the Creative Commons Attribution 4.0 License.
the Creative Commons Attribution 4.0 License.
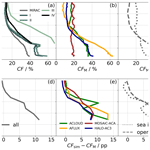
Assessing Arctic low-level clouds and precipitation from above – a radar perspective
Imke Schirmacher
Pavlos Kollias
Katia Lamer
Mario Mech
Lukas Pfitzenmaier
Manfred Wendisch
Susanne Crewell
Most Arctic clouds occur below 2 km altitude, as revealed by CloudSat satellite observations. However, recent studies suggest that the relatively coarse spatial resolution, low sensitivity, and blind zone of the radar installed on CloudSat may not enable it to comprehensively document low-level clouds. We investigate the impact of these limitations on the Arctic low-level cloud fraction, which is the number of cloudy points with respect to all points as a function of height, derived from CloudSat radar observations. For this purpose, we leverage highly resolved vertical profiles of low-level cloud fraction derived from down-looking Microwave Radar/radiometer for Arctic Clouds (MiRAC) radar reflectivity measurements. MiRAC was operated during four aircraft campaigns that took place in the vicinity of Svalbard during different times of the year, covering more than 25 000 km. This allows us to study the dependence of CloudSat limitations on different synoptic and surface conditions.
A forward simulator converts MiRAC measurements to synthetic CloudSat radar reflectivities. These forward simulations are compared with the original CloudSat observations for four satellite underflights to prove the suitability of our forward-simulation approach. Above CloudSat's blind zone of 1 km and below 2.5 km, the forward simulations reveal that CloudSat would overestimate the MiRAC cloud fraction over all campaigns by about 6 percentage points (pp) due to its horizontal resolution and by 12 pp due to its range resolution and underestimate it by 10 pp due to its sensitivity. Especially during cold-air outbreaks over open water, high-reflectivity clouds appear below 1.5 km, which are stretched by CloudSat's pulse length causing the forward-simulated cloud fraction to be 16 pp higher than that observed by MiRAC. The pulse length merges multilayer clouds, whereas thin low-reflectivity clouds remain undetected. Consequently, 48 % of clouds observed by MiRAC belong to multilayer clouds, which reduces by a factor of 4 for the forward-simulated CloudSat counterpart. Despite the overestimation between 1 and 2.5 km, the overall low-level cloud fraction is strongly reduced due to CloudSat's blind zone that misses a cloud fraction of 32 % and half of the total (mainly light) precipitation amount.
- Article
(5996 KB) - Full-text XML
- BibTeX
- EndNote
Low-level clouds are prominent features of the Arctic climate (Shupe et al., 2006; Liu et al., 2012; Mioche et al., 2015) and have a large impact on the radiative energy budget of the Arctic surface (e.g., Curry et al., 1996; Shupe and Intrieri, 2004; Wendisch et al., 2019). In contrast to the global cooling effect of low clouds, between 70 and 82∘ N they may create a positive (warming) cloud radiative forcing (CRF) of ∼ 10 W m−2 (Kay and L'Ecuyer, 2013). The terrestrial CRF dominates and warms the near-surface air due to low solar elevation during polar day and absent solar radiation during polar night (Lubin and Vogelmann, 2006; Stapf et al., 2021). Within the last 4 decades, the near-surface warming in the Arctic increased more strongly than the global average, which is referred to as Arctic amplification (Serreze and Barry, 2011; Wendisch et al., 2019). Diverse processes and interacting feedback mechanisms lead to Arctic amplification. An increased cloud cover and amount of water vapor and the lapse rate feedback from persistent clouds (Graversen et al., 2008) would enhance the terrestrial downward radiation (Francis and Hunter, 2006) and contribute more strongly to Arctic amplification than the sea ice–albedo feedback (Winton, 2006). Thus, there is high interest in accurate observations of Arctic low-level cloud properties and their changes.
Detailed ground-based remote sensing observations that measure the vertical distribution and variability in low-level clouds are available from very few stations in the Arctic (e.g., Liu et al., 2017; Gierens et al., 2020). They allow us to study the temporal variability over long time periods but at a specific place. In contrast, ship campaigns in the High Arctic (Intrieri and Shupe, 2004; Shupe et al., 2022) assess the spatial variability only over short time periods but over a larger yet still limited area. As recently highlighted by Griesche et al. (2021) using measurements from the RV Polarstern in the marginal sea ice zone (MIZ), however, the frequent occurrence of low-level stratus around 100 m is often missed by ground-based observations. On larger spatial scales, active satellite measurements resolve vertical cloud structures for long time periods. CloudSat (Stephens et al., 2002) has been frequently used for studies of Arctic clouds for example to investigate the correlation between low-level cloud occurrence and sea ice concentration (Zygmuntowska et al., 2012; Mioche et al., 2015). For the years 2006 to 2011, Liu et al. (2012) find that roughly 80 % of clouds over the Arctic Ocean occur below 2 km altitude.
CloudSat and ground-based observations at the Eureka site, in Canada, revealed different cloud occurrences below 2 km altitude (Blanchard et al., 2014; Mioche et al., 2015). Thus, it remains to be determined if CloudSat captures all low-level Arctic clouds due to its limitations. First, CloudSat's along-track sampling conceals spatial cloud patterns. Second, according to Lamer et al. (2020), the pulse length either stretches or fails to detect shallow clouds. Third, the lowest levels from CloudSat's vertical profiles suffer from ground clutter due to reflections at the surface called the blind zone. This blind zone prevents the cloud assessment roughly below the first kilometer (Palerme et al., 2019; Lamer et al., 2020; Liu, 2022). Using ground-based radar measurements for reference, Maahn et al. (2014) showed that CloudSat underestimates the total precipitation by 9 percentage points (pp) over Ny-Ålesund, Svalbard. The representation of Arctic low-level clouds in climate models is of high relevance to investigate, for example, its correlation with sea ice concentration (Morrison et al., 2019). To fully exploit CloudSat for improving climate models it is necessary to know its limitations and thus to evaluate CloudSat measurements with more finely resolved observations that ideally cover broad areas over land and ocean. These measurements should ultimately address how low-level cloud occurrence varies close to the surface, depends on surface characteristics and meteorological situation, and thus affects Arctic amplification.
CloudSat observations have been compared with airborne remote sensing (e.g., Gayet et al., 2009; Painemal et al., 2019) for relatively homogeneous clouds at higher altitudes to calibrate airborne instruments (Barker et al., 2008; Protat et al., 2009, 2011). For the first time, Liu (2022) investigates synthetic CloudSat cloud masks in the Arctic region. These data are based on radar reflectivities from QuickBeam radar forward simulations (Haynes et al., 2007) that used vertical profiles of retrieved cloud properties from ground-based radar and lidar during the SHEBA (Surface Heat Budget of the Arctic Ocean) experiment. Compared to ground-based observations, the forward-simulated data detected all clouds with heights above 1 km, but 25 pp less below 600 m. Nevertheless, in this study the synthetic data were generated under several assumptions and by low-temporal-resolution measurements that had a different viewing geometry.
In this study, we investigate vertical profiles of low-level cloud occurrences over the Fram Strait using CloudSat observations and measurements by the airborne Microwave Radar/radiometer for Arctic Clouds (MiRAC; Mech et al., 2019) operating at the same radar wavelength as CloudSat. MiRAC measured highly resolved profiles with a lower blind zone of about 150 m on board the Polar 5 (Wesche et al., 2016) research aircraft during four airborne campaigns conducted in the vicinity of Svalbard within the framework of the German Research Foundation (DFG) project TRR 172, “ArctiC Amplification: Climate Relevant Atmospheric and SurfaCe Processes, and Feedback Mechanisms” ((AC)3; Wendisch et al., 2023). The Svalbard region is of particular interest because the steady heat and moisture flux of the North Atlantic Ocean enhances cloud fraction and precipitation compared to the entire Arctic (Mioche et al., 2015; McCrystall et al., 2021). The campaigns, namely ACLOUD (Arctic CLoud Observations Using airborne measurements during polar Day; Wendisch et al., 2019; Ehrlich et al., 2019), AFLUX (Airborne measurements of radiative and turbulent FLUXes of energy and momentum in the Arctic boundary layer; Mech et al., 2022b), MOSAiC-ACA (Multidisciplinary drifting Observatory for the Study of Arctic Climate-Airborne observations in the Central Arctic; Mech et al., 2022b), and HALO–(AC)3 (High Altitude and LOng range research aircraft–(AC)3), covered periods from 2017 to 2022 between March and September. Since Polar 5 flies relatively slowly, a unique database has been gathered that covers more than 25 000 km and includes four underflights of Polar 5 below CloudSat. The larger spatial coverage of the airborne observations compared to observations from land stations allows for new insights into the cloud variability over open ocean and sea ice.
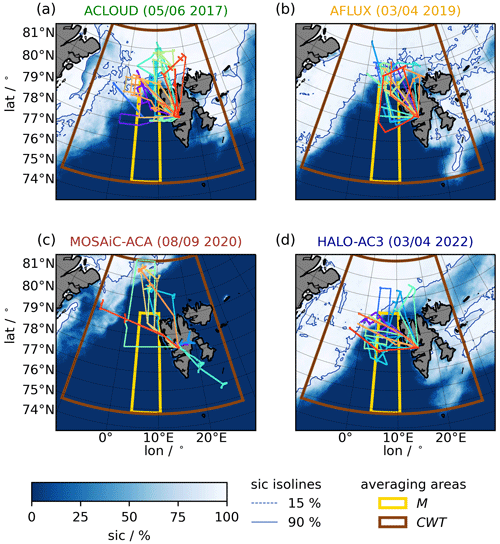
Figure 1Flight tracks and sea ice concentration (sic) during the airborne campaigns ACLOUD (a), AFLUX (b), MOSAiC-ACA (c), and HALO–(AC)3 (d). The indicated areas highlight the regions over which we calculate the marine cold-air-outbreak index (M; yellow) and determine the circulation weather type (CWT; brown).
Table 1Flight hours over several surfaces and covered distances during the analyzed flights of the four Polar 5 campaigns. Sea ice concentrations below 15 % and above 90 % represent open water and sea ice.
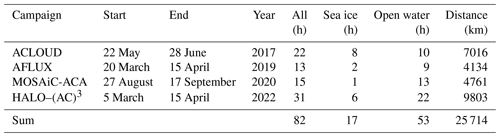
The paper is organized as follows. First, we present the CloudSat and airborne remote sensing data and describe how CloudSat's radar reflectivities are forward-simulated from MiRAC observations (Sect. 2). Second, we outline the meteorological situation encountered during the campaigns (Sect. 3). Section 4 evaluates the forward simulations for four underflights and investigates the effects of CloudSat's spatial resolution, blind zone, and sensitivity on its performance in detecting low-level clouds. Afterwards, Sect. 5 compares the fraction of the MiRAC and forward-simulated radar reflectivities across the entire data with height to analyze the variability in low-level Arctic cloud occurrence with respect to meteorological and surface conditions and to identify states that limit CloudSat's cloud detection the most. Section 6 concludes the study and discusses future steps.
Table 2Specifications of the Cloud Profiling Radar (CPR) on CloudSat and the airborne radar MiRAC, which are illustrated in Fig. 2.
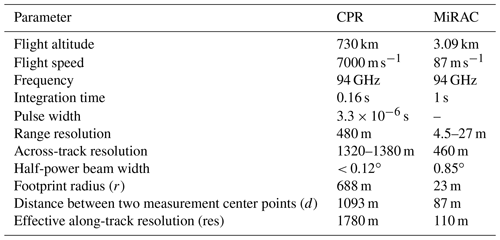
All four airborne campaigns were based in Longyearbyen, Svalbard, and included various flights focusing on the Fram Strait area with varying sea ice conditions during the different campaigns (Fig. 1, Table 1). This study uses only measurements with flight altitude above 2 km and omits measurements over land due to the complex topography. By using the daily sea ice concentration (sic) dataset (version 5.4) obtained by the second Advanced Microwave Scanning Radiometer (AMSR2), we differentiate between open water (sic < 15 %) and sea ice (sic > 90 %). This assumption is more strict than in previous studies (80 %; Strong and Rigor, 2013) to avoid cloud formation associated with leads. In total, 82 h of flight time corresponding to a distance exceeding 25 000 km is analyzed, with the majority (64 %) over open ocean.
