the Creative Commons Attribution 4.0 License.
the Creative Commons Attribution 4.0 License.
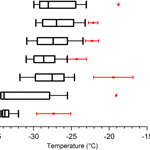
Cleaning up our water: reducing interferences from nonhomogeneous freezing of “pure” water in droplet freezing assays of ice-nucleating particles
Michael Polen
Thomas Brubaker
Joshua Somers
Droplet freezing techniques (DFTs) have been used for half a century to measure the concentration of ice-nucleating particles (INPs) in the atmosphere and determine their freezing properties to understand the effects of INPs on mixed-phase clouds. The ice nucleation community has recently adopted droplet freezing assays as a commonplace experimental approach. These droplet freezing experiments are often limited by contamination that causes nonhomogeneous freezing of the “pure” water used to generate the droplets in the heterogeneous freezing temperature regime that is being measured. Interference from the early freezing of water is often overlooked and not fully reported, or measurements are restricted to analyzing the more ice-active INPs that freeze well above the temperature of the background water. However, this avoidance is not viable for analyzing the freezing behavior of less active INPs in the atmosphere that still have potentially important effects on cold-cloud microphysics. In this work we review a number of recent droplet freezing techniques that show great promise in reducing these interferences, and we report our own extensive series of measurements using similar methodologies. By characterizing the performance of different substrates on which the droplets are placed and of different pure water generation techniques, we recommend best practices to reduce these interferences. We tested different substrates, water sources, droplet matrixes, and droplet sizes to provide deeper insight into what methodologies are best suited for DFTs. Approaches for analyzing droplet freezing temperature spectra and accounting and correcting for the background “pure” water control spectrum are also presented. Finally, we propose experimental and data analysis procedures for future homogeneous and heterogeneous ice nucleation studies to promote a more uniform and reliable methodology that facilitates the ready intercomparison of ice-nucleating particles measured by DFTs.
- Article
(3605 KB) - Full-text XML
-
Supplement
(81 KB) - BibTeX
- EndNote
Pure water experiences extensive supercooling. Water droplets of cloud-relevant diameters (∼10–20 µm) freeze homogeneously at temperatures < −38 ∘C, and this temperature increases with increasing droplet volume (Koop and Murray, 2016; O and Wood, 2016). Freezing between −38 and 0 ∘C requires a catalyst, which in the atmosphere is provided by rare ice-nucleating particles (INPs). Most precipitation over land is triggered through the ice phase (Mülmenstädt et al., 2015), and INPs may have large impacts on cold-cloud microphysics, optical properties, lifetime, and structure (Creamean et al., 2013; DeMott et al., 2010; Lohmann and Feichter, 2005; Vergara-Temprado et al., 2018b; Yin et al., 2002).
Droplet freezing techniques (DFTs) have been utilized for decades to assess the homogeneous freezing of pure water, and the immersion freezing properties of INPs immersed in the droplets (Bigg, 1953; Murray et al., 2012; Vali, 1971, 2014; Wex et al., 2015; Wright and Petters, 2013). In general, these experiments work by depositing droplets containing particles onto a surface which is then cooled down to a low temperature by a cold-plate heat sink (Cziczo et al., 2017). Droplets are then assigned a freezing temperature based on the temperature they were observed to freeze at during the cooling process. These data are compiled to produce a plot of a frozen fraction of droplets versus temperature, referred to as the droplet freezing temperature spectrum. DFTs are utilized for both homogeneous and heterogeneous ice nucleation experiments (Hiranuma et al., 2015; Murray et al., 2010, 2012; Vali and Stansbury, 1966; Wilson et al., 2015; Zobrist et al., 2008). Homogeneous freezing can sometimes present a challenge for DFTs as it is difficult to avoid interference from unintended heterogeneous freezing (Hader et al., 2014; O'Sullivan et al., 2015; Whale et al., 2015). There are a number of variables within DFT setups that can influence the apparent homogeneous freezing temperature of pure water droplets that determines the background temperature spectrum and sets the lower temperature limit for assessing heterogeneous ice nucleation. Water contamination or substrate interferences can also induce freezing well above the homogeneous freezing temperature regime that ensues in the temperature range of −35 to −40 ∘C (Koop and Murray, 2016), restricting the heterogeneous temperature regime accessible by DFTs. Particles and cloud droplets experience a wide range of cloud temperatures and it is important to characterize as much of the heterogeneous ice nucleation temperature spectrum down to −35 ∘C as possible. This requires reducing the influence of water contaminants and substrate effects in DFTs. Recently droplet freezing measurements in the warmer heterogeneous temperature regime > −25 ∘C have been combined with measurements in the colder regime of −20 < T < −35 ∘C by a continuous flow diffusion chamber to characterize the complete heterogeneous ice nucleation temperature spectrum of ambient particles (DeMott et al., 2017). We seek to improve and refine DFTs so that they can independently characterize the complete freezing temperature spectrum.
Nanoscale ice-active surface sites on particles, macromolecules, and other surfaces are thought to control heterogeneous ice nucleation by helping supercooled water molecules to arrange into an ice embryo, thus reducing the nucleation energy barrier (Gurganus et al., 2014; Koop and Murray, 2016; Marcolli et al., 2007). In DFTs the surface on which the droplets reside is thought to be one of the biggest factors that induces nonhomogeneous freezing behavior, similar to other nucleation and crystallization processes (Diao et al., 2011; Hader et al., 2014). Properties such as the contact angle between the droplets and the surface can be used to attempt to assess the ideality of the surface (Budke and Koop, 2015; Koop et al., 1998; Murray et al., 2010). However, despite a large contact angle, surfaces may have micro- or nanoscale defects that induce ice nucleation. Recent work indicates that cracks, scratches, and other surface defects on surfaces and particles impact heterogeneous freezing (Fitzner et al., 2015; Kiselev et al., 2017; Lo et al., 2017; Varanasi et al., 2010; Wang et al., 2016). In general, these studies have found that defects, especially those with crystalline faces similar to ice, lower the barrier for ice nucleation and enhance ice formation above homogeneous temperatures. Price et al. (2018) reported observing lower freezing temperatures when droplets were placed on a Teflon substrate compared to on a standard silanized hydrophobic glass surface. This provides further support for the important role that substrate choice can have on the freezing temperature spectrum observed in droplet freezing techniques.
Aside from surface induced effects, the environment surrounding the droplets may also influence freezing. Some research groups, including ours, deposit their droplets into an oil or other inert liquid to prevent contamination from the lab environment and eliminate the impact of the Wegener–Bergeron–Findeisen (WBF) process (Beydoun et al., 2017; Broadley et al., 2012; Polen et al., 2016; Pummer et al., 2015; Reicher et al., 2018; Wright et al., 2013; Zolles et al., 2015). The WBF process occurs when one droplet freezes and takes up water vapor at the expense of unfrozen droplets, potentially inducing evaporation of nearby droplets. Contact by the growing frost halo around the frozen droplet can also induce freezing of neighboring droplets (Budke and Koop, 2015; Jung et al., 2012). Freezing assays that do not use oil typically use fast cooling rates of up to 10 ∘C min−1 so there is not enough time for these WBF effects to manifest, but this shifts the observed freezing temperature several ∘C colder (Mason et al., 2015). A cooling rate of 1 ∘C min−1 is more representative of typical atmospheric updraft velocities and the associated cooling rates. The oil environment prevents evaporation and these interferences, enabling slower cooling rates and droplet refreeze experiments. However, little assessment has been done to determine how or if these oils are influencing droplet freezing. We found that the surrounding squalene oil reduces the observed freezing temperature of ice-active biological particles (protein aggregate macromolecules) in successive droplet freeze–thaw–refreeze experiments of Snomax bacterial ice nucleants (Polen et al., 2016). We interpreted this as caused by the hydrophobic partitioning of the largest and most ice-active macromolecules into the highly hydrophobic squalene oil that was accelerated by droplet freezing, which was previously suggested by Budke and Koop (2015). Some recent microfluidic ice nucleation techniques use fluorinated oils and/or large concentrations of surfactant to stabilize the emulsified droplets (Reicher et al., 2018; Stan et al., 2009; Tarn et al., 2018). Their measured homogeneous freezing temperatures are typically within the expected range (−35 to −37 ∘C), but the surfactant may have unrecognized influences on heterogeneous freezing processes since freezing is enhanced via contact between the immersed particle and droplet interface (Durant and Shaw, 2005; Fukuta, 1975; Gurganus et al., 2014; Tabazadeh et al., 2002). However, Tarn et al. (2018) concluded that surfactants seemed to have little effect on the heterogeneous freezing temperatures for a number of particle types examined using droplets prepared by microfluidics with added surfactant.
A number of nonoil immersion alternatives to DFTs have arisen in the last few years. Some groups choose to keep droplets open to air and rely on a clean, dry flow of air or N2 to prevent contamination and frost growth (Whale et al., 2015). One recent study created a completely enclosed droplet chamber by sandwiching an O-ring, water, and silicon substrate between cover slips (CSs) and sealing it with vacuum grease (Li et al., 2012). This resulted in a very clean environment conducive to homogeneous freezing of droplets with no need for a dry air flow over the droplets. In a comparison of droplets in oil and droplets in air, Inada et al. (2014) froze individual 3 mL droplets in n-heptane and in air and found similar freezing activity on noncoated glass slides. They correlated early freezing for these tests to the interfacial surface contact with the glass.
In addition to issues with surfaces and droplet matrixes, the “pure” water itself can introduce artifacts. Almost no work has comprehensively examined the impact of source or purity of water on homogeneous freezing. Inada et al. (2014) briefly compared tap water and MilliQ water, but these sources showed little difference when droplets were in n-heptane with a surfactant. Aside from this one report, to our knowledge, no one else has compared freezing temperatures of water from different sources. Most groups either use in-house MilliQ water systems or purchase commercial purified water, such as HPLC-grade water that is typically reserved for highly sensitive chemical analysis. A few groups additionally filter their water to remove larger particles (Hader et al., 2014; Hill et al., 2014). It is difficult to assess how well different substrates, water purification, and other method details influence the background water freezing spectrum as these important details are often not described in papers that use DFTs and the water background freezing spectrum is not always presented.
Here we report a series of experiments we have performed on the ice nucleation ability of “pure” water as is dictated by variables, including the substrate, water source, and droplet matrix. The following sections describe our experimental methodology, data analysis methods, results and analysis for the aforementioned method variables, as well as our recommendations for best practices for future ice nucleation experiments that use DFTs. We compare our results with those of previous reports that used analogous method parameters. Finally, we advance a simple proposal for future ice nucleation experiments that will allow ready comparison between different specific measurement systems, leading to more uniform analysis that will accelerate our understanding of ice nucleation. We believe the ice nucleation community has acquired many useful strategies for dealing with issues such as contamination but that this knowledge remains largely internal within research groups and is rarely properly communicated to the larger and quickly growing community. This can discourage further advances and improvements to current designs of droplet freezing systems for INP measurements and create barriers to new groups beginning ice nucleation research. We desire to make it common practice to report these important method details and observations of pure water controls that are currently often overlooked, and begin a discussion of best practices in the community for ice nucleation experiments and droplet freezing spectrum analysis.
The droplet freezing system used in this study has been updated slightly since we first described it in Polen et al. (2016). Briefly, we use an oil-immersion droplet freezing system composed of a cascade three-stage thermoelectric air-chiller (TECA, AHP-1200CAS) as the heat sink, mounted under a single-stage thermoelectric element (TE Technology Inc., VT-127-1.4-1.5-72) for fine temperature control. An aluminum sample dish sits atop an aluminum block that contains the single-stage thermoelectric element and a thermistor (TE Technology Inc., MP-3176) for temperature measurements. Our temperature measurement has an uncertainty of ±0.5 ∘C based on the thermistor's accuracy and our temperature calibrations. Droplets immersed in oil are placed in the aluminum dish, which is covered by a clear acrylic case for imaging by optical microscopy. No air is flown into the chamber over the oil.
