the Creative Commons Attribution 4.0 License.
the Creative Commons Attribution 4.0 License.
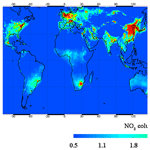
An improved total and tropospheric NO2 column retrieval for GOME-2
Song Liu
Pieter Valks
Gaia Pinardi
Isabelle De Smedt
Steffen Beirle
Andreas Richter
An improved algorithm for the retrieval of total and tropospheric nitrogen dioxide (NO2) columns from the Global Ozone Monitoring Experiment-2 (GOME-2) is presented. The refined retrieval will be implemented in a future version of the GOME Data Processor (GDP) as used by the EUMETSAT Satellite Application Facility on Atmospheric Composition and UV Radiation (AC-SAF). The first main improvement is the application of an extended 425–497 nm wavelength fitting window in the differential optical absorption spectroscopy (DOAS) retrieval of the NO2 slant column density, based on which initial total NO2 columns are computed using stratospheric air mass factors (AMFs). Updated absorption cross sections and a linear offset correction are used for the large fitting window. An improved slit function treatment is applied to compensate for both long-term and in-orbit drift of the GOME-2 slit function. Compared to the current operational (GDP 4.8) dataset, the use of these new features increases the NO2 columns by ∼1–3×1014 molec cm2 and reduces the slant column error by ∼24 %. In addition, the bias between GOME-2A and GOME-2B measurements is largely reduced by adopting a new level 1b data version in the DOAS retrieval. The retrieved NO2 slant columns show good consistency with the Quality Assurance for Essential Climate Variables (QA4ECV) retrieval with a good overall quality. Second, the STRatospheric Estimation Algorithm from Mainz (STREAM), which was originally developed for the TROPOspheric Monitoring Instrument (TROPOMI) instrument, was optimised for GOME-2 measurements to determine the stratospheric NO2 column density. Applied to synthetic GOME-2 data, the estimated stratospheric NO2 columns from STREAM shows good agreement with the a priori truth. An improved latitudinal correction is introduced in STREAM to reduce the biases over the subtropics. Applied to GOME-2 measurements, STREAM largely reduces the overestimation of stratospheric NO2 columns over polluted regions in the GDP 4.8 dataset. Third, the calculation of AMF applies an updated box-air-mass factor (box-AMF) look-up table (LUT) calculated using the latest version 2.7 of the Vector-LInearized Discrete Ordinate Radiative Transfer (VLIDORT) model with an increased number of reference points and vertical layers, a new GOME-2 surface albedo climatology, and improved a priori NO2 profiles obtained from the TM5-MP chemistry transport model. A large effect (mainly enhancement in summer and reduction in winter) on the retrieved tropospheric NO2 columns by more than 10 % is found over polluted regions. To evaluate the GOME-2 tropospheric NO2 columns, an end-to-end validation is performed using ground-based multiple-axis DOAS (MAXDOAS) measurements. The validation is illustrated for six stations covering urban, suburban, and background situations. Compared to the GDP 4.8 product, the new dataset presents improved agreement with the MAXDOAS measurements for all the stations.
- Article
(20052 KB) - Companion paper
-
Supplement
(3215 KB) - BibTeX
- EndNote
Nitrogen dioxide (NO2) is an important trace gas in the Earth's atmosphere. In the stratosphere, NO2 is strongly related to halogen compound reactions and ozone destruction (Solomon, 1999). In the troposphere, nitrogen oxides () serve as a precursor of zone in the presence of volatile organic compounds (VOCs) and of secondary aerosol through gas-to-particle conversion (Seinfeld et al., 1998). As a prominent air pollutant affecting human health and ecosystems, large amounts of NO2 are produced in the boundary layer by industrial processes, power generation, transportation, and biomass burning over polluted hot spots. For instance, a strong growth of NO2 during the past 2 decades has caused severe air pollution problems for China, with the largest NO2 columns in 2011; since then, cleaner techniques and stricter controlling have been applied to reduce the NO2 pollution (Richter et al., 2005; van der A et al., 2017; Liu et al., 2017). An increase in NO2 concentrations due to economic growth is also found over India, with a peak in 2012 (Hilboll et al., 2017). Despite the decrease in NOx emissions in Europe, around half of European Union member states still exceed the air quality standards, mainly caused by diesel car emissions (European Commission, 2017).