CloudSat's Cloud Profiling Radar (CPR) and MiRAC are both down-looking W-band radars operating at 94 GHz. We focus on the measurements of the equivalent radar reflectivity factor Z from MiRAC (ZM) and CPR (ZC). The fraction of Z signals in the measurement period with height is also called hydrometeor fraction and hereafter referred to as cloud fraction (CF).
Note that attenuation by supercooled liquid layers and precipitation affects the downward-looking observations of both instruments the same way. While dry air negligibly attenuates Z at 94 GHz, atmospheric water vapor and hydrometeors can significantly attenuate Z. In the dry Arctic, nonetheless, this attenuation is assumed small. With a total column water vapor amount of 15 kg m−2, which is relatively high for the Arctic, a two-way attenuation below 1 dBZ would occur (Kneifel et al., 2015). A 500 m thick cloud with a liquid water path of 100 g m−2 would weaken Z by less than 0.6 dBZ (Stephens et al., 2002). Note that unlike the CPR, MiRAC does not suffer from atmospheric attenuation by hydrometeors above Polar 5 flight altitude, which is mostly around 3 km.
2.1 Spaceborne CloudSat Cloud Profiling Radar (CPR)
The CloudSat satellite orbit reaches up to 82.5∘ latitude and provides the only domain-wide vertically resolved satellite observations sensitive to clouds, light precipitation, and snow in the Arctic region (Liu, 2008; Kulie and Bennartz, 2009; Palerme et al., 2014). The CPR (Table 2 for a list of specifications) is a pulsed radar, and the pulse width results in a range resolution of 480 m (Stephens et al., 2002; Tanelli et al., 2008).
The antenna half-power beam width and flight altitude cause a latitude-dependent across-track resolution of 1320 to 1380 m, which is 1375 m particularly around Svalbard (Fig. 2, Table 2). Due to the integration time, the distance between two adjacent measurement center points (d) is 1090 ± 10 m, which again depends slightly on latitude (Tanelli et al., 2008). As a result of the instantaneous footprint and the integration time, the effective along-track CloudSat resolution (res) during the campaigns is close to 1780 m. In 2006, the CPR sensitivity was close to −30 dBZ and was supposed to stay at least close to −26 dBZ (Stephens et al., 2002; Tanelli et al., 2008; Stephens et al., 2008). Due to ground clutter, CloudSat likely overestimates low-level cloud occurrences below 0.5 height over ocean and 1 km over land and sea ice, respectively (Maahn et al., 2014; Mioche et al., 2015; Marchand, 2018; Lamer et al., 2020).
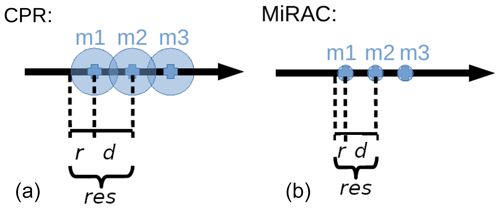
Figure 2Sketch of the horizontal resolution of the radar on CloudSat (CPR; a) and airborne radar MiRAC (b). The individual measurement center positions (m1, m2, and m3) are indicated by blue crosses and each footprint by blue circles; r is the radius of the footprint, d the distance between two measurements, and res the effective along-track resolution. For better illustration, MiRAC is scaled up by a factor of 10.
We analyze CPR data from the “2B-Geoprof” product version 5 (Marchand, 2018) over four underflights of Polar 5 below CloudSat (Table 3) following Blanchard et al. (2014) and Lamer et al. (2020). This product contains Z and a CPR cloud mask, which assigns a value for the cloud detection probability every 240 m in height and 1 km along-track. The 2B-Geoprof product hereby oversamples the return power by a factor of 2. The altitudes of the CloudSat range bins are slightly variable over time. For the analysis, the data are mapped to a constant grid with a grid size of 240 m by selecting the nearest neighbor. For the cloud mask, we settle for a given confidence value of 20 or higher following Lamer et al. (2020). This means that all range gates with lower values are considered to be cloud-free, filtering ground clutter and very weak signals (Marchand, 2018). Furthermore, only ZC values larger than −27 dBZ are considered to be cloud signals. This threshold is in accordance with the one applied by the CPR cloud mask above the blind zone (Marchand, 2018). Contrary to this study, Mioche et al. (2015) investigate the combined radar–lidar product DARDAR that might more successfully identify low-level cloud structures compared to the 2B-Geoprof product. However, DARDAR interpolates the CPR data in the vertical to the finer resolution of the lidar (Winker et al., 2003), still detects ground clutter erroneously as near-surface supercooled droplets, and thus overestimates near-surface cloud fraction (Blanchard et al., 2014).
2.2 Airborne
MiRAC is a frequency-modulated continuous-wave (FMCW) radar and operates at the same frequency (94 GHz) as CloudSat (Table 2 for list of specifications). Its sensitivity and vertical resolution depend on the chirp settings. During the campaigns the settings were such that the detection limit mostly reached below −40 dBZ (Mech et al., 2019). The vertical resolution is 4.5 m close to the aircraft and at most 27 m (Mech et al., 2019). During the processing, the vertical resolution of all flights is interpolated to 5 m. Considering the beam width, the radius of the beam's footprint at the surface is 23 m for the average flight altitude of about 3 km (Fig. 2). Due to the aircraft speed and temporal resolution of roughly 1 s, each measurement covers about 110 m. Hence, res of MiRAC is roughly 16 times higher than that of CPR. ZM is not investigated inside the lowest 150 m of the atmosphere due to surface-type-dependent ground clutter (Mech et al., 2019) and is linearly interpolated to a temporal resolution of 1 s. This study only accounts for measurements along straight flight segments over ocean that exceed a flight altitude of 2 km (Risse et al., 2022).
The Airborne Mobile Aerosol Lidar (AMALi; Stachlewska et al., 2010) also operated on Polar 5 is used to assess the cloud situation during the four underflights. It measures profiles of backscattered intensities at 532 (polarized parallel and perpendicularly) and 355 nm (not polarized). After averaging these profiles over 5 s and correcting them for the background signal and a drift, the attenuated backscatter coefficient is calculated (Ehrlich et al., 2019). By determining the highest altitude of consecutive heights that exceed the backscatter coefficient of a cloud-free section, the cloud top height is obtained with a vertical resolution of 7.5 m and a horizontal resolution of 375 m (Kulla et al., 2021a, b). For this study, we accessed all airborne data via the ac3airborne module that, among other things, stores all links to the data (Mech et al., 2022a).
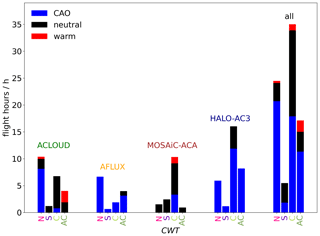
Figure 3Analyzed flight hours during the different circulation weather types (CWTs) for each campaign and over all campaigns. N, S, C, and AC stand for northerly, southerly, cyclonic, and anticyclonic flow, respectively. Each CWT class is divided into the occurrence of the marine cold-air-outbreak index (M): warm periods (red), neutral periods (black), and cold-air outbreaks (CAOs; blue).
2.3 Forward-simulation methodology
This section summarizes the steps applied to convert the more finely resolved and more sensitive MiRAC to CloudSat radar reflectivities.
-
Along-track convolution. We calculate a moving time average over 13 profiles, which represent the number of MiRAC along-track bins (res of 110 m) within the CloudSat footprint (1375 m), and consider an along-track weighting function that imitates the antenna pattern by a symmetrical Gaussian distribution covering the CloudSat footprint (Lamer et al., 2020).
-
Along-track integration. Here, the integration distance of CloudSat (1093 m) is considered by calculating an arithmetic mean over all convoluted profiles within the integration distance. For the underflights (Sect. 2.1), we assign to every CloudSat observation the averaged profile that best resembles the distance between CloudSat and the location where Polar 5 and CloudSat are closest (crossing location). For the statistical assessment over all campaigns, a profile is selected every 1093 m.
-
Along-range convolution. The range resolution of the ZC and ZM product is 240 m (Sect. 2.1) and 5 m (Sect. 2.2), respectively. To account for the pulse-limited range resolution of CloudSat, we average the convoluted observations from the previous step by applying a running mean with a symmetrical, 960 m long range-weighting function following Lamer et al. (2020). The range-weighting function is modeled with the help of a Gaussian distribution that produces a surface clutter echo profile similar to that observed by the CloudSat CPR postlaunch. The distribution spans 2 times the range resolution of CloudSat, i.e., 960 m, and thereby simulates ground clutter even more realistically, since the weight of signals far away from the center is tiny. Afterwards, we select Z values for every 240 m to mimic the digitization of CloudSat.
-
Sensitivity threshold. To obtain the fully forward-simulated equivalent radar reflectivities (Zsim), we apply a sensitivity threshold of −27 dBZ to eliminate signals that fall below the CloudSat sensitivity due to averaging over cloudy and cloud-free bins (i.e., partial beam filling).
The flights during the four campaigns (Fig. 1) span a range of meteorological conditions. We characterize and relate cloud occurrence to these conditions by determining the daily marine cold-air-outbreak index (M; Papritz et al., 2015) and the circulation weather type (CWT; Akkermans et al., 2012) from ERA5 reanalysis data provided for pressure levels (Hersbach et al., 2020).
3.1 Marine cold-air-outbreak index (M)
Following Papritz et al. (2015) and Kolstad (2017), M is defined as the difference between potential temperatures (θ) at the surface and 850 hPa altitude for each grid point over water:
For a more robust estimate, daily M values are averaged for the Fram Straight area (Fig. 1, yellow). A M below −8 K classifies a warm period, whereas a M above 0 K identifies cold-air outbreaks (CAOs) following Knudsen et al. (2018). CAOs typically occur when cold air masses form over the central Arctic ice and move southward over the warm open ocean, where they quickly saturate. Over the open water, cloud streets evolve, which grow in the vertical and horizontal directions with distance to the ice edge until they form convective cells. The heat release from the ocean enhances turbulence that deepens the cloud layer with time (Etling and Brown, 1993; Atkinson and Wu Zhang, 1996; Brümmer, 1999). Air-mass transformation during a CAO still poses many questions, requiring detailed measurements for testing high-resolution modeling.
In total, 63 % of the analyzed measurements were taken during CAOs, 32 % under neutral conditions, and 5 % under warm conditions (Fig. 3). Note that the sampling is affected by weather conditions suitable for flying. During warm conditions, a thick, continuous, low cloud layer often hinders Polar 5's take-off and landing at Longyearbyen airport (Svalbard). Therefore, warm periods do not appear representative.
ACLOUD (early summer) includes frequent CAO events and fewer warm periods (Fig. 3). During AFLUX and HALO–(AC)3, both taking place in early spring, CAO occurrences clearly dominate the analyzed flights. Conversely, neutral conditions (−8 K < M < 0 K) dominate MOSAiC-ACA, which was conducted in autumn. Only twice as much flight time was conducted during CAOs as during warm periods in this autumn campaign.
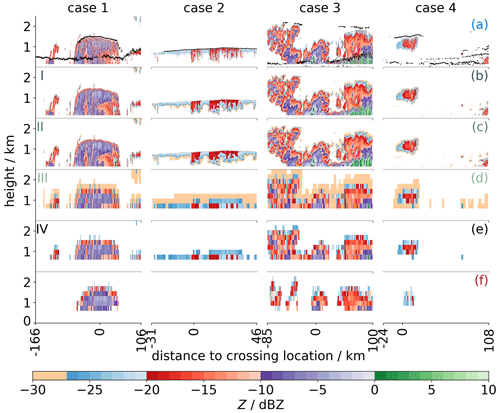
Figure 4Profiles of the equivalent radar reflectivity (Z) during the four underflights of Polar 5 below CloudSat (columns) as obtained from the airborne radar MiRAC (ZM; a), after along-track convolution (I; b), after additional along-track integration (II; c), after further along-range convolution (III; d), and after applying a sensitivity threshold of −27 dBZ (Zsim, IV; e). The CloudSat observations (ZC; f) are filtered by the CPR cloud mask. In addition, the cloud top height derived by the airborne lidar AMALi (a; black dots) is shown.
