the Creative Commons Attribution 4.0 License.
the Creative Commons Attribution 4.0 License.
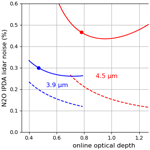
Design study for an airborne N2O lidar
Andreas Fix
Christian Fruck
Gerhard Ehret
Martin Wirth
Nitrous oxide (N2O) is the third most important greenhouse gas modified by human activities after carbon dioxide and methane. This study examines the feasibility of airborne differential absorption lidar to measure N2O concentration enhancements over agricultural, fossil fuel combustion, industrial, and biomass burning sources. The mid-infrared spectral region, where suitably strong N2O absorption lines exist, challenges passive remote sensing by means of spectroscopy due to both low solar radiation and thermal emission. Lidar remote sensing is principally possible thanks to the laser as an independent radiation source but has not yet been realized due to technological challenges. Mid-infrared N2O absorption bands suitable for remote sensing are investigated. Simulations show that a spectral trough position between two strong N2O lines in the 4.5 µm band is the favored option. A second option exists in the 3.9 µm band at the cost of higher laser frequency stability constraints and less measurement sensitivity. Both options fulfill the N2O measurement requirements for agricultural areal or point-source emission quantification (0.5 % measurement precision, 500 m spatial resolution) with technically realizable and affordable transmitter (100 mW average laser power) and receiver (20 cm telescope) characteristics for integrated-path differential absorption lidar that measures the column concentration beneath the aircraft. The development of an airborne N2O lidar is feasible yet would benefit from progress in infrared laser transmitter and low-noise-detection technology. It will also serve as a precursor to space versions, which are still out of reach due to the lack of space technology.
- Article
(897 KB) - Full-text XML
- BibTeX
- EndNote
The average concentration of nitrous oxide (N2O) amounts to only 337 ppb yet its global warming potential is nearly 300 times that of CO2 on a 100-year span (Arias et al., 2021). This makes N2O the third most important greenhouse gas contributing to human-induced global warming after carbon dioxide and methane. The major anthropogenic source is nitrogen fertilization on arable land. Further N2O sources are processes in the chemical industry and combustion processes. According to current knowledge, anthropogenic sources contribute ∼ 36 % to total global N2O emissions (Tian et al., 2024). Emissions from natural soils and oceans constitute the major natural sources. Agricultural N2O emissions are increasing due to interactions between nitrogen inputs and global warming, constituting an emerging positive N2O–climate feedback. The recent increase in global N2O emissions exceeds even the most pessimistic emission trend scenarios developed by the IPCC, underscoring the urgency to mitigate N2O emissions (Tian et al., 2024). Estimating N2O emissions from agriculture is inherently complex and comes with a high degree of uncertainty due to variability in weather and soil characteristics, in agricultural management options, and in the interaction of field management with environmental variables (Eckl et al., 2021). Moreover, N2O measurements are sparse. Consequently, more comprehensive N2O concentration measurements are needed, particularly by means of remote sensing. Recently, the World Meteorological Organization has launched the Global Greenhouse Gas Watch (G3W) initiative to endorse, among others, this need (WMO, 2024).
The mid-infrared (mid-IR) spectral region, where suitably strong N2O absorption lines exist, challenges passive remote sensing by means of spectroscopy due to both low solar radiation and thermal emission from Earth's surface (e.g., Ricaud et al., 2021; Vandenbussche et al., 2022). Lidar remote sensing is principally possible thanks to the laser as an independent radiation source but has not yet been realized due to technological challenges. While mid-IR lidars are employed for ground-based pollution detection (e.g., Robinson et al., 2011; Gong et al., 2020), to our knowledge, an airborne N2O lidar has not been realized yet, nor has passive remote sensing by means of spectroscopy been used to measure N2O concentrations in the lower troposphere.
Airborne N2O lidar remote sensing has the potential to combine the advantages of high measurement accuracy, large area coverage, and dawn/dusk or nighttime measurement capability to study diurnal concentration variations. Initial studies have shown that integrated-path differential absorption (IPDA) lidar from an airborne or even a satellite platform has the potential to measure N2O with high precision and low bias (Ehret and Kiemle, 2005; Ehret et al., 2008). It uses the Earth surface backscatter signal at an “online” laser wavelength tuned to an N2O absorption line to obtain column concentrations of N2O (Ehret et al., 2008; Amediek et al., 2017). A parallel reference measurement at the non-absorbed “offline” wavelength avoids biases generated by albedo variations or aerosol layers within the light path. In comparison to conventional lidar using backscatter from atmospheric molecules and aerosol, IPDA lidar yields high signal-to-noise ratio at comparatively low instrument size since the surface backscatter is about 100 times stronger than the atmospheric backscatter in terms of laser energy per range gate. Still, accurate ranging by means of short laser pulses is important for precise measurements of the individual column length. First airborne systems for CO2 and CH4 have demonstrated high measurement accuracy and the capability to measure in broken-cloud environments (Amediek et al., 2017; Sun et al., 2021; Barton-Grimley et al., 2022; Mao et al., 2024).
The objective to quantify agricultural areal or point-source emissions requires obtaining N2O column concentration gradients along the flight track between background levels outside the emission regions and the N2O source regions; cultivated soils; or exhaust plumes from, e.g., fertilizer production sites. The airborne lidar should point downward from a flight altitude of about 5 km, well above the boundary layer in which N2O surface emissions disperse vertically by turbulence. Gradients over agricultural regions measured by airborne in situ instruments (Eckl et al., 2021; Waldmann et al., 2024) suggest that the maximum uncertainty of the N2O column measurement should be 0.5 % and that an along-track horizontal measurement resolution of 500 m is sufficient. Consequently, the measurement instability due to instrumental drifts or changing biases should remain below 0.5 %. Experience from airborne lidar campaigns shows that long-term stability can be controlled by executing repeated flight legs over the same tracks, as well as over background concentrations in the case that those can be assumed constant. To detect smaller but denser N2O emission plumes from industrial production sites, the horizontal resolution can be improved at the cost of precision. Lidar allows such tradeoffs to adapt to the measurement objectives.
