the Creative Commons Attribution 4.0 License.
the Creative Commons Attribution 4.0 License.
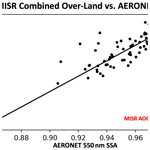
The new MISR research aerosol retrieval algorithm: a multi-angle, multi-spectral, bounded-variable least squares retrieval of aerosol particle properties over both land and water
James A. Limbacher
Ralph A. Kahn
Jaehwa Lee
Launched in December 1999, NASA's Multi-angle Imaging SpectroRadiometer (MISR) has given researchers the ability to observe the Earth from nine different views for the last 22 years. Among the many advancements that have since resulted from the launch of MISR is progress in the retrieval of aerosols from passive space-based remote sensing. The MISR operational standard aerosol (SA) retrieval algorithm has been refined several times over the last 20 years, resulting in significant improvements to spatial resolution (now 4.4 km) and aerosol particle properties. However, the MISR SA still suffers from large biases in retrieved aerosol optical depth (AOD) as aerosol loading increases. Here, we present a new MISR research aerosol (RA) retrieval algorithm that utilizes over-land surface reflectance data from the Multi-Angle Implementation of Atmospheric Correction (MAIAC) to address these biases. This new over-land and over-water algorithm produces a self-consistent aerosol and surface retrieval when aerosol loading is low (AOD <0.75); this is combined with a prescribed surface algorithm using a bounded-variable least squares solver when aerosol loading is elevated (AOD >1.5). The two algorithms (prescribed + retrieved surface) are then merged as part of our combined surface retrieval algorithm. Results are compared with AErosol RObotic NETwork (AERONET) validation sun-photometer direct-sun + almucantar inversion retrievals.
Over land, with AERONET AOD (550 nm) direct-sun observations as the standard, the root mean squared error (RMSE) of the MISR RA combined retrieval (n=11563) is 0.084, with a correlation coefficient (r) of 0.935 and expected error of ). For MISR RA retrieved AOD >0.5 (n=664), we report an Ångström exponent (ANG) RMSE of ∼0.35, with a correlation coefficient of 0.844. Retrievals of ANG, fine-mode fraction (FMF), and single-scattering albedo (SSA) improve as retrieved AOD increases. For AOD >1.5 (n=66), FMF RMSE is <0.09 with correlation >0.95, and SSA RMSE is 0.015 with a correlation coefficient of ∼0.75.
Over water, comparing AERONET AOD to the MISR RA combined retrieval (n=4596), MISR RA RMSE is 0.063 and r is 0.935, with an expected error of . ANG sensitivity is excellent when MISR RA reported AOD >0.5 (n=188), with an RMSE of 0.27 and r=0.89. Due to a lack of coincidences with AOD >1 (n=21), our conclusions about MISR RA high-AOD particle property retrievals over water are less robust (FMF RMSE =0.155 and r=0.94, whereas SSA RMSE =0.010 and r=0.50).
In general, better aerosol particle property constraints can be made at lower AOD over water compared to our over-land retrievals. It is clear from the results presented that the new MISR RA has quantitative sensitivity to FMF and SSA (and qualitative sensitivity to non-sphericity) when retrieved AOD exceeds 1, with qualitative sensitivity to aerosol type at lower AOD, while also eliminating the AOD bias found in the MISR SA at higher AODs. These results also demonstrate the advantage of using a prescribed surface when aerosol loading is elevated.
- Article
(8355 KB) - Full-text XML
-
Supplement
(2243 KB) - BibTeX
- EndNote
The first of three Along-Track Scanning Radiometer (ATSR) instruments was launched in July 1991, bringing to the attention of the research community some of what multi-angle remote sensing offers (e.g., Flowerdew and Haigh, 1995; North et al., 1999). As NASA began to develop its Earth Observing System in the late 1980s, it also chose to pursue a multi-angle imaging approach by selecting the Multi-angle Imaging SpectroRadiometer (MISR) as one of five instruments to be launched on its flagship Terra spacecraft. MISR was designed to image Earth's surface and atmosphere at nine angles (70.5, 60.0, 45.6, 26.1∘ in the forward and aft directions along the flight path, plus nadir) in each of four wavelengths (centered at 446, 558, 672, and 866 nm; Diner et al., 1998). Beginning in February 2000, MISR has since acquired more than 2 decades of approximately once-weekly, global data.
The initial concept for the MISR aerosol and over-land surface retrieval algorithm was developed by Diner and Martonchik (1984a, b, 1985). The method is inherently multi-angle; it assumes that aerosol amount and properties are constant over a retrieval region and uses empirical orthogonal functions (EOFs) in view angle to characterize the directional surface bidirectional reflectance factor (BRF) contributions to the top-of-atmosphere BRF. Implementation of this approach in the operational MISR standard aerosol (SA) retrieval algorithm is described by Martonchik et al. (1998, 2002, 2009). Substantial advances to the SA involved adding a separate process that assumes the shape of the surface angular BRF is independent of wavelength (Diner et al., 2005) and reducing the size of the retrieval regions from 17.6 to 4.4 km (Garay et al., 2020). Still, even with the upgrades described above, the MISR SA continues to show a significant negative bias in AOD when aerosol loading is elevated (Kahn et al., 2005, 2010; Kahn and Gaitley, 2015). In addition to this bias in AOD, it is also likely that SA-retrieved aerosol particle properties are negatively impacted at high AODs over land, as errors in the retrieved surface BRF will likely manifest themselves as errors in both AOD and aerosol type.
Among most EOS-era satellite imagers, aerosol property information is a unique contribution the MISR instrument can make. As such, a research aerosol (RA) retrieval algorithm was developed in parallel with the SA, focused primarily on deriving as much information as possible about particle microphysical properties (e.g., Kahn et al., 2001; Limbacher and Kahn, 2014, 2019). This means the RA includes a broader range of particle optical model options in the algorithm climatology than the MISR SA. It results in more subtle particle property distinctions under favorable retrieval conditions, for example, in smoke and volcanic plumes, when the AOD is sufficiently high (e.g., Flower and Kahn, 2020; Junghenn Noyes et al., 2020). However, especially at low AOD, when particle type discrimination is poorer, having a larger particle-type climatology can increase AOD uncertainty.
Previously, in the RA, the surface was characterized either by Fresnel-reflecting dark water with whitecaps and under-light contributions or by a more complex surface specified from external sources (Kahn et al., 2001; Chen et al., 2008). The MISR RA has also provided validation and suggested upgrades to the SA. Initial sensitivity studies established that three to five bins in particle size, two to four bins in particle single-scattering albedo (SSA), and spherical vs. randomly oriented non-spherical particle properties could be distinguished from MISR data, provided the mid-visible aerosol optical depth (AOD) exceeds about 0.15–0.2 (Kahn et al., 1997, 1998, 2001; Kalashnikova and Kahn, 2006). A high bias in retrieved low AOD values, along with limitations in the MISR radiometric calibration, the algorithm climatology of particle optical models, and the surface assumptions in these early algorithms (Kahn et al., 2010) were subsequently addressed. The advances initially focused on over-water retrievals. They included modernizing the code, allowing for regional coverage with pixel-level (1.1 km) retrievals, and improving the particle optical models, along with better pixel selection, cloud screening, and uncertainty assessment (Limbacher and Kahn, 2014). The MISR radiometric calibration applied in the RA was revised based on empirical image analysis, aimed primarily at improving sensitivity to particle properties (Limbacher and Kahn, 2015). Further refinements included self-consistently retrieving aerosol and chlorophyll a over a dark ocean surface, further refining the MISR radiometric calibration to account for temporal degradation (Limbacher and Kahn, 2017), and extending these retrievals to deriving spectral surface albedo for shallow, turbid, and eutrophic water under a Lambertian water-leaving BRF assumption (Limbacher and Kahn, 2019).
The current paper takes a further step in the advancement of the MISR RA by incorporating over-land aerosol retrievals with the surface optical model either retrieved self-consistently within the algorithm or prescribed from the MODerate resolution Imaging SpectroRadiometer (MODIS) Multi-Angle Implementation of Atmospheric Correction (MAIAC) product (Lyapustin et al., 2018; Lyapustin and Wang, 2018). MAIAC accumulates MODIS observations over 4–16 d (depending on latitude) to produce multi-angle data for the surface retrieval and reports the bidirectional reflectance distribution function (BRDF) at 1 km spatial resolution. The current paper is organized as follows: Sect. 2 describes the RA over-land and over-water retrieval algorithms in detail for both the prescribed and retrieved surfaces. It introduces the bounded-variable least squares (BVLS) approach adopted for the prescribed surface version of the algorithm, a new retrieved surface aerosol algorithm (over both land and water), and modifications to the aerosol optical model climatology and other differences from earlier RA versions. The aerosol quantities reported here are AOD at 550 nm, fine-mode AOD fraction at 550 nm, coarse-mode effective radius (in micrometers), fine-mode effective radius (in micrometers), SSA at 550 nm, brown-smoke AOD fraction at 550 nm (analogous to SSA spectral slope), and non-spherical AOD fraction at 550 nm (Junghenn Noyes et al., 2020). Section 3 presents the results: detailed validation of the over-land and over-water MISR RA retrievals against coincident AERONET sun-photometer data and inversions. Conclusions are given in Sect. 4.
2.1 MISR RA general description
The current MISR RA, presented in this paper, is essentially composed of two sets of retrieval algorithms, both of which derive aerosol loading and properties at 1.1 km resolution: the retrieved surface algorithm retrieves the Lambertian water-leaving radiance over water and applies a spectrally invariant angular shape similarity assumption to derive the surface BRF over land (Diner et al., 2005), whereas the other algorithm prescribes the surface BRF for both land (from MODIS-MAIAC) and water (using a static set of remote sensing BRFs). The MISR top-of-atmosphere (TOA) BRFs used for this study are identical to the set of MISR BRFs used in our 2019 turbid water aerosol retrieval paper (Limbacher and Kahn, 2019) and represent 4 years of MISR data interspersed between 2000 and 2016 (over select AERONET direct-sun aerosol validation sites; Holben et al., 1998).