3.2 Circulation weather type (CWT)
Several approaches to categorizing synoptic situations into CWTs by their large-scale atmospheric circulation exist, e.g., by analyzing the areal average of the vorticity, strength, and direction of the geostrophic flow. We follow Akkermans et al. (2012) and use the Jenkinson–Collison classification, which comprises eight directional classes (N, NE, E, SE, S, SW, W, and NW) and two vorticity regimes (cyclonic, C, and anticyclonic, AC; Philipp et al., 2016). For a representative assessment, the CWT is calculated from the geopotential height at 850 hPa over a larger area (Fig. 1, brown).
In general, the flow is directed in the meridional direction and flow directions W and E do not occur during the analyzed flights. Thus, the main flow directions are S, N, C, and AC, and classes in between are assigned to the neighboring main direction following von Lerber et al. (2022). In total, there was 0.6 h of flight time during NE and NW flows and 1.8 h during SE and SW conditions; thus, they contribute less than 1 %.
During all campaigns, northerly (30 %) and cyclonic (43 %) flows dominate, whereas southerly winds appear rarely, making up only 7 % of the analyzed flight time (Fig. 3). The fewest northerly winds occurred during the MOSAiC-ACA campaign (autumn). With 1.9 h, the amount of cyclonal flow is lowest during AFLUX. For AFLUX and HALO–(AC)3 (both early spring), the primary difference in the synoptic situation is the main flow type, being northern and cyclonic during AFLUX and HALO–(AC)3, respectively. Northerly winds generally implicate CAOs, except for MOSAiC-ACA, during which the number of CAOs is in general low. Cyclonic conditions frequently include CAOs during the spring campaigns AFLUX and HALO–(AC)3, while they are less frequent (< 30 %) during MOSAiC-ACA and ACLOUD (early summer).
Four CloudSat underflights (Table 3) were performed in the vicinity of Svalbard (Fig. A1) during ACLOUD and AFLUX, lasting about 35 min each. ZM time series resolve the fine structures inside the clouds (Fig. 4a) and demonstrate that the cloud conditions during the underflights differ significantly. The clouds during cases 1 and 3 reach altitudes of up to more than 2 km and show light precipitation, as evidenced by reflectivities in the lowest range gate. During case 2, a thin cloud layer with virga and ZM below −20 dBZ appears below 1 km. Case 4 is mostly cloud-free and exhibits only one small non-precipitating cloud below 2 km. During all cases, CloudSat observes no additional clouds at higher levels (not shown). Hence, no attenuation occurs through high clouds. The cloud top heights obtained from the AMALi lidar and MiRAC measurements generally agree well. Exceptions occur at very low levels when the lidar likely detects a thin supercooled layer, which is even beyond the sensitivity limit of MiRAC.
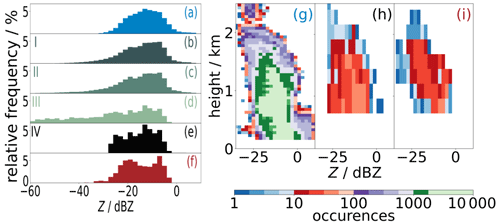
Figure 5Histogram (a–f) and contoured frequency by altitude diagram (CFAD; g–i) of the equivalent radar reflectivity (Z) over four underflights of Polar 5 below CloudSat. Histograms display the original MiRAC (ZM; a) and CloudSat data (ZC; f) and forward-simulated data obtained after each processing step (I–IV; Sect. 2.3). CFADs show ZM (g), the completely forward-simulated data (Zsim; h), and ZC (i). The color coding of the labels is equivalent to Fig. 4. The size of the bins equals 2 dBZ.
The horizontal cloud cover from the MiRAC observations during all underflights is 74 %, and 45 % of the cloud tops fall within the lowest kilometer. ZM ranges from −31 to 8 dBZ (Fig. 5a). Precipitation, which is hereafter defined as Z larger than −5 dBZ (Maahn et al., 2014), is rare. The vertically resolved ZM distribution (Fig. 5g) reveals that precipitation is confined to below 750 m height. ZM is most frequent at −15 dBZ due to signals between 0.15 and 1.2 km height that are mainly observed during case 1.
The mean CF profile of MiRAC (CFM; Fig. 6a, MiRAC) over all underflights shows almost no clouds above 2 km; the CF of clouds between 1.5 and 2 km height is on average 15 % and increases up to 40 % between 1.5 and 1 km height. At around 750 m, CFM maximizes, with 53 % over less than 500 m mainly due to the cloud layer captured during case 2.
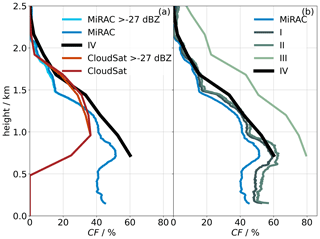
4.1 Effect of the forward simulation
At first, we illustrate how the different processing steps (Sect. 2.3) change the radar reflectivities when converting the MiRAC measurements (ZM) to those that would be observed by CloudSat (Zsim):
- I.
The along-track convolution (Fig. 4b) is independent of height and smooths hydrometeor-related signals in the horizontal. Therefore, especially broken cloud fields with small gaps are combined into clouds with larger horizontal extent. Furthermore, isolated reflectivities, such as during case 4 at altitudes below 200 m, become visible by smearing over a larger distance. The occurrence of very low-level clouds is confirmed by the lidar; however, they mostly fall even below the sensitivity limit of MiRAC. Note that at cloud boundaries, Z often declines below the sensitivity threshold of −27 dBZ (Fig. 5b). Compared to the original ZM distribution, which has its maximum at −15 dBZ (Fig. 5a), the distribution becomes bimodal (Fig. 5b).
- II.
The along-track integration (Fig. 4c) broadens and smears cloud structures in the horizontal; e.g., cloud gaps clearly shrink during case 1. The bimodality of Fig. 4b strengthens, and the distribution now has a global maximum at −8 dBZ and a local maximum at −20 dBZ (Fig. 5c).
- III.
After the along-range convolution (Fig. 4d), the coarser vertical resolution displays less fine cloud structures, stretches clouds in the vertical, and hence increases cloud top heights. To illustrate this in detail, the range-weighting function averages Z at the cloud top over a range of ± 480 m. Thus, cloud-free conditions above the cloud top now show a non-negligible radar reflectivity moving the cloud top upwards. Similar to the along-track averaging, Z decreases drastically even below −60 dBZ (Fig. 5d).
- IV.
The sensitivity threshold (Fig. 4e compared to c) reduces the number of signals along cloud boundaries and the number of whole clusters during case 4 and enlarges cloud gaps.
The lower resolution and sensitivity of Zsim do not change the contoured frequency by altitude diagram (CFAD) compared to ZM at the most frequented regions between −15 and −8 dBZ (Fig. 5g and h). However, the forward simulations decrease the number of Zsim values above 2.25 km and increase the number of Zsim values smaller than 22 dBZ below 960 m height. Zsim does not resolve the lowest 720 m and hence resolves almost no precipitation.
In a second step, we analyze how the mean CF profile averaged over all underflights changes in each processing step:
- I.
The along-track convolution (Fig. 6b, MiRAC compared to I) has no effect on CF above 1.5 km and increases CF by roughly 3 pp between 1 and 1.5 km and by 10 pp below. Near the surface, the overestimation of CF increases from 7 % to 25 % of CFM. The change in CF due to along-track averaging depends on how many individual clouds are encountered. A larger number of clouds with short gaps in between, e.g., across cloud streets, favors more horizontal cloud stretching and thus increases CF more. The clouds in case 3 enhance CF over all heights, whereas the precipitation and virga in cases 1 and 2 intensify the low-layer CF increase.
- II.
The along-track integration (Fig. 6b, I compared to II) acts in the same way as the along-track convolution but with a smaller effect. An additional increase in CF occurs, which is strongest below 1 km but less than 3 pp.
- III.
The range convolution (Fig. 6b, II compared to III) shifts the CF profile up by about 480 m due to cloud top stretching as Z is averaged over hydrometeor-free areas. Below 1 km, CF increases additionally, by around 30 pp in total. Here, the effect of the range-weighting function (Sect. 2.3) spanning ± 480 m is evident. At 720 m, Z between 240 m and 1.2 km affects CF after the range convolution. Hence, the range-weighted signals from non-precipitating low-level clouds might reach the lowest level. As we do not explicitly model a surface reflection signal, this weighting is also the reason why CFsim can only be calculated down to 720 m.
- IV.
CF after applying the sensitivity threshold (CFsim; Fig. 6b, III compared to IV) reduces by 25 pp particularly just above 1.5 km. Most cloud tops are directly below 1.5 km, where the gradient of the CFM profile is strongest. After cloud stretching, Z at the cloud tops is very small and often falls below the threshold. Thus, the effect of the threshold is predominant at 1.9 km, i.e., 480 m above the layer with most cloud tops. The sensitivity threshold reduces the cloud top height overestimation and leads to a net overshooting of about 240 m compared to CFM. Note that close to 1.9 km, some ZM values already fall below the threshold (Fig. 6a, MiRAC > −27 dBZ compared to MiRAC).
In summary, changes in sensitivity and resolution (Fig. 6a, MiRAC compared to IV) enhance CFsim compared to CFM most strongly below 1.5 km, i.e., 11 pp at 720 m, which is 25 % of CFM.
4.2 Evaluation of the forward simulation
Can we use Zsim as a proxy for ZC and thereby expand the analysis period over all campaigns? A comparison of Zsim, ZC, and the corresponding CF profiles shall answer this question and detect measurement biases between MiRAC and CPR. Note that differences in the observed cloud fields can arise due to the time and location shifts between the two radars. The highly spatially and temporally variable clouds (Fig. 4) do not allow clouds to be projected in space and time as done by Gayet et al. (2009) for extended ice clouds.
Zsim and ZC agree well (Fig. 4e and f), though a lower number of signals is measured by CloudSat. This is most striking in case 2, when CloudSat detects no clouds. Note that in the raw CloudSat data, i.e., without applying the cloud mask, weak ZC appears at 1 km height during case 2 (not shown). However, the mask attributes these signals to ground clutter and generally filters all signals below 720 m. The fact that MiRAC measurements for cases 1 and 3 evidence significant hydrometeor occurrence below 1 km demonstrates that the cloud mask is too strict, as pointed out in Lamer et al. (2020) (see their Fig. 1). Compared to the horizontal cloud cover from MiRAC, the one observed by CloudSat reduces by a factor of 2. Ground clutter could cause artificial echoes in the lower layers if the CPR mask is too gentle. In this case, ground clutter would enhance ZC but not affect Zsim, which is not dominant during the underflights (Fig. 7). Furthermore, CloudSat does not detect low ZM and thus shows separate clouds instead of a continuous cloud layer during case 3.
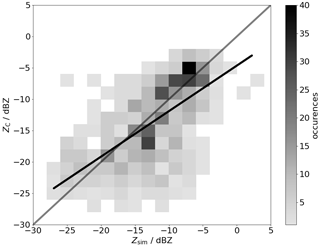
Figure 7Comparison of the equivalent radar reflectivity obtained from forward simulation (Zsim) and CloudSat (ZC) over four underflights of Polar 5 below CloudSat with the corresponding linear fit (black). The bin size equals 2 dBZ.
We investigate the realism of the forward simulation by directly comparing Zsim and ZC for each pixel (Fig. 7). ZC is on average 2 dB lower than Zsim for times when both instruments measured a signal. This bias is in the same range (1–2 dB) as that found by Protat et al. (2009). They processed the airborne Z the same way we do but used a threshold of −29 dBZ. Note that they achieve a better matching of air- and spaceborne Z because they only analyze extended non-precipitating ice clouds and minimize the time and spatial lag between the measurements to below 10 min and a few hundred meters. Thus, the RMSE (5.5 dB) and standard deviation (5.17 dB) of our data, which is twice the value claimed by Protat et al. (2009) (2–3 dB), are larger. However, the highly variable low clouds that are observed by each instrument differ due to the time shift and location mismatch of the platforms; thus ZC−Zsim (Fig. A2) is dependent on the distance to the underflight (time shift) and on the distance between both platforms (location shift). For measurements that are obtained within 30 km around the crossing location and when Polar 5 and CloudSat are closer than 35 km, the bias and standard deviation decrease to −0.37 and 3.18 dB, respectively.