This study first investigates the N2O spectroscopy to find suitable absorption lines. The chosen wavelength has consequences for the surface reflectance, the atmospheric absorption, the solar and thermal background radiation, and transmitter and detector options. All relevant environment, instrument, and spectroscopic constraints are implemented in a lidar simulation model to design the instrument in order to meet the above measurement requirements. Finally, although beyond the scope of this study, concepts for suitable lidar transmitter and detector technologies are briefly discussed.
Up-to-date spectroscopic data retrieved with the HITRAN (high-resolution transmission molecular absorption database) Application Programming Interface (HAPI) are used to find suitable absorption lines in the four major rotational–vibrational N2O bands located at 2.9, 3.9, 4.5, and 7.8 µm (Nemtchinov et al., 2004; Loos et al., 2015; Kochanov et al., 2016; Gordon et al., 2022). Molecular absorption cross sections are calculated line by line with a resolution of 0.001 cm−1 with the Hartmann–Tran scheme (Ngo et al., 2013) using standard atmosphere profiles of pressure, temperature, and trace gas concentrations within the lowest 5 km, below the foreseen flight altitude. The vertically integrated product of the absorption cross section σ and the trace gas molecule number density n, both varying with altitude z between the surface sfc and the flight height flh, is the optical depth od:
It is related to atmospheric transmission and represents the spectroscopic determining parameter for IPDA lidar column measurements. The IPDA technique is well described in, e.g., Ehret et al. (2008) and Amediek et al. (2017). Criteria for line selection are (a) trace gas molecule number density; (b) appropriate line strength, related to the optimal optical depth; (c) low temperature sensitivity; and (d) minimal influence by other gases. The optimal line strength or optical depth is a compromise between absorption that is too weak and saturation. The optimal optical depth typically lies between 0.5 and 1.2 and depends on column height and instrument noise (Ehret et al., 2008). Temperature sensitivity is determined using an atmospheric temperature profile shifted by 1 K and evaluating the difference in optical depth between the reference and the temperature-shifted optical depth.
The N2O bands at 2.9 and 3.9 µm contain absorption lines of comparable strength, yet the entire 2.9 µm N2O band is dominated by water vapor absorption lines whose wings are without exception stronger than the N2O lines within the lowest 5 km; hence the 2.9 µm band is not shown here. The 3.9 µm N2O band is illustrated in Fig. 1 showing the one-way column optical depth of the lowermost 5 km as a function of vacuum wavenumber and wavelength. The 3.9 µm band is composed of relatively weak lines suitable for satellite lidar (Ehret and Kiemle, 2005; Ehret et al., 2008) yet suboptimal for lower-tropospheric-concentration measurements. One of the strongest absorption lines, situated at 2576.54 cm−1, is selected here (red line), characterized by both the low influence of other absorbing gases and low temperature sensitivity. The offline reference wavenumber is set to a neighboring minimum absorption and temperature sensitivity position.
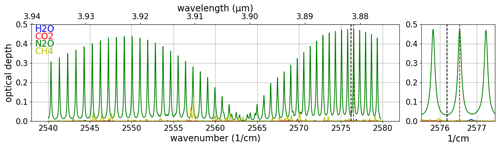
Figure 1Optical depth spectrum of trace gases in the 3.9 µm N2O band in a standard atmosphere vertical column covering the lowest 5 km. The selected online (offline) position is highlighted in red (black) and within a close-up (right). Absorption lines of N2O (green), water (blue), CO2 (red), and CH4 (yellow) need to be considered in this spectral region.
The 4.5 µm band contains much stronger N2O lines (Fig. 2). N2O lines with suitable strength exist at both edges of the band, around 2180 and 2255 cm−1, as well as in the center of the band. However, all of those are inappropriate due to overlapping water lines and high temperature sensitivity in the center of the band. Finally, Fig. 3 illustrates the 7.8 µm band which is so densely populated with strong water and methane lines that no suitable N2O line candidate is found. Water vapor is difficult to correct due to its high variability in the lower troposphere. Consequently, we restrict this study to the 3.9 and 4.5 µm N2O bands. In the 4.5 µm band, trough positions at minimum optical depth in between two strong lines can be selected for both on- and offline, as used for CH4 lidar and foreseen for MERLIN, the Remote Sensing Methane Lidar Mission (Kiemle et al., 2011; Amediek et al., 2017). A trough position has two advantages. First, it relaxes the laser frequency stability requirement due to a relatively flat optical depth in the center of the trough. More quantitatively, the derivative of optical depth with respect to wavenumber around the minimum of the trough is a factor 50 to 100 lower than outside the trough in the steep flank of a line (Kiemle et al., 2011). Second, the measurement sensitivity at low altitudes, i.e., near the surface where the N2O sources are located, is improved thanks to the pressure broadening of both lines surrounding the trough (Ehret et al., 2008). Figure 4 illustrates the differences in sensitivity between line center, wing, and trough (i.e., far wing) positions. Within the lowest 1 km a spectral position in the center of the trough is found to increase the near-surface sensitivity to N2O by about a factor of 1.5 (= 1.4 % 0.92 %) in comparison to a line center position.
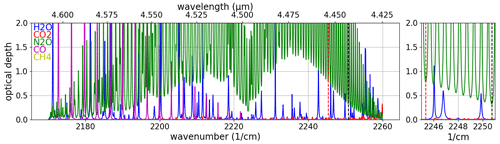
Figure 2Optical depth spectrum of trace gases in the 4.5 µm N2O band in a standard atmosphere vertical column covering the lowest 5 km. The selected online (offline) position is highlighted in red (black) and within a close-up (right). Absorption lines of CO have to be considered in addition (magenta).