NO2 column measurements have been provided by satellite instruments, e.g. Global Ozone Monitoring Experiment (GOME) (Burrows et al., 1999), SCanning Imaging Absorption SpectroMeter for Atmospheric CHartographY (SCIAMACHY) (Bovensmann et al., 1999), Ozone Monitoring Instrument (OMI) (Levelt et al., 2006), and Global Ozone Monitoring Experiment-2 (GOME-2) (Callies et al., 2000; Munro et al., 2016). NO2 observations will be continued by the new generation instruments with high spatial resolution such as TROPOspheric Monitoring Instrument (TROPOMI) (launched in October 2017; Veefkind et al., 2012) and by geostationary missions such as Sentinel-4 (Ingmann et al., 2012). The GOME-2 instrument, which is the main focus of this study, is included on a series of MetOp satellites as part of the EUMETSAT Polar System (EPS). The first GOME-2 was launched in October 2006 aboard the MetOp-A satellite, and a second GOME-2 was launched in September 2012 aboard MetOp-B. The consistent long-term dataset will be further extended by the third GOME-2 on the upcoming MetOp-C platform (to be launched in September 2018). NO2 measurements from GOME-2 have been widely used to characterise the distribution, evolution, or transport of NO2 (e.g. Hilboll et al., 2013, 2017; Zien et al., 2014), to estimate the NOx emission (e.g. Gu et al., 2014; Miyazaki et al., 2017; Ding et al., 2017), and to interpret VOC levels, ozone variation, or anthropogenic aerosol loading (e.g. Vrekoussis et al., 2010; Safieddine et al., 2013; Penning de Vries et al., 2015).
The GOME-2 total and tropospheric NO2 products are generated using the GOME Data Processor (GDP) algorithm at the German Aerospace Center (DLR). The retrieval algorithm has been first described by Valks et al. (2011) as implemented in the GDP version 4.4 and was later updated to the current operational version 4.8 (Valks et al., 2017). The NO2 retrieval for GOME-2 follows a classical three-step scheme.
First, the total NO2 slant columns (namely the concentration integrated along the effective light path from the sun through the atmosphere to the instrument) are derived using the differential optical absorption spectroscopy (DOAS) method (Platt and Stutz, 2008). The DOAS technique is a least-squares method fitting the molecular absorption cross sections to the measured GOME-2 sun-normalised radiances provided by the EUMETSAT's processing facility. The fit is applied on the data within a fitting window optimised for NO2. As analysed by Richter et al. (2011) and in the Quality Assurance for Essential Climate Variables (QA4ECV; http://www.qa4ecv.eu) project, extension of the fitting window for GOME-2 increases the signal-to-noise ratio and hence improves the NO2 slant column error. The total NO2 slant columns depend on the viewing geometry and also on parameters such as surface albedo and the presence of clouds and aerosol loads. They are therefore converted to initial total NO2 vertical columns through division by a stratospheric air mass factor.
Second, the stratospheric contribution is estimated and separated from the NO2 slant columns (referred to as “stratosphere–troposphere separation”). The GDP 4.8 algorithm applies a modified reference sector method, which uses measurements over clean regions to estimate the stratospheric NO2 columns based on the assumptions of longitudinally invariable stratospheric NO2 layers and of negligible tropospheric NO2 abundance over the clean areas. The modified reference sector method defines a global pollution mask to remove potentially polluted regions and applies an interpolation over the unmasked areas to derive the stratospheric NO2 columns. As a result of using a fixed pollution mask, the modified reference sector method in GDP 4.8 has larger uncertainties over polluted areas, because limited amount of information over continents is used. To overcome the shortcomings, the STRatospheric Estimation Algorithm from Mainz (STREAM) method (Beirle et al., 2016) has been developed for the TROPOMI instrument and was also successfully applied on GOME, SCIAMACHY, OMI, and GOME-2 measurements. Also belonging to the modified reference sector method, STREAM defines not a fixed pollution mask but rather weighting factors for each observation to determine its contribution to the stratospheric estimation.
Third, the tropospheric NO2 vertical columns are calculated from the tropospheric slant columns by an air mass factor (AMF) calculation, which contributes the largest uncertainty to the NO2 retrieval, particularly over polluted regions (Boersma et al., 2004). The AMFs are determined with a radiative transfer model (RTM) and stored in a look-up table (LUT) requiring ancillary information such as surface albedo, vertical shape of the a priori NO2 profile, clouds, and aerosols. Improvements in the RTM and LUT interpolation scheme, the ancillary parameters, and the cloud and aerosol correction approach have been reported for the OMI instrument (e.g. Boersma et al., 2011; Lorente et al., 2017; Vasilkov et al., 2017; Krotkov et al., 2017; Veefkind et al., 2016; Lin et al., 2014; Castellanos et al., 2015; Laughner et al., 2018), which in principle are beneficial for similar satellite instruments like GOME-2.
