou et al., 2016, 2018), in which the Pacific sector is also used as the cloud reference sector (CHORA Pacific Cloud, CPC), as described above for retrieving the above-cloud (“stratospheric”) ozone column.
Total ozone columns extracted from the operational offline/reprocessed total column product (Van Roozendael et al., 2012; Lerot et al., 2014; Garane et al., 2019) are used. The cloud parameters (cloud top height and cloud fraction) are retrieved with the OCRA/ROCINN-CRB algorithm (Loyola et al., 2018; Compernolle et al., 2021). The monthly mean data from the Cloud Height induced Ozone Variation Algorithm (CHOVA) (ESA, 2022) are used for the climatological correction of ACCO. The CHOVA climatology developed at our institute also uses TROPOMI data and is stored as monthly averages of volume mixing ratios from the Pacific sector (70° E to 170° W) for the latitude band from 20° S to 20° N. The standardised ACCO (normalised to 270 hPa) in each 0.5° latitude-wide Pacific band and the total ozone under clear-sky scenes (on a 0.5° × 0.5° latitude–longitude grid) are daily averaged to provide tropospheric-ozone columns on a daily basis. In a rare case scenario, if the final tropospheric-ozone value is negative, it is replaced by a fill value.
In general, the CPC algorithm shares the same methodology as that of the operational TROPOMI level-2 product (see Sect. 4.4), differing mainly in terms of the threshold for cloud fraction for the selection of non-cloudy scenes (CF ≤ 0.2), number of days of averaging, climatology, and grid resolution.
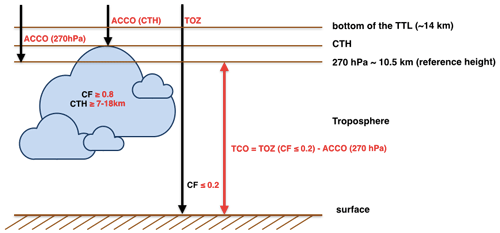
Figure 2Illustration of the CCD technique. TCO is the tropical tropospheric-column ozone, TOZ is the total ozone under clear-sky conditions, ACCO is the above-cloud-column ozone, CF is the cloud fraction, and CTH is the effective cloud top height. The partial ozone column between CTH and 270 hPa is estimated from the CHOVA climatology. TTL is the tropical tropospheric layer.
4.2 CHORA Local Cloud (CLC) algorithm
The assumption of stratospheric-ozone zonal invariance used in the CPC algorithm is only justified within the tropics (Ziemke et al., 1998, 2010; Valks et al., 2014; Thompson et al., 2017), which limits this approach to the latitude range between 20° S and 20° N. Since stratospheric-ozone variations are much larger at mid-latitudes (Weber et al., 2011; Godin-Beekmann et al., 2022), the cloud reference sector has to be locally defined. Hence, an advanced version of the CHORA algorithm (local-cloud reference sector, CLC) was developed to potentially extend this CCD method to higher latitudes by taking advantage of the high spatio-temporal resolution of TROPOMI (Fig. 4).
The CLC algorithm differs only in terms of the ACCO selection criteria compared to the CPC algorithm and climatology. CLC uses another version of the CHOVA climatology (CHOVA V6.7), which uses monthly averages of volume mixing ratios from the global sector (180° E to 180° W) for the latitude band from 20° S to 20° N (see Fig. 3).
An iterative approach has been used for automatically selecting the optimal local-cloud reference area around each retrieval grid box (0.5° × 0.5°), varying longitudinally from ± 5° to a maximum of ± 50°. The latitudinal extent is fixed at ± 1° for reasonable TCO retrievals and to restrict the stratospheric-ozone streamers by selecting a limited area around the retrieval grid box. The selection increases from the smallest cloud reference area to the largest and stops when the number of cloudy scenes exceeds 50.
4.3 CHORA Local Cloud Theil–Sen (CLCT) algorithm
4.3.1 Homogeneity criteria for total ozone column
The CLC algorithm is further adapted and optimised by introducing a homogeneity criterion for total ozone to overcome inhomogeneities in stratospheric ozone. Thus, TCO is only computed when the standard deviation (1σ) of the total ozone column under the cloudy conditions (CF ≥ 0.8) from the local-cloud reference sector is less than 10 DU; otherwise, it is overwritten by a fill value.
4.3.2 Theil–Sen regression for ACCO retrieval
An alternative method to directly estimate ACCO in relation to a reference altitude of 270 hPa is also introduced in the CLC algorithm based on the Theil–Sen regression (CLCT) (Fig. 5). The Theil–Sen estimator is a widely recognised non-parametric technique for performing a linear fit to empirical data. It is favoured for its computational simplicity and resistance to outliers (Sen, 1968). Consequently, this method has found frequent application in various remote sensing contexts (Fernandes and Leblanc, 2005).
The slopes between all possible pairs of ACCO and cloud top pressures (CTPs) retrieved over the auto-selected local-cloud reference sector are computed, and their median is selected as the Theil–Sen estimate of the slope (Fig. 6). The Theil–Sen estimate of the intercept can be obtained by applying the values of this estimated slope and the medians of ACCO and cloud top pressure in the linear regression equation (Wilcox, 2011). Applying these estimates of the Theil–Sen slope and intercept, the reference ACCO above the reference cloud top pressure of 270 hPa (and any other reference altitude) can be calculated directly.
This approach is very suitable in cases of strongly varying cloud top heights. From the slope of the linear regression between ACCO and cloud top height, the mean ozone volume mixing ratio within the range of cloud top heights can be determined, as is done in the cloud-slicing retrieval (ESA, 2021c). The Theil–Sen regression combines the CCD with the cloud-slicing approach. This approach is flexible as the ACCO can be calculated for arbitrary reference altitudes (in our case, 270 hPa), and it requires no ozone climatologies for converting ACCO to any reference height.
4.4 ESA TROPOMI level-2 tropospheric ozone (L2__O3_TCL)
The operational ESA algorithm (CCD-ESA) is quite similar to our CPC algorithm described above. While the CPC tropospheric ozone is calculated daily, CCD-ESA provides 3 d averages in a 0.5° × 1° latitude–longitude grid (ESA, 2021b; Hubert et al., 2021). In the following, we only describe the differences in relation to the CPC algorithm.
The ozone-sonde based-vertical ozone profile climatology by McPeters et al. (2007) is used to convert ACCO to the 270 hPa reference height. The mean ACCO for a given day is calculated over a 6 d period around the given date. Clear-sky total ozone (CF < 0.1) in a grid box is averaged over 3 d around the date of interest, and, thus, the tropospheric-ozone column is then provided as a 3 d average after subtracting ACCO. The homogeneity criteria for the CCD-ESA data are outlined in the metadata as follows: a minimum of 50 ACCO data points per latitude band is required, the maximum allowable standard deviation within the ACCO per latitude band is 15 DU, and the maximum difference between two neighbouring bands is limited to 5 DU. Additionally, the minimum ACCO value must be 200 DU. If these conditions are not met, the data will still be processed, but a stratospheric flag will be set, and the quality assurance (QA) value will be reduced accordingly. Here, we compare the ESA tropospheric-ozone data (CCD-ESA) with a quality value greater than 70 with ozonesondes and our algorithms from June 2018 to December 2022.
5.1 Uncertainty budget
In this subsequent section, we present a rough estimation of the total uncertainty in daily TCO retrievals using both the CLCT and CPC algorithms. The overall uncertainty of monthly averaged TCO (uTCO) for December 2022 is roughly calculated for both the CPC and CLCT methods (Fig. 7) using the following equation:
where represents the estimated uncertainty for a single total column ozone retrieval (≈3 DU), and signifies the same but for ACCO (≈ 2.5 DU) (Leventidou et al., 2016). The uncertainty of the measured cloud fraction uCF is about ±0.1 and contributes to the ACCO uncertainty of less than 1 DU (). The uncertainty in the cloud top height, uCTH, is about ± 500 m and adds an uncertainty of less than 0.5 DU () to TCO. NTOZ is the number of cloud-free total ozone measurements in the 0.5° × 0.5° grid box for both CPC and CLCT. NACCO is the number of above-cloud column ozone measurements in the cloud reference sector. and represent the standard deviation (1σ) of the average total ozone under clear-sky conditions in each 0.5° × 0.5° grid box and the above-cloud-column ozone from the local-cloud reference sector, respectively. Substituting all these values in Eq. (1), we can roughly estimate the total uncertainty of TCO. The average uncertainty is approximately 4.4 DU for CPC (Fig. 7a) and is around 3.1 DU for CLCT since the minimum number of ACCO values needed for averaging in the local-cloud reference sector is set at 50, with a similar requirement of one TOZ value (ground pixel) in the grid box under clear-sky conditions (Fig. 7b). Regions with the highest uncertainty exhibit fewer cloudy and clear-sky scenes. The data gaps in the figure result from the homogeneity criteria, as explained in the preceding sections, and from the absence of clear-sky and cloudy scenes. The primary source of uncertainty in the tropospheric-ozone column is considered to be ACCO (retrieval and cloud parameters) (Leventidou et al., 2016).
5.2 Comparison to ozonesondes
As a first step, CLC, CLCT, and CPC results are validated with SHADOZ ozonesondes (Thompson et al., 2017). Monthly means of tropospheric-ozone columns and corresponding standard deviations (1σ) from all three CHORA algorithms were calculated for the time period from June 2018 to December 2022. The ozonesonde stations available for validation are shown in Fig. 1. The frequency of the ozonesonde measurements varies between one and four per month, depending on the station.
We compare monthly averages with those from sonde stations located in the same satellite grid box. Upon reviewing our data, particularly for subtropical stations, we found that there were not enough temporally collocated data for reliable validation (the seasonal variations in daily collocated TROPOMI and ozonesondes are provided in Fig. S1 in the Supplement). Therefore, we decided to rely on temporally non-collocated data to ensure a more robust analysis.
Table 1 lists the mean difference and the standard deviation (1σ) between the various CHORA algorithms and ozonesondes. In the tropics, CLCT–TCO retrievals show the smallest bias at −2.4 to 1.6 DU and the smallest dispersion at +1.4 to +2.5 DU in relation to ozonesondes. On the other hand, CPC–TCO retrievals have both the largest bias at −0.3 to 7.5 DU and the largest dispersion at 1.3 to 5.0 DU. At seven of the nine stations, CPC–TCO shows a persistent positive bias (1.3 to 7.5 DU). The maximum positive bias was observed at Hilo at 7.5 DU, which is reduced to −1.1 DU using CLCT.
Figure 10 represents the seasonal variation of tropospheric ozone (2018–2022) from operational CCD-ESA and different CHORA algorithms in the grid box of the station and associated ozonesonde measurements. Figure 11 is the same as Fig. 10 but shows the ozone column differences between TROPOMI and ozonesondes.
In the following, we discuss the ozonesonde comparisons for different sectors.
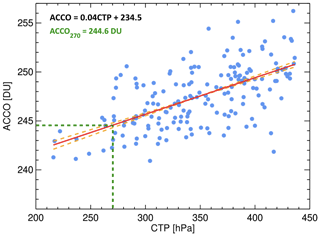
Figure 6A scatterplot of ACCO (DU) and CTPs (hPa) with a regression line (red). Individual data points are single measurements within the auto-selected reference sector (latitude band 2.25–0.25° S and longitude band 26.75–46.75° E) around Nairobi, Kenya (1.3° S, 36.8° E), on 1 January 2019. The orange lines show the upper and lower bounds of the 95 % confidence interval. The ACCO above the reference altitude 270 hPa is 244.6 DU (dashed green line). The regression line was determined using the Theil–Sen estimator.
5.2.1 Seasonal variability in TCO
Pacific sector (20° S–20° N, 70° E–170° W)
Analysing the seasonal variation of TCO over the Pacific sector reveals that locally available clouds during boreal winter and spring benefit the local-cloud algorithms more than CPC (Fig. 8a). The overall average mean bias and scatter across the four Pacific Ocean sites (Hilo, Kuala Lumpur, Samoa, and Fiji) shows that the local-cloud algorithms reduce the bias and scatter by more than half compared to CPC despite the fact that they all use the Pacific as the cloud reference sector (Fig. 9a). The CCD–TCO ESA data have a similar pattern to that of CPC, as expected. However, they overestimate ozonesonde measurements by 4 DU, which is the highest bias of all CCD algorithms shown here.
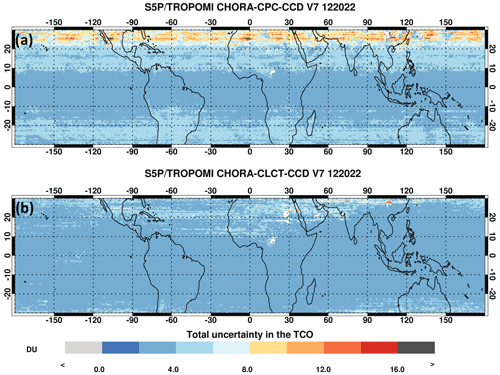
Figure 7The uncertainty in the TCO retrieval for December 2022 using (a) the CPC algorithm and (b) the CLCT algorithm.
A detailed examination of the biases indicates that the CPC–TCO bias is less than or equal to 1 DU (4 %–6 %) at three Pacific sites: Fiji, Kuala Lumpur, and Samoa. However, this does not hold true for Hilo (Hubert et al., 2021). Due to the close location of Hilo (19.4° N, 155.4° W) to the subtropics (Fig. 10c), the station experiences stratospheric-ozone intrusions from middle latitudes (Hübler et al., 1992; Cooper et al., 2005; Li et al., 2023). The tropospheric abundances are, therefore, generally higher in boreal spring (Cooper et al., 2014). Both the model and observations suggest that the spring maximum (April–May) in tropospheric ozone receives contributions from both biomass burning in Southeast Asia and the downward transport of stratospheric air (Oltmans et al., 1996; Wang and Jacob, 1998; Logan, 1999; Kentarchos et al., 2001; Liu et al., 2002). The pronounced late-boreal-summer minimum in ozone (July to October) is attributed to convective activity in the wet season, which mixes ozone-poor air from the marine boundary layer where photochemistry provides a net sink for ozone (Oltmans et al., 1996; Liu et al., 2002).