Modified TOA BRFs (with no solar angle dependence) are computed from the MISR radiance data according to the following:
where Lλ,c represents the observed TOA radiance (W m−2 µm−1 sr−1) in band λ and camera c, D is the Earth–Sun distance at the time of observation in astronomical units (AU), and is the exo-atmospheric solar irradiance at 1 AU (W m−2 µm−1). We then correct these TOA BRFs for the following: gas absorption, out-of-band light, stray light from instrumental artifacts, flat fielding, and temporal calibration trends (Limbacher and Kahn, 2015, 2017, 2019). Once the TOA BRFs have been corrected for these artifacts, MODIS-MAIAC surface BRF BRDF kernels (Lyapustin et al., 2018; Lyapustin and Wang, 2018) are linearly interpolated temporally to the MISR overpass date. These MAIAC data and the corresponding MISR data are then gridded to a static grid identical for each orbit at the native MISR 1.1 km resolution. Additionally, we use MISR's digital elevation model (DEM) surface height data from the MISR ancillary geographic product (AGP) and convert to surface pressure (at 1.1 km spatial resolution). To create the validation dataset used in the current paper, gridding is performed instead at 1 km resolution on a 48×48 pixel box centered on each AERONET site and ingested into the RA. Over land, where MAIAC BRDF kernels are available, the algorithm then converts MAIAC BRDF kernels to surface BRF for each of MISR's 36 channels (4 bands × 9 cameras), adjusting to ensure that the surface BRF at any angle never exceeds 3 times the albedo (for a given band) or drops below 33 % of the albedo for a given band, similar to constraints placed on MAIAC surface BRFs from Lyapustin et al. (2012). Over water, the prescribed remote sensing BRF, similar to a surface albedo if one ignores sun glint, is assumed to be Lambertian ([0.0257, 0.00668, 0.00093, 0.0000635] for the blue, green, red, and NIR bands, respectively) once glint is subtracted (Limbacher and Kahn, 2017). The algorithm then runs both sets of retrievals for each scene, one with a prescribed surface, using MAIAC over land and a fixed surface BRF over water, and one for which the surface BRF is retrieved. Using a newly created land–water mask derived from the MISR retrieved surface algorithm itself, we then consolidate the output (AOD, aerosol properties, cost function, etc.) from the four (retrieved + prescribed, land + water) retrievals into two (prescribed and retrieved surface).
Like most operational aerosol retrieval algorithms, the MISR RA uses a pre-built lookup table (LUT) of radiative transfer (RT) output in lieu of running RT code on the fly. Previous versions of the MISR RA relied on either modified linear mixing (Abdou et al., 1997) or external mixing of the phase functions (e.g., Limbacher and Kahn, 2019) to create aerosol mixture analogs from component particle optical analogs represented in our LUT. Although both approaches tend to yield more accurate modeled TOA BRFs at higher AOD, external mixing requires the generation of massive LUTs containing thousands of mixtures to fully account for the range of aerosol properties found in nature, and modified linear mixing requires a significant computational cost to generate reasonably accurate upwelling radiances. To improve our sensitivity to aerosol type, we have built a new LUT of aerosol model components (Table 1) that when linearly mixed with each other should more accurately account for the variability of aerosols seen in nature. This new component LUT contains TOA modeled BRF data as a function of spectral band, solar and viewing geometry, AOD, aerosol optical model (or component), surface pressure (for over-land retrievals), and prescribed 10 m wind speed (for over-water retrievals). The 6-hourly wind speeds are obtained from CCMP v2.0 data (Mears et al., 2019) and are spatially and temporally interpolated to the MISR domain and overpass time. The LUT values are interpolated during the retrieval process to the appropriate solar and viewing geometry, surface pressure, and wind speed.
Table 1Microphysical and optical properties of the new RA aerosol component climatology. Column 1 represents the component number, column 2 describes the aerosol analogs, and columns 3–7 represent minimum radius, maximum radius, lognormal characteristic radius, lognormal characteristic width, and effective radius (respectively). Column 8 is the Ångström exponent (calculated using all four MISR bands at 446–867 nm), column 9 is 550 nm single-scattering albedo (SSA), and the last column is the absorption Ångström exponent (AAE, calculated using all four MISR bands at 446–867 nm). Spherical aerosol component optical properties are modeled according to Mie theory, and all components are modeled with a lognormal particle size distribution. BlS corresponds to our black-smoke optical analogs, and BrS corresponds to our brown-smoke optical analogs. Red-colored rows correspond to models used only in the prescribed surface retrievals, whereas the one blue-colored row corresponds to the model only used by the retrieved surface aerosol retrieval. Purple-colored rows correspond to models used in both algorithms.
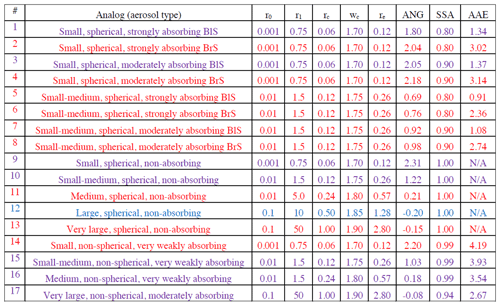
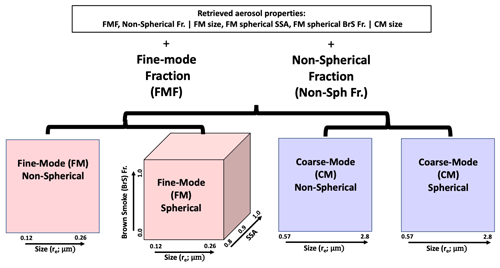
Figure 1The pink square on the left shows the bins corresponding to our fine-mode (FM) non-spherical aerosol models; the pink cube in the center left demonstrates how our 10 fine-mode spherical components are organized onto a rectangular grid. The center right blue square shows the two size bins for the coarse-mode non-spherical components, whereas the blue square on the right shows the same two size bins for our coarse-mode spherical components. These four discretized grids are then used to additionally retrieve the fine-mode fraction (FMF) and non-spherical fraction (both at 550 nm).
Because the two sets of aerosol retrieval algorithms diverge from this point, Sect. 2.1.1 describes the prescribed surface algorithm (PSA) and Sect. 2.1.2 delves into the retrieved surface algorithm (RSA).
2.1.1 MISR RA prescribed surface algorithm (PSA) using bounded-variable least squares (BVLS)
As the name suggests, the MISR RA prescribed surface algorithm requires external data on both surface angular spectral BRF and surface albedo for each individual MISR pixel. The process is summarized as Fig. S1 in the Supplement. Over water, we assume that the remote sensing BRF is Lambertian (once glint is subtracted), with the prescribed remote sensing BRFs given in Sect. 2.1. Because we do not use an over-water surface BRF database (analogous to MAIAC over land), our over-water prescribed surface results will likely be prone to error when aerosol loading is low. However, as described in Sect. 2.1.3 below, the combined surface algorithm addresses this limitation. Over land, the spectral albedo and angular dependence come from MAIAC data that are bias-corrected to remove artifacts that can originate in part from differences between the MISR and MODIS spectral band passes. A simple linear model was used for surface BRF (and albedo) corrections in each MISR band, with the following slopes (m) and offsets (b) used for the blue, green, red, and NIR bands, respectively (; ). These coefficients were identified by comparing RSA surface albedos (Sect. 2.1.2) with the PSA albedos from MAIAC in regions where the MISR-retrieved surface RA AOD agreed well with AERONET AOD and AERONET AOD <0.2. The fact that this bias correction was not sufficient to completely remove the AOD bias seen in the prescribed surface retrieval over land (especially at AODs <0.20) indicates that a camera-by-camera correction should probably be used in the future. However, because the primary focus of the prescribed surface aerosol retrieval is to improve our sensitivity to AOD and aerosol properties when aerosol loading is elevated (generally >0.75), we are not as concerned about the results of this retrieval when aerosol loading is low.
As our sensitivity to aerosol particle properties should be enhanced when optical loading is high, specifically because we are prescribing the surface BRF, the discrete set of mixtures used by the retrieved surface algorithm (Sect. 2.1.2) might be insufficient to describe the variability of aerosols seen in nature. Instead, we convert our component LUT (Table 1) into four regular grids composed of 10 fine-mode (FM) components and 4 coarse-mode components (as shown in Fig. 1). Rather than retrieve the non-spherical fraction independently for the fine and coarse modes, we instead retrieve the total non-spherical fraction for the combined fine + coarse modes. For our fine-mode spherical analogs, we include five fine-mode particles in each of two size distributions, with 550 nm SSA values of 0.8 0.9, and 1.0, as well as flat (black-smoke or BlS analog) and steep (brown-smoke or BrS analog) SSA spectral dependence. Because we retrieve the total non-spherical fraction, we also include a separate grid containing two fine-mode non-spherical aerosol models with the same size distributions as our fine-mode spherical analogs.
Two coarse-mode grids are also created, one corresponding to spherical aerosol (at 0.57 and 2.8 µm effective radius) and one corresponding to non-spherical aerosol (with the same size bins). All told, the algorithm retrieves 550 nm AOD and the following six pieces of information related to aerosol microphysical and optical properties: 550 nm fine-mode fraction (FMF), 550 nm non-spherical fraction, coarse-mode size (re; µm), fine-mode size (re; µm), 550 nm fine-mode spherical SSA, and 550 nm fine-mode spherical BrS fraction.
Once we have converted our component LUT into four regular grids (fine and coarse grids, spherical and non-spherical grids), the algorithm then needs a starting point to begin iterating towards a solution. This initial guess is set to the following: AOD =0.10, FMF =0.8, coarse-mode size = 1.28 µm, non-spherical fraction (fraction of aerosol extinction due to non-spherical aerosol) 0.2, fine-mode size 0.1202 µm, fine-mode SSA = 0.9985, and fine-mode spherical BrS fraction 0.00015. The algorithm then interpolates the LUTs separately before linearly combining the modeled fine and coarse (and spherical + non-spherical) grids. For a given solution vector (AOD + aerosol properties), we generate 36 TOA modeled BRFs , defined as
Here, BRFPath represents the modeled, interpolated path BRF, which is radiation that does not interact with the surface. To simplify the over-water algorithm, we also embed Fresnel reflection and whitecaps into this term. We estimate the TOA surface-reflected radiation as the normalized bottom-of-atmosphere downward irradiance multiplied by the azimuthally averaged surface-to-camera transmittance (TTλ,c) multiplied by the surface BRF (BRFSurf). We assume that the multiply reflected radiation can be accounted for with the normalization (1-sλ×Aλ), where sλ represents the effective atmospheric backscatter and Aλ represents the surface albedo. We recognize that this is only an approximation to account for multiple reflections of light off the surface.
We then calculate the derivatives of Eq. (2) with respect to all seven aerosol-related parameters and set up our linear system of equations. The weighted linear system of equations can be presented as
where ΔPar1 represents the change in our retrieved first parameter (AOD) from the last guess, and ΔPar7 represents the change in our retrieved seventh parameter (fine-mode spherical brown-smoke fraction) compared to its initial guess or the result of the previous iteration. The derivative matrix represents the change in modeled TOA BRF with respect to a change in one of our retrieved parameters, such as AOD. The difference vector (column vector on the right) represents the difference between the observations and the current modeled TOA BRFs. On average, the magnitude of this vector should decrease with every iteration as the algorithm converges to a better solution vector. The diagonal weight matrix (first matrix on the left on both sides of the equation), which convolves channel weights (w) with their respective channel uncertainties (Unc), is used to account for things such as excessive sun glint, topographic shadowing, and missing data, as well as accounting for the uncertainty in the model–measurement system (more detail on this can be found in Limbacher and Kahn, 2019). The fact that this is a diagonal matrix means that we assume our channel weights and uncertainties are uncorrelated (by channel).