Having shown good agreement between forward-simulated and measured reflectivities, we now focus on the vertical cloud fraction profile. Above 1.5 km, the profiles agree very well; CFC deviates from the CFsim profile by less than 5 pp (Fig. 6a, IV compared to CloudSat). This agreement worsens for lower altitudes; CFC is lower by 36 pp (16 pp) at 720 m (960 m) height, which is 60 % (31 %) of CFsim. This is consistent with the omission of signals by CloudSat due to a too-aggressive cloud mask, as discussed above. In summary, the comparison demonstrates that Zsim can be used as a good proxy for ZC above 1.5 km but that care has to be taken below, especially in the blind zone. This holds particularly for the maximum CFM of 50 % measured at 720 m, which CFsim overestimates, but CloudSat observations strongly underestimate.
Synthetic CloudSat reflectivity profiles (Zsim) are generated from the MiRAC observations carried out over the four campaigns (Tables 1) and serve as a base for assessing CloudSat's limitations. We first investigate the effect of these limitations on cloud fraction profiles (Sect. 5.1) derived from Zsim and specify the drivers for differences between forward simulations and “truth”. Furthermore, we analyze how much multilayer clouds (Sect. 5.2) and precipitation (Sect. 5.3) are affected. Note that these campaign measurements cannot be considered as a climatology; however, they provide unique data and insights into Arctic low-level clouds.
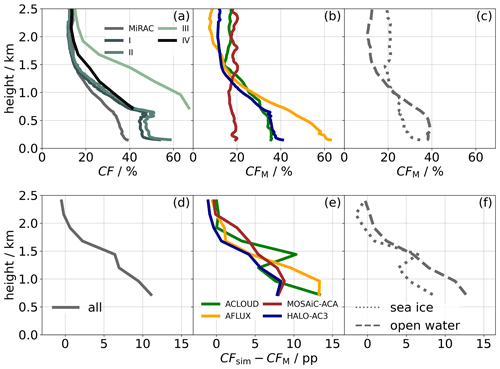
Figure 8Cloud fraction profiles from the airborne radar MiRAC (CFM) over four campaigns (a–c) and the difference compared to the forward-simulated profiles (CFsim; d–f). Profiles are averaged over all data (a, d), each campaign (b, e), and different surface covers (c, f). Sea ice concentrations below 15 % and above 90 % represent open water and sea ice. Moreover, the profiles after each processing step (I–IV; Sect. 2.3) are displayed in panel (a).
5.1 Cloud fraction profiles
Averaged over the four campaigns, the observed vertical cloud fraction profile (CFM) is 12 % for altitudes above 1.5 km and increases to 40 % towards the surface (Fig. 8a). The increase is strongest between 1.5 and 0.6 km, and the high values at MiRAC's lowest height of 150 m indicate frequent precipitation and probably very low clouds (Griesche et al., 2021). Excluding the blind zone, we average cloud fraction between 1 and 2.5 km and assess the impact of the different forward-simulation steps (Sect. 2.3). CF increases by 6 pp due to CloudSat's along-track convolution and integration (Fig. 8a, MiRAC compared to II), i.e., horizontal resolution; increases by 12 pp due to its range resolution (II compared to III); and decreases by 10 pp due to its sensitivity (III compared to IV). Vertically resolved, maximum effects of +25 pp (horizontal resolution), +20 pp (range resolution), and −30 pp (sensitivity) occur. The horizontal cloud cover between 1 and 2.5 km reduces by only 5 pp to 34 % during the forward simulation.
Mean CFM over the lowest 2.5 km varies between 17 % during MOSAiC-ACA and 25 % during AFLUX (Fig. 8b). This is even more pronounced below 1.25 km, where CFM differs between 20 % and 60 %, and might reflect a difference between autumn (MOSAiC-ACA) and spring (AFLUX). The profiles obtained during ACLOUD and HALO–(AC)3 resemble the mean profile over all campaigns. The shape of the CFM profile varies between the campaigns, again with the largest differences between AFLUX and MOSAiC-ACA. While MOSAiC-ACA features a roughly constant vertical CFM of around 20 % in the lower troposphere, AFLUX has the lowest CFM of about 10 % at higher altitudes, which strongly increases to 65 % towards the surface.
The average vertical profiles of the forward-simulated CFsim and measured CFM profiles show a similar shape (Fig. 8a, MiRAC and IV). However, the absolute difference between CFsim and CFM (Fig. 8d) reveals that CFsim is larger, and the difference increases towards the surface. At the lowest forward-simulated height (0.72 km), CFsim overestimates CFM by 11 pp; i.e., CloudSat would overestimate cloud fraction by one-third. The increasing overestimation towards the surface is evident for all campaigns (Fig. 8e), though differences of about 5 pp are evident. In particular, a peak in the overestimation at 1.5 km height occurs during ACLOUD (Fig. 8e) that might depend on differences in the cloud situation.
As already illustrated for the underflights (Sect. 4.1), CloudSat's lower vertical resolution shifts CFsim upwards vertically by roughly 240 m and stretches the clouds. In conclusion, low-level clouds are overestimated above the blind zone, but the dominant hydrometeor layer below 750 m is completely missed by the blind zone. CloudSat's performance, i.e., CFsim−CFM, does not show clear differences between the campaigns performed in different seasons. This might depend on the probed cloud types, their connection to different synoptic situations, and the way they are probed. In the following, we assess the dependence of CloudSat's performance on various parameters.
5.1.1 Surface cover
We analyze dissimilarities between CFM and CFsim over open water and sea ice (Fig. 8c and f). In general, cloud fraction profiles appear differently over sea ice, where they are relatively constant with height, and over open water, where higher levels have fewer and the lowest kilometer has more clouds. Over sea ice, a slight increase in CFM close to the surface occurs that might be related to very low-level clouds found by Griesche et al. (2021). CFsim overestimates CFM, especially below 1.75 km, with stronger overestimations closer to the ground (Fig. 8f). This overestimation is more pronounced over open water than over sea ice. Over ice, a second maximum in overestimation occurs at 1.5 km, where the cloud fraction is discontinuous.
5.1.2 Cold-air-outbreak index
CF is investigated for different M classes (Sect. 3.1). We only focus on CAOs and neutral conditions as too few cases for warm conditions exist, which would not allow valid conclusions to be drawn. During CAOs, CFM is close to 10 % above 1.5 km height (Fig. 9a) and increases linearly down to 1 km and more slowly until it reaches 50 %, close to the surface. In contrast, with about 18 %, CFM is more constant over height for neutral conditions. Then, no significant differences between sea ice and open water are visible, while differences up to 20 pp occur during CAOs. The latter differences vary with height similar to the overall difference between sea ice and open water (Fig. 8c). Clearly, CAOs are responsible for the highest low-level cloud fractions, with significant differences between measurements over ice and open water, where air-mass transformation changes cloud characteristics along the trajectory. The atmospheric boundary layer height increases with distance to the ice edge due to strong surface fluxes. Evaporation supports the cloud development from roll cloud streets close to the ice edge to cellular convection further downstream. CFsim overestimates CFM by up to 16 pp mainly during CAOs (Fig. 9c), when the coarse vertical resolution deepens the low-level cloud rolls over water, and less during neutral situations, when CFM is constant. The overestimation is strongest close to the surface, i.e., 14 and 9 pp during CAOs and neutral conditions, respectively. We speculate that the overestimation depends on cloud amount and orientation of the flight tracks in respect to the cloud streets as this influences the number of cloud gaps over which signals are averaged.
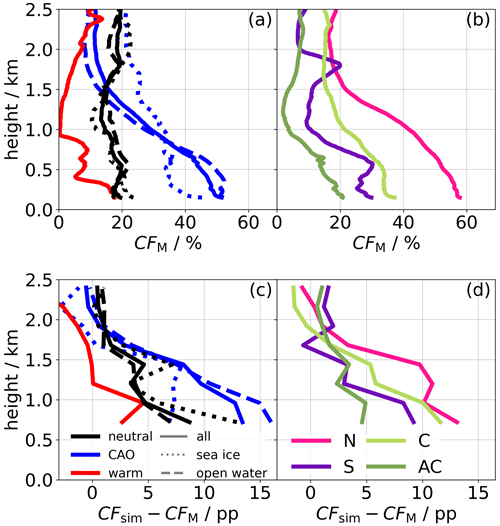
Figure 9Cloud fraction profiles from the airborne radar MiRAC (CFM) over four campaigns (a, b) and the difference compared to the forward-simulated profiles (CFsim; c, d). Profiles are averaged for different marine cold-air indices (M; a, c; solid lines): warm period (red), neutral period (black), and cold-air-outbreak indices (CAO; blue). The data are additionally categorized as being observed over sea ice (sea ice concentration, sic, < 15 %; dotted) and open water (sic > 90 %; dashed). Moreover, profiles are separated into circulation weather types (CWTs; b, d). N, S, C, and AC stand for northerly, southerly, cyclonic, and anticyclonic flow, respectively.
5.1.3 Circulation weather type
Cyclonic flows are the most frequent CWT (43 %) followed by northerly flows (Fig. 3). CFM shows a strong dependence on CWT, though for all regimes the highest cloud fraction occurs in CloudSat's blind zone (Fig. 9b). Northerly flows exhibit the largest CFM. During cyclonic conditions, the shape of the profile is similar, but CFM is lower. Both flows, particularly the northerly one, favor CAOs (Fig. 3) and associated cloud rolls. During southerly winds, non-precipitating clouds exist at different heights. CFM is generally lowest and often zero during anticyclonic conditions, which, however, are rare. Again CFsim overestimates CFM for all CWTs below 1.5 km. The effect is strongest during northerly conditions followed by cyclonic conditions. Although, CFM and its overestimation by CloudSat are largest during northerly winds, both seem to not be directly related to CWT; i.e., CFM is larger during AFLUX than HALO–(AC)3 regardless of CWT (not shown). In fact, CFM and the difference compared to the synthetic profiles are sorted in the same order, which implies a dependence on the amount of cloud fraction.
In conclusion, the errors imposed by CloudSat's limitations (CFsim−CFM) do not show a clear dependence on the surface type, M, or CWT but rather on cloud fraction and the shape of the profile. Significant errors only occur for clouds below 1.5 km. For the low-level cloud fraction we thus propose a simple correction in the form of a linear regression: the overestimation is 5 pp at 30 % cloud fraction and increases linearly to 15 pp for a cloud fraction of 60 %. While such a correction would reduce the overestimation of the vertically resolved cloud fraction with a residual uncertainty of about 5 pp (not shown), it has to be stressed that the blind zone neglects low-level clouds, which are the most common clouds in the Arctic (Fig. 6).
5.2 Multilayer clouds
The radiative characteristics of multilayer and single-layer cloud conditions often differ (Li et al., 2011). During ACLOUD, Mech et al. (2019) identified 38 % of the cloudy scenes as being composed of multilayer clouds that have a median thickness of 205 m. CloudSat might miss individual clouds due to its sensitivity, and its coarse resolution might merge separate hydrometeor layers to a single layer (Sect. 4.1). We investigate the overall effect on the frequency of multilayer cloud occurrence by defining a profile as containing a multilayer cloud for CloudSat if a gap of at least one range gate (240 m) occurs in the Zsim profile. For MiRAC a threshold of 90 m is used to take advantage of its finer resolution.
Averaged over all campaigns, 48 % of the cloud tops observed over all ZM profiles belong to multilayer clouds that have a mean thickness of 347 m (single-layer clouds: 762 m). During the forward simulations these multilayer clouds might merge to single-layer clouds. For Zsim only 12 % of the cloud tops belong to multilayer clouds, which have a mean thickness of 527 m. The coarse resolution deepens single-layer clouds by 140 m and multilayer clouds by 180 m. A total of 43 % of the observed multilayer cloud tops are below 1 km, which is less than for single-layer clouds (55 %); however, this implies that nearly every multilayer system has a layer with a cloud top below 1 km. With 48 % of all multilayer cloud tops, slightly more multilayer clouds are below 1 km for Zsim than for ZM.