During boreal winter and spring, the CPC algorithm significantly overestimates ozone compared to ozonesondes (Fig. 11c). The seasonal southward movement of the intertropical convergence zone (ITCZ) during this period results in fewer high-level clouds over the Pacific sector and complicates reliable ACCO retrievals (Leventidou et al., 2016; Hubert et al., 2021).
The notably high standard deviation in the monthly mean tropospheric-ozone column is not exclusive to the CPC but is also noticeable in the local-cloud algorithms, mainly due to the influence of remote clouds, particularly during the boreal winter and spring seasons (Figs. 12a and b, 13a and b).
The TROPOMI local-cloud algorithms (CLC and CLCT) exhibit a significantly improved agreement with ozonesondes compared to CPC. The implementation of the CLCT–TCO retrieval effectively reduced the scatter in the differences in ozonesondes from 5 to 2.2 DU. The CPC algorithm has a higher average bias (7.5 DU) and larger dispersion (5.0 DU) compared to CLCT (−1.1 and 2.2 DU, respectively) (Table 1).
Tropospheric-ozone levels over the Western Pacific Ocean station of Fiji (18.1° S, 178.4° E) indicate a significant decrease in February according to ozonesonde data (Fig. 10k). Although the CLC and CLCT TROPOMI data also display a similar trend, the decrease is not as prominent. The large scatter of ozonesonde data suggests that stratospheric intrusions are important because of the station's proximity to the mid-latitudes (Thompson et al., 2000).
Table 1Mean difference (md, TROPOMI−sonde) and standard deviation (1σ) (SD) in Dobson units between spatially collocated TROPOMI TCO retrievals (CLCT, CLC, CPC, and CCD-ESA) and ozonesondes during the time period from 2018 to 2022.
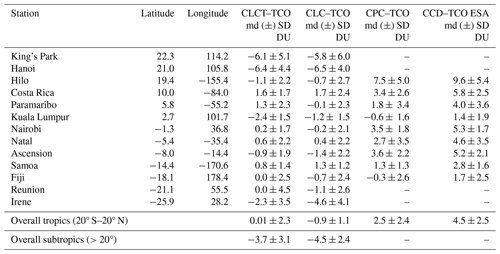
Like Samoa, Fiji is strongly influenced by strong marine convection. It is also impacted by the South Pacific convergence zone (SPCZ), transporting clean marine air to the upper troposphere (Pickering et al., 2001; Chandra et al., 2014). Advection from the southern mid-latitudes introduces upper-tropospheric and stratospheric ozone, along with emissions resulting from biomass burning. Being south of the SPCZ, Fiji is more exposed to the latter (Chandra et al., 2014). According to Oltmans et al. (2001), the impact of African wildfires is also prominent after August (Fig. 10k).
The tropospheric-ozone column retrievals using the CPC algorithm exhibit an overall bias of −0.3 DU and a scatter of 2.6 DU (Table 1). The CLCT algorithm slightly outperforms the CPC algorithm by showing a reduced bias of 0 DU with respect to the ozonesondes, even though the dispersion remains almost the same (2.5 DU).
In Kuala Lumpur (2.7° N, 101.7° E), the eastern Indian Ocean site, tropospheric ozone reaches an average of around 20 DU (Fig. 10f). During boreal summer, influenced by the Indian summer monsoon and the ITCZ, ozone decreases further. The summer monsoon's southwesterly winds bring moist, ozone-poor air over the region, resulting in reduced tropospheric-ozone levels (Slingo et al., 2005; Lu et al., 2018). During boreal summer (June–August), both local-cloud reference algorithms agree well with ozonesondes due to sufficient cloudy scenes and effective TCO retrievals.
During boreal spring, ozone levels rise due to influences like the Tibetan monsoon and biomass burning in the northern subtropics (Yonemura et al., 2002). The Tibetan monsoon transports polluted air from Southeast Asia, where biomass burning occurs from February to May. Stratospheric subsidence through Kelvin waves may also contribute to ozone enhancement. Additionally, ozone transport from the subtropics to the upper troposphere is possible after stratospheric subsidence (Poulida et al., 1996; Baray et al., 1998). Studies in urban areas, including Kuala Lumpur, have noted frequent high tropospheric-ozone levels (Mohtar et al., 2018; Ahamad et al., 2020).
The presence of tropospheric ozone from pollution and stratospheric-ozone transport possibly contributes to higher ACCO values, irrespective of the season (Fig. 12). This leads to TCO values by all three retrieval algorithms being too low, particularly in the middle of spring (April) (Fig. 11f).
Even though the local-cloud reference sector used in both the CLC and CLCT algorithms belongs to the Pacific sector, the fixed large Pacific cloud reference sector ensures the inclusion of more highly reflective clouds and comparatively less polluted backgrounds, which benefit the CPC retrievals over Kuala Lumpur. This explains the improved bias of CPC (−0.6 DU) compared to that of CLCT (−2.4 DU) even though the dispersion remains almost the same (∼ 2 DU). The CLC approach exhibits a reduced bias (−1.2 DU) compared to CLCT, possibly due to the presence of homogeneous clouds in the vicinity of the station throughout all seasons (Fig. 13).
In the South Pacific ocean site of Samoa (14.4° S, 170.6°W), there is an increase in tropospheric-column ozone during boreal summer and early autumn (June–September) (Fig. 10j). This ozone enhancement is associated with biomass burning, which follows a regular seasonal pattern and has a significant impact during the months of boreal summer and autumn (Hudson and Thompson, 1998; Jensen et al., 2012; Thompson et al., 2012). However, this pattern is somewhat subdued by the enhanced convection and the pristine marine environment characteristic of the Pacific sector, rendering the tropospheric-ozone seasonal cycle comparatively milder (∼ 19 DU) (Folkins et al., 2002; Thompson et al., 2003) (Fig. 10i). Moreover, the South Pacific convergence zone (SPCZ) nearby brings clean marine air to the upper troposphere (Chandra et al., 2014).
Trade winds also play a role in transporting ozone-poor air from the east, while mid-latitude air masses bring ozone-rich air, creating distinctive seasonal surface ozone patterns (Harris and Oltmans, 1997). Additionally, Samoa's location in the South Pacific Ocean exposes it to ozone enrichment from stratosphere–troposphere exchange (Daskalakis et al., 2022).
Despite the Pacific sector affiliation of the local-cloud reference sectors, the CLCT algorithm (0.8 ± 1.4 DU) performs better than the CPC algorithm (1.3 ± 1.3 DU), exhibiting a lower bias. This improvement can be attributed to the CLCT algorithm using more local ACCO measurements, particularly during the months of boreal winter when high cloud cover is more prevalent. This is highlighted in Fig. 13a.
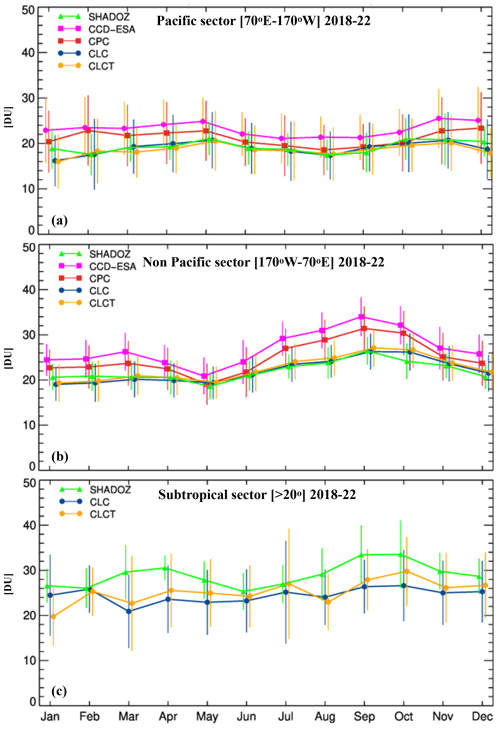
Figure 8Seasonal variation of TROPOMI and ozonesonde tropospheric-ozone columns up to 270 hPa for different regions in the tropics and subtropics from 2018 to 2022. The green line are ozonesondes. The red, blue, yellow, and magenta lines are CPC, CLC, CLCT, and CCD-ESA, respectively. The error bar is the corresponding standard deviation (1σ) of the monthly means.
Non-Pacific sector (20° S–20° N, 70° E–170° W)
The advantages of using local-cloud algorithms over CPC are more pronounced in the non-Pacific sector (Fig. 8b). Estimating ACCO over local clouds results in more accurate TCO retrievals than CPC, which consistently overestimates values in the non-Pacific sector in all seasons. The bias for the five non-Pacific sites (Costa Rica, Paramaribo, Nairobi, Natal, and Ascension Island) is reduced from 3 to 0.1 DU with CLCT and CLC. Additionally, the dispersion of 2 DU from CPC is halved by both local-cloud algorithms (Fig. 9b). At all sites around the Atlantic basin (Costa Rica, Paramaribo, Natal, and Ascension Island), the CPC–TCO bias is reduced by less than half using the local-cloud algorithms (Table 1). Even though CCD–TCO ESA retrievals from the non-Pacific sector show the same bias pattern as CPC, they show a higher bias and scatter (+5.0 ± 1.9 DU).
Costa Rica (10° N, 84° W) is a tropical site located in Central America. The tropospheric-ozone retrievals over this location set an example where the local-cloud reference sector (CLC, CLCT) works better than the Pacific sector (CPC), especially during boreal summer when the convective cloud activity is highest (see Fig. 10d, Pfister et al., 2010; Schoeberl et al., 2015). The ozone spread for CLCT is more pronounced during boreal winter, particularly in February, with an approximate spread of 6 DU. This seasonal variation is attributed to the winter-time migration of the ITCZ to the south, resulting in rare cloudy scenes in the proximity of the station (Fig. 12a). The inhomogeneity of cloud top heights introduced by distant clouds adds more dispersion to the ACCO and TCO values, as observed in previous studies (Leventidou et al., 2016) (Fig. 13a).
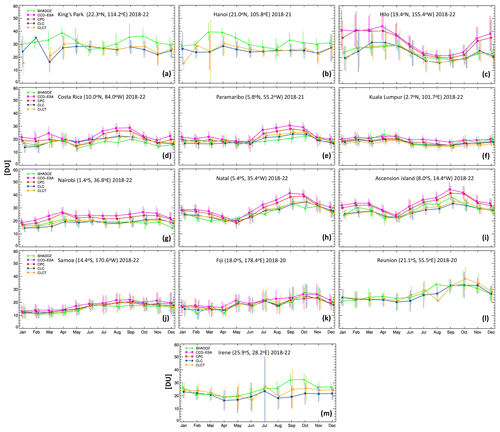
Figure 10Seasonal variation of TROPOMI and ozonesonde tropospheric-ozone columns up to 270 hPa at 13 sites from 2018 to 2022. The green line indicates ozonesondes. The magenta line indicates CCD–TCO ESA. The red, blue, and yellow lines are CPC, CLC, and CLCT results, respectively. The error bar is the corresponding standard deviation (1σ) of the monthly means. No error bars are shown for stations with only one launch per month. The time period varies with stations according to the data availability.
Overall, it is clear that the CLCT algorithm offers more accurate tropospheric-ozone columns. Compared to CPC (+3.4 ± 2.6 DU), CLCT exhibits a significantly reduced bias and scatter (+1.6 ± 1.7 DU) (Table 1).
Tropospheric ozone over Paramaribo (5.8° N, 55.2° W) in the equatorial Atlantic Ocean region is greatly influenced by meteorological variability (Fig. 10e). The ITCZ passes over Paramaribo twice per year (May and December), causing a semi-annual seasonality in tropospheric ozone (Peters et al., 2004; Thompson et al., 2000). The transport of polluted air from the Northern Hemisphere Atlantic region and from mid-latitudes to the lower troposphere and the descending ozone-rich air on the Equator-ward side of the North Atlantic provide high ozone enhancement in the mid-troposphere, which explains the slight increase in February. During the latter half of the year, the transport of biomass burning products from Africa across the Atlantic Ocean, lightning activity, and the subsidence of ozone-abundant air are possible reasons for the tropospheric-ozone rise (June–October), with a maximum in October (Peters et al., 2004; Thompson et al., 2003; Leventidou et al., 2016).
At the Northern Hemisphere station, Paramaribo, particularly during the late boreal-winter months of January and February, the ACCO values are possibly too high due to the limited presence of highly reflective clouds close to the station (Figs. 12a and 13a). This effect impacts both local-cloud algorithms, leading to a subsequent underestimation of TCO (Fig. 10e). However, comparisons with the sonde data unveil that the CLCT algorithm delivers more accurate tropospheric-ozone columns, with a smaller bias of 1.3 DU and a scatter of 2.3 DU in contrast to CPC (1.8 and 3.4 DU, respectively; Table 1).
The Nairobi site (1.3° S, 36.8° E), located in the capital of Kenya in eastern Africa (Fig. 10g), also shows low tropospheric-ozone abundance originating from oceanic south easterlies. Thompson et al. (2003) suggest that the high terrain and the frequent isolation of the station from the African pyrogenic ozone reduce the overall TCO over Nairobi.