Solving for the change in our retrieved parameter vector (ΔPar) is done using a bounded-variable least squares (BVLS) solver, which was originally written by Lawson and Hanson (1995) and updated to FORTRAN 90 by John Burkardt in 2014 (https://people.math.sc.edu/Burkardt/f_src/f_src.html, last access: 15 November 2022; Burkardt, 2014). This allows us to put constraints on ΔPar to ensure that our retrieved parameters stay within physical bounds (i.e., 0.005< AOD <9.95, 0< FMF <1.0). The iterative process of interpolating to a new model BRF (2), calculating its derivatives, and then iterating to a more optimal solution (3) continues for a minimum of five iterations until the change in our cost function,
falls below a certain tolerance (currently set to 0.00001) or 100 iterations have occurred (in practice this many iterations would very rarely occur). One of the problems with linear least squares retrievals is that the assumed linearity in model response may not be accurate far from where the derivatives were calculated. This can result in the solution vector “bouncing around”, slow convergence, or non-convergence. To address this, if the algorithm detects that the cost function has not decreased after a new iteration, it multiplies the change in our retrieved parameter vector (ΔPar) by 0.5 and recomputes the cost function. The algorithm will continue doing this until the new cost function is lower than the value calculated for ΔPar=0 (i.e., the cost function of the previous iteration).
Once the algorithm has converged to a solution, it converts the four particle property grids back into a one-dimensional list of 550 nm aerosol mixture fraction (for all 17 components), while also reporting 550 nm AOD, the prescribed surface albedo, and cost. This can be done because our list of 17 component aerosol particle analogs exactly maps to the bins shown in Fig. 1. To decrease file size, which is still ∼20 GB for all AERONET data in the validation dataset, we do not save the mixture fractions for all 17 components, but rather save information such as 550 nm fine-mode fraction and 550 nm SSA based on the aggregated results.
2.1.2 MISR RA retrieved surface algorithm (RSA) using discrete aerosol mixtures
Although MODIS-MAIAC-retrieved surface BRF allows the MISR RA to retrieve AOD and aerosol properties over land when aerosol loading is elevated, the quality of MISR RA retrievals is negatively impacted when the MAIAC surface is assumed and aerosol loading is low to moderate (AOD at 550 nm <0.75). This is due to factors such as differences between the MISR and MODIS spectral responses, gridding error, plane-parallel radiative transfer errors, and MAIAC-retrieved surface BRF error (which should be much larger for the MISR 70∘ viewing cameras than for the near-nadir cameras). As a result, a version of the MISR RA was developed that self-consistently retrieves AOD, aerosol properties, and surface properties at pixel-level resolution (1.0 km here). The MISR RA RSA is functionally identical to the algorithm described in Limbacher and Kahn (2019) with the following two exceptions, which are described briefly below: (1) a modification of the discrete list of aerosol mixtures used by the retrieval algorithm and (2) the addition of an over-land retrieval.
As in Limbacher and Kahn (2019), we use the same exponential weighted average of discrete aerosol mixtures (at their best-fitting AOD) to identify aggregate aerosol and surface properties. However, the discrete aerosol mixtures we use for this technique have been updated to reflect our new component climatology. As in Sect. 2.1.1, we break up our components into fine- and coarse-mode components. Here, we only consider a small subset of the total number of components for our retrieval. The six fine-mode components used for this retrieval correspond to component numbers 1, 3, 9, 10, 15, and 16, whereas the two coarse-mode components are 12 and 17. These components were selected in a way that allows the algorithm to maintain sensitivity to parameters such as single-scattering albedo (when AOD is elevated), while acknowledging that we are unlikely to have sensitivity to fine-mode brown-smoke fraction for AOD <1.5. The six fine-mode components are mixed with the two coarse-mode components in the following FMF proportions (which results in 104 mixtures): 1.0, 0.95, 0.9, 0.8, 0.7, 0.6, 0.5, 0.4, 0.2, and 0. These FMF proportions are more heavily weighted towards the fine mode, which allows us to better match validation Ångström exponents when AOD is low and sensitivity to aerosol particle size is minimal. For the sake of mixing fine- and coarse-mode components together, the algorithm treats component 16 as fine-mode here, even though all medium and larger components are considered to be coarse-mode for the comparison with AERONET. A flowchart describing this new retrieval is presented in Fig. S2; we provide a short summary of the technique below.
The addition of an over-land retrieval to the RSA represents a relatively simple extension and upgrade of our existing over-water retrieval that allows for shallow, turbid, and eutrophic water, as described in Limbacher and Kahn (2019). For both the over-land and over-water RSAs, we first redefine the surface BRF as follows:
where Aλ represents the view-invariant surface albedo and Bc represents the spectrally invariant angular brightness coefficient, which is set to 1.0 for over-water retrievals, and represents the remote sensing BRF over water. provides a reasonably accurate estimate of the impact of including multiple reflections into our modified surface albedo, as this significantly simplifies the surface retrieval with no adverse impacts (we disentangle this term later). Equation (5) is also known as a shape similarity assumption because the spectral surface BRF is assumed to vary by the same relative fraction at each view angle (surface brightness can change with view angle, but its color cannot). This shape similarity assumption has its heritage in the multiangle Along-Track Scanning Radiometer-2 (ATSR-2) instrument (Flowerdew and Haigh, 1995; Veefkind et al., 1998) and was adopted by the MISR team as part of the MISR standard aerosol retrieval algorithm (Diner et al., 2005).
To retrieve the surface BRF for any given AOD and aerosol model, we rewrite our cost function using Eqs. (2) and (4) by applying the shape similarity assumption (Eq. 5):
For every AOD and aerosol model in our LUT, we first estimate the modified surface albedo ( by assuming that the surface can be adequately described as Lambertian, which requires that we set Bc=1. We then take the derivative of Eq. (6) with respect to (here, we assume , set the result to 0, and analytically solve for the modified surface albedo:
For our over-water retrieval, this is the only step required to estimate the modified surface albedo for a given AOD and aerosol mixture. However, over land, we must solve for the shape similarity coefficient (Bc) by taking the derivative of Eq. (6) with respect to Bc, setting it equal to 0 (here we assume , and solving for Bc:
For our over-land retrieval, we then iterate through Eqs. (7) and (8) twice, as the algorithm typically converged after two iterations (based on prior experience), which results in further refinement of both and Bc. Constraints on and Bc are provided in Fig. S2 and act to provide limits to both the color and brightness of the surface.
Following Fig. S2 and as summarized above, we retrieve the modified surface albedo and shape similarity coefficient for all 104 discrete aerosol mixtures and 26 AODs found in our RT LUT (Table 2). To iterate towards the optimum AOD for each of those 104 aerosol mixtures, the algorithm also temporarily saves information such as cost function (104 mixtures × 26 AODs) and channel-specific residual (104 mixtures × 26 AODs × 4 bands × 9 cameras). These channel-specific residuals are simply a portion of our cost function . After computing this information on the coarse AOD grid of our LUT, the algorithm then iterates towards a better-fitting (and more precise) AOD and surface for each of the 104 aerosol mixtures using a bisectional approach with five iterations; given the coarse grid spacing shown in Table 2, the resulting AOD should have an algorithmic precision ranging from <0.001 at an AOD of 0.0 to ∼0.025 at an AOD of 10.
Table 2Updated LUT values and dimensionality. Each column lists the values of the variable in the heading that are included in the LUT. The number of values is given in parentheses at the top, The overall dimensionality of the LUT is eight, although it is broken up into a seven-dimensional over-land LUT (no wind speed dimension; 5.4×106 elements) and a seven-dimensional over-water LUT (surface pressure assumed to be 1013.25 mb; 1.34×107 elements).
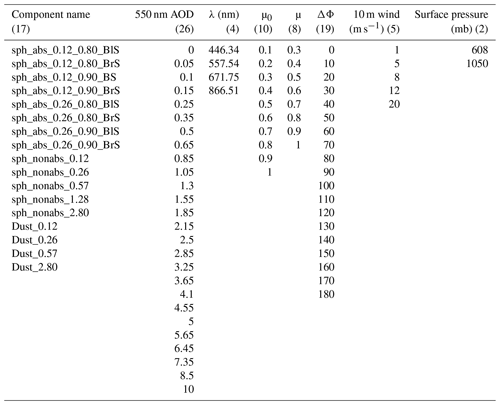
Once the optimum AOD and surface BRF properties have been calculated for each aerosol mixture, normalized mixture weights are calculated according to
where the subscript m represents aerosol mixture, Costm represents the lowest cost (best fit) for each of the 104 aerosol mixtures, and Costmin represents the lowest cost among all mixtures. Weighted aggregate parameters are then calculated for the following: 550 nm AOD, modified surface albedo (, shape similarity coefficient (Bc), aerosol component fraction (Table 1), and cost. Finally, Aλ is calculated using via division by (. As in the previous section, the algorithm then converts the aerosol component fraction into the 550 nm fine-mode fraction, ANG, and 550 nm SSA, while also reporting 550 nm AOD, the retrieved surface albedo, and cost.
Over water, this algorithm retrieves seven pieces of information about aerosol loading and properties as well as four pieces of information about the surface spectral BRF (Aλ). Over land, the algorithm retrieves an additional nine pieces of information about the surface BRF angular behavior, which yields a total of 20 retrieved pieces of information from 36 measurements. Even in the most topographically complex regions (where up to four MISR cameras may be eliminated due to obscuration) the number of observations will equal or exceed the number of retrieved parameters. A major limiting factor of this algorithm is the assumption of surface shape similarity. If the color of the surface changes significantly with view angle, as it does in some desert regions, the algorithm will alias those errors into the retrieved aerosol properties and AOD.
2.1.3 MISR RA combined surface algorithm (CSA)
The prescribed and retrieved surface approaches are described in Sects. 2.1.1 and 2.1.2. Over land, the combined surface approach uses PSA AOD from the algorithm described in Sect. 2.1.1 to identify the optimal retrieval type for a given pixel. If PSA AOD is less than 0.75, the CSA selects the AOD and aerosol properties from the RSA. If PSA AOD is greater than 1.5, the combined surface retrieval selects the AOD and aerosol properties from the PSA. If PSA AOD falls between 0.75 and 1.5, the CSA linearly interpolates AOD and aerosol properties between the RSA and PSA. The logic behind this combined surface algorithm is twofold. When aerosol loading is low, errors in the surface BRF based on the PSA tend to produce significant high biases in AOD and errors in aerosol particle properties. Conversely, when aerosol loading is high, the RSA is unable to properly separate the surface and atmospheric contributions, leading to a substantial low bias in AOD (Kahn et al., 2010, among many others). Empirically, we find that this approach with these domain boundaries also yields optimal results when compared to AERONET, as shown in Sect. 3 below.
Over water, the CSA is used with the same AOD constraints as described above. However, because our prescribed surface could be very inaccurate (and result in low-quality aerosol retrievals for the PSA), the algorithm instead uses the RSA AOD (from the algorithm described in Sect. 2.1.2) to determine the algorithm type to be used for the final aerosol result (PSA, RSA, or CSA). Even though the RSA suffers from an AOD low bias at high AOD, the RSA still appears to retain sensitivity to AOD even when AERONET AOD exceeds 3, which makes this algorithm suitable for determining the algorithm type used. Due to the low numbers of high AOD MISR–AERONET coincidences over water, CSA AOD bounds (0.75 and 1.5) may need to be modified when we have more data or if we begin using a surface BRF dataset for our prescribed surface over-water retrievals.