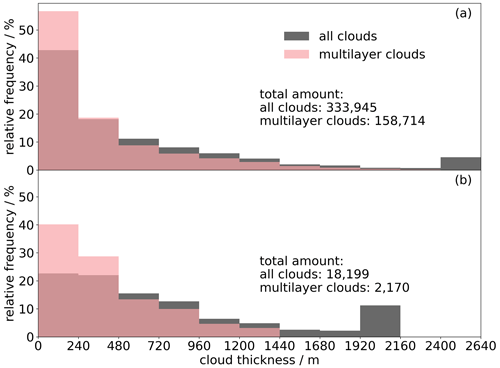
Figure 10Relative frequency of occurrence for the thickness over all (gray) and multilayer clouds (pink) derived from the equivalent radar reflectivity of the airborne radar MiRAC (ZM; a) and of the forward simulation (Zsim; b) over four campaigns. Note that MiRAC resolution is much finer but binned to match the one of CloudSat. The total number of all and multilayer clouds is displayed, with each radar reflectivity profile counting as an additional cloud.
The absolute number of cloudy ZM profiles containing medium-thickness (0.24–1.92 km) clouds reduces by a factor of 15 during the forward simulation (Fig. 10a and b, gray). For 240 m thick clouds, the factor is 2.3 times larger. Zsim does not detect more than twice as many shallow than medium-thickness clouds (Fig. 10b, gray). Furthermore, Zsim misses the thickest clouds. Because of the low vertical resolution, Zsim does not resolve the lowest 720 m of the atmosphere and thus thins the thickest clouds by 240 m to 2.16 km. The ratio between the number of clouds obtained by MiRAC and the forward simulations for clouds that are thicker than 1.92 km is 70 % of the averaged ratio due to cloud stretching.
For multilayer clouds only, the ratio between 480 and 240 m thick clouds increases for Zsim (Fig. 10a and b, pink). First, shallow clouds might get stretched. Second, Zsim might detect fewer thin clouds, which reduces the total number of cases. Furthermore, the absolute number of clouds over all cloud thicknesses excluding 240 m reduces by a factor of 68 during the forward simulation. The number of multilayer clouds diminishes 4 times as much as of all clouds due to the reduced resolution and sensitivity. Zsim could either not detect thin, second cloud layers anymore or merge multilayer to single-layer clouds. For 240 m thick clouds, the reduction factor of the number of clouds observed by MiRAC and the forward simulations is twice the average over the remaining cloud thicknesses. The detection omission is larger for shallow than for deeper clouds but decreases compared to all clouds. Thus, the detection omission of forward-simulated shallow clouds is a general shortcoming rather than one attributed to multilayer clouds. Hence, the merging of multiple cloud layers results in the 4-times-larger reduction factor of the number of multilayer clouds.
5.3 Precipitation
One of the most important applications of CloudSat is the derivation of snowfall in the Arctic. The Fram Strait is of particular interest as precipitation is most intense in this area (McCrystall et al., 2021), and snowfall estimates between CloudSat and regional climate models differ highly (von Lerber et al., 2022). From the “2C-Snow-Profile”, Edel et al. (2020) derived a mean snowfall rate (SC) of 200 to 500 mm yr−1 around Svalbard. The snowfall rate of the 2C-Snow-Profile product is calculated for bins that contain snow or snow-producing clouds via optimal estimation from snow size distribution parameters and uncertainties that are obtained by optimal estimation as well (Wood and L'Ecuyer, 2018). To calculate these snow size distribution parameters, radar reflectivity profiles of the 2B-Geoprof product and temperatures from ECMWF-AUX (European Centre for Medium Range Forecasts-AUXiliary), i.e., state variable data interpolated to the CPR grid, as well as a priori snow microphysical properties, radar scattering properties, and size distribution parameters are required as input. These microphysical parameters represent dry snow, and the scattering properties hold for irregularly shaped particles (Wood, 2011). However, SC, which is calculated for the near-surface bin at 1.2 km height, assumed to be the lowest bin not affected by ground clutter, deviates from the surface snowfall rate (Ssurf). Moreover, resolution limitations (Sect. 2.1) might affect SC.
We calculate snowfall rates from ZM (SM) and Zsim (Ssim) for Z larger than −5 dBZ via the Ze–S relation for three bullet rosettes following Maahn et al. (2014). Note that rosette habits might not capture the microphysical composition of oceanic snow-producing clouds under CAO conditions very well. We derive SM for all heights above 150 m to avoid ground clutter contamination for MiRAC (Sect. 2.2).
First, the effect of CloudSat's resolution on the Zsim and Ssim distributions is investigated at 1.2 km by comparing them with the respective ZM and SM distributions. Compared to ZM, the relative number of Zsim values between −5 and 3 dBZ is larger, and the number of stronger Zsim values is lower (Fig. 11a); i.e., CloudSat would overestimate very light snowfall and underestimate stronger snowfall. Z decreases during the spatial convolution. Note that ZM might fall below the threshold for precipitation ( dBZ) during the forward simulation, reducing the number of Ssim values. The histogram of snowfall rates shows that the number of Ssim and SM values decreases exponentially with their intensity (Fig. 11b). The relative number of Ssim values compared to SM values is larger for Ssim below 0.2 mm h−1, lower for Ssim between 0.2 and 2.0 mm h−1, and comparable for Ssim above 2 mm h−1. Due to its low resolution, CloudSat would overestimate low snowfall rates by 4 pp and underestimate higher rates by 4 pp.
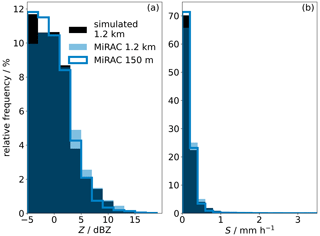
Figure 11Relative frequency of occurrence of the equivalent radar reflectivity (Z; a) and precipitation rate (S; b) for Z larger than −5 dBZ observed by the airborne radar MiRAC at 1.2 km (blue shade) and 150 m (blue line) and by the forward simulations at 1.2 km height (black shade) over four campaigns. The size of the bins equals 2 dBZ and 0.2 mm h−1.
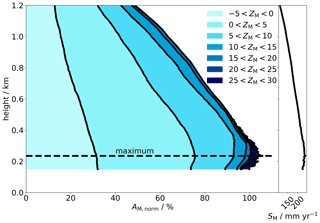
Figure 12Contribution from different intervals of equivalent radar reflectivity obtained from the airborne radar MiRAC (ZM) to the total precipitation amount over all campaigns (AM) with height. AM, norm is the integral over the snowfall rate (SM) for a specific height, which is calculated for ZM larger than −5 dBZ via the Ze–S relation for three bullet rosettes following Maahn et al. (2014), normalized by AM at 150 m, which is the nearest surface bin not affected by ground clutter. The dashed line at 235 m marks the height of maximal AM, norm. The profile of SM with height is shown in the right column.
We evaluate the influence of CloudSat's blind zone on its total precipitation amount (AC), which is the integral of the snowfall rate at a specific height over measurement time, following Maahn et al. (2014) but for ZM over ocean and sea ice. Over all campaigns, the total precipitation amount obtained from MiRAC (AM) is 1.0 mm (SM of 111 mm yr−1) at 1.2 km and with 2.1 mm (SM of 229 mm yr−1) more than twice as much at 150 m (Fig. 12). For a 1-year period at Ny-Ålesund, Maahn et al. (2014) found a larger Ssurf of 320 mm yr−1 using a ground-based radar, which might result from the choice of flight patterns that avoid storms and deep clouds. Due to its blind zone, CloudSat would underestimate AM at 150 m by 51 pp (Fig. 12), which is much stronger than for Ny-Ålesund (9 pp; Maahn et al., 2014).
To identify the ZM regime leading to the underestimation of AM caused by CloudSat's blind zone, AM is analyzed for different reflectivity classes (Fig. 12). Closest to the ground, with 90 %, light precipitation (ZM<10 dBZ) is the dominant contributor to AM. These reflectivities strongly increase from 1.2 km altitude down to 500 m and less below. ZM values between 10 and 20 dBZ equally contribute to AM over all heights. ZM values larger than 20 dBZ only occur below 400 m and contribute to AM more strongly closer to the ground. The total precipitation amount has its maximum at 235 m height because it strongly increases below 1.2 km, probably due to formation of light precipitation, and slightly decreases down to 150 m due to sublimation. The increase in occurrence of higher-reflectivity classes just above the maximum height is likely related to aggregation.
In this study, with 90 %, light precipitation (ZM<10 dBZ) plays a more important role than for Ny-Ålesund, where it is 35 % (Maahn et al., 2014), while moderate and strong precipitation is greatly reduced. We also find a lower height of maximum precipitation (235 vs. 600 m) and less sublimation (3 vs 20 pp). This might be related to the generally higher latitudes and colder conditions encountered during the flights. Moreover, the recorded cloud types favor light precipitation. In particular, many CAOs occurred throughout the campaigns (Fig. 3). At least 70 % of AM is measured during CAOs for all heights and ZM regimes. This ratio is higher at lower altitudes down to 250 m. During CAOs, the number of ZM values larger than 5 dBZ is so low that these ZM values do not enhance AM. In summary, CAOs produce mainly light precipitation, dominating AM.
Many studies use CloudSat observations to investigate Arctic clouds (Zygmuntowska et al., 2012; Liu et al., 2012; Mioche et al., 2015) and snowfall (von Lerber et al., 2022). However, CloudSat CPR has a blind zone of about 1 km, a coarse spatial resolution, and a limited sensitivity, which impact its usefulness in the assessment of warm marine-boundary-layer clouds and precipitation (Lamer et al., 2020). Our study extends this investigation for the Arctic using finely spatially resolved airborne radar reflectivity measurements by MiRAC obtained during four campaigns that took place over different seasons.
The measurements, which cover more than 25 000 km, are used to forward-simulate CloudSat measurements. During four underflights, these forward-simulated and CloudSat radar reflectivities agree within 2 dB; thus the forward simulation is a good proxy for CloudSat observations. The cloud fraction obtained by MiRAC over all campaigns is on average 30 %, with lower values of about 15 % at 2.5 km and a maximum of 40 % close to the ground. CloudSat's limitations increase the forward-simulated cloud fraction at 720 m by 11 pp, which is 33 % of the MiRAC cloud fraction. However, there are compensating effects at play: CloudSat's horizontal resolution increases the cloud fraction by a maximum of 25 pp and its range resolution by a maximum of 20 pp, and its sensitivity decreases the cloud fraction by a maximum of 30 pp. The lower spatial resolution fills cloud gaps, stretches clouds by 240 m at the cloud top and bottom, and hence increases the cloud fraction of the forward-simulated observations more strongly the closer to the ground. Our finding that MiRAC and CloudSat radar reflectivities differ substantially below 1.5 km supports the conclusion of Lamer et al. (2020) that the CPR cloud mask might be too restrictive, such that airborne remote sensing is necessary to resolve fine cloud structures and the lowest kilometer of the atmosphere.
We aimed to identify the drivers for CloudSat's over- and underestimations: fewer discrepancies between the forward-simulated and MiRAC cloud fraction occurred over sea ice than over open water. With 16 pp, the forward simulations overestimate the MiRAC cloud fraction most strongly over water during cold-air outbreaks, mostly due to cloud top stretching. Northerly flows, mainly connected with CAOs, show the highest low-level cloud fraction and overestimation by CloudSat. Therefore, we suggest a correction for profiles below 1.5 km that show fractions above 30 % which is simply a function of cloud fraction. In this way, the overestimation can be corrected roughly with a residual uncertainty of 5 pp. Note that cloud fractions and CloudSat's performance might depend on flight tracks.