Ozonesonde measurements over Nairobi from 2000 to 2014 suggest that the summer season (June–August) experiences the highest ozone levels (Shilenje et al., 2015). Kimayu et al. (2017) attribute this tropospheric enhancement to ozone-rich incursion from the south due to high biomass burning during this period. The current seasonal variability of Nairobi also exhibits a slight increase in ozone during boreal summer, as well as during late spring (April) (Fig. 11g). Notably, the CLCT algorithm effectively captures these seasonal fluctuations in ozone concentration.
Both the CCD-ESA and CPC algorithms consistently overestimate tropospheric ozone irrespective of the season. The TROPOMI TCO retrievals using the CPC algorithm display a substantial positive bias (3.5 ± 1.8 DU), which can be attributed to lower ACCO values from the Pacific reference sector. Among all the stations, the CLCT algorithm emerges as the most suitable algorithm, showing excellent agreement with ozonesondes (+0.2 ± 1.7 DU) (Table 1).
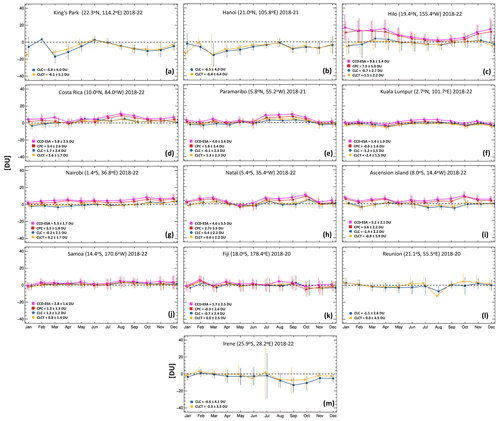
Figure 11Same as Fig. 10, but, here, ozone column differences between TROPOMI and ozonesondes are shown.
Ozone is greater at locations associated with continental convection, like in Natal (5.4° S, 35.4° W) (Folkins et al., 2002) (Fig. 10h). Here, TCO exhibits a significant seasonal cycle, with a maximum during late boreal summer and autumn and a minimum during spring. High tropospheric ozone observed in September is attributed to extensive biomass burning during the dry season, which lasts from May to September to the south and west of Natal (Ratisbona, 1976; Olson et al., 1996; Thompson et al., 1996). Lightning also contributes to ozone enhancement (Pickering et al., 1996). According to Thompson et al. (2000), this mid-tropospheric-ozone enhancement could also be due to the subsidence of aged upper-tropospheric air.
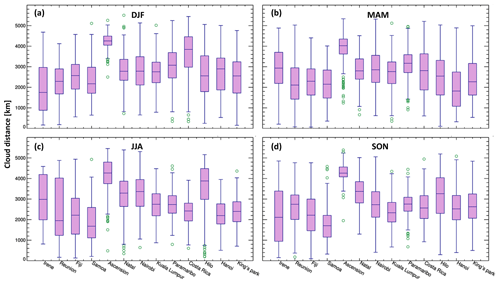
Figure 12Seasonal mean distance (km) of deep convective clouds observed by TROPOMI accounted for in the above-cloud-column estimation by CLC and CLCT from each ozonesonde station for the period of 2018 to 2022. Seasons are defined as DJF (December, January, February), MAM (March, April, May), JJA (June, July, August), and SON (September, October, November). The lines (whiskers) extending from the box indicate the variability outside the upper (75th) and lower (25th) quartiles. The horizontal line on the box represents the median or 50th percentile. The green circles represent outliers.
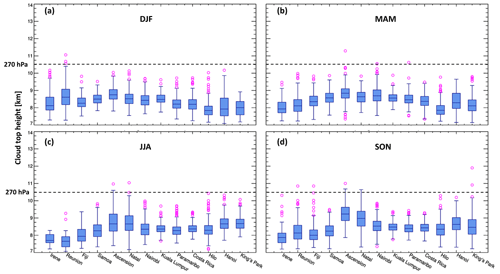
Figure 13Seasonal mean cloud top height (km) of deep convective clouds from TROPOMI accounted for in the above-cloud-column estimation by CLC and CLCT for the grid box around the ozonesonde station from 2018 to 2022. Seasons are defined as DJF (December, January, February), MAM (March, April, May), JJA (June, July, August), and SON (September, October, November). The dashed line represents the 270 hPa level (⋍ 10.5 km in the tropics). The lines (whiskers) extending from the box indicate the variability outside the upper (75th) and lower (25th) quartiles. The horizontal line on the box represents the median or 50th percentile. The green circles represent outliers.
The CPC algorithm consistently overestimates TCO values throughout most months, possibly attributable to ACCO retrieved from the Pacific sector being too low (Fig. 11h). Again, the CLCT algorithm effectively reduces the overall bias and scatter in tropospheric ozone compared to the CPC algorithm (from +2.7 ± 3.5 to 0.6 ± 2.2 DU) (Table 1). Results from both local-cloud algorithms clearly agree better with sondes than the CPC algorithm.
Ascension Island (8° S, 14.4° W) in the Atlantic Ocean (Fig. 10i) is located downwind of the primary source region, central and southern Africa, with emissions from the widespread burning of savannas and other biomasses. Tropospheric ozone over Ascension Island is higher than in Natal (∼ 30 DU) because of its proximity to Africa and due to the influence of the descent in the mean Walker circulation (Jensen et al., 2012). The ground-based measurements and TROPOMI show maximum ozone from July to October. The high ozone level observed in February is more likely to be due to biomass burning in western and northern Africa (Fishman et al., 1992; Jensen et al., 2012). Notably, the lowest TCO levels are observed during mid-spring (April), coinciding with the northward migration of the ITCZ.
Both CLC and CLCT algorithms show weak agreement with ozonesondes during late boreal summer and early autumn. This difference can be linked to a notable bias in ACCO values derived from distant clouds, particularly during summer (Figs. 11i, 12c, and 13c). The study by Wai et al. (2014) reveals that biomass burning in South Africa and South America is most prevalent during late boreal winter and autumn. Cloud measurements from these regions can result in an overestimation of ACCO and an underestimation of CLC–TCO and CLCT–TCO.
However, the CLCT algorithm showcases a smaller bias (−0.9 DU) compared to the CPC algorithm (+3.6 DU), while both algorithms share a similar scatter in comparison to the sondes (∼ 2 DU, Table 1).
Subtropics (>20°)
The overall seasonal variation of four subtropical sites (King's Park, Hanoi, Reunion, and Irene) is shown in Fig. 8c. Since these sites are located further north and south, they experience more clouds in local summer and winter, enhancing the performance of local-cloud algorithms during these seasons. The large standard deviations for the CHORA algorithms were expected due to the complex ozone dynamics and the relative lack of highly reflective clouds in the subtropics compared to in the tropics. The mean difference and standard deviation for these subtropical stations are similar for both local-cloud algorithms, with CLCT performing slightly better (−3.7 ± 2.6 DU) than CLC (−4.5 ± 2.4 DU) (Table 1) and with both underestimating ozonesonde results.
King's Park (22.3° N, 114.2° E) is an urban site roughly 66 m above mean sea level in China. Liao et al. (2021) analysed 20 years of ozonesonde data (2000–2019) from the station, revealing a seasonal ozone cycle with a notable boreal-spring peak (Fig. 10a). This pattern suggests rich ozone transport from mid-latitude regions and poor ozone from the tropics. Elevated tropospheric-ozone levels, particularly in early spring, are attributed to stratospheric intrusion and biomass burning.
TROPOMI consistently shows lower ozone levels than ozonesondes throughout the years, with February and June being an exception (Fig. 11a). This discrepancy is likely due to the wider cloud reference sector, which is particularly notable during boreal spring, autumn, and winter, resulting in an overestimation of ACCO (Fig. 12a, b, and d). During boreal summer, TCO retrievals from all CHORA versions exhibit comparatively better agreement with sondes, likely because of the abundance of highly reflective cloud data near the station and reduced stratospheric-ozone dynamics (Figs. 12c and 13c).
In general, TCO retrievals from the CLCT or CLC methods demonstrate more reasonable agreement with sonde measurements compared to CPC (Fig. 11a). Both local-cloud algorithms introduce a bias of approximately −6 DU, with CLCT showing slightly less scatter (5 DU) compared to CLC (6 DU).
Figure 10b shows the TROPOMI differences in Hanoi (21° N, 105.8° E), which is located in Southeast Asia and is part of the Asian summer monsoon circulation region, where tropospheric ozone exhibits a long-term increasing trend (Gaudel et al., 2018; Zhang et al., 2016). The mean tropospheric-ozone columns over Hanoi and King's Park are the highest among all the stations (∼ 32 DU), which is expected for a subtropical location (Cooper et al., 2014). The accelerated urbanisation and industrialisation in this mega-city cause high emissions of pollutants, including ozone precursors, and contribute to high tropospheric ozone over the station (Dam et al., 2008). The exchange between the tropical troposphere and extratropical stratosphere occurs in this region, which also contributes to this ozone enhancement.
Enhanced tropospheric ozone is observed in ozonesonde data during boreal spring from enhanced biomass burning in Thailand (Liao et al., 2021; Sonkaew and Macatangay, 2015; Ogino et al., 2022) and possibly also due to the lower-stratosphere–upper-troposphere transport related to the subtropical jet passing over Hanoi (Yonemura et al., 2002). The latter process is also responsible for the ozone increase in early autumn (September).
Likewise, at King's Park, TROPOMI underestimates ozonesondes throughout the year by up to 15 DU in boreal spring and in autumn and early winter (Fig. 11b). This can be related to the broader cloud reference sector, particularly in spring, autumn, and winter, which causes ACCO to be too high (Fig. 12a, b, and d). However, it is important to note that a more extended time series is required for a detailed analysis to better understand this variation.
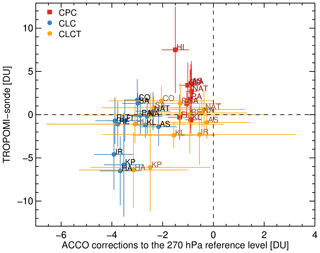
Figure 14A scatterplot of mean difference (md, TROPOMI−sonde) with standard deviation (1σ) in Dobson units between spatially collocated TROPOMI TCO retrievals (CLCT, CLC, CPC) and ozonesondes and the respective climatological corrections with standard deviations added or subtracted to the 270 hPa reference level (DU) during the time period from 2018 to 2022.
During boreal summer, TCO retrievals from local-cloud versions are closer to sondes due to sufficient cloud data available in the vicinity of the station (Fig. 12c). The increased dispersion observed in TCO retrievals by the local-cloud algorithms during late autumn, winter, and spring can be attributed to the lack of highly reflective cloud data and the presence of remote clouds exhibiting inhomogeneous cloud top heights (Figs. 12a, b, and d and 13a, b, and d). Despite the large dispersion, both CLC and CLCT exhibit good agreement with ozonesondes in February.
Overall, the TCO retrievals obtained through the CLCT or CLC methods yield good agreement with sonde measurements (Fig. 11b). The average difference between the local cloud algorithms and ozonesondes remains the same at DU, with a dispersion of ∼ 4 DU.
Reunion Island (21.1° S, 55.5° E), in the western Indian Ocean, is a subtropical station influenced by African biomass burning. Here, ozonesonde data (Fig. 10l) are more scattered (large standard deviations), mainly due to the subtropical location. The increased convective activities can explain the seasonal decrease in TCO during austral summer (December–February). During the Southern Hemisphere winter (June–August), TCO increases, likely linked to biomass burning activity south of the Equator (Thompson et al., 2000).
In August and October, two ozone peaks reach around 34 DU, with more variability in the latter, which CLC captures well. According to Thompson et al. (2000), the weak correlation between TCO variability and biomass burning signals during this period suggests the influence of additional dynamic factors. Air parcel trajectories indicate that the primary sources are over Africa, the eastern Indian Ocean, and Madagascar, which are the locations prone to stratospheric influences (Taupin et al., 1999; Randriambelo et al., 2000; Thompson et al., 2017). In August, both cloud reference algorithms exhibit a tendency to underestimate TCO, which may be attributed to the limited occurrence of highly reflective clouds, as shown in Fig. 13c.
Notably, TCO retrievals derived from CHORA's local-cloud algorithms demonstrate robust agreement with ozonesonde measurements during austral summer, primarily due to the elevated presence of local convective clouds, as showcased in Figs. 12a and 13a. In terms of overall performance, the CLCT algorithm exhibits an improvement in bias (0 DU) compared to the CLC algorithm (−1.1 ± 2.6 DU). However, there is an increase in dispersion by almost 2 DU (Table 1).
The southernmost ozonesonde station in this study is Irene (25.9° S, 28.2° E) in South Africa (Fig. 10m). The tropospheric-ozone measurements over Irene (on average ∼ 25 DU) indicate the presence of ozone from mid-latitude air via stratosphere–troposphere exchange events (Thompson et al., 2003; Mkololo et al., 2020). Irene exhibits Southern Hemisphere tropospheric-ozone seasonality, peaking during the austral spring (September–November) with maxima in September (33.6 DU) and October (31.3 DU) (Fig. 10m) (Diab et al., 2004). During these months, the station encounters air masses recirculated from southern Africa and the westerly flow from higher latitudes, both contributing to elevated ozone levels. Both local-cloud algorithms underestimate TCO during this period, but CLCT demonstrates a better agreement with this ozone enhancement than CLC, even with a larger dispersion.
The specific ozone increase in late austral autumn and winter (May–August) corresponds to a high concentration of ozone precursor originating from South America as well (Fishman et al., 1992; Thompson et al., 2003; Jensen et al., 2012). Both local-cloud algorithms show a significant deviation from ozonesonde measurements during the late austral-winter month of August. This difference primarily arises from the limited presence of highly convective clouds, as illustrated in Figs. 12c and 13c. The high scatter observed in July, introduced by both CLC and CLCT, is also a consequence of the same (Fig. 11m). The absence of these high-level clouds during this period presents a substantial validation challenge. In contrast, the late austral-summer season demonstrates improved agreement with ozonesondes, attributed to the increased availability of clouds, as illustrated in Figs. 12a and 13a.