In this paper, a new algorithm to retrieve the total and tropospheric NO2 for the GOME-2 instruments is described, which includes improvements in each of the three algorithm steps introduced above. The improved algorithm will be implemented in the next version of GDP (referred to as GDP 4.9 hereafter). We briefly introduce the GOME-2 instrument (Sect. 2) and the current operational (GDP 4.8) total and tropospheric NO2 retrieval algorithm (Sect. 3). We present the improvements to the DOAS slant column retrieval (Sect. 4), the stratosphere–troposphere separation (Sect. 5), and the AMF calculation (Sect. 6). Finally, we show an end-to-end validation of the tropospheric NO2 dataset using ground-based multiple-axis DOAS (MAXDOAS) datasets with different pollution conditions (Sect. 7).
GOME-2 is a nadir-scanning UV–VIS spectrometer aboard the MetOp-A and MetOp-B satellites (referred to as GOME-2A and GOME-2B throughout this study) with a satellite repeating cycle of 29 days and an equator crossing time of 19:30 local time (LT) (descending node). The GOME-2 instrument measures the Earth's backscattered radiance and extraterrestrial solar irradiance in the spectral range between 240 and 790 nm. The morning measurements from GOME-2 provide a better understanding of the diurnal variations of the NO2 columns in combination with afternoon observations from for example the OMI, and TROPOMI instruments (13:30 LT). The default swath width of GOME-2 is 1920 km, enabling a global coverage in ∼1.5 days. The default ground pixel size is 80 km ×40 km in the forward scan, which remains almost constant over the full swath width. In a tandem operation of MetOp-A and MetOp-B from July 2013 onwards, a decreased swath of 960 km and an increased spatial resolution of 40 km ×40 km are employed by GOME-2A. See Munro et al. (2016) for more details on instrument design and performance.
The operational GOME-2 NO2 product is provided by DLR in the framework of EUMETSAT's Satellite Application Facility on Atmospheric Composition Monitoring (AC-SAF). The product processing chain starts with the level 0 to 1b processing within the core ground segment at EUMETSAT in Darmstadt (Germany), where the raw instrument (level 0) data are converted into geolocated and calibrated (level 1b) (ir)radiances by the GOME-2 Product Processing Facility (PPF). The level 1b (ir)radiances are disseminated through the EUMETCast system to the AC-SAF processing facility at DLR in Oberpfaffenhofen (Germany) and further processed using the Universal Processor for UV/VIS Atmospheric Spectrometers (UPAS) system. Broadcasted via EUMETCast, WMO GTS, and the Internet, the resulting level 2 near-real-time total column products including NO2 columns can be received by user communities 2 h after sensing. Offline and reprocessed GOME-2 level 2 and consolidated products are also provided within 1 day by DLR, which can be ordered via FTP server and the EUMETSAT Data Centre (https://acsaf.org/, last access: 1 February 2019).
The first main step of the retrieval algorithm is the DOAS technique, which is applied to determine the total NO2 slant columns from the (ir)radiance spectra measured by the instrument. Based on the Beer–Lambert law, the DOAS fit is a least-squares inversion to isolate the trace gas absorption from the background processes, e.g. extinction resulting from scattering on molecules and aerosols, with a background polynomial P(λ) at wavelength λ:
The measurement-based term is defined as the natural logarithm of the measured earthshine radiance spectrum I(λ) divided by the daily solar irradiance spectrum I0(λ). The intensity offset correction offset(λ), which describes the additional contributions such as stray light in the spectrometer to the measured intensity, is modelled using a zero-order polynomial with the polynomial coefficient as the fitting parameter. The spectral effect from the absorption of species g is determined by the fitted slant column density Sg and associated absorption cross section σg(λ). An additional term with the Ring scaling factor αR and the Ring reference spectrum R(λ) describes the filling-in effect of Fraunhofer lines by rotational Raman scattering (the so-called Ring effect). The GDP 4.8 algorithm adopts a wavelength range of 425–450 nm to ensure prominent NO2 absorption structures and controllable interferences from other absorbing species, e.g. water vapour (H2Ovap), ozone (O3), and oxygen dimer (O4). Table 1 gives an overview of the DOAS settings for the current operational GDP 4.8 algorithm, the improved version 4.9 algorithm (see Sect. 4), and the algorithm used in the QA4ECV product (see Sect. 4.5).