Droplets are created using a variable electronic micropipette (SEOH, 3824-1LC) to deposit droplets of 1 or 0.1 µL volume. Droplets are deposited on a substrate that sits under squalene oil (VWR, squalene, ≥98 %), mineral oil (VWR, mineral oil light), or just air. Several types of substrates were tested in this study: hydrophobic silanized glass CSs (Hampton Research, HR3-231), silicon wafer chips (Ted Pella, 16007), Vaseline®, a gold wafer (Ted Pella, 16012-G), a “new” gold wafer (Angstrom Engineering, 2WAU500-Q1), gold-coated cover slips (Ted Pella, 260156-G), and solid polydimethylsiloxane (PDMS) polymer (Dow Corning, Sylgard 184). Water for these experiments is either from our in-house MilliQ water purifier (EMD Millipore) or bottled HPLC-grade water (Sigma Aldrich, HPLC Plus 34877).
Substrates were cleaned or prepared in the following ways for these experiments. Silanized cover slips were used fresh from the box without any additional cleaning. A new silanized cover slip was used for each subsequent experiment. Silicon wafer chips were cleaned with HPLC water and acetone and allowed to air dry before use. Gold wafer and gold cover slips were cleaned with acetone and allowed to air dry before use. PDMS solid substrates were soaked in squalene oil for several days before use.
A CMOS camera attached to the microscope (5× magnification) acquires an image every 5–6 s. We are able to view on average 40–50 1 µL droplets or 70–90 0.1 µL droplets. Frozen droplets appear black, except in the case of a gold background in which the droplets become white. These images are processed using a custom MATLAB program that determines freezing events based on a grayscale value (Budke and Koop, 2015; Jung et al., 2012; Reicher et al., 2018) and also determines the diameter of each droplet. Sizing is calibrated using a 1 mm micrometer with 0.01 mm divisions. Initial tests run on gold substrates could not be analyzed by this program because of the inverted color scale produced by the dark gold background, so they were analyzed manually; “new” runs were analyzed using an updated version of the program.
Data compilation and analysis is performed in one of two ways. The first is a typical statistical analysis to determine the average and standard deviation of all runs of the droplet frozen fraction as a function of temperature. This analysis is performed when numerous arrays of many droplets have been measured, where each array is treated as a replicate experiment. This allows us to determine standard deviations to evaluate experiment-to-experiment variability for replicate droplet arrays. The second approach combines all the individual arrays into a single dataset. As an example, in two arrays of the same sample type, one of the arrays had a single droplet freeze early at −25 ∘C and the second array had two droplets freeze at −25 ∘C. In this case, combining the data would result in 3 droplets freezing at −25 ∘C. This second method increases the number of droplets in a set when the number of droplets is fairly low per run; it is also used when the number of runs is small (e.g., two tests of a single substrate) because statistical methods are less meaningful for low droplet counts. Figure 1 shows an example of these two methods of data compilation of the freezing spectra. There is some deviation between the combined unified dataset (blue) and the average of the individual replicates (red), but the combined data never fall outside the standard deviation of the averaged data and thus we believe the combination approach is an acceptable representation of our results, especially when there are low droplet counts available for a given set of experimental parameters.
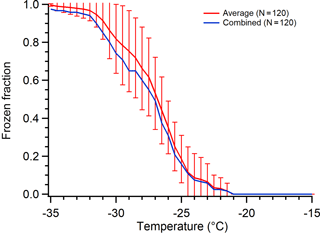
Figure 1Comparison of averaging data from droplet freezing experiments on 120 total droplets measured using three replicate arrays of 40 droplets each (red with error bars) versus combining those 120 droplets into one single hypothetical array of droplets (blue). The standard deviation from the average of the three replicate arrays is shown by the vertical error bars.
We present our data as the fraction of frozen droplets in combination with a metric derived from that freezing spectrum – the ice nuclei concentration (cIN) – using Eq. (1) (DeMott et al., 2017; Hader et al., 2014; Hill et al., 2016; Vali, 1971, 2008). cIN is a droplet volume-normalized representation of the unfrozen fraction of droplets,
where Vd is the average volume of the droplets as determined by the image analysis program and Nunfrozen is the fraction of droplets unfrozen at a given temperature. cIN has also been referred to as a cumulative nucleus concentration in Vali (1971) and depicted as K(θ) in his Eq. (13). We assume the droplets are close to spherical during imaging in determining Vd. Hader et al. (2014) describe the derivation of Eq. (1) and present the apparent INP (or ice nuclei) concentration for pure water spectra in comparison to their particle samples. The concentration of ice nuclei per droplet volume provides a way to directly assess the impact on freezing caused by a sample as compared to any contaminants or artifacts within the measurement. Normalizing the ice nuclei concentration by the surface area (or mass) of particles within the droplets defines the metric known as the ice nucleation active site density, ns (or nm). ns and nm are often used in the ice nucleation literature to compare different measurements of INPs. However, there are known discrepancies when assigning ns or nm values and then comparing identical particles under widely varying particle concentrations (Beydoun et al., 2016). In DFT one typically does not have any knowledge of the contaminants that induce freezing in pure water and thus we cannot determine the density of active sites (e.g., ns or nm) of the contaminants, unlike in studies of heterogeneous ice nucleation where the particle surface area or mass concentration is known or can be estimated. However, we still want to directly compare droplet freezing spectra from different experiments, and normalizing to the droplet volume provides a simple and useful way to do this. More importantly, the INP concentration is also the relevant parameter for assessing how INPs interact with and affect clouds (Hoose and Möhler, 2012). Finally, the cIN metric allows the ready comparison of droplet freezing spectra obtained using different droplet volumes, as different research groups use a range of droplet sizes in DFT. However, this is only possible if similar particle-in-water concentrations are used. ns or nm are often used to account for these particle concentration differences, but as discussed these metrics may not properly account for changes caused by differing surface area or mass concentrations. The cIN metric, when appropriately used, is advantageous as it only assumes that the INP concentration scales linearly with the droplet volume.
We include the theoretical homogeneous freezing spectrum for our droplet sizes in all our droplet freezing temperature spectra below. This was produced using the parameterization of Koop and Murray (2016) to calculate the freezing rate, J(T), and Eq. (9) from Beydoun et al. (2016) to determine the frozen fraction, Pf, using J(T).
Our results are divided into several sections that assess experimental variables tested in our DFT measurements such as substrate type and pure water generation methods. Each section begins with a brief review of previous results obtained by other ice nucleation groups using an analogous method and a discussion of why that specific method was chosen. The first section compares droplet freezing using oil immersion compared to in-air droplet freezing. The next section goes into detail on the impact of using different sources and water purification. Then we discuss a variety of substrates examined and compare them to identify what substrates display the best performance for droplet freezing. The final section discusses tests on two droplet generation methods we used.
4.1 Droplet immersion matrix: oil versus air
A number of droplet freezing methods have created droplets without an oil matrix, exposing the droplets directly to air (Li et al., 2012; Mason et al., 2015; Whale et al., 2015). This method requires very clean, dry conditions to avoid artifacts such as the Wegener–Bergeron–Findeisen process and droplet contamination by aerosolized INP. In the case of the BINARY system, droplets are physically separated from one another by a PDMS mask (Budke and Koop, 2015). For systems where droplet separation is not possible, dry air or nitrogen is typically flowed over the droplets to remove ambient water vapor (Whale et al., 2015). Flowing dry air, however, exacerbates the issue of droplet evaporation and thus large droplets must be used to limit the impact of evaporation over the whole course of the temperature ramp. One unique droplet-in-air measurement was achieved by sealing a chamber completely with a single water drop deposited on the substrate in the chamber and then evaporating and re-condensing the water vapor into many smaller droplets (Li et al., 2012). This method avoids the issue of ambient water vapor altogether by turning all the sample water into vapor and re-condensing before freezing.
We have attempted droplet-in-air measurements within our own system but consistently had issues with frost halo formation upon reaching −20 ∘C using a standard cooling rate of 1 ∘C min−1 (Budke and Koop, 2015; Jung et al., 2012). A series of images in Fig. 2 shows this frost growth, which resulted in the freezing of nearly all pure water droplets by −20 ∘C on hydrophobic cover slips when oil was not used. Frost growth similar to this has been reported previously by Whale et al. (2015) in their cold stage system. This suggests that our system is not airtight enough to perform this type of experiment when ambient humidity levels are elevated, such as during summer. Li et al. (2012) froze their samples between two glass cover slides which were sealed together with vacuum grease for the entire experiment. Our chamber must be opened between runs which causes water vapor to condense onto the sample dish and elsewhere within the sample chamber. In this experiment, we flushed the chamber with dry nitrogen similar to previous methods, but frost growth still occurred, though at much lower temperatures than tests without the nitrogen flow. Figure 2 shows the progression of frost starting at the bottom of the cover slip and continuing to grow toward the top of the glass. We consistently found that freezing and frost growth initiated around −20 ∘C, and we were never able to approach homogeneous freezing, likely due to our slow but realistic 1 ∘C min−1 cooling rate.

Figure 2Progression of frost halos in one pure water droplet freezing experiment without an oil matrix. Dark droplets are frozen. The black line highlights the frost growth (which is visible in the image but difficult to see) spreading from the bottom left toward the top of the image. Aside from the indicated frost growth, we can also see that other droplets induce freezing in neighboring droplets, such as the droplet on the far right in image 1 (red arrow) and the top right droplet in image 2 (yellow arrow). Subsequently induced droplets are indicated by similarly colored arrows.
Many droplet freezing measurements use an oil matrix to prevent frost halos, droplet evaporation, and external contamination (Broadley et al., 2012; Pummer et al., 2015; Wright et al., 2013; Zolles et al., 2015), which is why we chose to use squalene oil for our measurements. Oil also facilitates droplet refreeze experiments to evaluate the repeatability of the ice nucleation process, and any time-dependent effects such as particle sedimentation or aggregation (Emersic et al., 2015; Wright et al., 2013). In Polen et al. (2016), we proposed the use of mineral oil for biological samples, such as Snomax, to prevent changes in freezing behavior due to hydrophobic partitioning, which we suspected to be the case for refreezes performed in squalene oil (C30H50). However, in our attempts to use mineral oil (light) in pure water measurements, the mineral oil froze around −30 ∘C. We consistently saw what we at first assumed to be fogging, but upon closer inspection we found that the mineral oil had frozen completely solid, precluding droplet freezing experiments. Though we never saw mention of the freezing point in the material safety data sheets provided for the mineral oils, this is a known issue in the use of mineral oil for liquid chilling in desktop computers. However, the WISDOM microfluidic DFT device uses mineral oil for droplet creation and storage (Reicher et al., 2018). The device has successfully measured homogeneous ice nucleation down to −36 ∘C. Perhaps the surfactant (Span80, 2 wt %) used to stabilize the immersed droplets prevents freezing of the mineral oil. There are also a wide range of different mineral oils available from common chemicals suppliers, and the specific type of oil used in WISDOM is not known. Alternatively, the optical fogging may not be visible when such a small volume of oil is above the droplets, as is the case for microfluidic devices. Despite the promising results from the WISDOM method, we are wary to suggest that any other groups attempt the use of mineral oil for droplet freezing measurements before further investigation into how the oil's freezing may impact water droplet freezing. For all oil-immersion experiments mentioned in the following sections, squalene oil was used as the oil matrix, following the method of Wright and Petters (2013). Previously, we have shed light on squalene oil reducing the observed ice nucleation activity of Snomax bacterial particles and concluded that this was due to hydrophobic partitioning of large protein aggregates (Polen et al., 2016). This was only observed in droplet refreeze experiments of Snomax, and we do not observe this effect on any other particle sample type we have tested. Squalene oil remains our recommended immersion oil for most droplet freezing experiments.