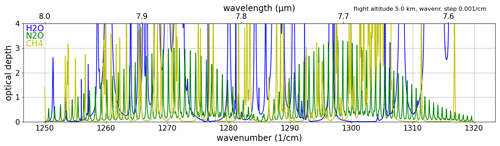
Figure 3Optical depth spectrum of trace gases in the 7.8 µm N2O band, as in Figs. 1 and 2. The atmospheric window ends at 1310 cm−1 where a large water vapor absorption band begins.
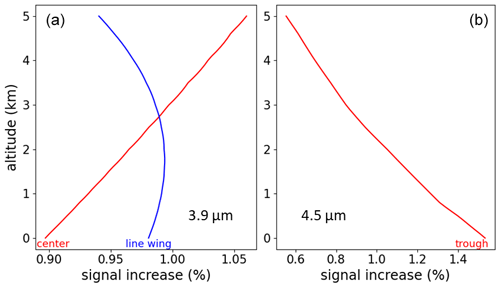
Figure 4N2O measurement sensitivity expressed as online optical depth (signal) increase under the assumption of a hypothetical 50 % N2O concentration increase within a 100 m thick layer, as a function of the altitude of this layer. (a) 3.9 µm line center (red) position of Fig. 1 in comparison with an online line wing (blue) position at 2576.57 cm−1 (very close to the line center; not further discussed). (b) 4.5 µm online trough position of Fig. 2.
Therefore, in the 4.5 µm band we select a trough position at the high-wavenumber side of the band at 2245.35 cm−1 because it is less influenced by other gases than the low-wavenumber side (Fig. 2). The closest possible offline position is at 2250.75 cm−1. The 10.7 nm distance from the online position may require separate lasers for the generation of the on- and offline wavelengths and may lead to uncertainties estimated to < 1 % if surface albedo or aerosol extinction are wavelength-dependent (Amediek et al., 2009). Table 1 summarizes the spectroscopic characteristics of the candidate lines in both bands. The temperature sensitivity is sufficiently low, especially at the online positions. The additional optical depth by line wings of other gases is insignificant at 3.9 µm and ∼ 0.01 at 4.5 µm. In the event of a 50 % concentration change possible for H2O yet very unlikely for CO2, the impact on the total optical depth at 4.5 µm would be 0.005 0.79 ≈ 0.6 % for the online and 0.005 0.34 ≈ 1.5 % for the offline, which we consider uncritical. Note that our line selection is provisional as new spectroscopic data may lead to better options.
Table 1Selected online and offline spectral positions in the 3.9 and 4.5 µm N2O absorption bands with corresponding N2O optical depth for a vertical standard atmosphere column in the lowest 5 km, relative N2O optical depth change per Kelvin temperature change, and optical depth of other trace gases.
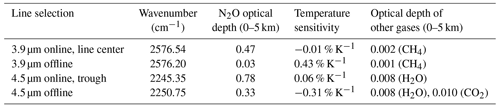
The albedo is key for IPDA lidar, which relies on surface backscatter intensity. It is generally low in the IR, compared to the near-IR and visible spectral ranges. Ehret and Kiemle (2005) and Ehret et al. (2008) used a value of 0.02 (2 %) for land surfaces. The Ecosystem Spaceborne Thermal Radiometer Experiment on Space Station (ECOSTRESS) spectral library contains a limited set of mid-IR reflectance data (Baldridge et al., 2009; Meerdink et al., 2019). Table 2 lists their agriculturally relevant values. We keep the small value of 2 % from our initial studies for the present simulation, which can be considered a safe worst case. Over agricultural soils, extinction by rural aerosol within the boundary layer is expected. Based on IR lidar measurements by Vaughan et al. (1995, 1998) and scaled to 3.9 and 4.5 µm using an Angström exponent of 1, we assume a worst-case maximum aerosol optical depth of 0.2 across the lowest 5 km. The Rayleigh optical depth due to air molecule extinction amounts to ∼ at around 4 µm in the lowest 5 km and is therefore negligible.
Table 2Mid-IR albedo, aerosol optical depth, and background radiation used for the N2O lidar simulation. The minimum albedo of 0.02 (bold) is selected as a worst case for the simulation. Soil has about 10 times higher albedo than grain or grass. The differential absorption optical depth is about equal in both N2O bands.
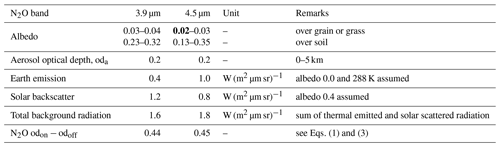
Photons from solar scattered and thermal emitted radiation cause noisy background signals in the detector. The mid-IR radiation emitted from Earth's surface is calculated with Planck's law (Stull, 2017) assuming a blackbody with albedo zero at 288 K temperature and without atmosphere. According to Kirchhoff's law this represents the worst-case maximum thermal emission, also because the atmosphere is mostly colder than Earth's surface. The solar radiation is calculated with Planck's law assuming an albedo of 0.4, a sun in the zenith, and no atmosphere, which also represents a maximum-radiation worst case. Table 2 summarizes the environmental boundary conditions for the lidar simulation. The low total radiation, close to the spectral minimum of the sum of thermal emitted and solar scattered radiation, represents a challenge for passive remote sensing in the mid-IR.
The airborne lidar performance is assessed by implementing the measurement requirements together with environmental, instrumental, and spectroscopic constraints in a lidar noise propagation model developed for earlier studies (Kiemle et al., 2011, 2017). Atmospheric transmission and surface scattering are evaluated to compute the backscattered signal power as a function of emitted laser power PL, surface reflectance ρ (= albedo π), receiver optical efficiency η, telescope area A, and range R (= 5 km) for the on- and offline wavelengths in the IPDA lidar equation:
According to the Beer–Lambert law the exponential term represents the atmospheric transmission along the vertical path, lowered by the aerosol optical depth oda and the N2O on- and offline optical depths. Solving Eq. (2) for the respective on- and offline optical depths and assuming constant surface albedo, optical efficiency, and aerosol optical depth for both on- and offline wavelengths, we obtain the differential absorption optical depth DAOD by subtracting the offline solution of Eq. (2) from the online solution:
where EL_on,off are the received on- and offline laser pulse energies, and Eref_on,off are energy reference measurements accounting for variations in the emitted pulse power PL. After Eq. (1) the DAOD is proportional to the N2O column concentration weighted with the absorption cross section. Table 2 lists the DAOD values expected in a reference atmosphere.