2.2 MISR RA updated aerosol component climatology
The updated LUT containing RT output was created using SCIATRAN version 3.8 (Rozanov et al., 2014, https://www.iup.uni-bremen.de/sciatran/index.html, last access: 17 August 2020). The RT code was run using the full-vector discrete ordinates method solver with 16 streams for our 10 spherical fine-mode optical analogs with effective radii smaller than 0.5 µm and 32 streams for the other seven optical analogs. Detailed information about our 17 updated aerosol components can be found in Table 1, and information about the size and dimensionality of the LUT is given in Table 2. Even though Table 2 appears to have eight dimensions, the LUTs are broken up into a seven-dimensional over-water LUT (pressure is assumed to be 1013.25 mb) and a seven-dimensional over-land LUT (no wind speed dimension needed). The goal in creating the individual aerosol components shown in Tables 1 and 2 is to capture aerosol particle property variability in as few components possible, under the assumption that we can linearly mix the radiances of these mixtures to create a continuum in terms of aerosol size, shape, and single-scattering albedo. For our spherical absorbing analogs, we now include aerosol sizes ranging from 0.12 to 0.26 µm effective radius, which adds analogs that were missing in our 2014 dataset (Limbacher and Kahn, 2014) and from the operational MISR product (Kahn et al., 2010). Previously, we used a dust model optimized for the red and NIR channels only (Kalashnikova et al., 2005). Here, we replaced it with five that are modeled consistently for all MISR spectral bands (Lee et al., 2017), as described in Sect. 2.2.1 below.
2.2.1 Updated dust optical models
The non-spherical dust optical models used in the RA were created following Lee et al. (2017), except with the MISR spectral bands. The non-spherical dust's phase matrix (for all spectral bands) is derived by integrating the single-scattering properties of individual non-spherical particles over both size and shape distributions. Thus, representative size and shape distributions as well as the spectral refractive indices for dust are determined from Aerosol Robotic Network (AERONET; Holben et al., 1998) inversion data at Cape Verde for heavy dust events (coarse-mode AOD >0.5 and FMF <0.2), with the medians of the data record taken as representative values. Note that the AERONET inversion assumes a fixed spheroid shape mixture (Dubovik et al., 2006), and thus the same is used for consistency. The single-scattering properties of individual spheroids are available from an aerosol single-scattering property database (Meng et al., 2010), enabling one to easily obtain the spectral optical properties of dust. Similar dust models have been widely used in various aerosol retrieval algorithms, as they reduce artificial biases in AOD and Ångström exponent (ANG) retrievals due to inaccurate representation of non-spherical dust by spherical aerosol modeled with Mie theory (Dubovik et al., 2014; Hsu et al., 2019; Lee et al., 2012, 2017; Lyapustin et al., 2018; Sayer et al., 2018; Zhou et al., 2020). Previous versions of the MISR RA used only one coarse-mode non-spherical component to model dust. Here we create five new dust models, using the same refractive indices, with the same size distributions used for our spherical non-absorbing aerosol components, with the expectation that this will improve our retrievals of fine-mode (less absorbing) and coarse-mode (more absorbing) dust.
2.3 AERONET data and validation methodology
With hundreds of sites scattered worldwide, AERONET sun photometers directly measure spectral AOD (Holben et al., 1998) at an uncertainty of ∼0.01 (Eck et al., 1999; Sinyuk et al., 2012) and offer excellent cloud screening as part of the version 3 algorithm (Giles et al., 2019). Provided that AOD is –0.2, AERONET ANG can also be reported very accurately (Wagner and Silva, 2008). As in Limbacher and Kahn (2019), we first interpolate AERONET spectral AOD to the MISR band centers using a second-order polynomial in log space. Here we use version 3, level 1.5 (L1.5) AOD from AERONET because version 3 cloud screening is much better than in previous AERONET versions, and L1.5 data offer many more retrieval results compared to L2. We then compute the Ångström exponent as a log–log fit of interpolated AOD to wavelength using all four MISR wavelengths. For the AERONET direct-sun parameters (AOD and ANG), we attempt to limit spatiotemporal variability from negatively impacting our comparison with MISR by masking out all AERONET data falling outside a ±30 min window centered on the MISR overpass. AERONET 550 nm AOD and ANG (446–867 nm) are then averaged over this window prior to comparison with the MISR RA.
Although AERONET almucantar inversions (Dubovik and King, 2000) represent retrievals of aerosol properties such as sphericity and SSA rather than direct measurements, they provide an opportunity to compare with aerosol particle properties retrieved from imagers such as MISR over diverse regions and temporal ranges that can span more than a decade. Because almucantar inversions are performed far less frequently than AOD is sampled, we limit potential coincidences to within ±4 h of the MISR overpass time, saving the following averaged (mean) 550 nm parameters: absorbing AOD, fine-mode AOD, coarse-mode AOD, and sphericity. Average single-scattering albedo is then calculated as 1.0 − (absorbing AOD)(fine-mode AOD + coarse-mode AOD). The AERONET fine-mode fraction (FMF) is calculated as fine-mode AOD(fine-mode AOD + coarse-mode AOD), and the MISR fine-mode fraction is defined as extinction due to aerosol having an effective radius smaller than 0.5 µm.
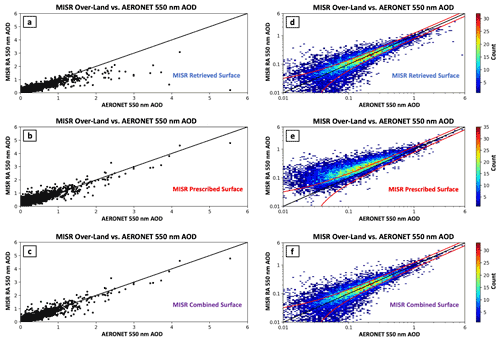
Figure 2Comparison of MISR RA over-land 550 nm AOD retrievals with AERONET direct-sun 550 nm AOD. The x axes represent AERONET 550 nm AOD and y axes represent MISR RA-retrieved 550 nm AOD. The MISR over-land retrieval algorithm type (prescribed surface algorithm – PSA, retrieved surface algorithm – RSA, or combined surface algorithm – CSA) is embedded in the lower right of each panel. Panels (a) and (d) present the MISR RSA, (b) and (e) present the MISR PSA, and (c) and (f) present the CSA. Panels (a)–(c) on the left show scatterplots of MISR RA AOD compared to AERONET, with a linear scale to allow for easier interpretation of high AOD results. Panels (d)–(f) on the right show two-dimensional histograms of MISR RA AOD compared to AERONET, with a logarithmic scale to allow for easier interpretation of low AOD results. Expected error of MISR CSA AOD is embedded as two red lines in panels (d)–(f).
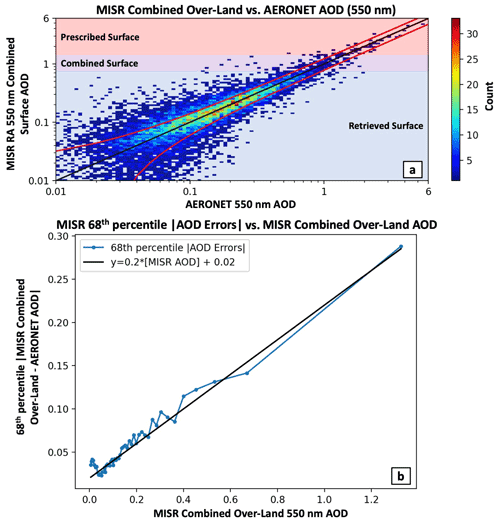
Figure 3Panel (a) is identical to Fig. 2f, with the addition of a color code added to identify regions where the prescribed and/or retrieved surface algorithms are used in the combined surface algorithm. Panel (b) shows MISR RA 68th percentile absolute AOD errors as a function of MISR combined over-land AOD. Data are plotted at increments of 2 % (∼160 coincidences per data point), with a black expected error line (derived from these data) plotted on top of the data.
3.1 MISR RA over-land validation using AERONET
As explained in the previous section, we use a ±30 min averaging window for comparing AERONET direct-sun results with the MISR RA and a ±4 h averaging window for comparing AERONET almucantar inversion results with the MISR RA. Because retrieval quality likely degrades dramatically in the presence of clouds, sea ice, and where retrieval fits are poor (i.e., a high cost function), we established a series of tests to help identify good-quality retrievals (for all 48×48 MISR RA retrievals centered on an AERONET station). Quality flags are set for each test.
-
MISR surface height (from the SA digital elevation model) is within 200 m of the given AERONET station height.
-
At least seven of nine MISR cameras contain valid BRF data.
-
MISR pixels must be masked as land.
-
MISR prescribed and retrieved cost functions are both <1.
-
MISR combined surface AOD is <9.
-
The second derivative of the prescribed surface cost function with respect to AOD is >10.
-
The normalized difference vegetation index (NDVI) using the prescribed surface albedos is >0.0.
-
Blue BRF max − blue BRF min (over all cameras) [MISR prescribed surface AOD]).
-
The MISR retrieved surface AOD standard deviation among all QA pixels is <1.
Quality flag 1 just makes sure that we compare pixels at roughly the same elevation to each other (as dust and other aerosols tend to be concentrated in layers) and is only used when comparing AERONET AOD to MISR retrieved AOD. The reasoning here is that the total column loading will likely differ with surface elevation, but aerosol particle properties will not vary as much. Quality flag 2 makes sure that a retrieval has enough “good” input data to give high-quality output, and quality flag 3 uses our previously computed land–water mask as we are only comparing the land algorithm to AERONET for the current validation exercise. Quality flag 4 uses the RSA and PSA cost functions to screen out poor-quality (mostly cloud-contaminated) retrievals. Quality flag 5 indicates that results with a combined retrieval AOD greater than 9 are likely cloud. As we saw in Limbacher and Kahn (2019), the second derivative of our cost function can be a good indicator of retrieval quality. A larger second derivative corresponds to a steeper minimum in our cost function with respect to AOD; we use 10 as a lower bound here in quality flag 6 as this tends to mask out some lower-quality results (mostly clouds). Quality flag 7 primarily masks unmasked water and clouds using the MAIAC prescribed surface albedos (these are input into the PSA). Here, NDVI is calculated as follows: NDVI = (NIR − Red)(NIR + Red). Quality flag 8 is used to mask partially cloudy MISR data (clouds in some cameras but not others), as the difference between the maximum and minimum BRF will be quite large for such pixels. Quality flag 9 attempts to remove stray clouds via a large-scale (low-frequency) variability filter.
3.1.1 AERONET direct-sun validation of MISR over-land RA
Applying the flags described in Sect. 3.1 and requiring at least 10 quality-assessed retrievals (out of 2304 potential, from 48×48 pixel patches) for each MISR–AERONET coincidence results in 11 563 averaged MISR RA–AERONET over-land coincidences for the 4 years of processed MISR data, interspersed between September 2000 and November 2016. AOD statistics for the MISR–AERONET validation are shown in Table 3 and are provided for the RSA, PSA, and CSA.