4.2 Water sources and purification
Many in the ice nucleation community use MilliQ water or similar commercial systems to purify their laboratory's in-house water (Inada et al., 2014; Pummer et al., 2015; Rigg et al., 2013; Tobo, 2016; Umo et al., 2015; Wright and Petters, 2013). Some groups have used bottled HPLC grade or other similar water for their DFT (Fornea et al., 2009; Wright and Petters, 2013). Still others use alternative methods, such as condensation, to create droplets (Campbell et al., 2015; Li et al., 2012; Mason et al., 2015). We compared water produced by our in-house MilliQ system with bottled HPLC-grade water from Sigma Aldrich (Fig. 3). Both water types were also filtered using 0.02 µm pore size Anotop filters before droplet generation. In general, the droplet freezing spectra obtained from the two types of water are very similar to one another. With ∼1000 droplets for each water type, we find little difference in the apparent INP concentration as well. The biggest deviation came from runs of HPLC water that was filtered multiple times over many weeks using the same Anotop filter, which shows an increase in ice nuclei around −25 ∘C, though this is not outside the standard deviation of our other samples. This result indicates that either purchased HPLC or produced MilliQ water could be useable for droplet freezing experiments. As MilliQ water systems use a series of filter cartridges and a membrane filter to remove dissolved contaminants, particles, and ions from the supplied water, the quality of the produced water achieved will depend on the quality of the original water supply source. The “house” water supply is beyond the control of most research groups. Along with other issues we have experienced using MilliQ water that we discuss below, high-quality bottled water with additional filtration may be a better and more reliable water source for ice nucleation studies.
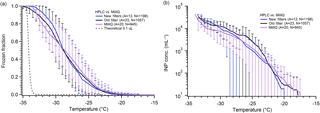
Figure 3Comparison of pure water freezing using filtered MilliQ-produced water and filtered purchased HPLC water. Shown are the measured droplet freezing temperature spectra (a) and the derived INP concentration (b). HPLC water was filtered using a new Anotop 0.02 µm filter for each bottle of water (blue), or the same filter for multiple stock bottles of water (black). The results from typical MilliQ water arrays are shown in purple. The parentheses next to each legend entry contain the number of arrays of droplets (A) and the total number of droplets across all arrays (N). The gray dashed line indicates the theoretical homogeneous freezing curve of 0.1 µL droplets, using the parameterization of Koop and Murray (2016).
We experienced significant and unexpected issues in continuing to use MilliQ water for our droplet freezing tests and experiments that caused us to switch to bottled HPLC water for all our future experiments. The MilliQ-produced water can result in very inconsistent results for pure water droplet controls if the particle membrane filter is not changed on a regular basis. These contaminants were apparently not removed by filtering the poor-quality MilliQ water with a 20 nm pore Anotop filter, for reasons unknown to us. This is a serious concern as there is no easy way to determine the status of the filter; the MilliQ system only measures the resistivity of the water as a measurement of the ionic strength, as well as total carbon concentration. Figure 4 shows results from trying to diagnose the issue behind a much warmer than typical background freezing spectrum for MilliQ water droplets. The results were highly inconsistent, with droplets in some arrays freezing in temperatures as warm as −13 ∘C, some droplet arrays freezing completely before −25 ∘C, and one array with a median freezing temperature, N50, of −28 ∘C that rivaled our least contaminated pure water tests at the time. We also found a significant decrease in the early freezing droplets when we let the MilliQ system run for 5 min before collecting water used to generate the control droplets. These caveats in using MilliQ water will likely depend greatly on different lab environments, protocols, the number of users, and differences in the original water supply sources. Thus, we chose to perform future experiments with bottled HPLC water in an attempt to improve experiment-to-experiment consistency by removing the variability posed by the MilliQ system's water quality. Additionally, we filter our water before use with a 20 nm pore-size Anotop filter to further reduce variability and remove small particles that may be a source of INPs. The use of an Anotop filter was suggested to us by Thomas Hill, as is used in the CSU Ice Spectrometer system (Garcia et al., 2012; Hill et al., 2016).
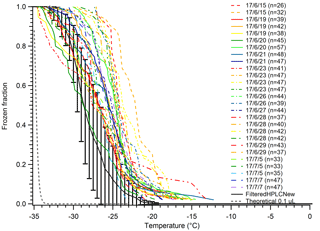
Figure 4A series of tests on MilliQ-generated water droplets to determine contamination sources. Droplets displayed inexplicably high freezing temperatures compared to filtered HPLC water at the time (solid brown with error bars). Temperatures for N50 ranged from −20 to −29 ∘C from day to day. Error bars indicate standard deviation of data for the filtered HPLC water. The gray dashed line indicates the theoretical homogeneous freezing curve of 0.1 µL droplets, using the parameterization of Koop and Murray (2016).
4.3 Substrate tests
In this section we discuss an extensive series of experiments in which we tested the effect of various substrates on the observed freezing spectra for pure water droplets. Our goal is to identify substrates that display a reproducibly low amount of interference in the pure water controls by allowing the droplets to freeze close to the expected homogeneous freezing temperature. This is −33 to −35 ∘C for the droplet volumes used here based on Eq. (7) from Pruppacher (1995) and the homogeneous freezing spectrum predicted using the parameterization of Koop and Murray (2016) (dashed lines in all the droplet freezing temperature spectra). Except when noted, all arrays were created using filtered HPLC water. Each of these substrates has been shown to work reasonably well for droplet freezing experiments in the past.
4.3.1 Hydrophobic cover slips
Hydrophobic cover slips are one of the most used substrates for DFTs (Bigg, 1953; Durant et al., 2008; Iannone et al., 2011; Mason et al., 2015; Murray et al., 2011; Wright and Petters, 2013). These can be made in-laboratory by silanizing a standard glass slide (Fornea et al., 2009; Wright and Petters, 2013) or can be purchased presilanized (Beydoun et al., 2016, 2017; Iannone et al., 2011; Mason et al., 2015; Polen et al., 2016; Whale et al., 2015; Wheeler et al., 2015). In general, results of pure water freezing on hydrophobic cover slips are variable. Whale et al. (2015) reported the 50 % droplet frozen fraction (N50) close to −26 ∘C for 1 µL droplets. Hader et al. (2014) reported N50 at −30 ∘C for 150 nL droplets, while Iannone et al. (2011) found N50 at −37 ∘C for 60 nL droplets. While an increase in homogeneous freezing temperature is expected for larger droplets, based on classical nucleation theory (CNT) we expect all of these droplet sizes to freeze homogeneously below −30 ∘C (Koop and Murray, 2016; Pruppacher, 1995; Vali, 1999). This implies that the larger droplets froze heterogeneously due to some unintended ice-nucleating material or surface.
Our results using presilanized hydrophobic cover slips are similar to those reported using analogous methods by Hader et al. (2014) and Whale et al. (2015) for our larger and smaller droplets, respectively. Figure 5 displays our freezing spectra for large and small HPLC droplets on hydrophobic cover slips. The N50 for smaller (0.1 µL) droplets (black and blue) is −29 ∘C, and −27 ∘C for larger (1.0 µL) droplets. Freezing onset begins consistently around −20 ∘C, and final droplets freeze between −33 to −35 ∘C as is expected for these droplet sizes. Importantly, we note that freezing pure water droplets simultaneously alongside sample droplets containing test particles (shown in green in Fig. 5) does not impact the freezing temperature spectrum when compared to the same droplet size data (red). This, in conjunction with the similar literature results, suggests that variability between different DFT systems for pure water controls using hydrophobic cover slips may be explained primarily by the droplet size. However, we find a counterintuitive trend when comparing the apparent INP concentration, cIN, for these measurements. When comparing larger and smaller droplets, the concentration of ice nuclei is actually lower for larger droplets (red vs. blue points in Fig. 5). This could mean that the INP concentration for these samples is not directly related to the droplet volume but instead is more directly tied to the contact surface area with the substrate. We propose that this may be caused by one of two effects: (1) smaller droplets have larger surface area-to-volume ratios and by normalizing to volume using cIN we are undercorrecting interferences caused by droplet–surface contact for small droplets, or (2) larger droplets have higher contact area with the surface and thus by correcting to volume we are overcorrecting interference experienced by larger droplets. More work is necessary to connect the contact area to the elevated pure water freezing temperature. This size effect is also observed for the gold-coated substrates discussed in Sect. 4.3.4.
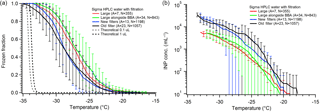
Figure 5Droplet freezing temperature spectra (a) and apparent ice nuclei concentration, cIN, (b) for pure water droplet freezing measurements on a hydrophobic cover slip. In all experiments HPLC water that was filtered using an Anotop 0.02 µm syringe filter was used. Each data series has been binned into 0.5 ∘C temperature increments. The red data series is from large (1.0 µL) droplets, green is from large (1.0 µL) droplets measured alongside biomass burning aerosol sample droplets (Fig. 12), blue is from small (0.1 µL) droplets using a new Anotop filter for each stock bottle of filtered water, and black is small droplets using a singular Anotop filter for many different stock bottles of water. The parentheses next to each legend entry contains the number of arrays of droplets (A) and the total number of droplets across all arrays (N) tested for each experiment type. Error bars are standard deviations for the replicate droplet arrays. The gray and black dashed lines indicate the theoretical homogeneous freezing curves of 0.1 and 1 µL droplets, respectively, using the parameterization of Koop and Murray (2016).
We have also observed some batches of purchased cover slips to induce freezing as warm as −18 ∘C, and with much greater variability in the freezing spectra. Thus, it is important to evaluate each batch of cover slips to test for these potential issues. Ideally pure water control droplets will be placed along with droplets containing the particle sample of interest on the same cover slip to directly evaluate the background freezing spectrum on that specific cover slip. This is especially important when working with particle systems of weak ice activity that freeze close to the background water temperature range.
4.3.2 Silicon wafers
A few groups have utilized silicon wafers for droplet freezing experiments (Li et al., 2012; Peckhaus et al., 2016). Peckhaus et al. (2016) used droplets of 107 µm in diameter and found 90 % of droplets froze below −35 ∘C. All droplets reported by Li et al. (2012) froze below −37.5 ∘C for 10–70 µm in diameter. Additionally, Li et al. performed detailed assessment of hydrophobic and hydrophilic silicon wafers used in pure water ice nucleation experiments. They found that both types of wafer produced nearly homogeneous freezing for pure water droplets.
We investigated ice nucleation on silicon wafer chips typically used for SEM analysis. Several silicon chips were placed in the sample dish with squalene oil, and 0.1 µL (∼600 µm) HPLC droplets were deposited on them. Due to the small size (5×7 mm) of the chips, the number of droplets on each wafer chip was very low (∼10), and thus we combined all the data from twelve chips as though it were a single surface containing 120 droplets (Fig. 6). We find similar freezing activity to the hydrophobic cover slips, with onset freezing beginning around −21 ∘C, reaching 50 % around −26 ∘C, and finishing at −35 ∘C. The apparent INP concentration for the silicon wafer also falls close to the cover slip data (Fig. 6). We are using much larger droplets (∼6–60× diameter) than the groups who have used silicon substrates previously, so we do see higher freezing temperatures as expected. However, due to the similar behavior and apparent INP concentration we observe using the glass cover slips and the silicon wafer, we cannot conclude that silicon provides a more ideal surface for INP studies than silanized hydrophobic glass. The superior performance reported by other groups using silicon wafers may be due to higher purity water than we have access to, or other method details that make a direct comparison challenging.