Table 3 lists the major instrument parameters. The larger the average laser power, surface reflectance (albedo), and telescope size, the stronger the received signal power and consequently the signal-to-noise ratio (SNR). Further parameters influencing the SNR are the flight altitude, the horizontal resolution, the laser pulse repetition rate, the receiver field of view and several detector parameters. Parametric analyses allow us to fine tune the instrument with the aim of optimizing efficiency or flexibility. Parameters can depend on each other, such as the aircraft velocity, the horizontal resolution, the repetition rate, and the number of averaged pulses. Likewise, for the laser, repetition rate, pulse energy, and average laser power are related. Finally, the telescope field of view is related to its diameter, its focal ratio, and the detector size. Equations implement all relevant dependencies in the model. For more details we refer the reader to our former studies (Ehret et al., 2008; Kiemle et al., 2011, 2017).
Table 3N2O lidar instrument parameters assumed for the simulation, partly adopted from earlier studies and regarded as achievable. Parameters of first importance to the performance are in bold.
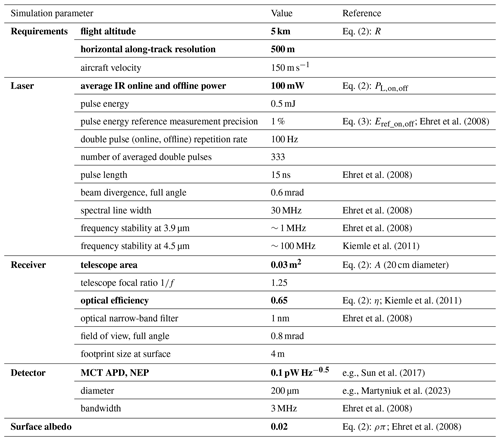
The simulation is run with the environmental conditions of Table 2 and the instrument parameters of Table 3. All noise contributors – Poisson noise from laser photons and background radiation, detector noise, laser speckle noise within the field of view, and energy reference measurement noise – are considered. Speckle noise can be more significant for laser measurements in the mid-IR since the speckle cell sizes are larger and there are fewer “speckles” (regions of constructive interference) on the detector surface. This effect is compensated for by a larger telescope field of view and laser beam divergence, compared to near-IR applications. As all noise sources are basically uncorrelated, error propagation on the basis of Eqs. (2) and (3) provides the overall 1σ precision of the N2O measurements as a function of the prescribed online optical depth for both N2O bands (Fig. 5). The offline optical depth is kept at a constant level of 0.03 (0.33) at 3.9 (4.5) µm. At 3.9 µm this level corresponds to a representative minimum optical depth within the band (Fig. 1), while at 4.5 µm the offline from Table 1 may serve several neighboring online trough options (Fig. 2). The dashed lines show the performance of an ideal noise-free detector for comparison. While the detector noise is assumed zero, all other noise contributors remain: Poisson and shot noise, speckle noise, and energy measurement noise. Their minima are right-shifted because the optimum online optical depth is larger under low-noise conditions (Ehret et al., 2008). The curves are flatter since a low-noise instrument is more tolerant with respect to suboptimal spectroscopic settings.
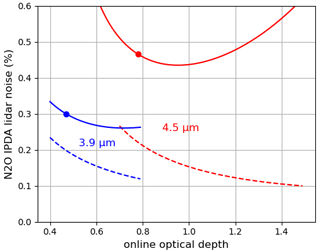
Figure 5Simulated N2O lidar measurement precision at 3.9 (blue) and 4.5 µm (red) as a function of online optical depth under the conditions listed in Tables 2 and 3. The dots indicate the selections of Table 1, and the dashed lines represent an ideal noise-free detector for comparison. The absence of strong lines in the 3.9 µm band limits the 3.9 µm online optical depth range.
Assuming, as a provisional proxy, a mercury–cadmium–telluride (MCT) avalanche photodiode detector (APD) with a noise-equivalent power (NEP) of 0.1 pW Hz, the optimum online optical depth for minimum noise is around 0.7 (0.9) for 3.9 (4.5) µm. Due to the lack of more comprehensive mid-IR detector data, the NEP is estimated on the basis of near-IR realizations and mid-IR prototypes (Sun et al., 2017; Martyniuk et al., 2023) using large security margins, likely representing a worst case. The absence of strong lines in the 3.9 µm band (Fig. 1) prevents an optimum online setting, yet the low offline optical depth of 0.03 provides satisfying low noise levels (Fig. 5). At 4.5 µm, a trough near the optimum optical depth could be selected (Fig. 2). Stronger neighboring troughs that would fit the optimum suffer from overlapping water line wings and larger temperature sensitivity. The 4.5 µm selection also allows for a measurement precision fulfilling the initial requirement of 0.5 %, although at a higher noise level, primarily due to the relatively high offline optical depth of 0.33. Away from the optimum, noise increases towards lower optical depths because of a smaller DAOD (Eq. 3) and towards higher optical depths because of online signal attenuation.