Despite the complex ozone dynamics, CLCT provides a significantly improved bias and dispersion (−2.3 ± 3.5 DU) in comparison with CLC (−4.6 ± 4.1 DU) (Table 1).
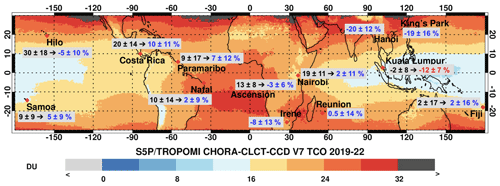
Figure 15Map of TROPOMI CLCT tropospheric-ozone column averaged from 2019 to 2022. Monthly mean bias and standard deviation (1σ) with respect to ozonesondes are indicated at the station's location. The numbers on the left are those from CPC, and the numbers on the right are from CLCT. Blue numbers indicate an improvement over CPC, and red numbers indicate larger differences for CLCT.
5.3 Possible sources of biases in TCO retrievals
5.3.1 CPC–TCO retrieval
A potential source of random bias in CPC–TCO is the assumption of the longitudinal invariance of stratospheric ozone in the tropics. Several studies (Ziemke et al., 2010; Valks et al., 2014; Thompson et al., 2017) conclude that it is approximately valid above 200 hPa in the tropics or at latitudes below 15°. In reality, the tropical stratospheric column can be affected by tropical waves (Kelvin and Rossby waves) in the stratosphere (Ziemke and Stanford, 1994). In addition to that, the occasional uplifting of highly polluted ozone-rich air to the upper-troposphere and lower-stratosphere regions can also influence stratospheric-ozone measurements (Avery et al., 2010). The ozone climatologies without longitudinal variations used for the satellite ozone retrievals can also cause uncertainties in the final results, even though this is considered to be a rather small effect (Thompson et al., 2003).
The sampling issue related to the presence or absence of convective clouds over the reference sector also introduces uncertainties in the TCO retrieval. The biases in retrieving cloud parameters, especially cloud top height, will lead to biases in the climatological correction of ACCO to the 270 hPa reference height, thereby generating a systematic error in ACCO and in the subsequent TCO computation over the corresponding latitude belt (Hubert et al., 2021). Sampling errors in TOZ retrievals under clear-sky conditions could also give rise to biases in TCO.
5.3.2 CLC(T)–TCO retrieval
The implementation of local-cloud reference regions leads to more accurate and realistic ACCO retrievals within and near the grid box compared to using a fixed large reference area in the Pacific sector.
Both CLC and CLCT algorithms may encounter potential biases when there are insufficient convective, high clouds within the selected local-cloud reference sector. This scarcity of highly reflective clouds can result in a lower sampling of ACCO data and a larger dispersion or scatter in the final TCO estimation. The variability of cloud characteristics, such as cloud cover and cloud top heights, is influenced by seasonal changes and the geophysical attributes of the retrieval area (Figs. 12 and 13), which poses a significant challenge for the local-cloud algorithms. Furthermore, the automatic selection of cloud reference sectors based solely on the characteristics and quantity of cloud data can sometimes lead to the selection of large reference sectors. Consequently, this could dilute or “wash out” the impacts of pollution or actual ozone dynamics over the retrieval area.
In the context of the CLC algorithm, the lack of highly reflective clouds and inhomogeneities in cloud top heights can introduce uncertainties during the 270 hPa standardisation step when applying climatological corrections. However, unlike the CPC algorithm, employing a longitudinally variant ozone climatology for climatological corrections helps to alleviate TCO biases to some extent.
The homogeneity criteria may lead to the exclusion of data points, with higher variations in total ozone columns under cloudy conditions. This exclusion can result in a reduced sample size for TCO calculations, which could affect the statistical significance of the results. In regions with naturally higher ozone variability, the method may excessively exclude data, leading to biased TCO estimates for those areas. Also, the choice of the homogeneity threshold (e.g. 10 DU) could influence the results. A different threshold may lead to different data inclusion and/or exclusion patterns and, consequently, could impact the final TCO estimates. However, after conducting sensitivity analyses, it has been confirmed that a threshold of 10 DU is a reasonable choice.
Even though the Theil–Sen regression method helps to directly estimate ACCO values, avoiding climatological corrections, it is essential to consider possible biases associated with this approach. The technique works better with variable cloud top heights. However, it may lead to less accurate slope estimates and reduced efficiency when the cloud top heights are more homogeneous. Additionally, the accuracy of the Theil–Sen method also depends on the sample size of the data. A very small sample size can lead to less accurate and less robust estimates. Our study accounts for this issue by setting the minimum number of ACCO values from the cloud reference sector to 50, thereby mitigating the bias to a greater extent. Despite its robustness in relation to outliers compared to the normal regression method, the Theil–Sen method can still be influenced by extreme outliers that significantly impact the estimated slope. However, in this particular case, the likelihood of such extreme outliers is minimal, particularly after implementing the filtering criteria.
Furthermore, biases in TCO can also stem from sampling errors in TOZ retrieval under clear-sky conditions. If satellite sensors have reduced sensitivity near the boundary layer, the accuracy of clear-sky ozone retrievals in this region may be compromised. This can introduce uncertainties into the estimation of TCO.
In conclusion, the biases and dispersion observed in CHORA–TCO retrievals may arise from a combination of systematic errors, including sampling errors in ozone and cloud retrievals. Nevertheless, confirming these potential sources of biases can be challenging (Hubert et al., 2021).
5.3.3 Impact of climatological corrections
Figure 14 displays the behaviour of the TROPOMI ozonesonde biases as a function of the climatological corrections needed for conversion to the reference height of 270 hPa.
The CPC algorithm uses the CHOVA climatology based on the Pacific sector. Since there are more highly reflective clouds over the Pacific region, the climatological corrections are the least for all the stations compared to the local-cloud algorithms. Hilo is the station where the climatological correction is the largest (−1.5 DU), which is still less than the climatological corrections by CLC at all stations. Nevertheless, CPC exhibits a high overestimation over Hilo. This overestimation is likely to be affected by the fewer boreal-wintertime clouds impacting the overall TCO retrieval.
Analysis of Fig. 14 reveals that Hanoi and King's Park are the two stations where both CLC and CLCT exhibit the largest bias and scatter, as well as the maximum climatological correction or difference added or subtracted. For CLC, the climatological corrections are based on global climatology (Fig. 3), specifically the zonal means of ozone for corresponding latitudes. In contrast, for CLCT, the correction is calculated from the Theil–Sen regression line and does not require a climatology.
The CLC approach introduces large corrections over Reunion and Irene (∼ 4 DU). This might be due to ACCO measurements from remote clouds over a wide range of distances from the station, with austral summer being a minor exception (Figs. 12 and 13). In contrast, the CLCT algorithm shows corrections around −1 DU but with large standard deviations of about 3–4 DU. The corresponding CLCT biases for these stations are also comparatively lower.
In general, CLCT exhibits a large scatter for these corrections across all stations, particularly at higher latitudes. The variability of ozone concentrations in the atmosphere can be naturally high in the subtropics at different pressure levels. These significant variations in ozone distribution with altitude can lead to large differences and, consequently, a high standard deviation. The lack of cloud data in the subtropics may also contribute to this increased standard deviation. Hilo, with its proximity to the subtropics, exemplifies this issue, showing a standard deviation of 3.5 DU. Such large scatters are not present in CLC climatological corrections.
The overall average climatological corrections are consistently negative across all algorithms and stations. This indicates that most clouds do not reach the 270 hPa level, even from the Pacific sector.
The overall analysis of Fig. 14 indicates no significant dependence between the corrections to the reference level and the mean bias and scatter for any of the three algorithms.
5.4 Meridional variation of statistical bias and dispersion
The operational TROPOMI tropospheric-ozone data (CCD-ESA) consistently exhibit a positive bias across all ozonesonde stations, which is at maximum among all satellite data sets (Fig. 16). This bias is similar to our CPC algorithm, except for the stations of Kuala Lumpur and Fiji, where the CPC algorithm shows a slight negative bias of −0.6 and −0.3 DU, respectively. Despite using the same Pacific sector as the cloud reference sector, differences between CCD–TCO ESA and CPC–TCO are due to differences in data processing, different averaging methods, and different ozone climatologies used to convert tropospheric-ozone columns to the reference height of 270 hPa. Both data sets exhibit a similar pattern with respect to the ozonesondes and have almost the same dispersion.
In this section, we focus more on comparing our local-cloud algorithms with the standard CPC method rather than the TROPOMI tropospheric-ozone data since the goal is to highlight improvements in tropospheric-ozone results stemming from differences in the cloud reference sectors.
The meridional variations of the biases and dispersions of TCO retrievals relative to ozonesondes from all three CHORA versions (Fig. 16) reveal that the CPC algorithm overestimates TCO across the entire tropical belt (20° S, 20° N), except over Kuala Lumpur and Fiji, where the bias is considerably smaller (−0.6 and −0.3 DU, respectively). In Kuala Lumpur, the CPC algorithm exhibits a marginal overestimation of ACCO, mainly during boreal winter and spring, which could be attributed to the reduced occurrence of deep convective clouds during this time frame. Consequently, this leads to a slight underestimation of TCO, irrespective of the expected ozone enhancement over the urban station (Fig. 10f).
In the region over Fiji, the most significant deviation from sonde measurements using the CPC algorithm is observed during late autumn (October–November). This discrepancy could be attributed to the possible overestimation of the influence of African wildfires when deriving ACCO values. Aerosols resulting from biomass burning have the capability to both scatter and absorb sunlight and can potentially affect the accuracy of ACCO and total column retrievals. The low number of ozonesonde measurements over Fiji poses a challenge in confirming this hypothesis.
In equatorial regions, the CPC retrievals display their highest positive bias over Nairobi (3.5 ± 1.8 DU). This bias is likely to be attributable to the underestimation of ACCO, possibly influenced by the clean and unpolluted environment characteristic of the Pacific sector, which is in contrast to Africa.
Out of nine stations, the maximum positive bias (7.5 ± 5.0 DU) introduced by CPC is observed over Hilo (19.4° N, 155.4° W) due to the overestimation of TCO, especially during boreal winter (Fig. 10c). The seasonal migration of the ITCZ to the south (Holton, 1973) results in the deficit of highly convective clouds over the corresponding latitudinal band of Hilo, which leads to inefficient ACCO and TCO estimations and a large scatter of the data (Leventidou et al., 2016). However, CPC's overall bias and dispersion over the tropical belt (+2.5 ± 2.4 DU) are significantly reduced by CLCT to 0.01 ± 2.3 DU and by CLC to −0.9 ± 1.1 DU.
In the subtropics (beyond 20° latitude), tropospheric ozone is more inhomogeneous in space and time. All subtropical stations are subject to the advection of highly polluted ozone-rich air from mid-latitudes and lower-stratosphere–upper-troposphere exchanges. Due to these subtropical characteristics and fewer highly reflective clouds, both CLC and CLCT algorithms overestimate ACCO and thereby underestimate TCO over all four subtropical stations: Reunion, Irene, Hanoi, and King's Park. The most notable improvements in retrieving TCO over the subtropics are seen with CLCT. The CLCT algorithm provides a reasonable bias and scatter of −3.7 ± 3.1 DU in the subtropics. In comparison, the CLC algorithm yields an overall bias of −4.5 ± 2.4 DU.
Over the southernmost subtropical station of Irene (25.9° S, 28.2° E), which is more diverse in terms of stratospheric and convective influences, CLCT retrievals yield better accuracy (−2.3 ± 3.5) than CLC (−4.6 ± 4.1), highlighting the effectiveness of the CLCT algorithm.
The homogeneity requirement for the standard deviation of the total ozone column under cloudy conditions in the local reference sector (CLCT) being less than 10 DU has proven to be effective in mitigating the influence of stratospheric-ozone streamers across subtropical stations. This approach achieves a more constrained variability of ACCO values at these locations. Ultimately, the local-cloud approach significantly reduces bias and scatter, resulting in better agreement of TCO with ozonesondes.
The CPC retrievals have a comparatively larger dispersion in the Northern Hemisphere (NH) (3.4 DU) than in the Southern Hemisphere (SH) (1.7 DU). The larger spread in TCO in the NH can be attributed to its stronger variability in terms of stratospheric ozone (Cooper et al., 2014; Williams et al., 2019). In the Northern (Southern) Hemisphere, CLCT yields a much-reduced dispersion of 1.9 DU (0.7 DU) compared to CPC, indicating the algorithm's efficiency in the tropics.
Moving from the Equator towards the higher subtropical latitudes, the bias and variability of TCO retrievals generated by both CHORA local-cloud algorithms show an increasing trend. This pattern aligns with the increased natural variability in TCO and stratospheric-ozone at higher latitudes (Hubert et al., 2021). For both the CLC and CLCT algorithms, the overall dispersion increases by ∼ 1 DU when moving from the tropics to the subtropics.
These results substantiate that the challenges in achieving accurate TCO retrievals arising from the complexities of stratospheric-ozone variability at higher latitudes can be effectively mitigated by applying the CLCT algorithm using a local-cloud reference sector. This highlights the suitability of the CLCT algorithm as a favourable option for conducting tropospheric-ozone retrievals in mid-latitude regions.