Table 3MISR RA vs. AERONET direct-sun over-land statistics. The rows under “AOD comparison” indicate the type of MISR retrieval being compared to AERONET. The rows under “ANG comparison” indicate the MISR RA AOD constraints being placed on the comparison with AERONET (MISR AOD must be >0.05, etc). For the first column, RSA corresponds to the MISR RA over-land retrieved surface algorithm, PSA corresponds to the MISR RA over-land prescribed surface algorithm, and CSA corresponds to the MISR RA over-land combined surface algorithm. The number of MISR–AERONET coincidences used to generate a given set of statistics is given in column 2 (No.). Root mean squared error (RMSE) is given in column 3, median absolute error (MAE) is given in column 4, the average MISR–AERONET bias is given in column 5, and the Pearson correlation coefficient (r) is given in column 6.
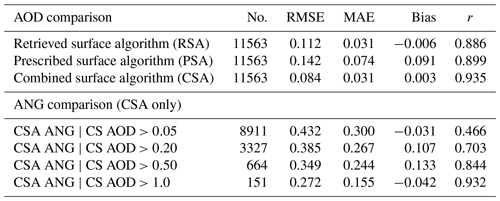
Figure 2 shows the MISR–AERONET over-land 550 nm AOD comparisons for the RSA (a and d), PSA (b and e), and the CSA (c and f). Comparisons are plotted in both linear and log space, as it is easier to evaluate the lower AOD comparisons with a log–log plot. Additionally, the red lines on the log–log plots correspond to , which is our estimate of the expected error of the combined retrieval (Fig. 3b). Figure 2d clearly demonstrates that the RSA performs well when AERONET AOD is low to moderate, whereas Fig. 2b clearly shows the advantages of the PSA when aerosol loading is high. The CSA described in Sect. 2.1.3 leverages the strengths of each algorithm, resulting in an RMSE (0.084) 25 % lower than the RSA (0.112) and 41 % lower than the PSA (0.142), as well as yielding a correlation coefficient (r=0.935) that is higher than either approach. Because the statistics for the CSA are significantly better than either the prescribed or retrieved surface algorithms, the rest of the over-land validation shows only results from the CSA.
Figure 3a presents a larger AOD scatterplot image of the MISR RA CSA with the different algorithm regimes color-coded. Because the different regimes are selected based on the MISR prescribed surface AOD (not the combined AOD), the background color codes are approximate. Comparing to Fig. 2, Fig. 3a demonstrates that the combined approach picks the best pieces from both algorithms. This algorithm also eliminates the tendency for the MISR RA (and the MISR SA) to significantly underestimate AOD when aerosol loading is elevated. Figure 3b shows that a prognostic error of fits the data very well, although this can be reduced by applying further quality constraints to the data. This prognostic error is taken as a line fitted to the 68th percentile absolute AOD errors (with respect to AERONET), binned at every 2 % of MISR-retrieved AOD (so 50 bins in total). As a prognostic error, this can be used to estimate pixel-level uncertainty of MISR RA AOD without the use of AERONET data (assuming the data are cloud- and quality-screened in the manner described above and that AERONET cloud screening is not significantly biassing the results).
Figure 4 compares the MISR CSA ANG to AERONET ANG for MISR-retrieved AOD greater than 0.05 (panel a), 0.20 (panel b), 0.50 (panel c), and 1.0 (panel d). Statistics for these plots are also provided in Table 3. Figure 4 (all panels) shows two clusters of ANG for AERONET, one at ∼0.25 (likely dust-dominated) and another at ∼1.5 (probably smoke- and/or pollution-dominated). The MISR RA captures the smoke- and/or pollution-dominated cluster very well but tends to overestimate ANG substantially as AERONET ANG decreases below ∼1.25, although this significantly improves as AOD increases. The fact that this bias improves with AOD suggests that the cause may be due to the fact that the RSA is more weighted towards the fine mode (unlike the PSA). Because the PSA is used only when AOD exceeds 0.75 (and fully only when >1.5), the largest change in this bias should show up from panels c to d (which it does). Regardless of the reasons for the discrepancies with AERONET, ANG RMSE of 0.385 and a correlation coefficient of 0.703 for MISR AOD >0.20 suggest that the algorithm still offers useful particle size constraints over land even at lower AOD. For AOD >1, an RMSE of 0.272 and correlation coefficient of 0.932 (n=151) indicate that the algorithm is in excellent agreement with AERONET and can offer substantial information on aerosol particle size at higher AOD, as expected.
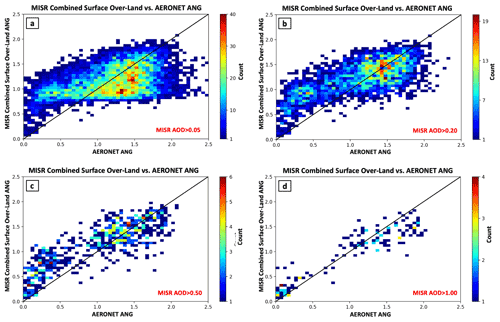
Figure 4Two-dimensional histograms of the MISR RA combined surface algorithm over-land ANG compared to AERONET ANG. The x axes are AERONET ANG and y axes are the MISR combined surface algorithm over-land ANG. Panel (a) shows MISR ANG vs. AERONET ANG constrained by MISR-retrieved AOD >0.05. Panels (b)–(d) show the same as (a), but for MISR-retrieved AOD constraints of >0.20 (b), >0.50 (c), and >1.0 (d).
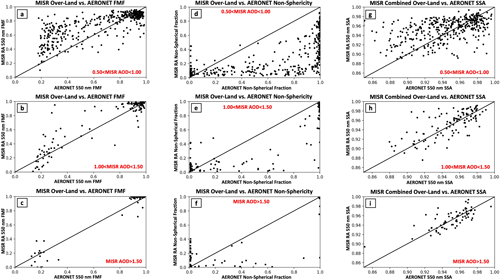
Figure 5Scatterplots of the MISR RA combined surface algorithm over-land 550 nm particle properties compared to AERONET 550 nm retrieved particle properties. The x axes are AERONET 550 nm particle properties and y axes are the MISR combined surface algorithm over-land 550 nm particle properties. AOD constraints are embedded in red for each panel. The first row of panels (a, d, g) corresponds to retrievals with 0.50< MISR AOD <1. The second row of panels (b, e, h) corresponds to retrievals with 1.00< MISR AOD <1.50. The third row of panels (c, f, i) corresponds to retrievals with MISR AOD >1.50. The first column of panels (a–c) shows MISR RA-retrieved fine-mode fraction (FMF) vs. AERONET-retrieved FMF, the second column of panels (d–f) shows MISR RA-retrieved non-spherical fraction vs. AERONET-retrieved non-spherical fraction, and the third column of panels (g–i) shows MISR RA-retrieved single-scattering albedo (SSA) vs. AERONET-retrieved SSA.
3.1.2 AERONET inversion validation of MISR over-land RA
Using the retrieval quality flags indicated in Sect. 3.1 combined with a 4 h averaging window (we still require at least one direct-sun data point within 30 min of the MISR overpass) and 10-pixel minimum (same as in Sect. 3.1.1), we found that a significant number of the high-AOD cases used for our inversion comparison were mistaken as clouds and screened out. The cause of this excessive masking is due to quality flags 6–8 (Sect. 3.1). For this inversion comparison we now ignore those three flags unless at least one of those flags is triggered (i.e., condition not met) and the fraction of good retrievals (out of 2304 potential retrievals) is less than 0.1. This new method allows us to increase the number of QA cases designated as “good” with AOD >1.0 by 65 %, while still eliminating most cloudy artifacts. It yields 2561 MISR–AERONET inversion coincidences with MISR AOD >0.2, 571 coincidences with AOD >0.5, and 177 coincidences with AOD >1. Statistics for all figures shown (SSA, FMF, and sphericity) can be found in Table 4.
Table 4MISR RA vs. AERONET almucantar inversion statistics over land. Same as Table 3, except for MISR RA vs. AERONET inversion statistics over land. All MISR data correspond to the combined surface algorithm. Note that AERONET inversion results are not ground truth; they represent retrieval results. The AERONET team cautions against the use of results when blue-band AOD <0.4, so comparisons for green-band AOD <0.50 should be considered qualitative rather than quantitative.
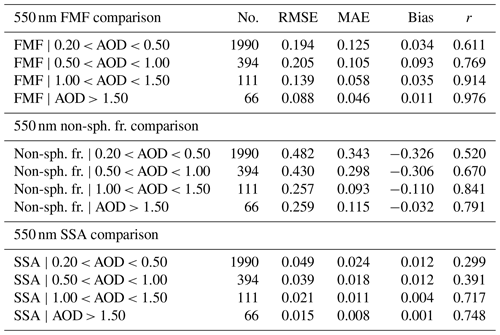
A comparison of MISR RA 550 nm FMF and AERONET 550 nm FMF is presented in Fig. 5a (0.5< AOD <1.0), Fig. 5b (1.0< AOD <1.5), and Fig. 5c (AOD >1.5). Figure 5a–c show very similar patterns compared to Fig. 4, with excellent sensitivity to retrievals of small (fine-mode) smoke and pollution aerosol and less sensitivity seen in the coarse-mode-dominated regions. However, as demonstrated in Fig. 5b–c and Table 4, overall sensitivity to FMF increases substantially for retrieved 1.0< AOD <1.5 (compared to 0.5< AOD <1.0), with RMSE dropping from 0.205 to 0.139, median absolute error (MAE) improving from 0.105 to 0.058, and the correlation coefficient increasing from 0.769 to 0.914.
Even though the current version of the MISR RA now includes fine-mode non-spherical components, the algorithm tends to dramatically underestimate the retrieved non-spherical fraction compared to the value retrieved from AERONET. This is in part due to the mixtures available to the RSA, as the algorithm is more dominated by fine-mode spherical analogs than either non-spherical or coarse-mode analogs. Just like FMF, MISR sensitivity to the non-spherical fraction over land dramatically improves as AOD increases. This is also the case for AERONET inversions, but probably occurs at lower AOD. Imposing more stringent AOD constraints (1.0< AOD <1.5 compared to 0.5< AOD <1.0), RMSE drops from 0.43 to 0.257, MAE drops from 0.298 to 0.093, and the correlation coefficient increases from 0.670 to 0.841.
A comparison of MISR 550 nm over-land retrieved SSA and AERONET 550 nm SSA is also presented in Fig. 5g–i. MISR SSA errors decrease significantly with increasing AOD, resulting in an RMSE of 0.021 for the 111 coincidences with 1.0< AOD <1.5. For AOD greater than 1.5, RMSE is 0.015 and MAE is 0.008, whereas the correlation coefficient is 0.748. It is likely that this improvement in SSA is in part due to the recent addition of multiple non-spherical particle models of different sizes, which allows the algorithm to better retrieve non-spherical particle size (and consequently SSA). Given that AERONET uncertainty for SSA at these higher AODs is likely ∼0.01 (Sinyuk et al., 2020), it is also likely that AERONET SSA uncertainty is propagating into our reported statistics, unless the errors for both MISR and AERONET are positively correlated.
3.2 MISR RA over-water validation using AERONET
We use the same temporal constraints for our over-water AERONET comparison as were used over land. We apply the following series of tests to help identify good-quality retrievals (for all 48×48 MISR RA retrievals centered on an AERONET station). Quality flags are set for each test.