In addition to uncorrelated random noise which averages out over longer data accumulation lengths, persisting systematic uncertainties (biases) may arise from errors in the spectroscopic parameters. The HITRAN database specifies the N2O line intensity uncertainties to within 2 % and 5 %. This does not threaten the objective to quantify agricultural areal or point-source emissions since those are derived from relative column gradients between sources and background rather than from absolute measurements. In addition, spectroscopy errors can be corrected by comparing the lidar columns against collocated profiles from high-accuracy in situ aircraft sensors (Amediek et al., 2017; Mao et al., 2024). Biases may also arise from variability in the actual aerosol, temperature, and pressure profiles within the columns. Experience and simulation (Ehret et al., 2008; Kiemle et al., 2011; Amediek et al., 2017) show that these usually remain below 1 %. Finally, albedo variations cause measurement uncertainties if the on- and offline surface laser spots are not fully overlapping, which is generally the case. Those, however, tend to behave like random deviations, leading to slightly increased noise levels (Amediek et al., 2009).
IR lidar transmitters that can be considered for the described purpose are (a) tunable solid-state lasers such as transition-metal (TM)-doped II-VI chalcogenide lasers, for example Fe:ZnS or Fe:ZnSe lasers; (b) optical parametric oscillators (OPOs) and amplifiers; and (c) laser sources based on nonlinear difference frequency generation (DFG) or Raman shifting. Comprehensive overviews on recent advances in those laser-based mid-infrared sources are given in Vodopyanov (2020) and Ren et al. (2021). Using OPOs, wavelengths in the 3.9 to 4.5 µm range are readily accessible. When pumping with the ubiquitous Nd:YAG laser at 1.064 µm, the corresponding signal wavelengths that lead to an idler wavelength at 3.9 or 4.5 µm are ∼ 1.46 or 1.39 µm, respectively. This requires suitable nonlinear crystals with transparency at all these wavelengths. But other pump laser sources at longer wavelength such as 2.05 µm (e.g., Medina et al., 2021), cascaded OPOs, or DFG sources are also options (e.g., DFG of 1.064 µm with 1.46 µm results in 3.9 µm and mixing 1.064 µm with 1.39 µm results in 4.5 µm). Using DFG, lidar measurements of species such as hydrocarbons in the wavelength range around 3.4 µm have been performed (Robinson et al., 2011; Gardiner et al., 2017).
Low-noise and high-bandwidth radiation detection is a challenge in the mid-IR. Prototype MCT APDs can achieve very low excess noise in the mid-IR, yet cooling down to at least 200 K is required to reduce dark currents. Sun et al. (2017, 2021) report a detector linear analog output with a dynamic range of 2–3 orders of magnitude at a fixed APD gain for MCT material that has a cut-off at 4.3 µm. These detectors work well at 3.9 µm yet have little response at 4.5 µm. Generally, developments are ongoing, but manufacturability is considered low (Chen et al., 2021; Martyniuk et al., 2023). In addition, the literature reveals that efforts go into the development of imaging sensors, while lidar requires a single sensor with a large photosensitive area in order to satisfy optical constraints (see Table 3). Due to the lack of data, we used in our study a conservative approach for the simulation, with a large security margin for the detector noise on the basis of data from near-IR realizations and mid-IR prototypes. An alternative to MCT is indium antimonide (InSb), yet mid-IR InSb APDs apparently have more noise and less bandwidth (Abautret et al., 2015; Alimi et al., 2020). Besides APDs, up-conversion detectors (UCDs; Hoegstedt et al., 2016; Meng et al., 2018) or superconducting nanowire single-photon detectors (SNSPDs) could offer high-efficiency, low-noise signal detection, yet SNSPDs require operating temperatures below 3 K, which is challenging on board aircraft. A single-photon response was reported up to 25 K at 1.5 µm (Charaev et al., 2023), unfortunately not much less challenging. Also, in this area, developments are underway.
Concerning methodical alternatives for active remote sensing, the more common differential absorption lidar (DIAL) technique exploits atmospheric instead of surface backscatter with the advantage of providing vertical profiles of trace gases instead of column concentrations. A dedicated simulation using the same lidar simulator shows that this is at the expense of a 100-times-larger laser power and telescope aperture product (PL⋅A = 0.3 W m2 instead of 0.003 W m2 from Table 3) to compensate for the roughly 100-times-weaker atmospheric backscatter signals (in terms of laser photons per range gate), even within a boundary layer with rural aerosol. A low-power alternative is IPDA or DIAL with heterodyne instead of direct signal detection. It requires a diffraction-limited optical setup and laser pulse repetition rates in the kilohertz range to manage speckle-induced noise. So far, ground-based profiling systems (Koch et al., 2008; Yu et al., 2024) and an airborne realization (Spiers et al., 2011; Jacob et al., 2019) for CO2 have been reported, yet only in the near-IR. Another low-power option for IPDA is (modulated) continuous-wave (cw) laser operation instead of emitting short pulsed signals (e.g., Campbell et al., 2020). For measurements with a precision requirement below 1 %, however, the length of the atmospheric column must be known to an accuracy of better than 3 m, which is only practicable with short laser pulses in combination with a sufficiently large detection bandwidth (Table 3; Ehret et al., 2008). Alternatively, a precision range finder had to be added, which annihilates the cost benefit of cw lidar. We conclude that either methodical alternative for N2O active remote sensing is too expensive or its maturity for airborne operation lags behind that of direct-detection pulsed IPDA.
With a resulting noise level of < 0.5 % an airborne IPDA lidar provides important new capabilities for N2O regional gradient or hot-spot detection with technically realizable and affordable transmitter (100 mW average laser power) and receiver (20 cm telescope, MCT APD) characteristics. Using an MCT APD that requires cooling to 200 K the system could fit into a small to mid-size research aircraft. The simulation results show better performance at 3.9 µm in terms of the noise level. On the other hand, the trough position at 4.5 µm yields higher measurement sensitivity at low altitudes and considerably relaxes the laser frequency stability requirement. Which option is finally preferred will depend on many factors including several aspects of laser technology such as availability, complexity, linewidth, frequency locking performance, detector availability, costs, and aircraft suitability. The simulation tool will be applied to trade off various instrument options as technology is developed. Better low-noise IR detectors will be particularly beneficial. While a satellite implementation is not impossible but still far away because of the as-yet-undeveloped space-proof technology, the development of an airborne N2O lidar at 3.9 or 4.5 µm is recommended given the technical feasibility and the scientific and societal need.