CHORA is an advanced version of the CCD technique developed at IUP Bremen. The Pacific cloud reference sector (CPC) scheme has been the established method for tropical tropospheric-ozone retrieval, which assumes a zonal invariance of stratospheric ozone. ACCO columns are retrieved over the tropical eastern Indian and western Pacific oceans (70° E–170° W) in the standard CCD method. These measurements are then subtracted from the total ozone column retrieved under clear-sky conditions for all grid boxes in the same zonal band (Ziemke et al., 1998; Valks et al., 2003, 2014; Leventidou et al., 2016, 2018; Hubert et al., 2021).
Due to the greater variability of stratospheric ozone in mid-latitudes, a local approach is necessary for the selection of the cloud reference sector. Thus, an advanced version of the CHORA algorithm has been developed. This version now employs a local-cloud reference sector (CLC) to compute the ACCO. The CLC algorithm also incorporates a homogeneity criterion for total ozone. Additionally, an alternative approach (CLCT) is introduced, allowing the direct ACCO calculation at the reference altitude of 270 hPa using Theil–Sen regression to retrieve the correction factor instead of an external ozone climatology.
Monthly averages of tropospheric-ozone columns were computed with the CPC, CLC, and CLCT algorithms for a latitude range from 26° S to 22° N using the S5P-TROPOMI reprocessed/offline version 02.04.01 cloud and ozone data for the time period from 2018 to 2022. The quality of the three algorithms was evaluated by comparing them with spatially collocated NASA/GSFC SHADOZ ozonesonde data and the TROPOMI level-2 tropospheric-ozone product from ESA.
Based on the analysis, CLCT exhibits a more accurate representation of seasonal variations in tropospheric ozone compared to the other CHORA algorithms (CPC and CLC). At eight out of nine tropical stations, the CLCT algorithm has demonstrated superior performance over the ESA operational product, delivering more precise and accurate tropospheric ozone. At eight of the nine ozonesonde stations, the CLCT algorithm yields better agreement with ozonesonde than CPC.
Local-cloud algorithms halve the overall mean bias and scatter compared to CPC across four Pacific sites (Hilo, Kuala Lumpur, Samoa, and Fiji), even with the cloud reference sectors for all being in the Pacific.
The advantage of local-cloud algorithms is more evident in the non-Pacific sector. They deliver more precise TCO retrievals and effectively mitigate CPC's consistent tendency to overestimate values across all seasons. They reduce the bias from 3 to 0.1 DU and halve the dispersion from 2 to 1 DU at five stations (Costa Rica, Paramaribo, Nairobi, Natal, and Ascension Island).
Within the tropical region, both the CCD-ESA and CPC algorithms tend to overestimate TCO retrievals, exhibiting a relative difference and standard deviation of 22 ± 10 % and 12 ± 10 %, respectively. This discrepancy arises from the CCD-ESA and CPC's tendency to underestimate ACCO derived from the Pacific sector. However, the CLCT algorithm significantly enhances retrieval accuracy by substantially mitigating this bias and reducing the associated dispersion (1 ± 7 %).
Notably, the performance improvement of the CLCT algorithm is exemplified by TCO retrievals at the equatorial ozonesonde station of Nairobi (1.3° S, 36.8° E), where the relative difference of CPC compared to CLCT is reduced from 19 % to 2 % (Fig. 15). Even at Hilo, a station subject to stratospheric intrusions from higher latitudes, the CLCT algorithm (−5 %) outperforms CPC (+30 %), yielding more accurate and sensible tropospheric-ozone retrievals.
The four sites in the subtropical sector (King's Park, Hanoi, Reunion, and Irene) experience more clouds during boreal summer and winter. This improves the effectiveness of local-cloud algorithms in these seasons. The local-cloud algorithms show larger variations due to complex ozone dynamics and fewer highly reflective clouds compared to the tropics. Both local-cloud approaches tend to underestimate TCO because of a high bias in ACCO. CLCT retrievals demonstrate better agreement with ozonesondes compared to CLC (−14 %), showing a reduced overall bias of −11 %.
Notably, at the subtropical station of Irene (25.9° S, 28.2° E), which experiences a unique combination of stratospheric and convective influences (Diab et al., 2004), TCO retrievals from CLCT (−7 %) show improved agreement with ozonesondes compared to TCO retrievals from CLC (−13 %) (Fig. 15).
The increased bias and scatter observed in the NH contributed by the CPC algorithm (14 ± 14 %) compared to the SH (10 ± 6 %) may be attributed to the complex dynamics of stratospheric ozone in this region. However, the application of the CLCT approach significantly mitigates this elevated scatter introduced by CPC and CCD-ESA in both hemispheres, reducing the bias and dispersion to −0.2 ± 10 % for the NH and 2 ± 3 % for the SH. This underscores the robustness of the CLCT algorithm in addressing challenges associated with substantial stratospheric-ozone variability.
In summary, the validation of TCO retrievals using CHORA algorithms against ozonesonde measurements at 13 stations located between 26° S and 22° N for the time period from 2018 to 2022 demonstrates that, except for one ozonesonde station, the CLCT algorithm consistently offers more accurate and dependable TCO retrievals in comparison to the CPC algorithm. In the tropics, the overall statistical bias is markedly reduced by both the CLC (−0.9 DU) and the CLCT approach (0.01 DU), representing more than half of that observed with CPC (2.5 DU).
These findings emphasise the advantages of the CLCT algorithm in yielding accurate TCO retrievals by mitigating the effects of spatio-temporal irregularities in stratospheric ozone in the outer tropics. This distinctive capability positions the CLCT algorithm as an optimal choice for tropospheric-ozone retrievals in ongoing and forthcoming missions of geostationary air quality satellites like ESA Sentinel 4, NASA Tempo, and GEMS (Korea), which predominantly cover mid-latitudes.
While both local-cloud algorithms contribute to enhanced accuracy and reduced variability in TCO retrievals compared to CPC, the CLCT algorithm slightly outperforms the CLC approach. This preference is primarily due to the CLCT algorithm's ability to circumvent unnecessary climatological corrections and its greater adaptability in accommodating varying cloud top pressures as reference altitudes. In addition to that, CLCT can combine the CCD method with cloud slicing to retrieve upper-tropospheric ozone volume mixing ratios. This integration enhances ozone measurement capabilities, showcasing the versatility of the CLCT algorithm. As a result, the CLCT algorithm is our preferred choice.
Using the CLCT approach in mid-latitudes may require the modification of the current retrieval technique, particularly the choice of the cloud reference sector due to different cloud and atmospheric conditions. Furthermore, a comprehensive assessment of S5P-TROPOMI cloud and ozone column statistics is crucial to establish suitable threshold values and boundary conditions for the CLCT algorithm. Additionally, refining the determination of the local-cloud reference sector could be essential to address the inhomogeneity of stratospheric ozone in mid-latitudes. This might involve an automated selection of the reference sector, including both longitudinal and latitudinal variations around each retrieval grid box.
In cases where the Theil–Sen method encounters limitations due to the uneven distribution of cloud top heights in mid-latitudes, the CLC algorithm can serve as a viable alternative. Notably, the CLC algorithm offers nearly equivalent accuracy in TCO retrievals. However, addressing the challenges posed by seasonal and geophysical variations in the occurrence of deep convective clouds remains critical for robustly estimating the stratospheric-ozone column. Furthermore, the scarcity and quality of ozonesonde data limit the effective validation of these retrieval methods. Addressing these challenges requires continuous improvement and refinement of the algorithms, which is crucial to advance the application of the CLCT approach to mid-latitude tropospheric-ozone retrievals.
Sentinel-5 Precursor TROPOMI data can be accessed through the Copernicus Open Access Hub at https://sentinels.copernicus.eu/documents/247904/2476257/ Sentinel-5P-ATBD-TROPOMI-Tropospheric-Ozone.pdf/ d2106102-b5c3-4d28-b752-026e3448aab2?t=1625507455328 (Heue et al., 2021). This data set is openly available for public use, subject to the data policy. Additionally, the ozonesonde data are also publicly accessible through the SHADOZ data archive according to the data policy available at https://doi.org/10.57721/SHADOZ-V06 (Stauffer and Thompson, 2021). The data that support the findings of this study are available from the authors (SMS, KE, or MW) upon request. The ozonesonde data for King's Park are publicly available through the WOUDC data archive at https://doi.org/10.14287/10000008 (WMO/GAW, 2023), in accordance with their respective data policies.
The supplement related to this article is available online at: https://doi.org/10.5194/amt-17-6459-2024-supplement.
SMS developed the CHORA local-cloud algorithms (CLC and CLCT), performed TCO retrievals using all CHORA algorithms, validated the results with CCD-ESA and SHADOZ ozonesonde measurements, and wrote the paper. KE provided the standard CHORA (CPC) algorithm and the CHOVA climatology and introduced the idea of the Theil–Sen regression. MW guided and provided expertise in developing the local-cloud algorithms. Both KE and MW actively supervised and participated in refining these algorithms. JPB provided scientific conceptual input and oversight. RS, AT, and DK provided the SHADOZ ozonesonde data. All authors provided comments and feedback to the manuscript.
At least one of the (co-)authors is a member of the editorial board of Atmospheric Measurement Techniques. The peer-review process was guided by an independent editor, and the authors also have no other competing interests to declare.
Publisher's note: Copernicus Publications remains neutral with regard to jurisdictional claims made in the text, published maps, institutional affiliations, or any other geographical representation in this paper. While Copernicus Publications makes every effort to include appropriate place names, the final responsibility lies with the authors.
This article is part of the special issue “Tropospheric Ozone Assessment Report Phase II (TOAR-II) Community Special Issue (ACP/AMT/BG/GMD inter-journal SI)”. It is not associated with a conference.
This work was funded, to a large degree, by the Federal Ministry for Economic Affairs and Climate Action (BMWi) under the TROPO3-MIDLAT project, which is part of the funding programme “Development of innovative methods for the creation of earth observation-based information products”. The support by the federal state of Bremen is gratefully acknowledged. This work uses Copernicus Sentinel-5 Precursor reprocessed/offline satellite data (2018–2022) and total ozone and cloud products, processed by the European Space Agency (ESA). The ozonesonde data were sourced from NASA's Southern Hemisphere ADditional OZonesondes programme (SHADOZ, https://doi.org/10.57721/SHADOZ-V06). We thank the principal investigators and staff at the ozonesonde stations for their dedicated efforts in maintaining high-quality measurements and for their valuable scientific contributions. The calculations for this research were conducted using IDL version 8.5.1.
This research has been supported by the Bundesministerium für Wirtschaft und Klimaschutz (grant no. 50EE1916).
The article processing charges for this open-access publication were covered by the University of Bremen.
This paper was edited by Steffen Beirle and reviewed by Klaus-Peter Heue and Juseon Bak.