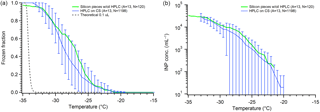
Figure 6Comparison of freezing on silicon wafer chips (green) against hydrophobic cover slips (blue), following Fig. 5. The freezing temperature spectrum is on (a), and the retrieved cIN is on (b). Both datasets use 0.1 µL droplets. The data from all replicate arrays using silicon (green) are combined into one series and thus no error bars can be determined. The parentheses next to each legend entry contains the number of arrays of droplets (A) and the total number of droplets across all arrays (N). The gray dashed line indicates the theoretical homogeneous freezing curve of 0.1 µL droplets, using the parameterization of Koop and Murray (2016).
4.3.3 Vaseline®
First utilized by Tobo (2016) for the Cryogenic Refrigerator Applied to Freezing Test (CRAFT) droplet freezing instrument, Vaseline® petroleum jelly can be spread onto a clean surface to create a makeshift hydrophobic substrate. The results from Tobo (2016) indicate great promise in this substrate for DFT as the large, 5 µL droplets froze with ∘C, approaching the temperature predicted by CNT for homogeneous freezing. We examined large (1.0 µL) droplets on Vaseline® spread onto our aluminum sample dish in air, similar to Tobo (2016), as well as smaller droplets (0.1 µL) on Vaseline®, and within a squalene oil matrix. The results are shown in Fig. 7. For tests without the oil matrix, we found quite warm onset freezing temperatures while only a few droplets approached the homogeneous regime. We found similar trends whether we used MilliQ water or filtered HPLC water. However, once we utilized smaller droplets in an oil matrix, the early onset freezing vanished and we observed good background freezing curves with lower onset and N50 temperatures. We hypothesize that our inability to reproduce pure water freezing near the homogeneous regime using a Vaseline® coated substrate as in Tobo (2016) is due to the difference in cleanliness between laboratory environments as well as differences in applying the Vaseline® layer. The oil matrix does eliminate much of the early, high-temperature freezing that is likely caused by contamination or an unevenly coated surface. This suggests that the use of a laminar flow hood or glove box may be necessary to achieve such low background freezing temperatures without oil when the droplets are exposed to air. Tobo prepared their droplet arrays inside a glove box within a clean room environment, and such clean conditions are not readily available to many research groups. Uniform application of Vaseline® requires precision and a specialized spatula to get around the lipped design, and nonuniform application will increase the risk of surface-induced freezing by any exposed underlying substrate. Interestingly, we note that one benefit to Vaseline® is we did not observe evidence of WBF effects on neighboring droplets when in air, which makes it favorable for droplets-in-air experiments if interferences can be reduced. Creation of a surface specifically designed for Vaseline® application is an important consideration if this promising technique is to be utilized more widely.
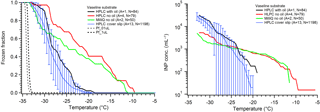
Figure 7Pure water droplet experiments on a Vaseline-coated substrate, following Fig. 5. The HPLC water using silanized cover slip data in blue are displayed for comparison, as are the data from the hydrophobic cover slip using small droplets (Fig. 5, blue). The data from replicate arrays for Vaseline are combined as described in Sect. 2 and thus no error bars are determined for these. Three sets of experiments on Vaseline are shown: black is small droplets (0.1 µL) of HPLC filtered water in oil, red is large droplets (1.0 µL) without oil, and green is large droplets of MilliQ water without oil. The parentheses next to each legend entry contains the number of arrays of droplets (A) and the total number of droplets across all arrays (N). The gray and black dashed lines indicate the theoretical homogeneous freezing curve of 0.1 and 1 µL droplets, respectively, using the parameterization of Koop and Murray (2016).
4.3.4 Gold-coated substrates
Limited tests have been reported using gold-coated substrates in DFTs. Häusler et al. (2018) etched the surface of a gold-coated substrate and found near-homogeneous freezing temperatures (3 ∘C) for pure water droplets (45 µm) despite obvious nanoscale features in the freezing chip's cavities. In our tests we used two substrates: a gold-coated silicon wafer and a gold-coated glass CS. Our results are shown in Fig. 8. The HPLC water on gold wafer produced a very low freezing temperature, with N50 around −32 ∘C; similarly small droplets of MilliQ water on the gold CS had N50 at −30.5 ∘C. Additionally, our first test on a second gold wafer (red) with many more droplets showed N50 at −33.9 ∘C. However, large HPLC water droplets on the gold CS ( ∘C) froze froze at a similar temperature to large droplets on the hydrophobic silanized cover slip ( ∘C). When comparing the apparent INP concentrations, we again see the trend of larger droplets having lower cIN than smaller droplets. In this case the difference is even starker, with nearly half an order of magnitude difference in cIN between large and small droplets on gold CS at T < −30 ∘C. Additionally, we find that upon cleaning and reusing a gold wafer (orange) the freezing spectrum and apparent INP concentration increased compared to the first use (red) and became similar to the silanized cover slip. This could suggest that cleaning the surface with acetone and drying with dry, particle-free air affects the surface in some way, making it more ice active, or just does not adequately clean the substrate. More analysis should be performed to identify the impacts of cleaning on the gold surface. If this issue can be solved or avoided and the surface can be cleaned without introducing contamination or ice-active surfaces, then gold has the potential to be a near-ideal substrate. One issue with gold surfaces is they are soft and easy to scratch, even with careful handling using Teflon-coated tweezers. This could create more ice-active surface sites over time and also be an interference in the droplet optical microscopy imaging. Gold is also much darker than the other substrates we tested, requiring manual retrieval of the droplet freezing spectrum.
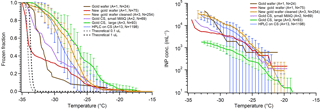
Figure 8Measurements of pure water droplet freezing on gold substrates are shown following Fig. 5. The data from small HPLC water droplets on a silanized cover slip are displayed in blue for comparison (Fig. 5, blue). The gold data displayed are using HPLC water droplets on a gold wafer substrate (brown), small MilliQ droplets on a gold-coated glass cover slip (gold CS) (lilac), and large HPLC water droplets on a gold CS (green). Also displayed are data from small droplets on another gold wafer upon first use (red), and subsequent small droplet arrays on the same wafer following cleaning and drying, with associated error bars (orange). The parentheses next to each legend entry contains the number of arrays of droplets (A) and the total number of droplets across all arrays (N). Error bars show standard deviation from replicate droplets arrays. The data from the gold wafer (brown and red) and small droplets on a gold CS (lilac) were combined into one series and so no error bars are derived. The gray and black dashed lines indicate the theoretical homogeneous freezing curve of 0.1 and 1 µL droplets, respectively, using the parameterization of Koop and Murray (2016).
4.3.5 Polydimethylsiloxane (PDMS)
Polydimethylsiloxane (PDMS) is a widely used hydrophobic, cross-linked polymeric material. PDMS has been used in microfluidic droplet freezing approaches (Reicher et al., 2018; Stan et al., 2009), but not as a substrate for conventional DFT. Reicher et al. (2018) provided a comparison of microfluidic systems with other DFTs that showed comparable homogeneous ice nucleation rates for all methods. The excellent performance of these published microfluidic techniques, and our own experience with microfluidic devices fabricated from PDMS for DFTs, led us to test PDMS as a droplet freezing substrate. We studied two types of PDMS: a squalene oil-soaked hydrophobic PDMS surface (untreated), and a surface that was exposed to a plasma, then baked at 180 ∘C, and soaked in squalene oil for several days (treated). The latter represents PDMS as would be typical for a microfluidic device fabricated using conventional soft lithography. One important note is the treated PDMS did return to its original hydrophobic form following plasma treatment and oil soaking and displayed similar freezing results to the untreated PDMS (Fig. 9). The pure water freezing spectra are again similar to our silanized cover slip results, as we have seen for most of the other substrates tested. Each of the PDMS tests was within the standard deviation of the glass CS data, suggesting that the PDMS surface does not provide any inherent benefit over hydrophobic silanized glass. However, PDMS is quite cheap and easy to manipulate if you have the resources to do so, which makes it a quite useful substrate for ice nucleation studies. The hydrophobic nature of the polymer can make it prone to contamination, however, and PDMS is often used as a sorbent in environmental contaminant sampling (Choi et al., 2011; Thomas et al., 2014). One other potential downside to PDMS for DFTs is its poor heat transfer properties. The thickness of the PDMS layer must be consistent for each experiment or the temperature calibration will be inaccurate.
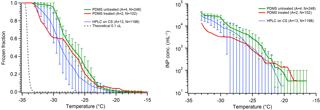
Figure 9Measurements of HPLC pure water droplet freezing on polydimethylsiloxane (PDMS) are shown in red and green, following Fig. 5. The data from small droplets on a silanized cover slip are displayed for comparison in blue (Fig. 5, blue). The PDMS data were obtained using treated (red) and untreated (green) PDMS polymer with small droplets. The parentheses next to each legend entry contains the number of arrays of droplets (A) and the total number of droplets across all arrays (N). Error bars on green data show standard deviation from replicate arrays, while the red data are combined into one series as explained in section 2. The gray dashed line indicates the theoretical homogeneous freezing curve of 0.1 µL droplets, using the parameterization of Koop and Murray (2016).
We have recently developed a new “store-and-create” microfluidic device that shows great promise in eliminating the interferences from surface interactions as seen in our and other groups' DFTs (Bithi and Vanapalli, 2010; Boukellal et al., 2009; Sun et al., 2011). This device will be fully described in a forthcoming paper. The PDMS device holds up to 600 droplets of ∼6 nL volume encased in squalene oil. Each droplet is stored in an isolated microwell, completely engulfed by oil. Initial results for pure water droplet freezing are shown in Fig. 10 and compared with hydrophobic silanized cover slips. We find a N50 around −34 ∘C, with less than 10 % of droplets freezing above −32 ∘C. Interestingly, we see that the apparent INP concentration continues the same trend as the 0.1 µL droplets on a hydrophobic cover slip. This is likely because the droplets lack contact with any solid surface inside the microfluidic device and the contaminants causing this nonhomogeneous freezing are related to water or oil contaminants.
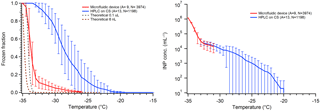
Figure 10Comparison of pure water droplet freezing in our new microfluidic chip (red) versus using a silanized cover slip (CS) (blue), following Fig. 5. Droplets in the microfluidic chip are 6 nL in volume and droplets on the CS are 0.1 µL. Error bars show variability of droplet freezing between different replicate arrays. The parentheses next to each legend entry contains the number of arrays of droplets (A) and the total number of droplets across all arrays (N). The gray and dark red dashed lines indicate the theoretical homogeneous freezing curves of 0.1 µL and 6 nL droplets, respectively, using the parameterization of Koop and Murray (2016).
4.4 Droplet creation methods
Some experimentation was done to compare two types of droplet creation techniques, using a syringe or autopipette. We have experienced issues with both approaches that we briefly describe here so that other users can be vigilant in avoiding these problems. Syringes create droplets with volumes of 0.1 µL that are very consistent in droplet size, much more consistent than pipettes working at similar volumes. However, using syringes has long-term usage issues when the water is not completely particle-free as they are difficult to clean. Each syringe (Hamilton Company, model 7001 KH) we used eventually became contaminated beyond use (evaluated by pure water control freezing spectra) and needed to be replaced. This becomes expensive when running freezing assays repeatedly for weeks and months at a time. Syringes are also not automated and can be fragile, requiring careful use that can be time consuming when creating an array of 50+ droplets.