Code and data can be provided by the corresponding author on request.
CK developed the model code, performed the simulations, and prepared the manuscript with contributions from all co-authors. AF, CF, GE, and MW contributed information on available laser and detector technology.
At least one of the (co-)authors is a member of the editorial board of Atmospheric Measurement Techniques. The peer-review process was guided by an independent editor, and the authors also have no other competing interests to declare.
Publisher’s note: Copernicus Publications remains neutral with regard to jurisdictional claims made in the text, published maps, institutional affiliations, or any other geographical representation in this paper. While Copernicus Publications makes every effort to include appropriate place names, the final responsibility lies with the authors.
The article processing charges for this open-access publication were covered by the German Aerospace Center (DLR).
This paper was edited by Thomas F. Hanisco and reviewed by two anonymous referees.
Abautret, J., Perez, J. P., Evirgen, A., Rothman, J., Cordat, A., and Christol, P.: Characterization of midwave infrared InSb avalanche photodiode, J. Appl. Phys., 117, 244502, https://doi.org/10.1063/1.4922977, 2015.
Alimi, Y., Pusino, V., Steer, M. J., and Cumming, D. R. S.: InSb Avalanche Photodiodes on GaAs Substrates for Mid-Infrared Detection, IEEE T. Electron Dev., 67, 179–184, https://doi.org/10.1109/TED.2019.2956283, 2020.
Amediek, A., Fix, A., Ehret, G., Caron, J., and Durand, Y.: Airborne lidar reflectance measurements at 1.57 µm in support of the A-SCOPE mission for atmospheric CO2, Atmos. Meas. Tech., 2, 755–772, https://doi.org/10.5194/amt-2-755-2009, 2009.
Amediek, A., Ehret, G., Fix, A., Wirth, M., Büdenbender, C., Quatrevalet, M., Kiemle, C., and Gerbig, C.: CHARM-F – a new airborne integrated-path differential-absorption lidar for carbon dioxide and methane observations: measurement performance and quantification of strong point source emissions, Appl. Optics, 56, 5182–5197, https://doi.org/10.1364/AO.56.005182, 2017.
Arias, P. A., Bellouin, N., Coppola, E., Jones, R. G., Krinner, G., Marotzke, J., Naik, V., Palmer, M. D., Plattner, G.-K., Rogelj, J., Rojas, M., Sillmann, J., Storelvmo, T., Thorne, P. W., and Trewin, B.: Technical Summary, in: Climate Change 2021: The Physical Science Basis. Contribution of Working Group I to the Sixth Assessment Report of the Intergovernmental Panel on Climate Change, Cambridge University Press, Cambridge, United Kingdom and New York, USA, 33–144, https://doi.org/10.1017/9781009157896.002, 2021.
Baldridge, A. M., Hook, S. J., Grove, C. I. and Rivera, G.: The ASTER Spectral Library Version 2.0, Remote Sens. Environ., 113, 711–715, 2009.
Barton-Grimley, R. A., Nehrir, A. R., Kooi, S. A., Collins, J. E., Harper, D. B., Notari, A., Lee, J., DiGangi, J. P., Choi, Y., and Davis, K. J.: Evaluation of the High Altitude Lidar Observatory (HALO) methane retrievals during the summer 2019 ACT-America campaign, Atmos. Meas. Tech., 15, 4623–4650, https://doi.org/10.5194/amt-15-4623-2022, 2022.
Campbell, J. F., Lin, B., Dobler, J., Pal, S., Davis, K., Obland, M. D., Erxleben, W., McGregor, D., O'Dell, C., Bell, E., Weir, B., Fan, T.-F., Kooi, S., Gordon, I., Corbett, A., and Kochanov, R.: Field evaluation of column CO2 retrievals from intensity-modulated continuous-wave differential absorption lidar measurements during the ACT-America campaign, Earth and Space Science, 7, e2019EA000847, https://doi.org/10.1029/2019EA000847, 2020.
Charaev, I., Bandurin, D. A., Bollinger, A. T., Phinney, I. Y., Drozdov, I., Colangelo, M., Butters, B. A., Taniguchi, T., Watanabe, K., He, X., Medeiros, O., Božović, I., Jarillo-Herrero, P., and Berggren, K. K.: Single-photon detection using high-temperature superconductors, Nat. Nanotechnol., 18, 343–349, 2023.
Chen, J., Chen, J., Li, X., He, J., Yang, L., Wang, J., Yu, F., Zhao, Z., Shen, C., Guo, H., Li, G., Chen, X., and Lu, W. High-performance HgCdTe avalanche photodetector enabled with suppression of band-to-band tunneling effect in mid-wavelength infrared, npj Quantum Materials, 6, 103, https://doi.org/10.1038/s41535-021-00409-3, 2021.
Eckl, M., Roiger, A., Kostinek, J., Fiehn, A., Huntrieser, H., Knote, C., Barkley, Z. R., Ogle, S., Baier, B. C. Sweeney, C., and Davis, K. J.: Quantifying nitrous oxide emissions in the U.S. Midwest: A top-down study using high resolution airborne in situ observations, Geophys. Res. Lett., 48, e2020GL091266, https://doi.org/10.1029/2020GL091266, 2021.
Ehret, G. and Kiemle, C.: Requirements Definition for Future DIAL Instruments, ESA Study Final Report, ESA-CR(P)-4513, ESA Contract no. 10880/03/NL/FF, 2005.
Ehret, G., Kiemle, C., Wirth, M., Amediek, A., Fix, A., and Houweling, S.: Space-borne remote sensing of CO2, CH4, and N2O by integrated path differential absorption lidar: a sensitivity analysis, Appl. Phys. B, 90, 593–608, 2008.