Müller et al. (2016)Valks et al. (2011, 2017)Boersma et al. (2018)The second component in the retrieval is the calculation of initial total vertical column densities Vinit using a stratospheric AMF (Mstrat) conversion:
Given the small optical thickness of NO2, Mstrat can be determined as
with ml the box-air-mass factors (box-AMFs) in layer l, xl the altitude-dependent sub-columns from a stratospheric a priori NO2 profiles climatology (Lambert et al., 1999), and cl a correction coefficient to account for the temperature dependency of NO2 cross section (Boersma et al., 2004; Nüß et al., 2006). The calculation of Vinit assumes negligible tropospheric NO2 and hence uses only the stratospheric a priori NO2 profiles to derive AMF. The box-AMFs ml are derived using the multilayered multiple scattering LInearized Discrete Ordinate Radiative Transfer (LIDORT) RTM (Spurr et al., 2001) and stored in a LUT as a function of various model inputs b, including GOME-2 viewing geometry, surface pressure, and surface albedo. The surface albedo is described by the Lambertian equivalent reflectivity (LER). The surface LER climatology used in the GDP 4.8 algorithm is derived from combined TOMS–GOME measurements (Boersma et al., 2004) for the years 1979–1993 with a spatial resolution of 1.25∘ long. × 1.0∘ lat.
In the presence of clouds, the calculation of Mstrat adopts the independent pixel approximation based on GOME-2 cloud parameters:
with ω being the cloud radiance fraction, the cloudy-sky stratospheric AMF, and the clear-sky stratospheric AMF. and are derived with Eq. (3) with mainly relying on the cloud pressure and the cloud albedo. The ω value is derived from the cloud fraction cf:
where Icloud is the radiance for a cloudy scene and Iclear for a clear scene. Icloud and Iclear are calculated using LIDORT, depending mostly on the GOME-2 viewing geometry, surface albedo, and cloud albedo. From GOME-2, cf is determined with the Optical Cloud Recognition Algorithm (OCRA) by separating a spectral scene into cloudy contribution and cloud-free background, and the cloud pressure and the cloud albedo are derived using the Retrieval Of Cloud Information using Neural Networks (ROCINN) algorithm by comparing simulated and measured radiance in and near the O2 A band (Loyola et al., 2007, 2011). Applied in the NO2 retrieval in GDP 4.8, the latest version 3.0 of the OCRA (Lutz et al., 2016) applies a degradation correction on the GOME-2 level 1 measurements as well as corrections for viewing angle and latitudinal dependencies. A new cloud-free background is constructed from 6 years of GOME-2A measurements from the years 2008–2013. The updated OCRA also includes an improved detection and removal of sun glint that affects most of the GOME-2 orbits. Version 3.0 of ROCINN (Loyola et al., 2018) applies a forward RTM calculation using updated surface albedo climatology and spectroscopic data as well as a new inversion scheme based on Tikhonov regularisation (Tikhonov and Arsenin, 1977; Doicu et al., 2010). The computation time of ROCINN is optimised with a smart sampling method (Loyola et al., 2016).
The next retrieval step is the separation of stratospheric and tropospheric components from the initial vertical total columns, namely the stratosphere–troposphere separation. Since no direct stratospheric measurements are available for GOME-2, a spatial filtering algorithm is applied to estimate the stratospheric NO2 columns in GDP 4.8. The spatial filtering algorithm belongs to the modified reference sector method, which uses total NO2 columns over clean regions to approximate the stratospheric NO2 columns based on the assumption of longitudinally invariable stratospheric NO2 layers and of negligible tropospheric NO2 abundance over the clean areas. The spatial filtering algorithm uses a pollution mask to filter the potentially polluted areas (tropospheric NO2 columns larger than 1×1015 molec cm2), followed by a low-pass filtering (with a zonal 30∘ boxcar filter) on the initial total columns of the unmasked areas, and afterwards a removal of a tropospheric background NO2 (1×1014 molec cm2) from the derived stratospheric columns.