Switching from a syringe to an electronic pipette with disposable tips improved the long-term consistency of droplet creation. In our experience sterilized tips in boxes remain contamination-free the longest. However, we are still uncertain about the amount of contamination introduced by the pipette tips. The best freezing experiments with pipetted droplets still show them freezing significantly above the homogeneous freezing regime, which could be caused in whole or in part by pipette tips, remaining water contaminants, or the silanized glass cover slip substrate.
The results presented above provide a detailed account of many tests run on pure water ice nucleation measurements using our cold-plate DFT. Figure 11 displays a summary of the major findings from different substrate tests. Vaseline provided the least consistency between droplet freezing temperatures with the highest onset freezing ( ∘C), even when droplets were surrounded by oil. However, Vaseline® had the one benefit of preventing frost-induced freezing compared to hydrophobic cover slips, when droplets were not in oil. Despite this, Vaseline® poses a significant number of issues, such as uneven surface coating and an unclean lab environment, which makes it impractical for many researchers. The gold wafer showed the most promise for our standard droplet freezing method, with N50 at −33.9 ∘C, but it also had some quite warm onset freezing ∘C) and when cleaned with acetone produced a similar freezing curve to other substrates (Fig. 11). Gold wafers have the caveats that they are quite expensive and the surface is easily scratched, as well as the potential for contamination when cleaning, which we saw when using the gold wafer (“Cleaned” vs. “New”, Fig. 11). PDMS, hydrophobic cover slips (both shown in Fig. 11), and silicon wafer chips (not shown) displayed very similar freezing behavior, with N50 between −27 and −29 ∘C, only slightly warmer than the gold wafer. Our new microfluidic device shows enormous improvements over these other methods, with less than 10 % of droplets freezing at temperatures warmer than −32 ∘C, consistently. The reason this device has such low freezing is likely because droplets are completely engulfed by a layer of oil and have little to no contact with the PDMS surface, unlike typical droplet-in-oil DFTs. We also observed mineral oil freezing at temperatures warmer than homogeneous freezing, and thus it should not be used for this type of analysis. We found that MilliQ water, when the system is operating properly, displays similar ice-nucleating properties to filtered HPLC water. Few studies in the past have analyzed and compared different water sources, so it is difficult to assess its impact on the ice nucleation results. We experienced significant interferences using MilliQ water, caused by the final particle membrane filter failing with no other indication of failure. This issue cost us several weeks of intensive testing to identify and resolve, which is why we recommend the use of bottled HPLC-grade water, with additional particle filtering, to remove the variability in the quality of the water used.
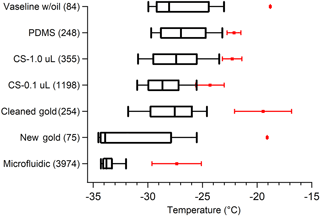
Figure 11Summary of pure water droplet freezing spectra for different substrates tested. Boxes show the 25 % and 75 % frozen quartiles and the median, N50, is indicated by the line inside each box. Red markers are the temperatures of the first onset freezing droplets with error bars showing variability between different replicate droplet arrays. CS represents droplets on a silanized glass cover slip. No error bars for the onset freezing for Vaseline and gold wafer are shown because only one array was run of each. Whiskers show the 10 % and 90 % droplet frozen fractions. Next to each substrate name in parentheses is the number of droplets tested. Filtered HPLC water droplets produced by an electronic pipette were used in all of these measurements, except for the microfluidic chip which generated the droplets on-chip. Droplets were 0.1 µL in volume, except for the 1.0 µL on the cover slip, and the 6 nL droplets created in the microfluidic chip.
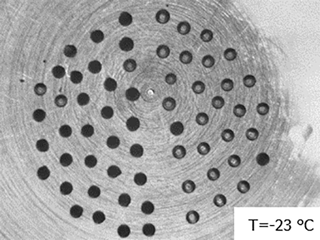
Figure 12Image of droplets containing biomass burning aerosol (left half) and pure water droplets (right half) immersed in squalene oil on a silanized glass cover slip. Droplets containing aerosol sample have mostly frozen (turned dark) and pure water droplets have remained largely unfrozen (grey) at −23 ∘C.
The intent of this study is to bring to light some of the unpublished and underreported results, experiences, and insights that are required to effectively examine heterogeneous ice nucleation using droplet freezing methods, especially when the ice-nucleating particles have low freezing activity. Providing a basic overview of the best results obtained for pure water controls in our tests and the literature can lead to a series of best practices or recommendations and more method standardization. While DFTs have improved to produce accurate and reliable immersion freezing measurements, we have certainly not achieved the ideal experimental methods and strategies. To continue to advance DFTs it is important that researchers present their raw data with all their imperfections, including pure water controls, comprehensive descriptions of method details and data analysis procedures, and raw droplet freezing temperature spectra. This is the information required for the ice nucleation community to learn from each other and continue to improve our experimental methods. This will also enable new research groups to start making accurate and reproducible freezing measurements more quickly and reliably. The following are recommendations that we propose all research groups incorporate into their droplet freezing experiments and publications of these results.
We suggest that researchers present an assessment of raw frozen fraction curves or spectra for all types of analysis performed (homogeneous and heterogeneous freezing). This practice is often followed in the literature, but there are plenty of instances where these data are not provided and instead the retrieved ice-active site density (ns, nm) is the exclusive result published. Frozen fraction spectrum is a base level analysis that all groups must do to retrieve any further parameters such as ns and nm. Thus, presenting the raw frozen fraction curves for all data is a simple addition to any paper, even if it is presented within the supplemental section. The raw spectra can be used by the authors and others to diagnose contaminants or inconsistencies between similar droplet freezing experiments and methods.
We encourage retrieving the apparent INP concentration, cIN, as an especially useful metric for quantifying the background freezing spectrum, and for comparison of different DFTs. This metric has often been used as an intermediate step to determine ice-active site density, but we believe it, in and of itself, is a useful metric that should be reported, especially when examining pure water controls. Since there is no way to know the specific properties of any contaminants within pure water droplets directly, having an idea of the level of contamination per volume of water provides useful insights into what may be preventing the homogeneous freezing temperature regime from being reached. Contrary to the frozen fraction curves, INP concentration is normalized to the droplet volume, which makes it an effective way to compare pure water controls in different DFTs that invariably measure different droplet sizes.
We will note there are some unexpected trends for our results regarding the retrieved cIN spectra when dealing with different droplet sizes. In particular, we see a lower concentration of ice nuclei when we use larger droplets, despite normalizing to the volume even when the same experimental conditions are used. This suggests that normalizing to volume may overcompensate for the differences between droplet sizes. We believe this may be because the apparent INP concentration is less influenced by the concentration of particles in the water and more influenced by the contact surface area between the droplet and the surface. Thus, normalizing to volume may not be the best metric for determining the activity of contaminants in homogeneous nucleation. Fixing the droplet volume can remove this issue and is another one of our recommendations below.
Procedures to correct the raw freezing spectra for interference from background freezing observed in “pure” water droplets should be reported. Retrieval of the cumulative nucleus concentration, K(T) or cIN, following previous approaches (DeMott et al., 2017; Hader et al., 2014; Hill et al., 2016; Vali, 1971, 2008) and as we have done here, is our recommended approach. This background freezing spectrum should be reported, and then subtracted from the sample's spectrum.
Alternatively, retrieval of the differential nucleus concentration, referred to as k(θ) in Vali (1971), is also recommended to assess the INP concentration in the sample versus that caused by background freezing. This approach can be used as a means of quantitatively attributing the INP signal to the sample versus the background for each droplet over the entire freezing spectrum. The differential nucleus concentration can be calculated using the following:
where k(T) is the differential ice nucleus concentration, Vd is the droplet volume, ΔT is a temperature step that must be prescribed in the analysis, ΔN is the number of droplets that froze in that ΔT temperature step, and N(T) is the total number of unfrozen droplets at T. An important aspect is that ΔT is not the temperature step of the actual measurements, such as from the frequency at which images are acquired. To produce meaningful k(T) spectra the ΔT should be large enough so that more than one droplet typically freezes in a given temperature step. In our initial k(T) analysis we found that a ΔT interval of 0.05 or 0.1 ∘C worked well for our experimental conditions. ΔT should be varied until a reasonable representation of the droplet freezing spectrum is produced that displays the important features of the spectrum and allows the sample to be distinguished from the background freezing of a control. Realizing that this is an important and nuanced detail, Gabor Vali is planning to produce a tutorial explaining the use of k(T) and selection of ΔT, using some of our data to illustrate this method. Referring back to Eq. (2), as an example, given an array of 100 droplets and a specified ΔT of 0.1 ∘C intervals, if the first 2 droplets freeze within one measurement interval, then ΔT=0.1 ∘C, ΔN=2, and N(T)=98. Using this metric, each freezing event in the interval ΔT is the result of at least one active INP, but given a small ΔT and a large N the interval can be approximately attributed to a single active INP.
Inherent to all droplet freezing methods is the assumption that the freezing of any droplet at a given temperature interval is caused by the combination of INPs present from the sample plus any background freezing due to impurities and substrate artifacts. The differential ice nucleus method, k(T), provides a quantitative assessment of the sample versus the background INP concentration at each temperature interval. k(T) is an alternative approach to the more commonly used method of just subtracting the cumulative K(T) or cINP background spectrum from the cumulative sample spectrum. This k(T) analysis method is discussed in detail by Gabor Vali in the comment (https://doi.org/10.5194/amt-2018-134-SC1) he provided on the discussion version of this paper (https://www.atmos-meas-tech-discuss.net/amt-2018-134/amt-2018-134-SC1-supplement.pdf, last access: 18 September 2018), based on the framework originally laid out in Vali (1971).
Restricting the freezing curve analysis to the 5 %–95 % frozen droplet fraction, as is now being done by some groups to exclude anomalously early and late freezing droplets, is not recommended. The ice activity of individual particles is very much a diverse spectrum, resulting in some droplets in a freezing array containing more rare ice-active INPs that induce freezing at warmer temperatures (Augustin-Bauditz et al., 2016; Conen et al., 2011; O'Sullivan et al., 2015; Petters and Wright, 2015; Pummer et al., 2012, 2015). This can occur even in experiments on “pure” single-particle-type samples such as Snomax bacterial and illite NX mineral particles (Beydoun et al., 2016, 2017). Excluding the early freezing droplets would erroneously omit information on these important rare INPs whose greater ice activity cause freezing at anomalously warm but atmospherically relevant temperatures.
Important method details should be documented. These include details related to the production of pure water used for droplet generation (including any additional filtration steps), any characterization of the purity of the water, and presentation of the freezing spectra for control droplets. Details regarding the substrate used and how it was prepared and cleaned are also important. Temperature calibration procedures should also be documented. DFTs are very subject to contamination, requiring new clean surfaces and sample handling vessels to be used. This is especially a concern when working with very ice-active biological particles such as Snomax and other bacteria. Droplet preparation methods such as the pipette, syringe, or microfluidic technique used; how the particle sample was (re-)suspended in the water; and the length of time the particles spent in water prior to analysis are additional method details that may appear trivial but can have important consequences on the observed ice nucleation properties. This is especially critical in DFT comparison studies between different groups using the same samples.
We recommend the use of bottled HPLC-grade or similar purchased water for droplet generation, as opposed to MilliQ-produced water. MilliQ systems can certainly produce high-quality water with freezing temperatures near the homogeneous regime but are subject to sudden unannounced changes in their water quality, and are also limited by the quality of the source water fed into the MilliQ system. Our own experiences and frustrations caused by the variability of MilliQ water has caused us to exclusively use HPLC-grade bottled water that we further filter with a 0.02 µm Anotop filter and then store in a clean glass bottle in the refrigerator. Interestingly, we have also heard that other research groups found bottled water is not as consistent as their MilliQ-produced water. This demonstrates the inconsistencies and variabilities that are common between research groups and supplies, further emphasizing the importance of routinely assessing and reporting the water background freezing spectrum that each group and method observes. We suggest that no matter what source of water is used that researchers regularly test it and report their findings in all publications when possible.