Ahamad, F., Griffiths, P. T., Latif, M. T., Juneng, L., and Xiang, C. J.: Ozone trends from two decades of ground level observation in Malaysia, Atmosphere, 11, 755, https://doi.org/10.3390/atmos11070755, 2020. a
Avery, M., Twohy, C., McCabe, D., Joiner, J., Severance, K., Atlas, E., Blake, D., Bui, T. P., Crounse, J., Dibb, J., Diskin, G., Lawson, P., McGill, M., Rogers, D., Sachse, G., Scheuer, E., Thompson, A. M., Trepte, C., Wennberg, P., and Ziemke, J.: Convective distribution of tropospheric ozone and tracers in the Central American ITCZ region: Evidence from observations during TC4, J. Geophys. Res.-Atmos., 115, D00J21, https://doi.org/10.1029/2009JD013450, 2010. a
Baray, J.-L., Ancellet, G., Taupin, F., Bessafi, M., Baldy, S., and Keckhut, P.: Subtropical tropopause break as a possible stratospheric source of ozone in the tropical troposphere, J. Atmos. Solar-Terrest. Phys., 60, 27–36, https://doi.org/10.1016/S1364-6826(97)00116-8, 1998. a
Chandra, A., Koshy, K., and Maata, M.: Surface ozone profiles at selected South Pacific sites, The South Pac. J. Nat. Appl. Sci., 32, 47–54, https://doi.org/10.1071/SP14008, 2014. a, b, c
Compernolle, S., Argyrouli, A., Lutz, R., Sneep, M., Lambert, J.-C., Fjæraa, A. M., Hubert, D., Keppens, A., Loyola, D., O'Connor, E., Romahn, F., Stammes, P., Verhoelst, T., and Wang, P.: Validation of the Sentinel-5 Precursor TROPOMI cloud data with Cloudnet, Aura OMI O2-O2, MODIS, and Suomi-NPP VIIRS, Atmos. Meas. Tech., 14, 2451–2476, https://doi.org/10.5194/amt-14-2451-2021, 2021. a, b
Cooper, O., Stohl, A., Hübler, G., Hsie, E., Parrish, D., Tuck, A., Kiladis, G., Oltmans, S., Johnson, B., Shapiro, M., et al.: Direct transport of midlatitude stratospheric ozone into the lower troposphere and marine boundary layer of the tropical Pacific Ocean, J. Geophys. Res.-Atmos., 110, D23310, https://doi.org/10.1029/2005JD005783, 2005. a
Cooper, O., Parrish, D., Ziemke, J., Balashov, N., Cupeiro, M., Galbally, I., and Gilge, H.: S., Jensen, L. H., Lamarque, J, F., Naik, V., Oltmans, S., Schwab, J., Shindell, DT, Thompson, AM, Thouret, V., Wang, Y., and Zbinden, RM: Global distribution and trends of tropospheric ozone: An observation-based review, Elem. Sci. Anth, 2, 18 pp., https://doi.org/10.12952/journal.elementa.000029, 2014. a, b, c, d
Cooper, O. R., Schultz, M. G., Schröder, S., Chang, K.-L., Gaudel, A., Carbajal Benítez, G., Cuevas, E., Fröhlich, M., Galbally, I. E., Molloy, S., Kubistin, D., Lu, X., McClure-Begley, A., Nédélec, P., O’Brien, J., Oltmans, S. J., Petropavlovskikh, I., Ries, L., Senik, I., Sjöberg, K., Solberg, S., Spain, G. T., Spangl, W., Steinbacher, M., Tarasick, D., Thouret, V., and Xu, X.: Multi-decadal surface ozone trends at globally distributed remote locations, Elementa Sci. Anthropo., 8, 23, https://doi.org/10.1525/elementa.420, 2020. a
Crutzen, P. J.: Geology of mankind, in: Paul J. Crutzen: A pioneer on atmospheric chemistry and climate change in the Anthropocene, 211–215 pp., Springer, https://doi.org/10.1007/978-3-319-27460-7_10, 2016. a
Dam, D. A., Nguyen, T. K. O., et al.: Photochemical smog introduction and episode selection for the ground-level ozone in Hanoi, Vietnam, VNU J. Sci.-Earth Environ. Sci., 24, http://repository.vnu.edu.vn/handle/11126/5022 (last access: 12 October 2023), 2008. a
Daskalakis, N., Gallardo, L., Kanakidou, M., Nüß, J. R., Menares, C., Rondanelli, R., Thompson, A. M., and Vrekoussis, M.: Impact of biomass burning and stratospheric intrusions in the remote South Pacific Ocean troposphere, Atmos. Chem. Phys., 22, 4075–4099, https://doi.org/10.5194/acp-22-4075-2022, 2022. a
Diab, R., Thompson, A., Mari, K., Ramsay, L., and Coetzee, G.: Tropospheric ozone climatology over Irene, South Africa, from 1990 to 1994 and 1998 to 2002, J. Geophys. Res.-Atmos., 109, D20301, https://doi.org/10.1029/2004JD004793, 2004. a, b
ESA: S5P/TROPOMI ATBD Cloud Products, Technical note S5P-DLR-L2-ATBD-400I, ESA, https://sentinel.esa.int/documents/247904/2476257/Sentinel-5P-TROPOMI-ATBD-Clouds (last access: 21 June 2023), 2021a. a, b
ESA: Sentinel-5 precursor/TROPOMI Level 2 Product User Manual O3 Tropospheric Column, Technical note S5P-L2-IUP-ATBD-400C, ESA, https://sentinel.esa.int/documents/247904/2474726 (last access: 2 November 2023), 2021b. a
ESA: TROPOMI /S5P ATBD of tropospheric ozone data products, Technical note S5P-L2-IUP-ATBD-400C, ESA, https://sentinel.esa.int/documents/247904/2476257/Sentinel-5P-ATBD-TROPOMI-Tropospheric-Ozone (last access: 2 November 2023), 2021c. a
ESA: S5P/TROPOMI Total Ozone ATBD, Technical note S5P-L2-DLR-ATBD-400A, ESA, https://sentinel.esa.int/documents/247904/2476257/Sentinel-5P-TROPOMI-ATBD-Total-Ozone (last access: 21 June 2023), 2022. a, b, c
Fernandes, R. and Leblanc, S. G.: Parametric (modified least squares) and non-parametric (Theil–Sen) linear regressions for predicting biophysical parameters in the presence of measurement errors, Remote Sens. Environ., 95, 303–316, https://doi.org/10.1016/j.rse.2005.01.005, 2005. a
Fishman, J., Brackftt, V., and Fakhruzzaman, K.: Distribution of tropospheric ozone in the tropics from satellite and ozonesonde measurements, J. Atmos. Terrest. Phys., 54, 589–597, https://doi.org/10.1016/0021-9169(92)90099-7, 1992. a, b
Fleming, Z. L., Doherty, R. M., von Schneidemesser, E., Malley, C. S., Cooper, O. R., Pinto, J. P., Colette, A., Xu, X., Simpson, D., Schultz, M. G., Lefohn, A. S., Hamad, S., Moolla, R., Solberg, S., and Feng, Z.: Tropospheric Ozone Assessment Report: Present-day ozone distribution and trends relevant to human health, Elem. Sci. Anth., 6, 12, https://doi.org/10.1525/elementa.273, 2018. a
Folkins, I., Braun, C., Thompson, A. M., and Witte, J.: Tropical ozone as an indicator of deep convection, J. Geophys. Res.-Atmos., 107, ACH–13, https://doi.org/10.1029/2001JD001178, 2002. a, b
Garane, K., Koukouli, M.-E., Verhoelst, T., Lerot, C., Heue, K.-P., Fioletov, V., Balis, D., Bais, A., Bazureau, A., Dehn, A., Goutail, F., Granville, J., Griffin, D., Hubert, D., Keppens, A., Lambert, J.-C., Loyola, D., McLinden, C., Pazmino, A., Pommereau, J.-P., Redondas, A., Romahn, F., Valks, P., Van Roozendael, M., Xu, J., Zehner, C., Zerefos, C., and Zimmer, W.: TROPOMI/S5P total ozone column data: global ground-based validation and consistency with other satellite missions, Atmos. Meas. Tech., 12, 5263–5287, https://doi.org/10.5194/amt-12-5263-2019, 2019. a, b
Gaudel, A., Cooper, O. R., Ancellet, G., Barret, B., Boynard, A., Burrows, J., Clerbaux, C., Coheur, P.-F., Cuesta, J., Cuevas, E., et al.: Tropospheric Ozone Assessment Report: Present-day distribution and trends of tropospheric ozone relevant to climate and global atmospheric chemistry model evaluation, Elementa: Sci. Anthropo., 6, 39, https://doi.org/10.1525/elementa.291, 2018. a, b
Godin-Beekmann, S., Azouz, N., Sofieva, V. F., Hubert, D., Petropavlovskikh, I., Effertz, P., Ancellet, G., Degenstein, D. A., Zawada, D., Froidevaux, L., Frith, S., Wild, J., Davis, S., Steinbrecht, W., Leblanc, T., Querel, R., Tourpali, K., Damadeo, R., Maillard Barras, E., Stübi, R., Vigouroux, C., Arosio, C., Nedoluha, G., Boyd, I., Van Malderen, R., Mahieu, E., Smale, D., and Sussmann, R.: Updated trends of the stratospheric ozone vertical distribution in the 60° S–60° N latitude range based on the LOTUS regression model , Atmos. Chem. Phys., 22, 11657–11673, https://doi.org/10.5194/acp-22-11657-2022, 2022. a
Harris, J. M. and Oltmans, S. J.: Variations in tropospheric ozone related to transport at American Samoa, J. Geophys. Res.-Atmos., 102, 8781–8791, https://doi.org/10.1029/97JD00238, 1997. a
Heue, K.-P., Coldewey-Egbers, M., Delcloo, A., Lerot, C., Loyola, D., Valks, P., and van Roozendael, M.: Trends of tropical tropospheric ozone from 20 years of European satellite measurements and perspectives for the Sentinel-5 Precursor, Atmos. Meas. Tech., 9, 5037–5051, https://doi.org/10.5194/amt-9-5037-2016, 2016. a, b
Heue, K.-P., Eichmann, K.-U., and Valks, P.: TROPOMI/S5P ATBD of tropospheric ozone data products, Issue 2.3, Deutsches Zentrum für Luft-und Raum- fahrt e.V. in der Helmholtz-Gemeinschaft [data set], https://sentinels.copernicus.eu/documents/247904/2476257/Sentinel-5P-ATBD-TROPOMI-Tropospheric-Ozone.pdf/d2106102-b5c3-4d28-b752-026e3448aab2?t=1625507455328 (last access: 25 May 2024), 2021. a
Heue, K.-P., Loyola, D., Romahn, F., Zimmer, W., Chabrillat, S., Errera, Q., Ziemke, J., and Kramarova, N.: Tropospheric ozone retrieval by a combination of TROPOMI/S5P measurements with BASCOE assimilated data, Atmos. Meas. Tech., 15, 5563–5579, https://doi.org/10.5194/amt-15-5563-2022, 2022. a
Holton, J. R.: An introduction to dynamic meteorology, Am. J. Phys., 41, 752–754, https://doi.org/10.1119/1.1987371, 1973. a
Huang, G., Liu, X., Chance, K., Yang, K., Bhartia, P. K., Cai, Z., Allaart, M., Ancellet, G., Calpini, B., Coetzee, G. J. R., Cuevas-Agulló, E., Cupeiro, M., De Backer, H., Dubey, M. K., Fuelberg, H. E., Fujiwara, M., Godin-Beekmann, S., Hall, T. J., Johnson, B., Joseph, E., Kivi, R., Kois, B., Komala, N., König-Langlo, G., Laneve, G., Leblanc, T., Marchand, M., Minschwaner, K. R., Morris, G., Newchurch, M. J., Ogino, S.-Y., Ohkawara, N., Piters, A. J. M., Posny, F., Querel, R., Scheele, R., Schmidlin, F. J., Schnell, R. C., Schrems, O., Selkirk, H., Shiotani, M., Skrivánková, P., Stübi, R., Taha, G., Tarasick, D. W., Thompson, A. M., Thouret, V., Tully, M. B., Van Malderen, R., Vömel, H., von der Gathen, P., Witte, J. C., and Yela, M.: Validation of 10-year SAO OMI Ozone Profile (PROFOZ) product using ozonesonde observations, Atmos. Meas. Tech., 10, 2455–2475, https://doi.org/10.5194/amt-10-2455-2017, 2017. a
Hubert, D., Heue, K.-P., Lambert, J.-C., Verhoelst, T., Allaart, M., Compernolle, S., Cullis, P. D., Dehn, A., Félix, C., Johnson, B. J., Keppens, A., Kollonige, D. E., Lerot, C., Loyola, D., Maata, M., Mitro, S., Mohamad, M., Piters, A., Romahn, F., Selkirk, H. B., da Silva, F. R., Stauffer, R. M., Thompson, A. M., Veefkind, J. P., Vömel, H., Witte, J. C., and Zehner, C.: TROPOMI tropospheric ozone column data: geophysical assessment and comparison to ozonesondes, GOME-2B and OMI, Atmos. Meas. Tech., 14, 7405–7433, https://doi.org/10.5194/amt-14-7405-2021, 2021. a, b, c, d, e, f, g, h, i, j, k, l
Hübler, G., Montzka, D. D., Norton, R. B., Murphy, P. C., Fehsenfeld, F. C., Liu, S. C., Ridley, B. A., Walega, J. G., Atlas, E., Grahek, F. E., Heidt, L. E., Merrill, J., Huebert, B. J., and Bodhaine, B. A.: Total reactive oxidized nitrogen (NOy) in the remote Pacific troposphere and its correlation with O3 and CO: Mauna Loa Observatory Photochemistry Experiment 1988, J. Geophys. Res.-Atmos., 97, 10427–10447, https://doi.org/10.1029/91JD03112, 1992. a
Hudson, R. D. and Thompson, A. M.: Tropical tropospheric ozone from total ozone mapping spectrometer by a modified residual method, J. Geophys. Res.-Atmos., 103, 22129–22145, https://doi.org/10.1029/98JD00729, 1998. a
Ingmann, P., Veihelmann, B., Langen, J., Lamarre, D., Stark, H., and Courrèges-Lacoste, G. B.: Requirements for the GMES Atmosphere Service and ESA's implementation concept: Sentinels-4/-5 and-5p, Remote Sens. Environ., 120, 58–69, https://doi.org/10.1016/j.rse.2012.01.023, 2012. a
Iriti, M. and Faoro, F.: Oxidative stress, the paradigm of ozone toxicity in plants and animals, Water Air Soil Pollut., 187, 285–301, https://doi.org/10.1007/s11270-007-9517-7, 2008. a
Jaffe, D. A., Cooper, O. R., Fiore, A. M., Henderson, B. H., Tonnesen, G. S., Russell, A. G., Henze, D. K., Langford, A. O., Lin, M., and Moore, T.: Scientific assessment of background ozone over the US: Implications for air quality management, Elementa Sci. Anthropo., 6, 56, https://doi.org/10.1525/elementa.309, 2018. a