Gardiner, T., Helmore, J., Innocenti, F., and Robinson, R.: Field Validation of Remote Sensing Methane Emission Measurements, Remote Sens.-Basel, 9, 956, https://doi.org/10.3390/rs9090956, 2017.
Gong, Y., Bu, L., Yang, B., and Mustafa, F.: High Repetition Rate Mid-Infrared Differential Absorption Lidar for Atmospheric Pollution Detection, Sensors-Basel, 20, 2211, https://doi.org/10.3390/s20082211, 2020.
Gordon, I. E., Rothman, L. S., Hargreaves, R. J., et al.: The HITRAN2020 molecular spectroscopic database, J. Quant. Spectrosc. Ra., 277, 107949, https://doi.org/10.1016/j.jqsrt.2021.107949, 2022.
Hoegstedt, L., Fix, A., Wirth, M., Pedersen, C., and Tidemand-Lichtenberg, P.: Upconversion-based lidar measurements of atmospheric CO2, Opt. Express, 24, 5152–5161, https://doi.org/10.1364/OE.24.005152, 2016.
Jacob, J. C., Menzies, R. T. and Spiers G. D.: Data Processing and Analysis Approach to Retrieve Carbon Dioxide Weighted-Column Mixing Ratio and 2-μm Reflectance With an Airborne Laser Absorption Spectrometer, IEEE T. Geosci. Remote, 57, 958–971, https://doi.org/10.1109/TGRS.2018.2863711, 2019.
Kiemle, C., Quatrevalet, M., Ehret, G., Amediek, A., Fix, A., and Wirth, M.: Sensitivity studies for a space-based methane lidar mission, Atmos. Meas. Tech., 4, 2195–2211, https://doi.org/10.5194/amt-4-2195-2011, 2011.
Kiemle, C., Ehret, G., Amediek, A., Fix, A., Quatrevalet, M., and Wirth, M.: Potential of Spaceborne Lidar Measurements of Carbon Dioxide and Methane Emissions from Strong Point Sources, Remote Sens.-Basel, 9, 1137, https://doi.org/10.3390/rs9111137, 2017.
Koch, G. J., Beyon, J. Y., Gibert, F., Barnes, B. W., Ismail, S., Petros, M., Petzar, P. J., Yu, J., Modlin, E. A., Davis, K. J., and Singh, U. N.: Side-line tunable laser transmitter for differential absorption lidar measurements of CO2: design and application to atmospheric measurements, Appl. Optics, 47, 944–956, 2008.
Kochanov, R. V., Gordon, I. E., Rothman, L. S., Wcislo, P., Hill, C., and Wilzewski, J. S.: HITRAN Application Programming Interface (HAPI): A comprehensive approach to working with spectroscopic data, J. Quant. Spectrosc. Ra., 177, 15–30, 2016.
Loos, J., Birk, M., and Wagner, G.: Pressure broadening, -shift, speed dependence and line mixing in the ν3 rovibrational band of N2O, J. Quant. Spectrosc. Ra., 151, 300–309, 2015.
Mao, J., Abshire, J. B., Kawa, S. R., Sun, X., and Riris, H.: Airborne lidar measurements of atmospheric CO2 column concentrations to cloud tops made during the 2017 ASCENDS/ABoVE campaign, Atmos. Meas. Tech., 17, 1061–1074, https://doi.org/10.5194/amt-17-1061-2024, 2024.
Martyniuk, P., Wang, P., Rogalski, A., Gu, Y., Jiang, R., Wang, F., and Hu, W.: Infrared avalanche photodiodes from bulk to 2D materials, Light: Science & Applications, 12, 212, https://doi.org/10.1038/s41377-023-01259-3, 2023.
Meng, L., Fix, A., Wirth, M., Hoegstedt, L., Tidemand-Lichtenberg, P., Pedersen, C., and Rodrigo, P. J.: Upconversion detector for range-resolved DIAL measurement of atmospheric CH4, Opt. Express, 26, 3850-3860, 2018.
Meerdink, S. K., Hook, S. J., Roberts, D. A., and Abbott, E. A.: The ECOSTRESS spectral library version 1.0, Remote Sens. Environ., 230, 111196, https://doi.org/10.1016/j.rse.2019.05.015, 2019.
Medina, M. A., Piotrowski, M., Schellhorn, M., Wagner, F. R., Berrou, A., and Hildenbrand-Dhollande, A.: Beam quality and efficiency of ns-pulsed high-power mid-IR ZGP OPOs compared in linear and non-planar ring resonators, Opt. Express, 29, 21727–21737, https://doi.org/10.1364/OE.430717, 2021.
Nemtchinov, V., Sun, C., and Varanasi, P.: Measurements of Line Intensities and Line Widths in the ν3-fundamental Band of Nitrous Oxide at Atmospheric Temperatures, J. Quant. Spectrosc. Ra., 83, 267–284, 2004.
Ngo, N. H., Lisak, D., Tran, H., and Hartmann, J.-M.: An isolated line-shape model to go beyond the Voigt profile in spectroscopic databases and radiative transfer codes, J. Quant. Spectrosc. Ra., 129, 89–100, https://doi.org/10.1016/j.jqsrt.2013.05.034, 2013.
Ren, T., Wu, C., Yu, Y., Dai, T., Chen, F., and Pan, Q.: Development Progress of 3–5 µm Mid-Infrared Lasers: OPO, Solid-State and Fiber Laser, Appl. Sci., 11, 11451, https://doi.org/10.3390/app112311451, 2021.
Ricaud, P., Attié, J.-L., Chalinel, R., Pasternak, F., Léonard, J., Pison, I., Pattey, E., Thompson, R. L., Zelinger, Z., Lelieveld, J., Sciare, J., Saitoh, N., Warner, J., Fortems-Cheiney, A., Reynal, H., Vidot, J., Brooker, L., Berdeu, L., Saint-Pé, O., Patra, P. K., Dostál, M., Suchánek, J., Nevrlý, V., and Zwaaftink, C. G.: The Monitoring Nitrous Oxide Sources (MIN2OS) satellite project, Remote Sens. Environ., 266, 112688, https://doi.org/10.1016/j.rse.2021.112688, 2021.