-
The MISR surface height (from DEM) is within 200 m of the given AERONET station height.
-
At least seven of nine MISR cameras have valid BRF data.
-
MISR pixels must be masked as water.
-
The MISR retrieved surface cost function is <1.
-
The MISR retrieved surface AOD is <9.
-
MISR BRF NDVI (minimized over all nine cameras) of MISR BRFs is .
-
(MISR prescribed surface AOD − MISR retrieved surface AOD) MISR retrieved surface AOD +0.05).
-
The MISR retrieved surface AOD standard deviation among all QA pixels is < 0.25.
As for our over-land results, quality flag 1 ensures that we compare pixels at roughly the same elevation to each other and is only used when comparing AERONET AOD to MISR-retrieved AOD. Quality flag 2 ensures that a retrieval has enough “good” input data to give high-quality output, which is especially important over water where up to four cameras could be glint-contaminated. Quality flag 3 uses our previously computed land–water mask to make sure that a given pixel is water. Quality flag 4 uses the retrieved surface cost function to screen out poor-quality retrievals. Quality flag 5 screens out pixels with a retrieved surface AOD >9 (likely cloud), and quality flag 6 masks out clouds and ephemeral waterways that are not currently water-covered. Quality flag 7 is used to identify clouds that have not been screened by the other quality filters. Because the over-water RSA does not suffer from the same dramatic loss of sensitivity to AOD seen by the over-land RSA, the PSA and RSA values should be similar except in the presence of clouds or over very bright waters. As such, quality flag 7 will also likely eliminate many retrievals over bright waters. Quality flag 8 attempts to remove stray clouds via a large-scale variability filter.
Table 5MISR RA vs. AERONET direct-sun statistics over water. Same as Table 3, except for MISR RA over water.
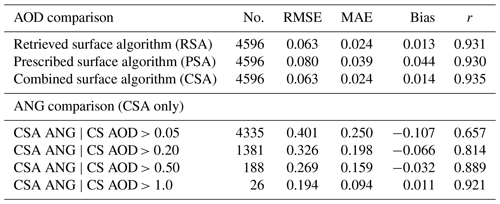
3.2.1 AERONET direct-sun validation of MISR over-water RA
As in our over-land comparison, we apply the flags listed above and require at least 10 quality-assessed retrievals (pixels) for each AERONET coincidence; otherwise, the spatially averaged MISR results are not included in the statistics. AOD and ANG statistics for the 4596 MISR quality-assessed and AERONET coincidences are shown Table 5.
Figure 6, the over-water equivalent to Fig. 2, presents the comparison of MISR over-water AOD for all three retrieval types (RSA, PSA, and CSA) as both a scatterplot in linear space (to emphasize higher AOD results) and a log–log 2-D histogram (to compare lower AOD results). Specifically, the MISR RSA over-water retrieval does not suffer from the same level of degradation in results as AERONET AOD increases (there is still a small low bias), which is why we use the RSA to identify the bounds for the CSA over water. Compared to Fig. 2 and Table 3, results appear much more consistent with AERONET AOD over water than over land, with a combined surface RMSE of 0.063 over water vs. 0.084 over land, MAE of 0.024 over water vs. 0.031 over land, and correlation coefficient of 0.935 over water vs. 0.935 over land. Although there is little improvement in the total statistics between the RSA and CSA over-water surface results, this may be due to the very limited number of MISR over-water–AERONET coincidences when AOD is elevated (>1; 26 over water vs. 177 over land). As such (and to be consistent with the previous section), ANG and particle property results are presented subsequently only for the CSA.
Figure 7a shows the MISR CSA over-water AOD compared to AERONET AOD, with colored rectangular boxes to indicate the retrieval regime of the MISR combined retrieval. Figure 7b shows a plot of MISR–AERONET 68th percentile errors as a function of MISR CSA over-water AOD. The line (0.15× [MISR AOD] +0.02) fits the MISR error very well for all ranges of retrieved AOD, indicating that this should be a good estimate of expected error.
Figure 8 shows the comparison of MISR over-water CSA ANG with AERONET ANG as a 2-D histogram for the same AOD bins presented in Fig. 4: MISR AOD >0.05 (panel a), MISR AOD >0.2 (panel b), MISR AOD >0.50 (panel c), and MISR AOD >1 (panel d). It is clear from Fig. 8 that the MISR over-water retrieval algorithm suffers from a small low bias in ANG for pollution and smoke aerosol when AOD is low (<0.2), and this is also represented in the statistics found in Table 5. As expected, the statistics for the MISR over-water retrieval appear better than the over-land results for every AOD regime. Compared to the over-land results for MISR-retrieved AOD >0.20, RMSE is 0.326 over water vs. 0.385 over land, MAE is 0.198 vs. 0.267, and the correlation coefficient is 0.814 vs. 0.703, suggesting that the MISR over-water retrieval has good sensitivity to retrievals of spectral AOD (ANG is derived from this) when AOD is >0.20.
3.2.2 AERONET inversion validation of MISR over-water RA
As in our over-land comparison, we use the MISR CSA results with a 4 h averaging window (requiring at least one valid direct-sun data point) and 10-pixel minimum. Although we are likely eliminating some high-AOD events, it was not feasible to develop an additional cloud-screening metric to use for the inversion comparison. The result of our quality assessment is 948 coincidences with MISR AOD >0.2, 144 coincidences with AOD >0.5, and 21 coincidences with AOD >1. Statistics for the MISR over-water vs. AERONET inversion comparison are shown in Table 6 for the 550 nm fine-mode fraction, 550 nm non-spherical fraction, and 550 nm single-scattering albedo. Due to the limited number of MISR over-water–AERONET coincidences with AOD >1.0, the conclusions one can draw from this dataset will also be limited.
Table 6MISR RA vs. AERONET almucantar inversion statistics over water. Same as Table 4, except for MISR RA vs. AERONET inversion statistics over water. All MISR data correspond to the combined surface algorithm. Note that AERONET inversion results are not ground truth; they represent retrieval results. The AERONET team cautions against the use of results when blue-band AOD <0.4, so comparisons for green-band AOD <0.50 should be considered qualitative rather than quantitative.
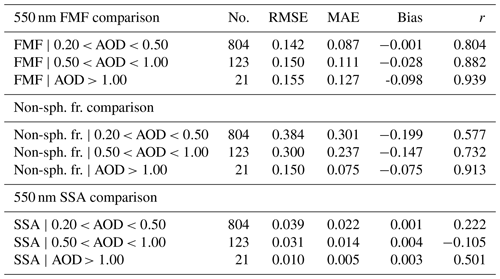
Figure 9a–c show scatterplots of MISR over-water FMF compared to AERONET FMF for AOD ranges presented in Table 6. MISR over-water FMF statistics are better than the over-land results for MISR-retrieved AOD <1, especially for the AOD range of 0.2–0.5. In this range, over-water vs. over-land statistics are as follows: RMSE is 0.142 vs. 0.194, MAE is 0.087 vs. 0.125, and the correlation coefficient is 0.804 vs. 0.611. Interestingly, MISR over-water FMF RMSE and MAE slightly deteriorate from the 0.2–0.5 retrieved AOD regime onwards, even though correlation improves substantially (from 0.804 to 0.939 for AOD >1). The fact that the FMF bias becomes more negative in magnitude with increasing AOD suggests that this is in part due to algorithmic differences between the PSA and RSA (more fine-mode-dominated for the RSA), although it could also be partly due to differences in the definition of fine mode between the MISR RA and AERONET.
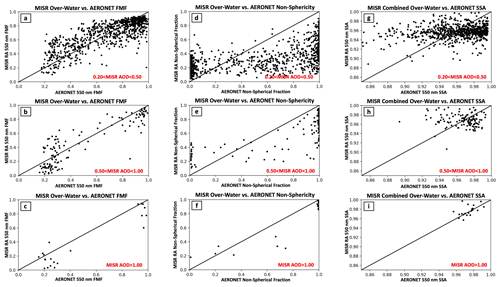
Figure 9Same as Fig. 5, except for the MISR over-water retrieval. Note the different AOD (compared to Fig. 5) bounds embedded in red.
Figure 9d–f show scatterplots of the MISR over-water non-spherical fraction compared to the AERONET non-spherical fraction. Unlike with FMF, errors and correlation improve with AOD for all bins. It is likely that the addition of multiple non-spherical particle models (now included in both fine and coarse modes for the MISR RA) is contributing to the improvement in retrieved non-sphericity with AOD, with RMSE at 0.384 for the 0.2–0.5 bin, dropping to 0.3 for the 0.5–1.0 bin, and dropping further to 0.15 for AOD >1. Correlation dramatically improves as well, increasing from 0.577 to 0.913 for the 0.2–0.5 bin and AOD >1, respectively.
Figure 9g–i show scatterplots of MISR over-water 550 nm single-scattering albedo compared to retrievals of AERONET single-scattering albedo (with the same AOD ranges as above). Although the correlation is quite a bit lower than the results over land, RMSE and MAE are better for the water algorithm compared to the over-land algorithm (for the same AOD bin). For instance, for over-water AOD , we report an RMSE of 0.010, an MAE of 0.005, and a correlation coefficient of 0.501, whereas for the 1.0–1.5 AOD bin over-land retrieval (n=62) we report an RMSE of 0.021, MAE of 0.011 and correlation coefficient of 0.717. Considering how dust-dominated the over-water results are at high AOD (AERONET mean non-sphericity is 0.799 for AOD >1), it is very likely that the addition of multiple non-spherical particle models has significantly improved our retrievals of SSA, especially in dust-dominated regions. However, the conclusions we can draw from this are still limited by the small number (21) of QA cases with AOD >1.0.
In Limbacher and Kahn (2019), we demonstrated the MISR RA's ability to retrieve AOD and the Ångström exponent over ice-free water of any color (turbid, shallow, eutrophic, etc.). Using the same dataset we used in that study, we develop, test, and present a new version of the MISR RA capable of retrieving aerosol and surface properties over both land and ice-free water. We also test the approach of imposing a prescribed surface BRF at higher AOD using MODIS-MAIAC Ross-Thick Li-Sparse (RTLS) RTLS 8 d surface BRF kernels over land and static values over water. In addition to validating AOD and the Ångström exponent, we dig more deeply into this dataset by evaluating the retrieved fine-mode fraction (FMF), retrieved non-spherical fraction (Non-Sph Fr), and retrieved single-scattering albedo (SSA; all parameters at 550 nm).