Based on the findings in this study, we recommend silanized cover slips as the primary substrate for DFT as they are the least expensive option that display the most consistent freezing behavior. Alternatively, if the cost of gold wafers is not prohibitive and measures are taken to avoid scratching the surface, then gold is a suitable substrate. Additionally, we note the incredible potential of microfluidic devices used in this study and others. We also recommend autopipettors over syringes for droplet generation due to their ease of use and reduction of potential contamination from repeated use compared to syringes.
Droplet volumes and particle-in-water concentrations should be standardized as much as possible. The commonly used ice-active surface site density metric (ns, nm) has regrettably been found to not properly normalize and correct for differences in the particle surface area or mass present in droplets during DFT. For example, just by changing particle concentration the ns values we retrieved for illite NX shifted by several orders of magnitude (Beydoun et al., 2016). Many groups purposefully vary particle concentration to access different observable freezing temperatures, but the ice nucleation properties retrieved using different concentrations of the same system may not be consistent. The best way to evaluate this (in)consistency is to ensure overlap in the ns spectrum retrieved versus temperature, so these values can be directly compared at the same temperature. This requires using small steps in particle concentration of about a factor of 5. Reporting the raw freezing spectra also helps to evaluate these issues. Standardizing the total particle surface area present, by standardizing the droplet volume and particle concentration used, may also reduce these discrepancies.
Interferences from the substrate and/or immersion oil used, the pure water, and other potentially unrecognized sources should be regularly evaluated using pure water controls that are prepared using procedures identical to those used for the sample droplets. Controls should be run with a frequency determined by the level of variability in the background freezing spectrum observed using these controls, and by how close the particle sample's freezing spectrum lies compared to the background spectra. We also suggest that researchers perform handling or method blanks alongside any experimental particle collection. Method blanks simulate all aspects of the particle collection and extraction process, without having a particle sample. This accounts for contamination or other issues that may occur as the sample filter is being handled better than methods running only a pure water control blank. For example, Vergara-Temprado et al. (2018a) found that the freezing spectrum of their filter handling blanks for their soot aerosol measurements showed similar droplet freezing spectra to the soot samples themselves, and significantly higher than their water blanks. Any new batch of purchased substrates must be evaluated to assess batch-to-batch differences, which we have observed for silanized glass cover slips. Studies of low ice-activity systems such as soot particles and biomass burning aerosol require careful and extensive background control experiments. In our measurements of biomass burning aerosol we prepare a droplet array on a silanized cover slip that consists of a 1:1 ratio of pure water control droplets and biomass burning aerosol-containing sample droplets (Fig. 12). This provides a direct assessment of any interferences from the same substrate used for sample analysis, and equal statistics for control and sample droplets.
DFTs are often evaluated by comparing measurements to published results for the same particle system. Unfortunately, we lack good reliable INP standards for proper comparison and calibration. Snomax is commonly used (Wex et al., 2015) but we identified serious issues stemming from the instability of the most ice-active ice nucleants in Snomax over time (Polen et al., 2016). This precludes Snomax as a reliable INP standard. Good comparisons have been found using illite NX minerals, but it is critical to ensure that an identical particle sample is used by each method (Hiranuma et al., 2015). Methods that collect aerosolized particles must take special care to account for their collection efficiency versus size. Just placing some material from the bulk sample into water can avoid these issues. The ice activity of mineral particles can also change with time spent in water, or by attack from strong acids. The very ice-active K-feldspar minerals are especially subject to degradation in water due to surface ion etching, particularly for those displaying hyperactive ice activity (Banfield and Eggleton, 1990; Holdren and Berner, 1979; Kumar et al., 2018; Peckhaus et al., 2016). Harrison et al. (2016) found that a particular and common type of feldspar that does not display hyper ice activity, BCS 376, was able to maintain its IN activity over many months in water. Engineered nanoparticles from inert metal oxides with reproducible particles sizes, surface properties, and pore sizes may be the most reliable type of INP standard, though this has not yet been evaluated and may be restricted to a narrow freezing temperature range (Alstadt et al., 2017; Archuleta et al., 2005; Findenegg et al., 2008; Marcolli et al., 2016). Until then illite NX mineral particles are likely the best INP standard choice, provided all the above caveats are accounted for.
This study and the above series of recommendations are intended to shine light on some potential sources of inconsistencies between droplet freezing methods and create a simple, unified analysis and representation for all ice nucleation community members to follow for future publications. Many researchers already have much of the above information available before publication and use that data for detailed analysis. In the interest of moving the community forward, we seek increased transparency regarding the aforementioned information by documenting important method details and the raw spectra for background water freezing control in all publications using droplet freezing methods.
The data used to produce each figure are available in the online supplement. A tab-delimited text file or set of files is provided corresponding to each figure. The data files contain variables including temperature, frozen fraction, standard deviation, ns (ice active surface site density), and other relevant parameters.
The supplement related to this article is available online at: https://doi.org/10.5194/amt-11-5315-2018-supplement.
MP and JS performed droplet freezing experiments and analysis. TB designed the microfluidic chip and analysis program and performed microfluidic device experiments. RS devised the project and recommendations for future DFT analysis. MP and RS wrote the paper, with input from all co-authors.
The authors declare that they have no conflict of interest.
We thank Tom Hill at Colorado State University for valuable suggestions and
providing the Anotop filters for our testing. Hassan Beydoun and Leif Jahn
provided comments on a draft of the paper. Comments provided by Gabor Vali, Benjamin Murray, and an anonymous referee during review significantly
improved this paper. This work was supported by the National Science
Foundation (CHE-1554941). Michael Polen was supported by a Graduate Research Fellowship
from the National Science Foundation.
Edited by: Mingjin Tang
Reviewed by: Benjamin Murray and one anonymous referee
Alstadt, V. J., Dawson, J. N., Losey, D. J., Sihvonen, S. K., and Freedman, M. A.: Heterogeneous Freezing of Carbon Nanotubes: A Model System for Pore Condensation and Freezing in the Atmosphere, J. Phys. Chem. A, 121, 8166–8175, https://doi.org/10.1021/acs.jpca.7b06359, 2017.
Archuleta, C. M., DeMott, P. J., and Kreidenweis, S. M.: Ice nucleation by surrogates for atmospheric mineral dust and mineral dust/sulfate particles at cirrus temperatures, Atmos. Chem. Phys., 5, 2617–2634, https://doi.org/10.5194/acp-5-2617-2005, 2005.
Augustin-Bauditz, S., Wex, H., Denjean, C., Hartmann, S., Schneider, J., Schmidt, S., Ebert, M., and Stratmann, F.: Laboratory-generated mixtures of mineral dust particles with biological substances: characterization of the particle mixing state and immersion freezing behavior, Atmos. Chem. Phys., 16, 5531–5543, https://doi.org/10.5194/acp-16-5531-2016, 2016.
Banfield, J. F. and Eggleton, R. A.: Analytical transmission electron microscope studies of plagioclase, muscovite, and K-feldspar weathering, Clay. Clay Miner., 38, 77–89, 1990.
Beydoun, H., Polen, M., and Sullivan, R. C.: Effect of particle surface area on ice active site densities retrieved from droplet freezing spectra, Atmos. Chem. Phys., 16, 13359–13378, https://doi.org/10.5194/acp-16-13359-2016, 2016.
Beydoun, H., Polen, M., and Sullivan, R. C.: A new multicomponent heterogeneous ice nucleation model and its application to Snomax bacterial particles and a Snomax-illite mineral particle mixture, Atmos. Chem. Phys., 17, 13545–13557, https://doi.org/10.5194/acp-17-13545-2017, 2017.
Bigg, E. K.: The formation of atmospheric ice crystals by the freezing of droplets, Q. J. Roy. Meteor. Soc., 79, 510–519, https://doi.org/10.1002/qj.49707934207, 1953.
Bithi, S. S. and Vanapalli, S. A.: Behavior of a train of droplets in a fluidic network with hydrodynamic traps, Biomicrofluidics, 4, 044110, https://doi.org/10.1063/1.3523053, 2010.
Boukellal, H., Selimović, Š., Jia, Y., Cristobal, G., and Fraden, S.: Simple, robust storage of drops and fluids in a microfluidic device, Lab Chip, 9, 331–338, https://doi.org/10.1039/B808579J, 2009.
Broadley, S. L., Murray, B. J., Herbert, R. J., Atkinson, J. D., Dobbie, S., Malkin, T. L., Condliffe, E., and Neve, L.: Immersion mode heterogeneous ice nucleation by an illite rich powder representative of atmospheric mineral dust, Atmos. Chem. Phys., 12, 287–307, https://doi.org/10.5194/acp-12-287-2012, 2012.
Budke, C. and Koop, T.: BINARY: an optical freezing array for assessing temperature and time dependence of heterogeneous ice nucleation, Atmos. Meas. Tech., 8, 689–703, https://doi.org/10.5194/amt-8-689-2015, 2015.
Campbell, J. M., Meldrum, F. C., and Christenson, H. K.: Is ice nucleation from supercooled water insensitive to surface roughness?, J. Phys. Chem. C, 119, 1164–1169, https://doi.org/10.1021/jp5113729, 2015.
Choi, S.-J., Kwon, T.-H., Im, H., Moon, D.-I., Baek, D. J., Seol, M.-L., Duarte, J. P., and Choi, Y.-K.: A Polydimethylsiloxane (PDMS) Sponge for the Selective Absorption of Oil from Water, ACS Appl. Mater. Inter., 3, 4552–4556, https://doi.org/10.1021/am201352w, 2011.
Conen, F., Morris, C. E., Leifeld, J., Yakutin, M. V., and Alewell, C.: Biological residues define the ice nucleation properties of soil dust, Atmos. Chem. Phys., 11, 9643–9648, https://doi.org/10.5194/acp-11-9643-2011, 2011.
Creamean, J. M., Suski, K. J., Rosenfeld, D., Cazorla, A., DeMott, P. J., Sullivan, R. C., White, A. B., Ralph, F. M., Minnis, P., Comstock, J. M., Tomlinson, J. M., and Prather, K. A.: Dust and Biological Aerosols from the Sahara and Asia Influence Precipitation in the Western U.S., Science, 339, 1572–1578, https://doi.org/10.1126/science.1227279, 2013.
Cziczo, D. J., Ladino, L., Boose, Y., Kanji, Z. A., Kupiszewski, P., Lance, S., Mertes, S., and Wex, H.: Measurements of Ice Nucleating Particles and Ice Residuals, Meteorol. Monogr., 58, 8.1–8.13, https://doi.org/10.1175/AMSMONOGRAPHS-D-16-0008.1, 2017.
DeMott, P. J., Prenni, A. J., Liu, X., Kreidenweis, S. M., Petters, M. D., Twohy, C. H., Richardson, M. S., Eidhammer, T., and Rogers, D. C.: Predicting global atmospheric ice nuclei distributions and their impacts on climate, P. Natl. Acad. Sci. USA, 107, 11217–22, https://doi.org/10.1073/pnas.0910818107, 2010.
DeMott, P. J., Hill, T. C. J., Petters, M. D., Bertram, A. K., Tobo, Y., Mason, R. H., Suski, K. J., McCluskey, C. S., Levin, E. J. T., Schill, G. P., Boose, Y., Rauker, A. M., Miller, A. J., Zaragoza, J., Rocci, K., Rothfuss, N. E., Taylor, H. P., Hader, J. D., Chou, C., Huffman, J. A., Pöschl, U., Prenni, A. J., and Kreidenweis, S. M.: Comparative measurements of ambient atmospheric concentrations of ice nucleating particles using multiple immersion freezing methods and a continuous flow diffusion chamber, Atmos. Chem. Phys., 17, 11227–11245, https://doi.org/10.5194/acp-17-11227-2017, 2017.