Finally, the tropospheric NO2 columns Vtrop can be computed as
where Mstrat is the stratospheric AMF in Eq. (3), Mtrop is the tropospheric AMF, and T is the tropospheric residues (). Mtrop is determined using Eqs. (3) and (4) with tropospheric a priori NO2 profiles. The calculation of Mtrop relies on the same model parameters as of Mstrat, but the dependency on the parameters like surface albedo and cloud properties as well as on the a priori NO2 profiles is much stronger. The GDP 4.8 adopts the tropospheric a priori NO2 profiles from a run of the global chemistry transport model MOZART version 2 (Horowitz et al., 2003) with anthropogenic emissions from the EDGAR2.0 inventory (Olivier et al., 1996) for the early 1990s. The monthly average vertical profiles are calculated from MOZART-2 data from the year 1997 for the overpass time of GOME-2 (09:30 LT) with a resolution of 1.875∘ long × 1.875∘ lat.
A larger 425–497 nm wavelength fitting window for the DOAS method (Richter et al., 2011) is implemented in the GDP 4.9 to retrieve the NO2 slant columns, which improves the signal-to-noise ratio by including more NO2 absorption structures. Compared to the extended 405–465 nm range, as employed by the QA4ECV GOME-2 NO2 product and used in the NO2 retrieval for OMI instrument (Boersma et al., 2002; van Geffen et al., 2015), the 425–497 nm fitting window has stronger sensitivity to NO2 columns in the boundary layer because the importance of scattering decreases with wavelength (Richter and verification team, 2015). In this study, the slant columns are derived using QDOAS software developed at the Belgian Institute for Space Aeronomy (BIRA-IASB) (Danckaert et al., 2015)1. Table 1 summarises the new settings of the GDP 4.9 algorithm.
4.1 Absorption cross sections
In the fitting window optimised for NO2 retrieval, the DOAS fit includes species with strong and unique absorption structures and describes their spectral effect using absorption cross sections from literature. In our GDP 4.9 algorithm, the absorption cross sections of NO2, H2Ovap, O3, and O4 are updated mainly with newly released datasets as
-
NO2 absorption at 220 K from Vandaele et al. (2002);
-
O3 absorption at 228 K from Brion et al. (1998);
-
H2Ovap absorption at 293 K from HITEMP (Rothman et al., 2010), rescaled as in Lampel et al. (2015);
-
O4 absorption at 293 K from Thalman and Volkamer (2013).
In addition, to compensate for the larger spectral interference from liquid water (H2Oliq), a H2Oliq absorption (Pope and Fry, 1997) is included to reduce systematic errors above ocean for the wider wavelength range. Two additional GOME-2 polarisation key data (EUMETSAT, 2009) are included to correct for remaining polarisation correction problems, particularly for GOME-2B:
-
H2Oliq absorption at 297 K from Pope and Fry (1997), smoothed as in Peters et al. (2014);
-
Eta and Zeta from GOME-2 calibration key data (EUMETSAT, 2009).
It is worth noting that our improved DOAS retrieval in the GDP 4.9 adopts a decreased temperature of NO2 cross section (220 instead of 240 K in GDP 4.8; Valks et al., 2017) for a consistency with other NO2 retrievals from GOME-2, OMI, and TROPOMI (Müller et al., 2016; Boersma et al., 2002; van Geffen et al., 2015, 2016), with a minor effect on the fit quality (∼0.02 %) from the two temperatures. Changing the temperature of the NO2 cross section from 240 to 220 K reduces the NO2 slant columns by ∼6 %–9 %, but this temperature dependency is corrected in the AMF and vertical column calculation (see Eq. 3).
The spectral signature of sand absorption has been investigated by Richter et al. (2011) for GOME-2 data, but it is not applied here because of the potential interference with the broadband liquid water structure (Peters et al., 2014), which might lead to non-physical results over the ocean.
4.2 Intensity offset correction
Besides the radiances backscattered by the Earth's atmosphere, a number of both natural (i.e. the Ring effect) and instrumental (e.g. stray light in the spectrometer and change of detector's dark current) sources contribute to an additional “offset” to the scattering intensity. To correct for this drift, an intensity offset correction with a linear wavelength dependency (i.e. polynomial degree of 1) is applied for the large fitting window in this study. Figure 1 illustrates the effect of using a linear intensity offset correction for the large fitting window on 3 March 2008. The use of a linear offset correction increases the NO2 columns by up to 3×1014 molec cm2 (17 %) and decreases the fitting residues (retrieval root mean square, rms) by up to 30 %. Larger differences are found at the eastern scans (eastern part of GOME-2 swath), possibly suggesting instrumental issues specific to GOME-2. For the retrieval rms, stronger improvements are mainly located above ocean, arguably from the compensation of inelastic vibrational Raman scattering in water bodies (Vountas et al., 2003).