Over land, using our combined surface algorithm, the dataset yields 11 563 quality-assessed MISR–AERONET direct-sun coincidences. The MISR RA over-land 550 nm AOD is highly correlated with AERONET 550 nm AOD (r=0.935). The error statistics are also quite favorable, with a root mean squared error (RMSE) of 0.084, median absolute error (MAE) of 0.031, and a small bias of 0.003. Constraining MISR RA-retrieved AOD errors by MISR RA-retrieved AOD, we identify a prognostic pixel-level MISR RA over-land AOD uncertainty of , which holds true even when AOD exceeds unity over land, unlike for the MISR operational standard algorithm (SA; which suffers from extreme biases in this regime). For the 664 MISR–AERONET direct-sun coincidences with MISR-retrieved AOD greater than 0.50, we report the following Ångström exponent statistics: RMSE is 0.349, MAE is 0.244, the bias is 0.133, and the correlation coefficient is 0.844. The AERONET almucantar inversion dataset yields 571 quality-assessed MISR–AERONET coincidences with MISR-retrieved AOD >0.50 and 177 coincidences with MISR-retrieved AOD >1. For 1.0< MISR AOD <1.5, we report FMF RMSE of 0.139 and FMF r=0.914, non-sph fr. RMSE of 0.257 and r=0.841, and SSA RMSE of 0.021 and r=0.717. With the exception of the retrieved non-spherical fraction, over-land statistics continue to improve for AOD >1.5. Taken together with the Ångström exponent statistics, the over-land MISR RA yields some qualitative information about aerosol size (FMF and ANG) if retrieved AOD exceeds 0.2, with excellent quantitative comparison to AERONET beginning at an AOD ∼1.0. Qualitative retrievals (RMSE ∼0.25) of non-sphericity can be made at higher AOD (generally >1) than is needed to get constraints on size. Depending on retrieval conditions, qualitative retrieval of SSA can be made at an AOD ranging from 0.5–1.0, with quantitative results (RMSE ∼0.02) apparent when AOD exceeds 1. MISR RA SSA error statistics continue to improve above an AOD of 1.5, with an RMSE =0.015 and MAE <0.01. Overall, we note that our assessment of retrieved particle properties from the MISR RA is consistent with the study performed by Kahn and Gaitley (2015) using the previous version (V22) of the MISR operational aerosol product. However, that work was limited to AOD <0.6, as the MISR SA suffers from systematic biases in AOD above this. For the first time, partly because the MISR RA prescribed surface algorithm allows us to perform aerosol retrievals accurately at much higher AOD, we can extend their qualitative conclusions about MISR-retrieved aerosol type into a more quantitative over-land comparison with AERONET.
Over water our combined surface algorithm yields 4596 MISR quality-assessed and AERONET direct-sun coincidences. As with the over-land retrieval, over-water AOD is highly correlated (r=0.935) with AERONET 550 nm AOD. Error statistics also improve, with an RMSE of 0.063, MAE of 0.024, and a small bias of 0.014. Prognostic pixel-level AOD error improves slightly to ). For the 188 MISR–AERONET direct-sun coincidences with MISR-retrieved AOD greater than 0.50, we report the following Ångström exponent statistics: RMSE is 0.269, MAE is 0.159, the bias is −0.032, and the correlation coefficient is 0.889. The AERONET almucantar inversion dataset yields 144 quality-assessed MISR–AERONET coincidences with MISR-retrieved AOD >0.50 and 21 coincidences with MISR-retrieved AOD >1, which greatly limits our ability to draw conclusions about retrieved aerosol particle properties over water. For MISR AOD >1.0, we report FMF RMSE of 0.155 and FMF r=0.939, non-sphericity RMSE of 0.150 and r=0.913, and SSA RMSE of 0.010 and r=0.501. Qualitative retrievals of aerosol type appear similar to the over-land retrieval, with the expectation that better constraints on all aerosol particle properties can be made at lower AODs. Due to the addition of multiple non-spherical particle models (including in the fine mode), it appears likely that quantitative retrievals (RMSE <0.2) of aerosol non-sphericity can be made over water if the AOD exceeds unity, although this will need to be confirmed in future work.
This paper represents the first iteration of a combined MISR RA over-land + over-water retrieval. The authors plan to use to results of this study to further refine the aerosol particle properties used by the algorithm and improve our characterization of the surface used by the prescribed surface algorithm over both land and water. In the future, we will likely include all AERONET direct-sun and inversion coincidences with MISR for the entire 22-year data record rather than the 4 years that were included here, as this will improve our ability to draw conclusions about aerosol particle properties, especially over water.
All MISR RA validation data used for this paper are available at NASA's Atmospheric Science Data Center (ASDC). The data can be downloaded at https://asdc.larc.nasa.gov/micro-article/new-misr-research-aerosol-retrieval-algorithm (last access: 9 November 2022, Kahn and Limbacher, 2022). CCMP vector winds are produced by Remote Sensing Systems and sponsored by NASA. Data are available at https://remss.com/ (last access: 1 November 2022; Mears et al., 2019).
The supplement related to this article is available online at: https://doi.org/10.5194/amt-15-6865-2022-supplement.
Originally developed by RAK, the MISR RA has been a joint effort of JAL and RAK since early 2011. The updated algorithm presented here was developed by JAL (with supervision by RAK), while the paper was produced with input from both JAL and RAK. JL developed the dust aerosol models used for this paper.
The contact author has declared that none of the authors has any competing interests.
Publisher's note: Copernicus Publications remains neutral with regard to jurisdictional claims in published maps and institutional affiliations.
We thank our colleagues on the Jet Propulsion Laboratory's MISR instrument team and at the NASA Langley Research Center's Atmospheric Sciences Data Center for their roles in producing the MISR standard datasets, as well as Brent Holben at NASA Goddard and the AERONET team for producing and maintaining this critical validation dataset. We thank Alexei Lyapustin and the MAIAC team for the MODIS-MAIAC products used in this paper. We also thank the Rozanovs and SCIATRAN team for their work on the SCIATRAN product, as well as Meng Gao, Stefan Kinne, Alexei Lyapustin, and one anonymous reviewer for careful reading and constructive commenting on the paper.
This research has been supported by the National Aeronautics and Space Administration, Earth Sciences Division (Terra Project grant, MISR Project grant, Climate and Radiation Research and Analysis Program grant, and Atmospheric composition Program grant). This research is supported in part by NASA's Climate and Radiation Research and Analysis Program under Hal Maring, NASA's Atmospheric Composition Program under Richard Eckman, and the NASA EOS MISR and Terra projects.
This paper was edited by Otto Hasekamp and reviewed by Alexei Lyapustin, Meng Gao, Stefan Kinne, and one anonymous referee.
Abdou, W. A., Martonchik, J. V., Kahn, R. A., West, R. A., and Diner, D. J.: A modified linear-mixing method for calculating atmospheric path radiances of aerosol mixtures, J. Geophys. Res., 102, 16883– 16888, https://doi.org/10.1029/96JD03434, 1997.
Burkardt, J.: BVLS source, https://people.math.sc.edu/Burkardt/f_src/bvls/bvls.html (last access: 15 November 2022), 2014.
Chen, W.-T., Kahn, R. A., Nelson, D., Yau, K., and Seinfeld, J.: Sensitivity of multi-angle imaging to optical and microphysical properties of biomass burning aerosols, J. Geophys. Res., 113, D10203, https://doi.org/10.1029/2007JD009414, 2008.
Diner, D. J. and Martonchik, J. V.: Atmospheric Transfer of Radiation Above an Inhomogeneous Non-Lambertian Reflective Ground. I: Theory, J. Quant. Spectrosc. Ra., 31, 97–125, 1984a.
Diner, D. J. and Martonchik, J. V.: Atmospheric Transfer of Radiation Above an Inhomogeneous Non-Lambertian Reflective Ground. II: Computational Considerations and Results, J. Quant. Spectrosc. Ra., 32, 279–304, 1984b.
Diner, D. J. and Martonchik, J. V.: Atmospheric transmittance from spacecraft using multiple view angle imagery, Appl. Opt., 24, 3503–3511, 1985.
Diner, D. J., Beckert, J. C., Reilly, T. H., Bruegge, C. J., Conel, J. E., Kahn, R. A., Martonchik, J. V., Ackerman, T. P., Davies, R., Gerstl, S. A. W., Gordon, H. R., Muller, J.-P., Myneni, R. B., Sellers, P. J., Pinty, B., and Verstraete, M. M.: Multiangle Imaging SpectroRadiometer (MISR) description and experiment overview, IEEE Trans. Geosci. Remt. Sens., 36, 107–1087, https://doi.org/10.1109/36.700992, 1998.
Diner, D. J., Martonchik, J. V., Kahn, R. A., Pinty, B., Gobron, N., Nelson, D. L., and Holben, B. N.: Using angular and spectral shape similarity constraints to improve MISR aerosol and surface retrievals over land, Remote Sen. Environ., 94, 155–171, https://doi.org/10.1016/j.rse.2004.09.009, 2005.
Dubovik, O. and King, M. D.: A flexible inversion algorithm for retrieval of aerosol optical properties from Sun and sky radiance measurements, J. Geophys. Res., 105, 20673–20696, 2000.
Dubovik, O., Sinyuk, A., Lapyonok, T., Holben, B. N., Mishchenko, M., Yang, P., Eck, T. F., Volten, H., Muñoz, O., Veihelmann, B., van der Zande, W. J., Leon, J.-F., Sorokin, M., and Slutsker, I.: Application of spheroid models to account for aerosol particle nonsphericity in remote sensing of desert dust, J. Geophys. Res., 111, D11208, https://doi.org/10.1029/2005JD006619, 2006.
Dubovik, O., Lapyonok, T., Litvinov, P., Herman, M., Fuertes, D., Ducos, F., Lopatin, A., Chaikovsky, A., Torres, B., Derimian, Y., and Huang, X.: GRASP: A Versatile Algorithm for Characterizing the Atmosphere, SPIE: Newsroom, https://doi.org/10.1117/2.1201408.005558, 2014.
Eck, T. F., Holben, B. N., Reid, J. S., Dubovik, O., Smirnov,A., O'Neill, N. T., Slutsker, I., and Kinne, S.: Wavelength dependence of the optical depth of biomass burning, urban, and desert dust aerosols, J. Geophys. Res., 104, 31333–31349, https://doi.org/10.1029/1999JD900923, 1999.
Flower, V. J. B. and Kahn, R. A.: The evolution of Iceland volcano emissions, as observed from space, J. Geophys. Res., 125, e2019JD031625, https://doi.org/10.1029/2019JD031625, 2020.
Flowerdew, R. J. and Haigh, J. D.: An approximation to improve accuracy in the derivation of surface reflectances from multi-look satellite radiometers, Geophys. Res. Lett., 22, 1693–1696, 1995.
Garay, M. J., Witek, M. L., Kahn, R. A., Seidel, F. C., Limbacher, J. A., Bull, M. A., Diner, D. J., Hansen, E. G., Kalashnikova, O. V., Lee, H., Nastan, A. M., and Yu, Y.: Introducing the 4.4 km spatial resolution Multi-Angle Imaging SpectroRadiometer (MISR) aerosol product, Atmos. Meas. Tech., 13, 593–628, https://doi.org/10.5194/amt-13-593-2020, 2020.
Giles, D. M., Sinyuk, A., Sorokin, M. G., Schafer, J. S., Smirnov, A., Slutsker, I., Eck, T. F., Holben, B. N., Lewis, J. R., Campbell, J. R., Welton, E. J., Korkin, S. V., and Lyapustin, A. I.: Advancements in the Aerosol Robotic Network (AERONET) Version 3 database – automated near-real-time quality control algorithm with improved cloud screening for Sun photometer aerosol optical depth (AOD) measurements, Atmos. Meas. Tech., 12, 169–209, https://doi.org/10.5194/amt-12-169-2019, 2019.