Robinson, R., Gardiner, T., Innocenti, F., Woods, P., and Coleman, M.: Infrared differential absorption Lidar (DIAL) measurements of hydrocarbon emissions, J. Environ. Monitor., 13, 2213–2220, https://doi.org/10.1039/C0EM00312C, 2011.
Spiers, G. D., Menzies, R. T., Jacob, J., Christensen, L. E., Phillips, M. W., Choi, Y., and Browell, E. V.: Atmospheric CO2 measurements with a 2 µm airborne laser absorption spectrometer employing coherent detection, Appl. Optics, 50, 2098–2111, https://doi.org/10.1364/AO.50.002098, 2011.
Stull, R.: Practical Meteorology: An Algebra-based Survey of Atmospheric Science, version 1.02b, Univ. of British Columbia, 940 pp., ISBN 978-0-88865-283-6, 2017.
Sun, X., Abshire, J. B., Beck, J. D., Mitra, P., Reiff, K., and Yang, G.: HgCdTe avalanche photodiode detectors for airborne and spaceborne lidar at infrared wavelengths, Opt. Express, 25, 16589–16602, https://doi.org/10.1364/OE.25.016589, 2017.
Sun, X., Abshire, J. B., Ramanathan, A., Kawa, S. R., and Mao, J.: Retrieval algorithm for the column CO2 mixing ratio from pulsed multi-wavelength lidar measurements, Atmos. Meas. Tech., 14, 3909–3922, https://doi.org/10.5194/amt-14-3909-2021, 2021.
Tian, H., Pan, N., Thompson, R. L., Canadell, J. G., Suntharalingam, P., Regnier, P., Davidson, E. A., Prather, M., Ciais, P., Muntean, M., Pan, S., Winiwarter, W., Zaehle, S., Zhou, F., Jackson, R. B., Bange, H. W., Berthet, S., Bian, Z., Bianchi, D., Bouwman, A. F., Buitenhuis, E. T., Dutton, G., Hu, M., Ito, A., Jain, A. K., Jeltsch-Thömmes, A., Joos, F., Kou-Giesbrecht, S., Krummel, P. B., Lan, X., Landolfi, A., Lauerwald, R., Li, Y., Lu, C., Maavara, T., Manizza, M., Millet, D. B., Mühle, J., Patra, P. K., Peters, G. P., Qin, X., Raymond, P., Resplandy, L., Rosentreter, J. A., Shi, H., Sun, Q., Tonina, D., Tubiello, F. N., van der Werf, G. R., Vuichard, N., Wang, J., Wells, K. C., Western, L. M., Wilson, C., Yang, J., Yao, Y., You, Y., and Zhu, Q.: Global nitrous oxide budget (1980–2020), Earth Syst. Sci. Data, 16, 2543–2604, https://doi.org/10.5194/essd-16-2543-2024, 2024.
Vandenbussche, S., Langerock, B., Vigouroux, C., Buschmann, M., Deutscher, N. M., Feist, D. G., García, O., Hannigan, J. W., Hase, F., Kivi, R., Kumps, N., Makarova, M., Millet, D. B., Morino, I., Nagahama, T., Notholt, J., Ohyama, H., Ortega, I., Petri, C., Rettinger, M., Schneider, M., Servais, C. P., Sha, M. K., Shiomi, K., Smale, D., Strong, K., Sussmann, R., Té, Y., Velazco, V. A., Vrekoussis, M., Warneke, T., Wells, K. C., Wunch, D., Zhou, M., and De Mazière, M.: Nitrous Oxide Profiling from Infrared Radiances (NOPIR): Algorithm Description, Application to 10 Years of IASI Observations and Quality Assessment, Remote Sens.-Basel, 14, 1810. https://doi.org/10.3390/rs14081810, 2022.
Vaughan, J. M., Brown, D. W., Nash, C., Alejandro, S. B., and Koenig, G. G.: Atlantic Atmospheric Aerosol Studies: 2. Compendium of Airborne Backscatter Measurements at 10.6 µm, J. Geophys. Res.-Atmos., 100, 1043–1065, https://doi.org/10.1029/94JD01817, 1995.
Vaughan, J. M., Geddes, N. J., Flamant P. H., and Flesia, C.: Establishment of a backscatter coefficient and atmospheric database, DERA Report for ESA Contract no. 12510/97/NL/RE, DERA/EL/ISET/CR980139/1.0, 1998.
Vodopyanov, K. L.: Laser-Based Mid-infrared Sources and Applications, John Wiley & Sons, Incorporated, https://doi.org/10.1002/9781119074557, Print ISBN: 9781118301814, Online ISBN: 9781119074557, 2020.
Waldmann, P., Eckl, M., Gottschaldt, K.-D., Knez, L., Förster, E., Mallaun, C., Röckmann, T., Hutjes, R., Chen, H., Gerbig, C., Galkowski, M., Kiemle, C., and Roiger, A.: Quantifying agricultural CH4 and N2O emissions of the Netherlands using a novel airborne eddy-covariance measurements system: First results of the GHGMon campaign in June 2023, EGU General Assembly 2024, Vienna, Austria, 14–19 Apr 2024, EGU24-19467, https://doi.org/10.5194/egusphere-egu24-19467, 2024.
WMO: Global Greenhouse Gas Watch (G3W), https://wmo.int/activities/global-greenhouse-gas-watch-g3w (last access: 12 November 2024), 2024.
Yu, S., Guo, K., Li, S., Han, H., Zhang, Z., and Xia, H.: Three-dimensional detection of CO2 and wind using a 1.57 µm coherent differential absorption lidar, Opt. Express 32, 21134–21148, https://doi.org/10.1364/OE.523904, 2024.