This study confirms the finding of Kulie et al. (2016) and Kulie and Milani (2018) that CloudSat observes mainly light snow events at high latitudes during CAOs. The previous studies highlight the until then unresolved blind zone limitations. This study resolves these caveats on snowfall occurrence and amount that lead to an underestimation of the total precipitation amount by 51 pp. This finding hampers efforts to quantify snowfall, especially light snowfall during CAOs, with the best available spaceborne instruments. Moreover, CloudSat's pulse length merges layers of multilayer clouds; thus the number of multilayer clouds obtained by MiRAC (48 %) reduces by a factor of 4 during the forward simulations.
Additionally, some interesting insights on Arctic low-level clouds have been revealed: cloud fractions over sea ice showed a rather constant vertical profile, while low-level cloud formation strongly enhances cloud fraction over water up to around 1 km. The cloud fractions obtained by MiRAC indicate that low-level strati appear at their lowest heights over sea ice. These strati were also frequently found below 150 m during a Polarstern cruise taking place simultaneously with ACLOUD (Griesche et al., 2020). These surface-coupled clouds have a strong radiative effect, but their spatial extent is mainly unknown due to the gaps in the current observation system. Hence, further measurements are needed to study them in more detail (Griesche et al., 2021).
To generalize our findings to the broader Arctic region, further air- or shipborne measurements such as MOSAiC campaign data, which cover a larger area, have to be studied. Moreover, winter- and summertime observations are needed to determine cloud occurrence year-round. To mimic CloudSat observations more accurately, the resolution adaption of the finely resolved radar measurements should comprise an across-track convolution in the future as well. Follow-on studies could test the performance of the EarthCARE CPR to detect Arctic low-level clouds and complement the study of Lamer et al. (2020) for warm marine-boundary-layer clouds. Compared to the CloudSat CPR, the EarthCARE CPR is more sensitive and has the same range resolution (500 m; Burns et al., 2016). Due to the higher sensitivity but remaining cloud stretching effect of about 250 m, we expect that it will observe more clouds than CloudSat.
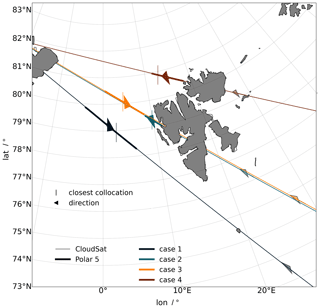
Figure A1Map highlighting the tracks of CloudSat (light colors) and Polar 5 (intense colors) during the four underflights (cases 1–4). The arrows and vertical lines indicate the flight direction of each platform and the location of the crossing, respectively.
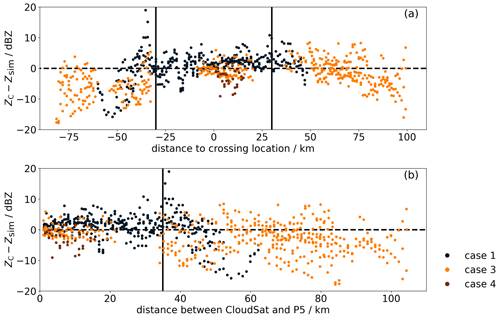
Figure A2Dependence of the difference between the forward-simulated and CloudSat equivalent radar reflectivities (ZC−Zsim) over four underflights of Polar 5 below CloudSat on distance to the crossing location (a) and distance between the platforms (b). Note that CloudSat resolves no signals during case 2.
The MiRAC, AMALi, and AMSR2 ARTIST Sea Ice (ASI) sea ice concentration data (version 5.4; provided by the University of Bremen) are accessed via the ac3airborne intake catalog (Mech et al., 2022a, https://doi.org/10.5281/zenodo.7305585). The MiRAC measurements during ACLOUD (Mech et al., 2022c), AFLUX (Mech et al., 2022c), and MOSAiC-ACA (Mech et al., 2022c) as well as the cloud top heights from AMALi during ACLOUD (Kulla et al., 2021a, https://doi.org/10.1594/PANGAEA.932454) and AFLUX (Kulla et al., 2021b, https://doi.org/10.1594/PANGAEA.932455) and the AMSR2 ASI observations (Melsheimer and Spreen, 2019, https://doi.org/10.1594/PANGAEA.898399) are stored on the PANGAEA database. Data that are not yet published are stored on the Nextcloud server of the (AC)3 project. The marine cold-air-outbreak indices and circulation weather types are calculated from ERA5 reanalysis data (Hersbach et al., 2020, https://doi.org/10.1002/qj.3803).
IS performed the analysis, visualization, and writing and developed and conducted the methodology. IS, SC, and MM conceptualized the paper. PK, KL, and LP provided CloudSat expertise and contributed to the algorithm that simulates the CloudSat observations. All authors contributed to manuscript revisions.
At least one of the (co-)authors is a member of the editorial board of Atmospheric Measurement Techniques. The peer-review process was guided by an independent editor, and the authors also have no other competing interests to declare.
Publisher's note: Copernicus Publications remains neutral with regard to jurisdictional claims in published maps and institutional affiliations.
We gratefully acknowledge the funding by the Deutsche Forschungsgemeinschaft (DFG, German Research Foundation) within the Transregional Collaborative Research Center (TRR 172) “ArctiC Amplification: Climate Relevant Atmospheric and SurfaCe Processes, and Feedback Mechanisms (AC)3” (project number 268020496, subproject B03). We acknowledge the support from the Alfred Wegener Institute and Polar 5 captains during the campaigns.
This research has been supported by the Deutsche Forschungsgemeinschaft (grant no. 268020496 – TRR 172).
This open-access publication was funded by Universität zu Köln.
This paper was edited by Stefan Kneifel and reviewed by two anonymous referees.
Akkermans, T., Böhme, T., Demuzere, M., Crewell, S., Selbach, C., Reinhardt, T., Seifert, A., Ament, F., and van Lipzig, N. P. M.: Regime-dependent evaluation of accumulated precipitation in COSMO, Theor. Appl. Climatol., 108, 39–52, https://doi.org/10.1007/s00704-011-0502-0, 2012. a, b
Atkinson, B. W. and Wu Zhang, J.: Mesoscale shallow convection in the atmosphere, Rev. Geophys., 34, 403–431, https://doi.org/10.1029/96RG02623, 1996. a
Barker, H. W., Korolev, A. V., Hudak, D. R., Strapp, J. W., Strawbridge, K. B., and Wolde, M.: A comparison between CloudSat and aircraft data for a multilayer, mixed phase cloud system during the Canadian CloudSat-CALIPSO Validation Project, J. Geophys. Res.-Atmos., 113, D00A16, https://doi.org/10.1029/2008JD009971, 2008. a
Blanchard, Y., Pelon, J., Eloranta, E. W., Moran, K. P., Delanoë, J., and Sèze, G.: A Synergistic Analysis of Cloud Cover and Vertical Distribution from A-Train and Ground-Based Sensors over the High Arctic Station Eureka from 2006 to 2010, J. Appl. Meteorol. Clim., 53, 2553–2570, https://doi.org/10.1175/JAMC-D-14-0021.1, 2014. a, b, c
Brümmer, B.: Roll and Cell Convection in Wintertime Arctic Cold-Air Outbreaks, J. Atmos. Sci., 56, 2613–2636, https://doi.org/10.1175/1520-0469(1999)056<2613:RACCIW>2.0.CO;2, 1999. a
Burns, D., Kollias, P., Tatarevic, A., Battaglia, A., and Tanelli, S.: The performance of the EarthCARE Cloud Profiling Radar in marine stratiform clouds, J. Geophys. Res.-Atmos., 121, 14,525–14,537, https://doi.org/10.1002/2016JD025090, 2016. a
Curry, J. A., Schramm, J. L., Rossow, W. B., and Randall, D.: Overview of Arctic Cloud and Radiation Characteristics, J. Climate, 9, 1731–1764, https://doi.org/10.1175/1520-0442(1996)009<1731:OOACAR>2.0.CO;2, 1996. a
Edel, L., Claud, C., Genthon, C., Palerme, C., Wood, N., L'Ecuyer, T., and Bromwich, D.: Arctic Snowfall from CloudSat Observations and Reanalyses, J. Climate, 33, 2093–2109, https://doi.org/10.1175/JCLI-D-19-0105.1, 2020. a
Ehrlich, A., Wendisch, M., Lüpkes, C., Buschmann, M., Bozem, H., Chechin, D., Clemen, H.-C., Dupuy, R., Eppers, O., Hartmann, J., Herber, A., Jäkel, E., Järvinen, E., Jourdan, O., Kästner, U., Kliesch, L.-L., Köllner, F., Mech, M., Mertes, S., Neuber, R., Ruiz-Donoso, E., Schnaiter, M., Schneider, J., Stapf, J., and Zanatta, M.: A comprehensive in situ and remote sensing data set from the Arctic CLoud Observations Using airborne measurements during polar Day (ACLOUD) campaign, Earth Syst. Sci. Data, 11, 1853–1881, https://doi.org/10.5194/essd-11-1853-2019, 2019. a, b
Etling, D. and Brown, R. A.: Roll vortices in the planetary boundary layer: A review, Bound.-Lay. Meteorol., 65, 215–248, https://doi.org/10.1007/BF00705527, 1993. a
Francis, J. A. and Hunter, E.: New insight into the disappearing Arctic sea ice, Eos T. Am. Geophys. Un., 87, 509–511, https://doi.org/10.1029/2006EO460001, 2006. a
Gayet, J.-F., Mioche, G., Dörnbrack, A., Ehrlich, A., Lampert, A., and Wendisch, M.: Microphysical and optical properties of Arctic mixed-phase clouds. The 9 April 2007 case study., Atmos. Chem. Phys., 9, 6581–6595, https://doi.org/10.5194/acp-9-6581-2009, 2009. a, b
Gierens, R., Kneifel, S., Shupe, M. D., Ebell, K., Maturilli, M., and Löhnert, U.: Low-level mixed-phase clouds in a complex Arctic environment, Atmos. Chem. Phys., 20, 3459–3481, https://doi.org/10.5194/acp-20-3459-2020, 2020. a
Graversen, R. G., Mauritsen, T., Tjernström, M., Källén, E., and Svensson, G.: Vertical structure of recent Arctic warming, Nature, 451, 53–56, https://doi.org/10.1038/nature06502, 2008. a
Griesche, H. J., Seifert, P., Ansmann, A., Baars, H., Barrientos Velasco, C., Bühl, J., Engelmann, R., Radenz, M., Zhenping, Y., and Macke, A.: Application of the shipborne remote sensing supersite OCEANET for profiling of Arctic aerosols and clouds during Polarstern cruise PS106, Atmos. Meas. Tech., 13, 5335–5358, https://doi.org/10.5194/amt-13-5335-2020, 2020. a
Griesche, H. J., Ohneiser, K., Seifert, P., Radenz, M., Engelmann, R., and Ansmann, A.: Contrasting ice formation in Arctic clouds: surface-coupled vs. surface-decoupled clouds, Atmos. Chem. Phys., 21, 10357–10374, https://doi.org/10.5194/acp-21-10357-2021, 2021. a, b, c, d
Haynes, J. M., Marchand, R. T., Luo, Z., Bodas-Salcedo, A., and Stephens, G. L.: A Multipurpose Radar Simulation Package: QuickBeam, B. Am. Meteorol. Soc., 88, 1723–1728, https://doi.org/10.1175/BAMS-88-11-1723, 2007. a