Holben, B. N., Eck, T. F., Slutsker, I., Tanre, D., Buis, J. P., Sezter, A., Vermote, E., Reagan, J. A., Kaufman, Y. J., Nakajima, T., Lavenu, F., Jankowiak, I., and Smirnov, A.: AERONET – a federated instrument network and data archive for aerosol characterization, Remote Sens. Environ., 66, 1–16, 1998.
Hsu, N. C., Lee, J. Sayer, A. M., Kim, W., Bettenhausen, C., and Tsay, S.-C.: VIIRS Deep Blue aerosol products over land: Extending the EOS long-term aerosol data records, J. Geophys. Res.-Atmos., 124, 4026–4053, https://doi.org/10.1029/2018JD029688, 2019.
Junghenn Noyes, K. T., Kahn, R. A., Limbacher, J. A., Li, Z., Fenn, M. A., Giles, D. M., Hair, J. W., Katich, J. M., Moore, R. H., Robinson, C. E., Sanchez, K. J., Shingler, T. J., Thornhill, K. L., Wiggins, E. B., and Winstead, E. L.: Wildfire Smoke Particle Properties and Evolution, From Space-Based Multi-Angle Imaging II: The Williams Flats Fire during the FIREX-AQ Campaign, Remote Sens., 12, 3823, https://doi.org/10.3390/rs12223823, 2020.
Kahn, R. and Limbacher, J.: AOD_AERONET_Comparison and Inversion_AERONET_Comparison, ASDC [data set], https://asdc.larc.nasa.gov/micro-article/new-misr-research-aerosol-retrieval-algorithm, last access: 9 November 2022.
Kahn, R. A. and Gaitley, B. J.: An analysis of global aerosol type as retrieved by MISR, J. Geophys. Res.-Atmos., 120, 4248–4281, https://doi.org/10.1002/2015JD023322, 2015.
Kahn, R. A., West, R., McDonald, D., Rheingans, B., and Mishchenko, M. I.: Sensitivity of Multi-angle remote sensing observations to aerosol sphericity, J. Geophys. Res., 102, 16861–16870, https://doi.org/10.1029/96JD01934, 1997.
Kahn, R. A., Banerjee, P., McDonald, D., and Diner, D.: Sensitivity of Multiangle imaging to Aerosol Optical Depth, and to Pure-Particle Size Distribution and Composition Over Ocean, J. Geophys. Res., 103, 32195–32213, https://doi.org/10.1029/98JD01752, 1998.
Kahn, R. A., Banerjee, P., and McDonald, D.: The Sensitivity of Multiangle Imaging to Natural Mixtures of Aerosols Over Ocean, J. Geophys. Res., 106, 18219–18238, https://doi.org/10.1029/2000JD900497, 2001.
Kahn, R. A., Gaitley, B. J., Martonchik, J. V., Diner, D. J., Crean, K. A., and Holben, B.: Multiangle Imaging Spectroradiometer (MISR) global aerosol optical depth validation based on 2 years of coincident Aerosol Robotic Network (AERONET) observations, J. Geophys. Res., 110, D10S04, https://doi.org/10.1029/2004JD004706, 2005.
Kahn, R. A., Gaitley, B. J., Garay, M. j., Diner, D. J., Eck, T., Smirnov, A., and Holben, B. N.: Multiangle Imaging SpectroRadiometer global aerosol product assessment by comparison with the Aerosol Robotic Network, J. Geophys. Res., 115, D23209, https://doi.org/10.1029/2010JD014601, 2010.
Kalashnikova O. V. and Kahn, R. A.: Ability of multiangle remote sensing observations to identify and distinguish mineral dust types: Part 2. Sensitivity over dark water, J. Geophys. Res., 111, D11207, https://doi.org/10.1029/2005JD006756, 2006.
Kalashnikova, O. V., Kahn, R., Sokolik, I. N., and Li, W.-H.: Ability of multiangle remote sensing observations to identify and distinguish mineral dust types: Optical models and retrievals of optically thick plumes, J. Geophys. Res., 110, D18S14, https://doi.org/10.1029/2004JD004550, 2005.
Lawson, C. L. and Hanoson, R. J.: Solving least squares problems, Society for Industrial and Applied Mathematics, John Wiley and Sons, New York, p. 350, ISBN: 0898713560, 1995.
Lee, J., Kim, J., Yang, P., and Hsu, N. C.: Improvement of aerosol optical depth retrieval from MODIS spectral reflectance over the global ocean using new aerosol models archived from AERONET inversion data and tri-axial ellipsoidal dust database, Atmos. Chem. Phys., 12, 7087–7102, https://doi.org/10.5194/acp-12-7087-2012, 2012.
Lee, J., Hsu, N. C., Sayer, A. M., Bettenhausen, C., and Yang, P.: AERONET-based nonspherical dust optical models and effects on the VIIRS Deep Blue/SOAR over water aerosol product, J. Geophys. Res.-Atmos., 122, 10384–10401, https://doi.org/10.1002/2017JD027258, 2017.
Limbacher, J. A. and Kahn, R. A.: MISR research-aerosol-algorithm refinements for dark water retrievals, Atmos. Meas. Tech., 7, 3989–4007, https://doi.org/10.5194/amt-7-3989-2014, 2014.
Limbacher, J. A. and Kahn, R. A.: MISR empirical stray light corrections in high-contrast scenes, Atmos. Meas. Tech., 8, 2927–2943, https://doi.org/10.5194/amt-8-2927-2015, 2015.
Limbacher, J. A. and Kahn, R. A.: Updated MISR dark water research aerosol retrieval algorithm – Part 1: Coupled 1.1 km ocean surface chlorophyll a retrievals with empirical calibration corrections, Atmos. Meas. Tech., 10, 1539–1555, https://doi.org/10.5194/amt-10-1539-2017, 2017.
Limbacher, J. A. and Kahn, R. A.: Updated MISR over-water research aerosol retrieval algorithm – Part 2: A multi-angle aerosol retrieval algorithm for shallow, turbid, oligotrophic, and eutrophic waters, Atmos. Meas. Tech., 12, 675–689, https://doi.org/10.5194/amt-12-675-2019, 2019.
Lyapustin, A. and Wang, Y.: MCD19A3 MODIS/Terra + Aqua BRDF Model Parameters 8-Day L3 Global 1 km SIN Grid V006, NASA EOSDIS Land Processes DAAC, https://doi.org/10.5067/MODIS/MCD19A3.006, 2018.
Lyapustin, A., Wang, Y., Laszlo, I., Hilker, T., Hall, F., Sellers, P., Tucker, C., and Korkin, S.: Multi-angle implementation of atmospheric correction for MODIS (MAIAC): 3. Atmospheric correction, Remote Sens. Environ., 127 385–393, https://doi.org/10.1016/j.rse.2012.09.002, 2012.
Lyapustin, A., Wang, Y., Korkin, S., and Huang, D.: MODIS Collection 6 MAIAC algorithm, Atmos. Meas. Tech., 11, 5741–5765, https://doi.org/10.5194/amt-11-5741-2018, 2018.
Martonchik, J. V., Diner, D. J., Kahn, R. A., Ackermann, T. P., Verstraete, M. M., Pinty, B., and Gordon, H. R.: Techniques for the retrieval of aerosol properties over land and ocean using multiangle imaging, IEEE Trans. Geosci. Remote Sens., 36, 1212–1227, 1998.
Martonchik, J. V., Diner, D. J., Crean, K. A., and Bull, M. A.: Regional aerosol retrieval results from MISR, IEEE Trans. Geosci. Remote Sens., 40, 1520–1531, 2002.
Martonchik, J. V., Kahn, R. A., and Diner, D. J.: Retrieval of aerosol properties over land using MISR observations, in: Satellite Aerosol Remote Sensing Over Land, edited by: Kokhanovsky, A., Springer, Berlin, 267–293, https://doi.org/10.1007/978-3-540-69397-0_9, 2009.
Mears, C. A., Scott, J., Wentz, F. J., Ricciardulli, L., Leidner, S. M., Hoffman, R., and Atlas, R.: A near‐real‐time version of the cross‐calibrated multiplatform (CCMP) ocean surface wind velocity data set, J. Geophys. Res.-Oceans, 124, 6997–7010, 2019.
Meng, Z., Yang, P., Kattawar, G. W., Bi, L., Liou, K. N., and Laszlo, I.: Single-scattering properties of tri-axial ellipsoidal mineral dust aerosols: A database for application to radiative transfer calculations, J. Aerosol Sci., 41, 501–512, https://doi.org/10.1016/j.jaerosci.2010.02.008, 2010.
North, P. R. J., Briggs, S. A., Plummer, S. E., and Settle, J. J.: Retrieval of land surface bidirectional reflectance and aerosol opacity from ATSR-2 multi-angle imagery, IEEE Trans. Geosci. Remote Sens., 37, 526–537, https://doi.org/10.1109/36.739106, 1999.
Rozanov, V. V., Rozanov, A. V., Kokhanovsky, A. A., and Burrows, J. P.: Radiative transfer through terrestrial atmosphere and ocean: Software package SCIATRAN, J. Quant. Spectrosc. Ra., 133, 13–71, https://doi.org/10.1016/j.jqsrt.2013.07.004, 2014.
Sayer, A. M., Hsu, N. C., Lee, J., Bettenhausen, C., Kim, W. V., and Smirnov, A.: Satellite Ocean Aerosol Retrieval (SOAR) algorithm extension to S-NPP VIIRS as part of the “Deep Blue” aerosol project, J. Geophys. Res.-Atmos., 123, 380–400, https://doi.org/10.1002/2017JD027412, 2018.
Sinyuk, A., Holben, B. N., Smirnov, A., Eck, T. F., Slutsker, I., Schafer, J. S., Giles, D. M., and Sorokin, M.: Assessment of error in aerosol optical depth measured by AERONET due to aerosol forward scattering, Geophys. Res. Lett., 39, L23806, https://doi.org/10.1029/2012GL053894, 2012.
Sinyuk, A., Holben, B. N., Eck, T. F., Giles, D. M., Slutsker, I., Korkin, S., Schafer, J. S., Smirnov, A., Sorokin, M., and Lyapustin, A.: The AERONET Version 3 aerosol retrieval algorithm, associated uncertainties and comparisons to Version 2, Atmos. Meas. Tech., 13, 3375–3411, https://doi.org/10.5194/amt-13-3375-2020, 2020.
Veefkind, J. P., de Leeuw, G., and Durkee, P.: Retrieval of aerosol optical depth over land using two-angle view satellite radiometry during TARFOX, Geophys. Res. Lett., 25, 3135–3138, 1998.
Wagner, F. and Silva, A. M.: Some considerations about Ångström exponent distributions, Atmos. Chem. Phys., 8, 481–489, https://doi.org/10.5194/acp-8-481-2008, 2008.
Zhou, Y., Levy, R. C., Remer, L. A., Mattoo, S., and Espinosa, W. R.: Dust aerosol retrieval over the oceans with the MODIS/VIIRS Dark Target algorithm: 2. Nonspherical dust model, Earth Space Sci., 7, e2020EA001222, https://doi.org/10.1029/2020EA001222, 2020.