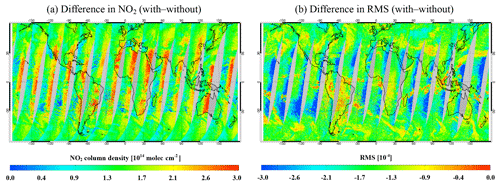
Figure 1Difference in NO2 columns (slant columns scaled by geometric AMFs) (a) and retrieval rms (b) estimated with and without a linear intensity offset correction for GOME-2A on 3 March 2008.
The intensity offset can also be fitted using only the constant term, as employed by the GDP 4.8 algorithm (with 425–450 nm wavelength window) and as recommended by the QA4ECV algorithm (with 405–465 nm). Compared to the use of the linear intensity offset correction, the application of a constant term on our retrieval shows a decrease in the NO2 columns by up to 3.5×1014 molec cm2 (17 %) and an increase in the retrieval rms by up to 14 %, which implies the necessity of using a linear intensity offset correction for the large 425–497 nm wavelength range.
4.3 GOME-2 slit function treatment
An accurate treatment of the instrumental slit function is essential for the wavelength calibration and the convolution of high-resolution laboratory cross sections. In spite of a generally good spectral stability of GOME-2 in orbit, the width of GOME-2 slit function has been changing on both long and short timescales (Munro et al., 2016), which needs to be accounted for in the DOAS analysis. In this study, an improved treatment of GOME-2 slit function in the DOAS fit is achieved by calculating effective slit functions from GOME-2 irradiance measurements to correct for the long-term variations (see Sect. 4.3.1) and by including an additional cross section in the DOAS fit to correct for the short-term variations (see Sect. 4.3.2).
4.3.1 Long-term variations
To analyse the long-term variations of the GOME-2 instrumental slit function and the impact on our retrieval, effective slit functions are derived by convolving a high-resolution reference solar spectrum (Chance and Kurucz, 2010) with a stretched preflight GOME-2 slit function and aligning to the GOME-2 daily irradiance measurements with stretch factors as fit parameters. The effective slit functions are calculated in 13 sub-windows covering the full fitting window (425–497 nm). Figure 2 displays the long-term evolution of the fitted GOME-2 slit function width (full width at half maximum, FWHM) calculated from the stretch factors. The GOME-2 slit function has narrowed after the launch by ∼5 % for GOME-2A and ∼3.5 % for GOME-2B at 451 nm, in agreement with Dikty et al. (2011), Azam et al. (2015), and Munro et al. (2016). For GOME-2A, visible discontinuities of the slit function width are related to the in-orbit instrument operations, including an apparent anomaly in September 2009 when a major throughput test was performed (EUMETSAT, 2012). After the throughput test, the narrowing of the slit function slowed down. For GOME-2B, stronger seasonal fluctuations of the FWHM are found. The seasonal and long-term variations in the GOME-2 slit function are caused by changing temperatures of the optical bench due to the seasonal variation in solar heating and the lack of thermal stability of the optical bench, respectively (Munro et al., 2016). Although the variations are only a few percent, the effect on the DOAS retrieval is significant. Compared to the application of the preflight slit function, the use of a stretched slit function improves the calibration residuals by ∼40 % for both GOME-2A and GOME-2B (not shown).
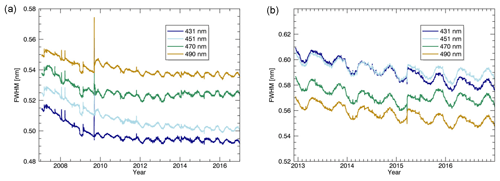
Figure 2Temporal evolution of the fitted slit function FWHM for GOME-2A (a, January 2007–December 2016) and GOME-2B (b, December 2012–December 2016.)