Diao, Y., Myerson, A. S., Hatton, T. A., and Trout, B. L.: Surface Design for Controlled Crystallization: The Role of Surface Chemistry and Nanoscale Pores in Heterogeneous Nucleation, Langmuir, 27, 5324–5334, https://doi.org/10.1021/la104351k, 2011.
Durant, A. J. and Shaw, R. A.: Evaporation freezing by contact nucleation inside-out, Geophys. Res. Lett., 32, 1–4, https://doi.org/10.1029/2005GL024175, 2005.
Durant, A. J., Shaw, R. A., Rose, W. I., Mi, Y., and Ernst, G. G. J.: Ice nucleation and overseeding of ice in volcanic clouds, J. Geophys. Res.-Atmos., 113, 1–13, https://doi.org/10.1029/2007JD009064, 2008.
Emersic, C., Connolly, P. J., Boult, S., Campana, M., and Li, Z.: Investigating the discrepancy between wet-suspension- and dry-dispersion-derived ice nucleation efficiency of mineral particles, Atmos. Chem. Phys., 15, 11311–11326, https://doi.org/10.5194/acp-15-11311-2015, 2015.
Findenegg, G. H., Jähnert, S., Akcakayiran, D., and Schreiber, A.: Freezing and Melting of Water Confined in Silica Nanopores, Chem. Phys. Chem., 9, 2651–2659, https://doi.org/10.1002/cphc.200800616, 2008.
Fitzner, M., Sosso, G. C., Cox, S. J., and Michaelides, A.: The Many Faces of Heterogeneous Ice Nucleation: Interplay Between Surface Morphology and Hydrophobicity, J. Am. Chem. Soc., 137, 13658–13669, https://doi.org/10.1021/jacs.5b08748, 2015.
Fornea, A. P., Brooks, S. D., Dooley, J. B., and Saha, A.: Heterogeneous freezing of ice on atmospheric aerosols containing ash, soot, and soil, J. Geophys. Res.-Atmos., 114, 1–12, https://doi.org/10.1029/2009JD011958, 2009.
Fukuta, N.: A Study of the Mechanism of Contact Ice Nucleation, J. Atmos. Sci., 32, 1597–1603, https://doi.org/10.1175/1520-0469(1975)032<1597:ASOTMO>2.0.CO;2, 1975.
Garcia, E., Hill, T. C. J., Prenni, A. J., DeMott, P. J., Franc, G. D., and Kreidenweis, S. M.: Biogenic ice nuclei in boundary layer air over two U.S. high plains agricultural regions, J. Geophys. Res.-Atmos., 117, 1–12, https://doi.org/10.1029/2012JD018343, 2012.
Gurganus, C. W., Charnawskas, J. C., Kostinski, A. B., and Shaw, R. A.: Nucleation at the Contact Line Observed on Nanotextured Surfaces, Phys. Rev. Lett., 113, 235701, https://doi.org/10.1103/PhysRevLett.113.235701, 2014.
Hader, J. D., Wright, T. P., and Petters, M. D.: Contribution of pollen to atmospheric ice nuclei concentrations, Atmos. Chem. Phys., 14, 5433–5449, https://doi.org/10.5194/acp-14-5433-2014, 2014.
Harrison, A. D., Whale, T. F., Carpenter, M. A., Holden, M. A., Neve, L., O'Sullivan, D., Vergara Temprado, J., and Murray, B. J.: Not all feldspars are equal: a survey of ice nucleating properties across the feldspar group of minerals, Atmos. Chem. Phys., 16, 10927–10940, https://doi.org/10.5194/acp-16-10927-2016, 2016.
Häusler, T., Witek, L., Felgitsch, L., Hitzenberger, R., and Grothe, H.: Freezing on a Chip – A New Approach to Determine Heterogeneous Ice Nucleation of Micrometer-Sized Water Droplets, Atmosphere, 9, 140, https://doi.org/10.3390/atmos9040140, 2018.
Hill, T. C. J., Moffett, B. F., DeMott, P. J., Georgakopoulos, D. G., Stump, W. L., and Franc, G. D.: Measurement of ice nucleation-active bacteria on plants and in precipitation by quantitative PCR, Appl. Environ. Microbiol., 80, 1256–1267, https://doi.org/10.1128/AEM.02967-13, 2014.
Hill, T. C. J., DeMott, P. J., Tobo, Y., Fröhlich-Nowoisky, J., Moffett, B. F., Franc, G. D., and Kreidenweis, S. M.: Sources of organic ice nucleating particles in soils, Atmos. Chem. Phys., 16, 7195–7211, https://doi.org/10.5194/acp-16-7195-2016, 2016.
Hiranuma, N., Augustin-Bauditz, S., Bingemer, H., Budke, C., Curtius, J., Danielczok, A., Diehl, K., Dreischmeier, K., Ebert, M., Frank, F., Hoffmann, N., Kandler, K., Kiselev, A., Koop, T., Leisner, T., Möhler, O., Nillius, B., Peckhaus, A., Rose, D., Weinbruch, S., Wex, H., Boose, Y., DeMott, P. J., Hader, J. D., Hill, T. C. J., Kanji, Z. A., Kulkarni, G., Levin, E. J. T., McCluskey, C. S., Murakami, M., Murray, B. J., Niedermeier, D., Petters, M. D., O'Sullivan, D., Saito, A., Schill, G. P., Tajiri, T., Tolbert, M. A., Welti, A., Whale, T. F., Wright, T. P., and Yamashita, K.: A comprehensive laboratory study on the immersion freezing behavior of illite NX particles: a comparison of 17 ice nucleation measurement techniques, Atmos. Chem. Phys., 15, 2489–2518, https://doi.org/10.5194/acp-15-2489-2015, 2015.
Holdren, G. R. and Berner, R. A.: Mechanism of feldspar weathering – I. Experimental studies, Geochim. Cosmochim. Ac., 43, 1161–1171, https://doi.org/10.1016/0016-7037(79)90109-1, 1979.
Hoose, C. and Möhler, O.: Heterogeneous ice nucleation on atmospheric aerosols: a review of results from laboratory experiments, Atmos. Chem. Phys., 12, 9817–9854, https://doi.org/10.5194/acp-12-9817-2012, 2012.
Iannone, R., Chernoff, D. I., Pringle, A., Martin, S. T., and Bertram, A. K.: The ice nucleation ability of one of the most abundant types of fungal spores found in the atmosphere, Atmos. Chem. Phys., 11, 1191–1201, https://doi.org/10.5194/acp-11-1191-2011, 2011.
Inada, T., Tomita, H., and Koyama, T.: Ice nucleation in water droplets on glass surfaces: From micro- to macro-scale, Int. J. Refrig., 40, 294–301, https://doi.org/10.1016/j.ijrefrig.2013.11.024, 2014.
Jung, S., Tiwari, M. K., and Poulikakos, D.: Frost halos from supercooled water droplets, P. Natl. Acad. Sci. USA, 109, 16073–16078, https://doi.org/10.1073/pnas.1206121109, 2012.
Kiselev, A., Bachmann, F., Pedevilla, P., Cox, S. J., Michaelides, A., Gerthsen, D., and Leisner, T.: Active sites in heterogeneous ice nucleation – the example of K-rich feldspars, Science, 355, 367–371, https://doi.org/10.1126/science.aai8034, 2017.
Koop, T. and Murray, B. J.: A physically constrained classical description of the homogeneous nucleation of ice in water, J. Chem. Phys., 145, 211915, https://doi.org/10.1063/1.4962355, 2016.
Koop, T., Ng, H. P., Molina, L. T., and Molina, M. J.: A New Optical Technique to Study Aerosol Phase Transitions: The Nucleation of Ice from H2SO4 Aerosols, J. Phys. Chem. A, 102, 8924–8931, https://doi.org/10.1021/jp9828078, 1998.
Kumar, A., Marcolli, C., Luo, B., and Peter, T.: Ice nucleation activity of silicates and aluminosilicates in pure water and aqueous solutions – Part 1: The K-feldspar microcline, Atmos. Chem. Phys., 18, 7057–7079, https://doi.org/10.5194/acp-18-7057-2018, 2018.
Li, K., Xu, S., Shi, W., He, M., Li, H., Li, S., Zhou, X., Wang, J., and Song, Y.: Investigating the effects of solid surfaces on Ice nucleation, Langmuir, 28, 10749–10754, https://doi.org/10.1021/la3014915, 2012.
Lo, C.-W., Sahoo, V., and Lu, M.-C.: Control of Ice Formation, ACS Nano, 11, 2665–2674, https://doi.org/10.1021/acsnano.6b07348, 2017.
Lohmann, U. and Feichter, J.: Global indirect aerosol effects: a review, Atmos. Chem. Phys., 5, 715–737, https://doi.org/10.5194/acp-5-715-2005, 2005.
Marcolli, C., Gedamke, S., Peter, T., and Zobrist, B.: Efficiency of immersion mode ice nucleation on surrogates of mineral dust, Atmos. Chem. Phys., 7, 5081–5091, https://doi.org/10.5194/acp-7-5081-2007, 2007.
Marcolli, C., Nagare, B., Welti, A., and Lohmann, U.: Ice nucleation efficiency of AgI: review and new insights, Atmos. Chem. Phys., 16, 8915–8937, https://doi.org/10.5194/acp-16-8915-2016, 2016.
Mason, R. H., Chou, C., McCluskey, C. S., Levin, E. J. T., Schiller, C. L., Hill, T. C. J., Huffman, J. A., DeMott, P. J., and Bertram, A. K.: The micro-orifice uniform deposit impactor-droplet freezing technique (MOUDI-DFT) for measuring concentrations of ice nucleating particles as a function of size: improvements and initial validation, Atmos. Meas. Tech., 8, 2449–2462, https://doi.org/10.5194/amt-8-2449-2015, 2015.
Mülmenstädt, J., Sourdeval, O., Delanoë, J., and Quaas, J.: Frequency of occurrence of rain from liquid-, mixed-, and ice-phase clouds derived from A-Train satellite retrievals, Geophys. Res. Lett., 42, 6502–6509, https://doi.org/10.1002/2015GL064604, 2015.
Murray, B. J., Broadley, S. L., Wilson, T. W., Bull, S. J., Wills, R. H., Christenson, H. K., and Murray, E. J.: Kinetics of the homogeneous freezing of water, Phys. Chem. Chem. Phys., 12, 10380–10387, https://doi.org/10.1039/c003297b, 2010.
Murray, B. J., Broadley, S. L., Wilson, T. W., Atkinson, J. D., and Wills, R. H.: Heterogeneous freezing of water droplets containing kaolinite particles, Atmos. Chem. Phys., 11, 4191–4207, https://doi.org/10.5194/acp-11-4191-2011, 2011.
Murray, B. J., O'Sullivan, D., Atkinson, J. D., and Webb, M. E.: Ice nucleation by particles immersed in supercooled cloud droplets, Chem. Soc. Rev., 41, 6519–54, https://doi.org/10.1039/c2cs35200a, 2012.
O, K.-T. and Wood, R.: Exploring an approximation for the homogeneous freezing temperature of water droplets, Atmos. Chem. Phys., 16, 7239–7249, https://doi.org/10.5194/acp-16-7239-2016, 2016.
O'Sullivan, D., Murray, B. J., Ross, J. F., Whale, T. F., Price, H. C., Atkinson, J. D., Umo, N. S., and Webb, M. E.: The relevance of nanoscale biological fragments for ice nucleation in clouds, Sci. Rep., 5, 8082, https://doi.org/10.1038/srep08082, 2015.