Hersbach, H., Bell, B., Berrisford, P., Hirahara, S., Horányi, A., Muñoz-Sabater, J., Nicolas, J., Peubey, C., Radu, R., Schepers, D., Simmons, A., Soci, C., Abdalla, S., Abellan, X., Balsamo, G., Bechtold, P., Biavati, G., Bidlot, J., Bonavita, M., De Chiara, G., Dahlgren, P., Dee, D., Diamantakis, M., Dragani, R., Flemming, J., Forbes, R., Fuentes, M., Geer, A., Haimberger, L., Healy, S., Hogan, R. J., Hólm, E., Janisková, M., Keeley, S., Laloyaux, P., Lopez, P., Lupu, C., Radnoti, G., de Rosnay, P., Rozum, I., Vamborg, F., Villaume, S., and Thépaut, J.-N.: The ERA5 global reanalysis, Q. J. Roy. Meteor. Soc., 146, 1999–2049, https://doi.org/10.1002/qj.3803, 2020. a, b
Intrieri, J. M. and Shupe, M. D.: Characteristics and Radiative Effects of Diamond Dust over the Western Arctic Ocean Region, J. Climate, 17, 2953–2960, https://doi.org/10.1175/1520-0442(2004)017<2953:CAREOD>2.0.CO;2, 2004. a
Kay, J. E. and L'Ecuyer, T.: Observational constraints on Arctic Ocean clouds and radiative fluxes during the early 21st century, J. Geophys. Res.-Atmos., 118, 7219–7236, https://doi.org/10.1002/jgrd.50489, 2013. a
Kneifel, S., von Lerber, A., Tiira, J., Moisseev, D., Kollias, P., and Leinonen, J.: Observed relations between snowfall microphysics and triple-frequency radar measurements, J. Geophys. Res.-Atmos., 120, 6034–6055, https://doi.org/10.1002/2015JD023156, 2015. a
Knudsen, E. M., Heinold, B., Dahlke, S., Bozem, H., Crewell, S., Gorodetskaya, I. V., Heygster, G., Kunkel, D., Maturilli, M., Mech, M., Viceto, C., Rinke, A., Schmithüsen, H., Ehrlich, A., Macke, A., Lüpkes, C., and Wendisch, M.: Meteorological conditions during the ACLOUD/PASCAL field campaign near Svalbard in early summer 2017, Atmos. Chem. Phys., 18, 17995–18022, https://doi.org/10.5194/acp-18-17995-2018, 2018. a
Kolstad, E. W.: Higher ocean wind speeds during marine cold air outbreaks, Q. J. Roy. Meteor. Soc., 143, 2084–2092, https://doi.org/10.1002/qj.3068, 2017. a
Kulie, M. S. and Bennartz, R.: Utilizing Spaceborne Radars to Retrieve Dry Snowfall, J. Appl. Meteorol. Clim., 48, 2564–2580, https://doi.org/10.1175/2009JAMC2193.1, 2009. a
Kulie, M. S. and Milani, L.: Seasonal variability of shallow cumuliform snowfall: A CloudSat perspective, Q. J. Roy. Meteor. Soc., 144, 329–343, https://doi.org/10.1002/qj.3222, 2018. a
Kulie, M. S., Milani, L., Wood, N. B., Tushaus, S. A., Bennartz, R., and L'Ecuyer, T. S.: A Shallow Cumuliform Snowfall Census Using Spaceborne Radar, J. Hydrometeorol., 17, 1261–1279, https://doi.org/10.1175/JHM-D-15-0123.1, 2016. a
Kulla, B. S., Mech, M., Risse, N., and Ritter, C.: Cloud top altitude retrieved from Lidar measurements during ACLOUD at 1 second resolution, PANGAEA [data set], https://doi.org/10.1594/PANGAEA.932454, 2021a. a, b
Kulla, B. S., Mech, M., Risse, N., and Ritter, C.: Cloud top altitude retrieved from Lidar measurements during AFLUX at 1 second resolution, PANGAEA [data set], https://doi.org/10.1594/PANGAEA.932455, 2021b. a, b
Lamer, K., Kollias, P., Battaglia, A., and Preval, S.: Mind the gap – Part 1: Accurately locating warm marine boundary layer clouds and precipitation using spaceborne radars, Atmos. Meas. Tech., 13, 2363–2379, https://doi.org/10.5194/amt-13-2363-2020, 2020. a, b, c, d, e, f, g, h, i, j, k
Li, J., Yi, Y., Minnis, P., Huang, J., Yan, H., Ma, Y., Wang, W., and Ayers, J. K.: Radiative effect differences between multi-layered and single-layer clouds derived from CERES, CALIPSO, and CloudSat data, J. Quant. Spectrosc. Ra., 112, 361–375, 2011. a
Liu, G.: Deriving snow cloud characteristics from CloudSat observations, J. Geophys. Res.-Atmos., 113, D00A09, https://doi.org/10.1029/2007JD009766, 2008. a
Liu, Y.: Impacts of active satellite sensors' low-level cloud detection limitations on cloud radiative forcing in the Arctic, Atmos. Chem. Phys., 22, 8151–8173, https://doi.org/10.5194/acp-22-8151-2022, 2022. a, b
Liu, Y., Key, J. R., Ackerman, S. A., Mace, G. G., and Zhang, Q.: Arctic cloud macrophysical characteristics from CloudSat and CALIPSO, Remote Sens. Environ., 124, 159–173, https://doi.org/10.1016/j.rse.2012.05.006, 2012. a, b, c
Liu, Y., Shupe, M. D., Wang, Z., and Mace, G.: Cloud vertical distribution from combined surface and space radar–lidar observations at two Arctic atmospheric observatories, Atmos. Chem. Phys., 17, 5973–5989, https://doi.org/10.5194/acp-17-5973-2017, 2017. a
Lubin, D. and Vogelmann, A. M.: A climatologically significant aerosol longwave indirect effect in the Arctic, Nature, 439, 453–456, https://doi.org/10.1038/nature04449, 2006. a
Maahn, M., Burgard, C., Crewell, S., Gorodetskaya, I. V., Kneifel, S., Lhermitte, S., Van Tricht, K., and van Lipzig, N. P. M.: How does the spaceborne radar blind zone affect derived surface snowfall statistics in polar regions?, J. Geophys. Res.-Atmos., 119, 13604–13620, https://doi.org/10.1002/2014JD022079, 2014. a, b, c, d, e, f, g, h, i
Marchand, R.: Level 2 GEOPROF Product Process Description and Interface Control Document, Product Version P1_R05, NASA JPL CloudSat project, document revision 0, 27 pp., https://www.cloudsat.cira.colostate.edu/cloudsat-static/info/dl/2b-geoprof/2B-GEOPROF_PDICD.P1_R05.rev0__0.pdf (last access: 6 July 2022), 2018. a, b, c, d
McCrystall, M. R., Stroeve, J., Serreze, M., Forbes, B. C., and Screen, J. A.: New climate models reveal faster and larger increases in Arctic precipitation than previously projected, Nat. Commun., 12, 6765, https://doi.org/10.1038/s41467-021-27031-y, 2021. a, b
Mech, M., Kliesch, L.-L., Anhäuser, A., Rose, T., Kollias, P., and Crewell, S.: Microwave Radar/radiometer for Arctic Clouds (MiRAC): first insights from the ACLOUD campaign, Atmos. Meas. Tech., 12, 5019–5037, https://doi.org/10.5194/amt-12-5019-2019, 2019. a, b, c, d, e
Mech, M., Risse, N., Marrollo, G., and Paul, D.: Ac3airborne, Zenodo [code], https://doi.org/10.5281/zenodo.7305585, 2022a. a, b
Mech, M., Ehrlich, A., Herber, A., Lüpkes, C., Wendisch, M., Becker, S., Boose, Y., Chechin, D., Crewell, S., Dupuy, R., Gourbeyre, C., Hartmann, J., Jäkel, E., Jourdan, O., Kliesch, L.-L., Klingebiel, M., Kulla, B. S., Mioche, G., Moser, M., Risse, N., Ruiz-Donoso, E., Schäfer, M., Stapf, J., and Voigt, C.: MOSAiC-ACA and AFLUX – Arctic airborne campaigns characterizing the exit area of MOSAiC, Scientific Data, 9, 790, https://doi.org/10.1038/s41597-022-01900-7, 2022b. a, b
Mech, M., Risse, N., Crewell, S., and Kliesch, L.-L.: Radar reflectivities at 94 GHz and microwave brightness temperature measurements at 89 GHz during the ACLOUD Arctic airborne campaign in early summer 2017 out of Svalbard, PANGAEA [data set], https://doi.org/10.1594/PANGAEA.945988, 2022c. a, b, c
Melsheimer, C. and Spreen, G.: AMSR2 ASI sea ice concentration data, Arctic, version 5.4 (NetCDF) (July 2012–December 2019), PANGAEA [data set], https://doi.org/10.1594/PANGAEA.898399, 2019. a
Mioche, G., Jourdan, O., Ceccaldi, M., and Delanoë, J.: Variability of mixed-phase clouds in the Arctic with a focus on the Svalbard region: a study based on spaceborne active remote sensing, Atmos. Chem. Phys., 15, 2445–2461, https://doi.org/10.5194/acp-15-2445-2015, 2015. a, b, c, d, e, f, g
Morrison, A. L., Kay, J. E., Frey, W. R., Chepfer, H., and Guzman, R.: Cloud Response to Arctic Sea Ice Loss and Implications for Future Feedback in the CESM1 Climate Model, J. Geophys. Res.-Atmos., 124, 1003–1020, https://doi.org/10.1029/2018JD029142, 2019. a
Painemal, D., Clayton, M., Ferrare, R., Burton, S., Josset, D., and Vaughan, M.: Novel aerosol extinction coefficients and lidar ratios over the ocean from CALIPSO–CloudSat: evaluation and global statistics, Atmos. Meas. Tech., 12, 2201–2217, https://doi.org/10.5194/amt-12-2201-2019, 2019. a
Palerme, C., Kay, J. E., Genthon, C., L'Ecuyer, T., Wood, N. B., and Claud, C.: How much snow falls on the Antarctic ice sheet?, The Cryosphere, 8, 1577–1587, https://doi.org/10.5194/tc-8-1577-2014, 2014. a
Palerme, C., Claud, C., Wood, N. B., L'Ecuyer, T., and Genthon, C.: How Does Ground Clutter Affect CloudSat Snowfall Retrievals Over Ice Sheets?, IEEE Geosci. Remote S., 16, 342–346, https://doi.org/10.1109/LGRS.2018.2875007, 2019. a
Papritz, L., Pfahl, S., Sodemann, H., and Wernli, H.: A Climatology of Cold Air Outbreaks and Their Impact on Air–Sea Heat Fluxes in the High-Latitude South Pacific, J. Climate, 28, 342–364, https://doi.org/10.1175/JCLI-D-14-00482.1, 2015. a, b
Philipp, A., Beck, C., Huth, R., and Jacobeit, J.: Development and comparison of circulation type classifications using the COST 733 dataset and software, Int. J. Climatol., 36, 2673–2691, https://doi.org/10.1002/joc.3920, 2016. a
Protat, A., Bouniol, D., Delanoë, J., O'Connor, E., May, P. T., Plana-Fattori, A., Hasson, A., Görsdorf, U., and Heymsfield, A. J.: Assessment of Cloudsat Reflectivity Measurements and Ice Cloud Properties Using Ground-Based and Airborne Cloud Radar Observations, J. Atmos. Ocean. Tech., 26, 1717–1741, https://doi.org/10.1175/2009JTECHA1246.1, 2009. a, b, c
Protat, A., Bouniol, D., O'Connor, E. J., Baltink, H. K., Verlinde, J., and Widener, K.: CloudSat as a Global Radar Calibrator, J. Atmos. Ocean. Tech., 28, 445–452, https://doi.org/10.1175/2010JTECHA1443.1, 2011. a
Risse, N., Marrollo, G., Paul, D., and Mech, M.: Ac3airborne – Flight-Phase-Separation, Zenodo [code], https://doi.org/10.5281/zenodo.7305558, 2022. a
Serreze, M. C. and Barry, R. G.: Processes and impacts of Arctic amplification: A research synthesis, Global Planet. Change, 77, 85–96, https://doi.org/10.1016/j.gloplacha.2011.03.004, 2011. a