Jensen, A. A., Thompson, A. M., and Schmidlin, F.: Classification of Ascension Island and Natal ozonesondes using self-organizing maps, J. Geophys. Res.-Atmos., 117, D04302, https://doi.org/10.1029/2011JD016573, 2012. a, b, c, d
Kentarchos, A., Roelofs, G., and Lelieveld, J.: Altitude distribution of tropospheric ozone over the northern hemisphere during 1996, simulated with a chemistry-general circulation model at two different horizontal resolutions, J. Geophys. Res.-Atmos., 106, 17453–17469, https://doi.org/10.1029/2000JD900770, 2001. a
Kimayu, J. M., Gikuma-Njuru, P., and Musembi, D. K.: Temporal and spatial variability of tropospheric ozone in Nairobi City, Kenya, Physical Science International Journal Article, ISSN 2348-0130, https://doi.org/10.9734/PSIJ/2017/31452, 2017. a
Kleipool, Q., Ludewig, A., Babić, L., Bartstra, R., Braak, R., Dierssen, W., Dewitte, P.-J., Kenter, P., Landzaat, R., Leloux, J., Loots, E., Meijering, P., van der Plas, E., Rozemeijer, N., Schepers, D., Schiavini, D., Smeets, J., Vacanti, G., Vonk, F., and Veefkind, P.: Pre-launch calibration results of the TROPOMI payload on-board the Sentinel-5 Precursor satellite, Atmos. Meas. Tech., 11, 6439–6479, https://doi.org/10.5194/amt-11-6439-2018, 2018. a, b
Lerot, C., Van Roozendael, M., Lambert, J.-C., Granville, J., Van Gent, J., Loyola, D., and Spurr, R.: The GODFIT algorithm: a direct fitting approach to improve the accuracy of total ozone measurements from GOME, International J. Remote Sens., 31, 543–550, https://doi.org/10.1080/01431160902893576, 2010. a
Lerot, C., Van Roozendael, M., Spurr, R., Loyola, D., Coldewey-Egbers, M., Kochenova, S., van Gent, J., Koukouli, M., Balis, D., Lambert, J.-C., Granville, J., and Zehner, C.: Homogenized total ozone data records from the European sensors GOME/ERS-2, SCIAMACHY/Envisat, and GOME-2/MetOp-A, J. Geophys. Res.-Atmos., 119, 1639–1662, https://doi.org/10.1002/2013JD020831, 2014. a, b
Leventidou, E., Eichmann, K.-U., Weber, M., and Burrows, J. P.: Tropical tropospheric ozone columns from nadir retrievals of GOME-1/ERS-2, SCIAMACHY/Envisat, and GOME-2/MetOp-A (1996–2012), Atmos. Meas. Tech., 9, 3407–3427, https://doi.org/10.5194/amt-9-3407-2016, 2016. a, b, c, d, e, f, g, h, i, j
Leventidou, E., Weber, M., Eichmann, K.-U., Burrows, J. P., Heue, K.-P., Thompson, A. M., and Johnson, B. J.: Harmonisation and trends of 20-year tropical tropospheric ozone data, Atmos. Chem. Phys., 18, 9189–9205, https://doi.org/10.5194/acp-18-9189-2018, 2018. a, b, c, d
Li, S., Yang, Y., Wang, H., Li, P., Li, K., Ren, L., Wang, P., Li, B., Mao, Y., and Liao, H.: Rapid increase in tropospheric ozone over Southeast Asia attributed to changes in precursor emission source regions and sectors, Atmos. Environ., 304, 119776, https://doi.org/10.1016/j.atmosenv.2023.119776, 2023. a
Liao, Z., Ling, Z., Gao, M., Sun, J., Zhao, W., Ma, P., Quan, J., and Fan, S.: Tropospheric ozone variability over Hong Kong based on recent 20 years (2000–2019) ozonesonde observation, J. Geophys. Res.-Atmos., 126, e2020JD033054, https://doi.org/10.1029/2020JD033054, 2021. a, b
Liu, H., Jacob, D. J., Chan, L. Y., Oltmans, S. J., Bey, I., Yantosca, R. M., Harris, J. M., Duncan, B. N., and Martin, R. V.: Sources of tropospheric ozone along the Asian Pacific Rim: An analysis of ozonesonde observations, J. Geophys. Res.-Atmos., 107, ACH–3, https://doi.org/10.1029/2001JD002005, 2002. a, b
Logan, J. A.: An analysis of ozonesonde data for the troposphere: Recommendations for testing 3-D models and development of a gridded climatology for tropospheric ozone, J. Geophys. Res.-Atmos., 104, 16115–16149, https://doi.org/10.1029/1998JD100096, 1999. a
Loyola, D. and Ruppert, T.: A new PMD cloud-recognition algorithm for GOME, ESA Earth Observ. Q., 58, 45–47, https://elib.dlr.de/53/ (last access: 20 June 2023), 1998. a
Loyola, D. G., Koukouli, M. E., Valks, P., Balis, D. S., Hao, N., Van Roozendael, M., Spurr, R. J. D., Zimmer, W., Kiemle, S., Lerot, C., and Lambert, J.-C.: The GOME-2 total column ozone product: Retrieval algorithm and ground-based validation, J. Geophys. Res.-Atmos., 116, D07302, https://doi.org/10.1029/2010JD014675, 2011. a
Loyola, D. G., Gimeno García, S., Lutz, R., Argyrouli, A., Romahn, F., Spurr, R. J. D., Pedergnana, M., Doicu, A., Molina García, V., and Schüssler, O.: The operational cloud retrieval algorithms from TROPOMI on board Sentinel-5 Precursor, Atmos. Meas. Tech., 11, 409–427, https://doi.org/10.5194/amt-11-409-2018, 2018. a, b
Lu, X., Zhang, L., Liu, X., Gao, M., Zhao, Y., and Shao, J.: Lower tropospheric ozone over India and its linkage to the South Asian monsoon, Atmos. Chem. Phys., 18, 3101–3118, https://doi.org/10.5194/acp-18-3101-2018, 2018. a
Ludewig, A., Kleipool, Q., Bartstra, R., Landzaat, R., Leloux, J., Loots, E., Meijering, P., van der Plas, E., Rozemeijer, N., Vonk, F., and Veefkind, P.: In-flight calibration results of the TROPOMI payload on board the Sentinel-5 Precursor satellite, Atmos. Meas. Tech., 13, 3561–3580, https://doi.org/10.5194/amt-13-3561-2020, 2020. a
McPeters, R. D., Labow, G. J., and Logan, J. A.: Ozone climatological profiles for satellite retrieval algorithms, J. Geophys. Res.-Atmos., 112, D05308, https://doi.org/10.1029/2005JD006823, 2007. a
Mills, G., Pleijel, H., Malley, C. S., Sinha, B., Cooper, O. R., Schultz, M. G., Neufeld, H. S., Simpson, D., Sharps, K., Feng, Z., Gerosa, G., Harmens, H., Kobayashi, K., Saxena, P., Paoletti, E., Sinha, V., and Xu, X.: Tropospheric Ozone Assessment Report: Present-day tropospheric ozone distribution and trends relevant to vegetation, Elem. Sci. Anth., 6, 47, https://doi.org/10.1525/elementa.302, 2018. a
Mkololo, T., Mbatha, N., Sivakumar, V., Bègue, N., Coetzee, G., and Labuschagne, C.: Stratosphere–Troposphere exchange and O3 variability in the lower stratosphere and upper troposphere over the irene SHADOZ site, South Africa, Atmosphere, 11, 586, https://doi.org/10.3390/atmos11060586, 2020. a
Mohtar, A. A. A., Latif, M. T., Baharudin, N. H., Ahamad, F., Chung, J. X., Othman, M., and Juneng, L.: Variation of major air pollutants in different seasonal conditions in an urban environment in Malaysia, Geosci. Lett., 5, 1–13, https://doi.org/10.1186/s40562-018-0122-y, 2018. a
Monks, P. S., Archibald, A. T., Colette, A., Cooper, O., Coyle, M., Derwent, R., Fowler, D., Granier, C., Law, K. S., Mills, G. E., Stevenson, D. S., Tarasova, O., Thouret, V., von Schneidemesser, E., Sommariva, R., Wild, O., and Williams, M. L.: Tropospheric ozone and its precursors from the urban to the global scale from air quality to short-lived climate forcer, Atmos. Chem. Phys., 15, 8889–8973, https://doi.org/10.5194/acp-15-8889-2015, 2015. a
Ogino, S.-Y., Miyazaki, K., Fujiwara, M., Nodzu, M. I., Shiotani, M., Hasebe, F., Matsumoto, J., Witte, J., Thompson, A. M., Nguyen-Thi, H. A., and Nguyen, T. V.: Cause of a Lower-Tropospheric High-Ozone Layer in Spring Over Hanoi, J. Geophys. Res.-Atmos., 127, e2021JD035727, https://doi.org/10.1029/2021JD035727, 2022. a
Olson, J. R., Fishman, J., Kirchhoff, V. W., Nganga, D., and Cros, B.: Analysis of the distribution of ozone over the southern Atlantic region, J. Geophys. Res.-Atmos., 101, 24083–24093, https://doi.org/10.1029/95JD03273, 1996. a
Oltmans, S. J., Lefohn, A. S., Shadwick, D., Harris, J. M., Scheel, H. E., Galbally, I., Tarasick, D. W., Johnson, B. J., Brunke, E.-G., Claude, H., Zeng, G., Nichol, S., Schmidlin, F., Davies, J., Cuevas, E., Redondas, A., Naoe, H., Nakano, T., and Kawasato, T.: Recent tropospheric ozone changes–A pattern dominated by slow or no growth, Atmos. Environ., 67, 331–351, https://doi.org/10.1016/j.atmosenv.2012.10.057, 2013. a
Oltmans, S. J., Hofmann, D. J., Lathrop, J. A., Harris, J. M., Komhyr, W. D., and Kuniyuki, D.: Tropospheric ozone during Mauna Loa Observatory Photochemistry Experiment 2 compared to long-term measurements from surface and ozonesonde observations, J. Geophys. Res.-Atmos., 101, 14569–14580, https://doi.org/10.1029/95JD03004, 1996. a, b
Oltmans, S. J., Johnson, B. J., Harris, J. M., Vömel, H., Thompson, A. M., Koshy, K., Simon, P., Bendura, R. J., Logan, J. A., Hasebe, F., Shiotani, M., Kirchhoff, V. W. J. H., Maata, M., Sami, G., Samad, A., Tabuadravu, J., Enriquez, H., Agama, M., Cornejo, J., and Paredes, F.: Ozone in the Pacific tropical troposphere from ozonesonde observations, J. Geophys. Res.-Atmos., 106, 32503–32525, https://doi.org/10.1029/2000JD900834, 2001. a
Peters, W., Krol, M., Fortuin, J., Kelder, H., Thompson, A., Becker, C., Lelieveld, J., and Crutzen, P.: Tropospheric ozone over a tropical Atlantic station in the Northern Hemisphere: Paramaribo, Surinam (6N, 55W), Tellus B, 56, 21–34, https://doi.org/10.3402/tellusb.v56i1.16398, 2004. a, b
Pfister, L., Selkirk, H., Starr, D., Rosenlof, K., and Newman, P.: A meteorological overview of the TC4 mission, J. Geophys. Res.-Atmos., 115, D00J12, https://doi.org/10.1029/2009JD013316, 2010. a
Pickering, K. E., Thompson, A. M., Wang, Y., Tao, W.-K., McNamara, D. P., Kirchhoff, V. W. J. H., Heikes, B. G., Sachse, G. W., Bradshaw, J. D., Gregory, G. L., and Blake, D. R.: Convective transport of biomass burning emissions over Brazil during TRACE A, J. Geophys. Res.-Atmos., 101, 23993–24012, https://doi.org/10.1029/96JD00346, 1996. a
Pickering, K. E., Thompson, A. M., Kim, H., DeCaria, A. J., Pfister, L., Kucsera, T. L., Witte, J. C., Avery, M. A., Blake, D. R., Crawford, J. H., Heikes, B. G., Sachse, G. W., Sandholm, S. T., and Talbot, R. W.: Trace gas transport and scavenging in PEM-Tropics B South Pacific Convergence Zone convection, J. Geophys. Res.-Atmos., 106, 32591–32607, https://doi.org/10.1029/2001JD000328, 2001. a
Poulida, O., Dickerson, R. R., and Heymsfield, A.: Stratosphere-troposphere exchange in a midlatitude mesoscale convective complex: 1. Observations, J. Geophys. Res.-Atmos., 101, 6823–6836, https://doi.org/10.1029/95JD03523, 1996. a
Putero, D., Cristofanelli, P., Chang, K.-L., Dufour, G., Beachley, G., Couret, C., Effertz, P., Jaffe, D. A., Kubistin, D., Lynch, J., Petropavlovskikh, I., Puchalski, M., Sharac, T., Sive, B. C., Steinbacher, M., Torres, C., and Cooper, O. R.: Fingerprints of the COVID-19 economic downturn and recovery on ozone anomalies at high-elevation sites in North America and western Europe, Atmos. Chem. Phys., 23, 15693–15709, https://doi.org/10.5194/acp-23-15693-2023, 2023. a
Randriambelo, T., Baray, J.-L., and Baldy, S.: Effect of biomass burning, convective venting, and transport on tropospheric ozone over the Indian Ocean: Reunion Island field observations, J. Geophys. Res.-Atmos., 105, 11813–11832, https://doi.org/10.1029/1999JD901097, 2000. a
Ratisbona, L.: The climate of Brazil, in: Climates of Central and South America, edited by: Schwerdtfeger, W., 1976. a
Rodriguez, D. G. L., Thomas, W., Livschitz, Y., Ruppert, T., Albert, P., and Hollmann, R.: Cloud properties derived from GOME/ERS-2 backscatter data for trace gas retrieval, IEEE T. Geosci. Remote Sens., 45, 2747–2758, https://doi.org/10.1109/TGRS.2007.901043, 2007. a
Schoeberl, M. R., Selkirk, H. B., Vömel, H., and Douglass, A. R.: Sources of seasonal variability in tropical upper troposphere and lower stratosphere water vapor and ozone: Inferences from the Ticosonde data set at Costa Rica, J. Geophys. Res.-Atmos., 120, 9684–9701, https://doi.org/10.1002/2015JD023299, 2015. a