Peckhaus, A., Kiselev, A., Hiron, T., Ebert, M., and Leisner, T.: A comparative study of K-rich and Na/Ca-rich feldspar ice-nucleating particles in a nanoliter droplet freezing assay, Atmos. Chem. Phys., 16, 11477–11496, https://doi.org/10.5194/acp-16-11477-2016, 2016.
Petters, M. D. and Wright, T. P.: Revisiting ice nucleation from precipitation samples, Geophys. Res. Lett., 42, 8758–8766, https://doi.org/10.1002/2015GL065733, 2015.
Polen, M., Lawlis, E., and Sullivan, R. C.: The unstable ice nucleation properties of Snomax® bacterial particles, J. Geophys. Res.-Atmos., 121, 11666–11678, https://doi.org/10.1002/2016JD025251, 2016.
Price, H. C., Baustian, K. J., McQuaid, J. B., Blyth, A., Bower, K. N., Choularton, T., Cotton, R. J., Cui, Z., Field, P. R., Gallagher, M., Hawker, R., Merrington, A., Miltenberger, A., Neely III, R. R., Parker, S. T., Rosenberg, P. D., Taylor, J. W., Trembath, J., Vergara-Temprado, J., Whale, T. F., Wilson, T. W., Young, G., and Murray, B. J.: Atmospheric Ice-Nucleating Particles in the Dusty Tropical Atlantic, J. Geophys. Res.-Atmos., 123, 2175–2193, https://doi.org/10.1002/2017JD027560, 2018.
Pruppacher, H. R.: A New Look at Homogeneous Ice Nucleation in Supercooled Water Drops, J. Atmos. Sci., 52, 1924–1933, https://doi.org/10.1175/1520-0469(1995)052<1924:ANLAHI>2.0.CO;2, 1995.
Pummer, B. G., Bauer, H., Bernardi, J., Bleicher, S., and Grothe, H.: Suspendable macromolecules are responsible for ice nucleation activity of birch and conifer pollen, Atmos. Chem. Phys., 12, 2541–2550, https://doi.org/10.5194/acp-12-2541-2012, 2012.
Pummer, B. G., Budke, C., Augustin-Bauditz, S., Niedermeier, D., Felgitsch, L., Kampf, C. J., Huber, R. G., Liedl, K. R., Loerting, T., Moschen, T., Schauperl, M., Tollinger, M., Morris, C. E., Wex, H., Grothe, H., Pöschl, U., Koop, T., and Fröhlich-Nowoisky, J.: Ice nucleation by water-soluble macromolecules, Atmos. Chem. Phys., 15, 4077–4091, https://doi.org/10.5194/acp-15-4077-2015, 2015.
Reicher, N., Segev, L., and Rudich, Y.: The WeIzmann Supercooled Droplets Observation on a Microarray (WISDOM) and application for ambient dust, Atmos. Meas. Tech., 11, 233–248, https://doi.org/10.5194/amt-11-233-2018, 2018.
Rigg, Y. J., Alpert, P. A., and Knopf, D. A.: Immersion freezing of water and aqueous ammonium sulfate droplets initiated by humic-like substances as a function of water activity, Atmos. Chem. Phys., 13, 6603–6622, https://doi.org/10.5194/acp-13-6603-2013, 2013.
Stan, C. A., Schneider, G. F., Shevkoplyas, S. S., Hashimoto, M., Ibanescu, M., Wiley, B. J., and Whitesides, G. M.: A microfluidic apparatus for the study of ice nucleation in supercooled water drops, Lab Chip, 9, 2293–305, https://doi.org/10.1039/b906198c, 2009.
Sun, M., Bithi, S. S., and Vanapalli, S. A.: Microfluidic static droplet arrays with tuneable gradients in material composition, Lab Chip, 11, 3949–3952, https://doi.org/10.1039/c1lc20709a, 2011.
Tabazadeh, A., Djikaev, Y. S., and Reiss, H.: Surface crystallization of supercooled water in clouds, P. Natl. Acad. Sci., 99, 15873–15878, https://doi.org/10.1073/pnas.252640699, 2002.
Tarn, M. D., Sikora, S. N. F., Porter, G. C. E., O'Sullivan, D., Adams, M., Whale, T. F., Harrison, A. D., Vergara-Temprado, J., Wilson, T. W., Shim, J., and Murray, B. J.: The study of atmospheric ice-nucleating particles via microfluidically generated droplets, Microfluid. Nanofluid., 22, 52, https://doi.org/10.1007/s10404-018-2069-x, 2018.
Thomas, C., Lampert, D., and Reible, D.: Remedy performance monitoring at contaminated sediment sites using profiling solid phase microextraction (SPME) polydimethylsiloxane (PDMS) fibers, Environ. Sci. Process. Impacts, 16, 445–452, https://doi.org/10.1039/C3EM00695F, 2014.
Tobo, Y.: An improved approach for measuring immersion freezing in large droplets over a wide temperature range, Sci. Rep., 6, 32930, https://doi.org/10.1038/srep32930, 2016.
Umo, N. S., Murray, B. J., Baeza-Romero, M. T., Jones, J. M., Lea-Langton, A. R., Malkin, T. L., O'Sullivan, D., Neve, L., Plane, J. M. C., and Williams, A.: Ice nucleation by combustion ash particles at conditions relevant to mixed-phase clouds, Atmos. Chem. Phys., 15, 5195–5210, https://doi.org/10.5194/acp-15-5195-2015, 2015.
Vali, G.: Quantitative Evaluation of Experimental Results an the Heterogeneous Freezing Nucleation of Supercooled Liquids, J. Atmos. Sci., 28, 402–409, https://doi.org/10.1175/1520-0469(1971)028<0402:QEOERA>2.0.CO;2, 1971.
Vali, G.: Ice Nucleation – Theory: A Tutorial, NCAR/ASP 1999 Summer Colloq., 1–22, available at: http://www-das.uwyo.edu/~vali/nucl_th.pdf (last access: 18 September 2019), 1999.
Vali, G.: Repeatability and randomness in heterogeneous freezing nucleation, Atmos. Chem. Phys., 8, 5017–5031, https://doi.org/10.5194/acp-8-5017-2008, 2008.
Vali, G.: Interpretation of freezing nucleation experiments: singular and stochastic; sites and surfaces, Atmos. Chem. Phys., 14, 5271–5294, https://doi.org/10.5194/acp-14-5271-2014, 2014.
Vali, G. and Stansbury, E. J.: Time-Dependent Characteristics of the Heterogeneous Nucleation of Ice, Can. J. Phys., 44, 477–502, https://doi.org/10.1139/p66-044, 1966.
Varanasi, K. K., Deng, T., Smith, J. D., Hsu, M., and Bhate, N.: Frost formation and ice adhesion on superhydrophobic surfaces, Appl. Phys. Lett., 97, 234102, https://doi.org/10.1063/1.3524513, 2010.
Vergara-Temprado, J., Holden, M. A., Orton, T. R., O'Sullivan, D., Umo, N. S., Browse, J., Reddington, C., Baeza-Romero, M. T., Jones, J. M., Lea-Langton, A., Williams, A., Carslaw, K. S., and Murray, B. J.: Is Black Carbon an Unimportant Ice-Nucleating Particle in Mixed-Phase Clouds?, J. Geophys. Res.-Atmos., 123, 4273–4283, https://doi.org/10.1002/2017JD027831, 2018a.
Vergara-Temprado, J., Miltenberger, A. K., Furtado, K., Grosvenor, D. P., Shipway, B. J., Hill, A. A., Wilkinson, J. M., Field, P. R., Murray, B. J., and Carslaw, K. S.: Strong control of Southern Ocean cloud reflectivity by ice-nucleating particles, P. Natl. Acad. Sci., 115, 2687–2692, https://doi.org/10.1073/pnas.1721627115, 2018b.
Wang, B., Knopf, D. A., China, S., Arey, B. W., Harder, T. H., Gilles, M. K., and Laskin, A.: Direct observation of ice nucleation events on individual atmospheric particles, Phys. Chem. Chem. Phys., 18, 29721–29731, https://doi.org/10.1039/C6CP05253C, 2016.
Wex, H., Augustin-Bauditz, S., Boose, Y., Budke, C., Curtius, J., Diehl, K., Dreyer, A., Frank, F., Hartmann, S., Hiranuma, N., Jantsch, E., Kanji, Z. A., Kiselev, A., Koop, T., Möhler, O., Niedermeier, D., Nillius, B., Rösch, M., Rose, D., Schmidt, C., Steinke, I., and Stratmann, F.: Intercomparing different devices for the investigation of ice nucleating particles using Snomax® as test substance, Atmos. Chem. Phys., 15, 1463–1485, https://doi.org/10.5194/acp-15-1463-2015, 2015.
Whale, T. F., Murray, B. J., O'Sullivan, D., Wilson, T. W., Umo, N. S., Baustian, K. J., Atkinson, J. D., Workneh, D. A., and Morris, G. J.: A technique for quantifying heterogeneous ice nucleation in microlitre supercooled water droplets, Atmos. Meas. Tech., 8, 2437–2447, https://doi.org/10.5194/amt-8-2437-2015, 2015.
Wheeler, M. J., Mason, R. H., Steunenberg, K., Wagstaff, M., Chou, C., and Bertram, A. K.: Immersion Freezing of Supermicron Mineral Dust Particles: Freezing Results, Testing Different Schemes for Describing Ice Nucleation, and Ice Nucleation Active Site Densities, J. Phys. Chem. A, 119, 4358–4372, https://doi.org/10.1021/jp507875q, 2015.
Wilson, T. W., Ladino, L. A., Alpert, P. A., Breckels, M. N., Brooks, I. M., Browse, J., Burrows, S. M., Carslaw, K. S., Huffman, J. A., Judd, C., Kilthau, W. P., Mason, R. H., McFiggans, G., Miller, L. A., Nájera, J. J., Polishchuk, E., Rae, S., Schiller, C. L., Si, M., Temprado, J. V., Whale, T. F., Wong, J. P. S., Wurl, O., Yakobi-Hancock, J. D., Abbatt, J. P. D., Aller, J. Y., Bertram, A. K., Knopf, D. A., and Murray, B. J.: A marine biogenic source of atmospheric ice-nucleating particles, Nature, 525, 234–238, https://doi.org/10.1038/nature14986, 2015.
Wright, T. P. and Petters, M. D.: The role of time in heterogeneous freezing nucleation, J. Geophys. Res.-Atmos., 118, 3731–3743, https://doi.org/10.1002/jgrd.50365, 2013.
Wright, T. P., Petters, M. D., Hader, J. D., Morton, T., and Holder, A. L.: Minimal cooling rate dependence of ice nuclei activity in the immersion mode, J. Geophys. Res.-Atmos., 118, 1053–10543, https://doi.org/10.1002/jgrd.50810, 2013.
Yin, Y., Wurzler, S., Levin, Z., and Reisin, T. G.: Interactions of mineral dust particles and clouds: Effects on precipitation and cloud optical properties, J. Geophys. Res.-Atmos., 107, 1–14, https://doi.org/10.1029/2001JD001544, 2002.
Zobrist, B., Marcolli, C., Peter, T., and Koop, T.: Heterogeneous Ice Nucleation in Aqueous Solutions: the Role of Water Activity, J. Phys. Chem. A, 112, 3965–3975, https://doi.org/10.1021/jp7112208, 2008.
Zolles, T., Burkart, J., Häusler, T., Pummer, B., Hitzenberger, R., and Grothe, H.: Identification of Ice Nucleation Active Sites on Feldspar Dust Particles, J. Phys. Chem. A, 119, 2692–2700, https://doi.org/10.1021/jp509839x, 2015.