Shupe, M. D. and Intrieri, J. M.: Cloud Radiative Forcing of the Arctic Surface: The Influence of Cloud Properties, Surface Albedo, and Solar Zenith Angle, J. Climate, 17, 616–628, https://doi.org/10.1175/1520-0442(2004)017<0616:CRFOTA>2.0.CO;2, 2004. a
Shupe, M. D., Matrosov, S. Y., and Uttal, T.: Arctic Mixed-Phase Cloud Properties Derived from Surface-Based Sensors at SHEBA, J. Atmos. Sci., 63, 697–711, https://doi.org/10.1175/JAS3659.1, 2006. a
Shupe, M. D., Rex, M., Blomquist, B., Persson, P. O. G., Schmale, J., Uttal, T., Althausen, D., Angot, H., Archer, S., Bariteau, L., Beck, I., Bilberry, J., Bucci, S., Buck, C., Boyer, M., Brasseur, Z., Brooks, I. M., Calmer, R., Cassano, J., Castro, V., Chu, D., Costa, D., Cox, C. J., Creamean, J., Crewell, S., Dahlke, S., Damm, E., de Boer, G., Deckelmann, H., Dethloff, K., Dütsch, M., Ebell, K., Ehrlich, A., Ellis, J., Engelmann, R., Fong, A. A., Frey, M. M., Gallagher, M. R., Ganzeveld, L., Gradinger, R., Graeser, J., Greenamyer, V., Griesche, H., Griffiths, S., Hamilton, J., Heinemann, G., Helmig, D., Herber, A., Heuzé, C., Hofer, J., Houchens, T., Howard, D., Inoue, J., Jacobi, H.-W., Jaiser, R., Jokinen, T., Jourdan, O., Jozef, G., King, W., Kirchgaessner, A., Klingebiel, M., Krassovski, M., Krumpen, T., Lampert, A., Landing, W., Laurila, T., Lawrence, D., Lonardi, M., Loose, B., Lüpkes, C., Maahn, M., Macke, A., Maslowski, W., Marsay, C., Maturilli, M., Mech, M., Morris, S., Moser, M., Nicolaus, M., Ortega, P., Osborn, J., Pätzold, F., Perovich, D. K., Petäjä, T., Pilz, C., Pirazzini, R., Posman, K., Powers, H., Pratt, K. A., Preußer, A., Quéléver, L., Radenz, M., Rabe, B., Rinke, A., Sachs, T., Schulz, A., Siebert, H., Silva, T., Solomon, A., Sommerfeld, A., Spreen, G., Stephens, M., Stohl, A., Svensson, G., Uin, J., Viegas, J., Voigt, C., von der Gathen, P., Wehner, B., Welker, J. M., Wendisch, M., Werner, M., Xie, Z., and Yue, F.: Overview of the MOSAiC Expedition: Atmosphere, Elementa: Science of the Anthropocene, 10, 00060, https://doi.org/10.1525/elementa.2021.00060, 2022. a
Stachlewska, I. S., Neuber, R., Lampert, A., Ritter, C., and Wehrle, G.: AMALi – the Airborne Mobile Aerosol Lidar for Arctic research, Atmos. Chem. Phys., 10, 2947–2963, https://doi.org/10.5194/acp-10-2947-2010, 2010. a
Stapf, J., Ehrlich, A., and Wendisch, M.: Influence of Thermodynamic State Changes on Surface Cloud Radiative Forcing in the Arctic: A Comparison of Two Approaches Using Data From AFLUX and SHEBA, J. Geophys. Res.-Atmos., 126, e2020JD033589, https://doi.org/10.1029/2020JD033589, 2021. a
Stephens, G. L., Vane, D. G., Boain, R. J., Mace, G. G., Sassen, K., Wang, Z., Illingworth, A. J., O'connor, E. J., Rossow, W. B., Durden, S. L., Miller, S. D., Austin, R. T., Benedetti, A., and Mitrescu, C.: THE CLOUDSAT MISSION AND THE A-TRAIN: A New Dimension of Space-Based Observations of Clouds and Precipitation, B. Am. Meteorol. Soc., 83, 1771–1790, https://doi.org/10.1175/BAMS-83-12-1771, 2002. a, b, c, d
Stephens, G. L., Vane, D. G., Tanelli, S., Im, E., Durden, S., Rokey, M., Reinke, D., Partain, P., Mace, G. G., Austin, R., L'Ecuyer, T., Haynes, J., Lebsock, M., Suzuki, K., Waliser, D., Wu, D., Kay, J., Gettelman, A., Wang, Z., and Marchand, R.: CloudSat mission: Performance and early science after the first year of operation, J. Geophys. Res.-Atmos., 113, D00A18, https://doi.org/10.1029/2008JD009982, 2008. a
Strong, C. and Rigor, I. G.: Arctic marginal ice zone trending wider in summer and narrower in winter, Geophys. Res. Lett., 40, 4864–4868, https://doi.org/10.1002/grl.50928, 2013. a
Tanelli, S., Durden, S. L., Im, E., Pak, K. S., Reinke, D. G., Partain, P., Haynes, J. M., and Marchand, R. T.: CloudSat's Cloud Profiling Radar After Two Years in Orbit: Performance, Calibration, and Processing, IEEE T. Geosci. Remote, 46, 3560–3573, https://doi.org/10.1109/TGRS.2008.2002030, 2008. a, b, c
von Lerber, A., Mech, M., Rinke, A., Zhang, D., Lauer, M., Radovan, A., Gorodetskaya, I., and Crewell, S.: Evaluating seasonal and regional distribution of snowfall in regional climate model simulations in the Arctic, Atmos. Chem. Phys., 22, 7287–7317, https://doi.org/10.5194/acp-22-7287-2022, 2022. a, b, c
Wendisch, M., Macke, A., Ehrlich, A., Lüpkes, C., Mech, M., Chechin, D., Dethloff, K., Velasco, C. B., Bozem, H., Brückner, M., Clemen, H.-C., Crewell, S., Donth, T., Dupuy, R., Ebell, K., Egerer, U., Engelmann, R., Engler, C., Eppers, O., Gehrmann, M., Gong, X., Gottschalk, M., Gourbeyre, C., Griesche, H., Hartmann, J., Hartmann, M., Heinold, B., Herber, A., Herrmann, H., Heygster, G., Hoor, P., Jafariserajehlou, S., Jäkel, E., Järvinen, E., Jourdan, O., Kästner, U., Kecorius, S., Knudsen, E. M., Köllner, F., Kretzschmar, J., Lelli, L., Leroy, D., Maturilli, M., Mei, L., Mertes, S., Mioche, G., Neuber, R., Nicolaus, M., Nomokonova, T., Notholt, J., Palm, M., Pinxteren, M. v., Quaas, J., Richter, P., Ruiz-Donoso, E., Schäfer, M., Schmieder, K., Schnaiter, M., Schneider, J., Schwarzenböck, A., Seifert, P., Shupe, M. D., Siebert, H., Spreen, G., Stapf, J., Stratmann, F., Vogl, T., Welti, A., Wex, H., Wiedensohler, A., Zanatta, M., and Zeppenfeld, S.: The Arctic Cloud Puzzle: Using ACLOUD/PASCAL Multiplatform Observations to Unravel the Role of Clouds and Aerosol Particles in Arctic Amplification, B. Am. Meteorol. Soc., 100, 841–871, https://doi.org/10.1175/BAMS-D-18-0072.1, 2019. a, b, c
Wendisch, M., Brückner, M., Crewell, S., Ehrlich, A., Notholt, J., Lüpkes, C., Macke, A., Burrows, J. P., Rinke, A., Quaas, J., Maturilli, M., Schemann, V., Shupe, M. D., Akansu, E. F., Barrientos-Velasco, C., Bärfuss, K., Blechschmidt, A.-M., Block, K., Bougoudis, I., Bozem, H., Böckmann, C., Bracher, A., Bresson, H., Bretschneider, L., Buschmann, M., Chechin, D. G., Chylik, J., Dahlke, S., Deneke, H., Dethloff, K., Donth, T., Dorn, W., Dupuy, R., Ebell, K., Egerer, U., Engelmann, R., Eppers, O., Gerdes, R., Gierens, R., Gorodetskaya, I. V., Gottschalk, M., Griesche, H., Gryanik, V. M., Handorf, D., Harm-Altstädter, B., Hartmann, J., Hartmann, M., Heinold, B., Herber, A., Herrmann, H., Heygster, G., Höschel, I., Hofmann, Z., Hölemann, J., Hünerbein, A., Jafariserajehlou, S., Jäkel, E., Jacobi, C., Janout, M., Jansen, F., Jourdan, O., Jurányi, Z., Kalesse-Los, H., Kanzow, T., Käthner, R., Kliesch, L. L., Klingebiel, M., Knudsen, E. M., Kovács, T., Körtke, W., Krampe, D., Kretzschmar, J., Kreyling, D., Kulla, B., Kunkel, D., Lampert, A., Lauer, M., Lelli, L., von Lerber, A., Linke, O., Löhnert, U., Lonardi, M., Losa, S. N., Losch, M., Maahn, M., Mech, M., Mei, L., Mertes, S., Metzner, E., Mewes, D., Michaelis, J., Mioche, G., Moser, M., Nakoudi, K., Neggers, R., Neuber, R., Nomokonova, T., Oelker, J., Papakonstantinou-Presvelou, I., Pätzold, F., Pefanis, V., Pohl, C., van Pinxteren, M., Radovan, A., Rhein, M., Rex, M., Richter, A., Risse, N., Ritter, C., Rostosky, P., Rozanov, V. V., Donoso, E. R., Saavedra Garfias, P., Salzmann, M., Schacht, J., Schäfer, M., Schneider, J., Schnierstein, N., Seifert, P., Seo, S., Siebert, H., Soppa, M. A., Spreen, G., Stachlewska, I. S., Stapf, J., Stratmann, F., Tegen, I., Viceto, C., Voigt, C., Vountas, M., Walbröl, A., Walter, M., Wehner, B., Wex, H., Willmes, S., Zanatta, M., and Zeppenfeld, S.: Atmospheric and Surface Processes, and Feedback Mechanisms Determining Arctic Amplification: A Review of First Results and Prospects of the (AC)3 Project, B. Am. Meteorol. Soc., 104, E208–E242, https://doi.org/10.1175/BAMS-D-21-0218.1, 2023. a
Wesche, C., Steinhage, D., and Nixdorf, U.: Polar Aircraft Polar 5 and Polar 6 Operated by the Alfred-Wegener-Institute, Journal of large-scale research facilities JLSRF, 2, 87, https://doi.org/10.17815/jlsrf-2-153, 2016. a
Winker, D. M., Pelon, J. R., and McCormick, M. P.: The CALIPSO mission: spaceborne lidar for observation of aerosols and clouds, Proc. SPIE 4893, Lidar Remote Sensing for Industry and Environment Monitoring III, https://doi.org/10.1117/12.466539, 2003. a
Winton, M.: Amplified Arctic climate change: What does surface albedo feedback have to do with it?, Geophys. Res. Lett., 33, L03701, https://doi.org/10.1029/2005GL025244, 2006. a
Wood, N. B.: ESTIMATION OF SNOW MICROPHYSICAL PROPERTIES WITH APPLICATION TO MILLIMETER-WAVELENGTH RADAR RETRIEVALS FOR SNOWFALL RATE, Diss., Colorado State University, 2011. a
Wood, N. B. and L'Ecuyer, T. S.: Level 2C Snow Profile Process Description and Interface Control Document, Product Version P1 R05, NASA JPL CloudSat project document revision 0., 26 pp., https://www.cloudsat.cira.colostate.edu/cloudsat-static/info/dl/2c-snow-profile/2C-SNOW-PROFILE_PDICD.P1_R05.rev0_.pdf (last access: 16 June 2023), 2018. a
Zygmuntowska, M., Mauritsen, T., Quaas, J., and Kaleschke, L.: Arctic Clouds and Surface Radiation – a critical comparison of satellite retrievals and the ERA-Interim reanalysis, Atmos. Chem. Phys., 12, 6667–6677, https://doi.org/10.5194/acp-12-6667-2012, 2012. a, b
- Abstract
- Introduction
- Data and methods
- Meteorological conditions during airborne campaigns
- CloudSat underflights
- Evaluation of CloudSat limitations during campaigns
- Conclusions and outlook
- Appendix A
- Data availability
- Author contributions
- Competing interests
- Disclaimer
- Acknowledgements
- Financial support
- Review statement
- References
- Abstract
- Introduction
- Data and methods
- Meteorological conditions during airborne campaigns
- CloudSat underflights
- Evaluation of CloudSat limitations during campaigns
- Conclusions and outlook
- Appendix A
- Data availability
- Author contributions
- Competing interests
- Disclaimer
- Acknowledgements
- Financial support
- Review statement
- References