Seguel, R. J., Castillo, L., Opazo, C., Rojas, N. Y., Nogueira, T., Cazorla, M., Gavidia-Calderón, M., Gallardo, L., Garreaud, R., Carrasco-Escaff, T., and Elshorbany, Y.: Changes in South American Surface Ozone Trends: Exploring the Influences of Precursors and Extreme Events, EGUsphere [preprint], https://doi.org/10.5194/egusphere-2024-328, 2024. a
Sen, P. K.: Estimates of the regression coefficient based on Kendall's tau, J. Am. Stat. Assoc., 63, 1379–1389, https://doi.org/10.1080/01621459.1968.10480934, 1968. a
Shilenje, Z., Ongoma, V., and Ogwang, B.: Upper tropospheric and stratospheric ozone over equatorial East Africa; case study of Nairobi County, Kenya, Ethiopian J. Environ. Stud. Manage., 8, 290–300, https://doi.org/10.4314/ejesm.v8i3.6, 2015. a
Škerlak, B., Sprenger, M., and Wernli, H.: A global climatology of stratosphere–troposphere exchange using the ERA-Interim data set from 1979 to 2011, Atmos. Chem. Phys., 14, 913–937, https://doi.org/10.5194/acp-14-913-2014, 2014. a
Slingo, J., Spencer, H., Hoskins, B., Berrisford, P., and Black, E.: The meteorology of the Western Indian Ocean, and the influence of the East African Highlands, Philosophical Transactions of the Royal Society A: Mathematical, Phys. Eng. Sci., 363, 25–42, https://doi.org/10.1098/rsta.2004.1473, 2005. a
Sonkaew, T. and Macatangay, R.: Determining relationships and mechanisms between tropospheric ozone column concentrations and tropical biomass burning in Thailand and its surrounding regions, Environ. Res. Lett., 10, 065009, https://doi.org/10.1088/1748-9326/10/6/065009, 2015. a
Stauffer, R. M. and Thompson, A. M.: SHADOZ – Southern Hemi- sphere ADditional OZonesondes, NASA/Goddard Space Flight Center [data set], https://doi.org/10.57721/SHADOZ-V06, 2021. a
Sterling, C. W., Johnson, B. J., Oltmans, S. J., Smit, H. G. J., Jordan, A. F., Cullis, P. D., Hall, E. G., Thompson, A. M., and Witte, J. C.: Homogenizing and estimating the uncertainty in NOAA's long-term vertical ozone profile records measured with the electrochemical concentration cell ozonesonde, Atmos. Meas. Tech., 11, 3661–3687, https://doi.org/10.5194/amt-11-3661-2018, 2018. a
Szopa, S., Naik, V., Adhikary, B., Artaxo, P., Berntsen, T., Collins, W. D., Fuzzi, S., Gallardo, L., Kiendler-Scharr, A., Klimont, Z., Liao, H., Unger, N., and Zanis, P.: Short-Lived Climate Forcers (Chap. 6), https://doi.org/10.1017/9781009157896.008, 2021. a
Tarasick, D. W., Smit, H. G., Thompson, A. M., Morris, G. A., Witte, J. C., Davies, J., Nakano, T., Van Malderen, R., Stauffer, R. M., Johnson, B. J., et al.: Improving ECC ozonesonde data quality: Assessment of current methods and outstanding issues, Earth Space Sci., 8, 27 pp., https://doi.org/10.1029/2019EA000914, 2021. a
Taupin, F., Bessafi, M., Baldy, S., and Bremaud, P.: Tropospheric ozone above the southwestern Indian Ocean is strongly linked to dynamical conditions prevailing in the tropics, J. Geophys. Res.-Atmos., 104, 8057–8066, https://doi.org/10.1029/98JD02456, 1999. a
Thompson, A., Pickering, K., McNamara, D., Schoeberl, M., Hudson, R., Kim, J., Browell, E., Kirchhoff, V., and Nganga, D.: Where did tropospheric ozone over southern Africa and the tropical Atlantic come from in October 1992? Insights from TOMS, GTE TRACE A, and SAFARI 1992, J. Geophys. Res.-Atmos., 101, 24251–24278, https://doi.org/10.1029/96JD01463, 1996. a
Thompson, A. M., Doddridge, B. G., Witte, J. C., Hudson, R. D., Luke, W. T., Johnson, J. E., Johnson, B. J., Oltmans, S. J., and Weller, R.: A tropical Atlantic paradox: Shipboard and satellite views of a tropospheric ozone maximum and wave-one in January–February 1999, Geophys. Res. Lett., 27, 3317–3320, https://doi.org/10.1029/1999GL011273, 2000. a, b, c, d, e
Thompson, A. M., Witte, J. C., Oltmans, S. J., Schmidlin, F. J., Logan, J. A., Fujiwara, M., Kirchhoff, V. W. J. H., Posny, F., Coetzee, G. J. R., Hoegger, B., Kawakami, S., Ogawa, T., Fortuin, J. P. F., and Kelder, H. M.: Southern Hemisphere Additional Ozonesondes (SHADOZ) 1998–2000 tropical ozone climatology 2. Tropospheric variability and the zonal wave-one, J. Geophys. Res.-Atmos., 108, 8241, https://doi.org/10.1029/2002JD002241, 2003. a, b, c, d, e, f
Thompson, A. M., Miller, S. K., Tilmes, S., Kollonige, D. W., Witte, J. C., Oltmans, S. J., Johnson, B. J., Fujiwara, M., Schmidlin, F. J., Coetzee, G. J. R., Komala, N., Maata, M., Mohamad, M. B. N., Nguyo, J., Mutai, C., Ogino, S.-Y., Da Silva, F. R., Paes Leme, N. M., Posny, F., Scheele, R., Selkirk, H. B., Shiotani, M., Stübi, R., Levrat, G., Calpini, B., Thouret, V., Tsuruta, H., Valverde Canossa, J., Vömel, H., Yonemura, S., Díaz, J. A., Thanh, N. T. T., and Thuy Ha, H. T.: Southern Hemisphere Additional Ozonesondes (SHADOZ) ozone climatology (2005–2009): Tropospheric and tropical tropopause layer (TTL) profiles with comparisons to OMI-based ozone products, J. Geophys. Res.-Atmos., 117, D23301, https://doi.org/10.1029/2011JD016911, 2012. a
Thompson, A. M., Witte, J. C., Sterling, C., Jordan, A., Johnson, B. J., Oltmans, S. J., Fujiwara, M., Vömel, H., Allaart, M., Piters, A., Coetzee, G. J. R., Posny, F., Corrales, E., Diaz, J. A., Félix, C., Komala, N., Lai, N., Nguyen, H. T. A., Maata, M., Mani, F., Zainal, Z., Ogino, S., Paredes, F., Penha, T. L. B., Silva, F. R., Sallons-Mitro, S., Selkirk, H. B., Schmidlin, F. J., Stübi, R., and Thiongo, K.: First reprocessing of Southern Hemisphere Additional Ozonesondes (SHADOZ) ozone profiles (1998–2016): 2. Comparisons with satellites and ground-based instruments, J. Geophys. Res.-Atmos., 122, 13–000, https://doi.org/10.1002/2017JD027406, 2017. a, b, c, d, e
Thompson, A. M., Smit, H. G. J., Witte, J. C., Stauffer, R. M., Johnson, B. J., Morris, G., von der Gathen, P., Van Malderen, R., Davies, J., Piters, A., Allaart, M., Posny, F., Kivi, R., Cullis, P., Nguyen, T. H. A., Corrales, E., Machinini, T., da Silva, F. R., Paiman, G., Thiong’o, K., Zainal, Z., Brothers, G. B., Wolff, K. R., Nakano, T., Stübi, R., Romanens, G., Coetzee, G. J. R., Diaz, J. A., Mitro, S., Mohamad, M., and Ogino, S.-Y.: Ozonesonde quality assurance: The JOSIE–SHADOZ (2017) experience, B. Am. Meteorol. Soc., 100, 155–171, https://doi.org/10.1175/BAMS-D-17-0311.1, 2019. a
Thompson, A. M., Stauffer, R. M., Wargan, K., Witte, J. C., Kollonige, D. E., and Ziemke, J. R.: Regional and seasonal trends in tropical ozone from SHADOZ profiles: Reference for models and satellite products, J. Geophys. Res.-Atmos., 126, e2021JD034691, https://doi.org/10.1029/2021JD034691, 2021. a
Valks, P., Koelemeijer, R., Van Weele, M., Van Velthoven, P., Fortuin, J., and Kelder, H.: Variability in tropical tropospheric ozone: Analysis with Global Ozone Monitoring Experiment observations and a global model, J. Geophys. Res.-Atmos., 108, 4328, https://doi.org/10.1029/2002JD002894, 2003. a, b, c
Valks, P., Hao, N., Gimeno Garcia, S., Loyola, D., Dameris, M., Jöckel, P., and Delcloo, A.: Tropical tropospheric ozone column retrieval for GOME-2, Atmos. Meas. Tech., 7, 2513–2530, https://doi.org/10.5194/amt-7-2513-2014, 2014. a, b, c, d, e
Van Roozendael, M., Loyola, D., Spurr, R., Balis, D., Lambert, J.-C., Livschitz, Y., Valks, P., Ruppert, T., Kenter, P., Fayt, C., and Zehner, C.: Ten years of GOME/ERS-2 total ozone data—The new GOME data processor (GDP) version 4: 1. Algorithm description, J. Geophys. Res.-Atmos., 111, D14311, https://doi.org/10.1029/2005JD006375, 2006. a
Van Roozendael, M., Spurr, R., Loyola, D., Lerot, C., Balis, D., Lambert, J.-C., Zimmer, W., van Gent, J., van Geffen, J., Koukouli, M., Granville, J., Doicu, A., Fayt, C., and Zehner, C.: Sixteen years of GOME/ERS-2 total ozone data: The new direct-fitting GOME Data Processor (GDP) version 5—Algorithm description, J. Geophys. Res.-Atmos., 117, https://doi.org/10.1029/2011JD016471, 2012. a, b
Veefkind, J.P., Aben, I., McMullan, K., Förster, H., de Vries, J., Otter, G., Claas, J., Eskes, H.J., de Haan, J.F., Kleipool, Q., van Weele, M., Hasekamp, O., Hoogeveen, R., Landgraf, J., Snel, R., Tol, P., Ingmann, P., Voors, R., Kruizinga, B., Vink, R., Visser, H., and Levelt, P. F.: TROPOMI on the ESA Sentinel-5 Precursor: A GMES mission for global observations of the atmospheric composition for climate, air quality and ozone layer applications, Remote Sens. Environ., 120, 70–83, https://doi.org/10.1016/j.rse.2011.09.027, 2012. a
Wai, K. M., Wu, S., Kumar, A., and Liao, H.: Seasonal variability and long-term evolution of tropospheric composition in the tropics and Southern Hemisphere, Atmos. Chem. Phys., 14, 4859–4874, https://doi.org/10.5194/acp-14-4859-2014, 2014. a
Wang, Y. and Jacob, D. J.: Anthropogenic forcing on tropospheric ozone and OH since preindustrial times, J. Geophys. Res.-Atmos., 103, 31123–31135, https://doi.org/10.1029/1998JD100004, 1998. a
Weber, M., Dikty, S., Burrows, J. P., Garny, H., Dameris, M., Kubin, A., Abalichin, J., and Langematz, U.: The Brewer-Dobson circulation and total ozone from seasonal to decadal time scales, Atmos. Chem. Phys., 11, 11221–11235, https://doi.org/10.5194/acp-11-11221-2011, 2011. a
Wilcox, R. R.: Introduction to robust estimation and hypothesis testing, Academic press, 690 pp., https://doi.org/10.1080/00401706.1998.10485491, 2011. a
Williams, R. S., Hegglin, M. I., Kerridge, B. J., Jöckel, P., Latter, B. G., and Plummer, D. A.: Characterising the seasonal and geographical variability in tropospheric ozone, stratospheric influence and recent changes, Atmos. Chem. Phys., 19, 3589–3620, https://doi.org/10.5194/acp-19-3589-2019, 2019. a
Witte, J. C., Thompson, A. M., Smit, H. G. J., Fujiwara, M., Posny, F., Coetzee, G. J. R., Northam, E. T., Johnson, B. J., Sterling, C. W., Mohamad, M., Ogino, S.-Y., Jordan, A., and da Silva, F. R.: First reprocessing of Southern Hemisphere ADditional OZonesondes (SHADOZ) profile records (1998–2015): 1. Methodology and evaluation, J. Geophys. Res.-Atmos., 122, 6611–6636, https://doi.org/10.1002/2016JD026403, 2017. a
WMO/GAW: Ozone Monitoring Community: OzoneSonde, World Meteorological Organization-Global Atmosphere Watch Program (WMO-GAW)/World Ozone and Ul- traviolet Radiation Data Centre (WOUDC), Government of Canada [data set], https://doi.org/10.14287/10000008, 2023. a
Yonemura, S., Tsuruta, H., Kawashima, S., Sudo, S., Peng, L. C., Fook, L. S., Johar, Z., and Hayashi, M.: Tropospheric ozone climatology over Peninsular Malaysia from 1992 to 1999, J. Geophys. Res.-Atmos., 107, ACH–1, https://doi.org/10.1029/2001JD000993, 2002. a, b
Zhang, Y., Cooper, O. R., Gaudel, A., Thompson, A. M., Nédélec, P., Ogino, S.-Y., and West, J. J.: Tropospheric ozone change from 1980 to 2010 dominated by equatorward redistribution of emissions, Nat. Geosci., 9, 875–879, https://doi.org/10.1038/ngeo2827, 2016. a
Ziemke, J. and Stanford, J.: Kelvin waves in total column ozone, Geophys. Res. Lett., 21, 105–108, https://doi.org/10.1029/93GL03287, 1994. a
Ziemke, J., Chandra, S., and Bhartia, P.: Two new methods for deriving tropospheric column ozone from TOMS measurements: Assimilated UARS MLS/HALOE and convective-cloud differential techniques, J. Geophys. Res.-Atmos., 103, 22115–22127, https://doi.org/10.1029/98JD01567, 1998. a, b, c, d, e
Ziemke, J., Chandra, S., and Bhartia, P.: A 25-year data record of atmospheric ozone in the Pacific from Total Ozone Mapping Spectrometer (TOMS) cloud slicing: Implications for ozone trends in the stratosphere and troposphere, J. Geophys. Res.-Atmos., 110, D15105, https://doi.org/10.1029/2004JD005687, 2005. a
Ziemke, J. R., Chandra, S., Oman, L. D., and Bhartia, P. K.: A new ENSO index derived from satellite measurements of column ozone, Atmos. Chem. Phys., 10, 3711–3721, https://doi.org/10.5194/acp-10-3711-2010, 2010. a, b, c