In previous studies, slit functions have also been fitted using various Gaussian shapes. For instance, De Smedt et al. (2012) have derived effective GOME-2 slit functions for formaldehyde retrieval using an asymmetric Gaussian with its width and shape as fit parameters. For NO2 retrieval, the use of effective slit functions with an asymmetric Gaussian leads to similar results as using a preflight slit function. In addition, Beirle et al. (2017) have proposed a slit function parameterisation using a super Gaussian, which is proved to quickly and robustly describe the slit function changes for satellite instrument OMI or TROPOMI. In the case of GOME-2, the super Gaussian obtains nearly identical results as the asymmetric Gaussian and is therefore not applied in here.
4.3.2 In-orbit variations
To correct for the in-orbit variations of GOME-2 slit function, a “resolution correction function” (Azam et al., 2015) is included as an additional cross section in the DOAS fit (see Table 1). The cross section is derived by dividing a high-resolution solar spectrum (Chance and Kurucz, 2010) convolved with a stretched preflight GOME-2 slit function (see Sect. 4.3.1) by itself but convolved with a slightly modified slit function. Figure 3 shows an example of the fit coefficients and the influence on our DOAS retrieval on 1 February 2013. As shown in the left panel, the slit function width increases along the orbit by nm (∼0.4 %) for GOME-2A (see Beirle et al., 2017, Fig. 8 therein) and nm (∼1 %) for GOME-2B (a fit coefficient of corresponds to a change in the slit function width of nm). This in-orbit broadening of the slit function is caused by the increasing temperature of the instrument along the orbit. Taking into account the in-orbit broadening in the DOAS fit decreases the retrieval rms by up to 5 % for GOME-2A and up to 12 % for GOME-2B in Fig. 3b.
4.4 GOME-2 level 1b data
As described in Sect. 2, the level 0 to 1b processing by the PPF at EUMETSAT calculates the geolocation and calibration parameters and produces the calibrated level 1b (ir)radiances. Due to the incomplete removal of Xe-line contamination in the GOME-2B calibration key data (calibration key data are taken during the on-ground campaign and required as an input to the level 0 to 1b processing), artefacts at wavelengths larger than 460 nm have been reported by Azam et al. (2015) for GOME-2B irradiances. Mainly focusing on the cleaning of contamination in the GOME-2B calibration key data, a new 6.1 version of the GOME-2 level 0 to 1b processor has been activated from 25 June 2015 onwards (EUMETSAT, 2015). To study the impact of the new level 1b data on our GDP 4.9 algorithm using the 425–497 nm fitting window, the retrieval is analysed using both the new 6.1 version (testing dataset provided by EUMETSAT for March 2015) and the previous version 6.0 data for the same period. Figure 4 presents a comparison of the retrieved NO2 columns over the Pacific for GOME-2A and GOME-2B. The application of the version 6.1 level 1b data slightly reduces the NO2 columns by ∼1–1.5×1014 molec cm2 (∼6 %–11 %) for GOME-2A. A larger effect is observed for GOME-2B with a decrease of NO2 columns by ∼3–4×1014 molec cm2 (∼15 %–23 %) and a reduction of rms error by ∼27 %–33 % (not shown). The stronger decrease of GOME-2B NO2 columns leads to a better consistency between the datasets from GOME-2A and GOME-2B with an overall bias reduced from molec cm2 to molec cm2.
4.5 Comparison to QA4ECV data
The quality of the GDP 4.9 retrieval is evaluated using the GOME-2 NO2 dataset from QA4ECV, which is a project aiming at quality-assured satellite products using a retrieval algorithm harmonised for GOME, SCIAMACHY, OMI and GOME-2. The GOME-2A NO2 columns from QA4ECV (version 1.1) for the years 2007–2015 have shown an improved quality over previous datasets (Zara et al., 2018). Table 1 gives an overview of the DOAS settings used in the QA4ECV project. Figure 5 shows a comparison of the NO2 columns over the Pacific from the GDP 4.8 algorithm, the GDP 4.9 algorithm, and the QA4ECV data for February 2007. For comparison, only ground pixels with a solar zenith angle smaller than 80∘ are considered. The GDP 4.8 dataset has been adjusted using a 220 K (Vandaele et al., 2002) NO2 cross section to remove the influence of temperature dependency of the NO2 cross section (see discussion in Sect. 4.1). Compared to the GDP 4.8 dataset, the improved DOAS retrieval in GDP 4.9 increases the NO2 columns by ∼1–3×1014 molec cm2 (up to 27 %). Compared to the QA4ECV product, a good overall consistency is found with the GDP 4.9 dataset at all latitudes considering the different DOAS settings such as fitting window, offset correction, and slit function characterisation.
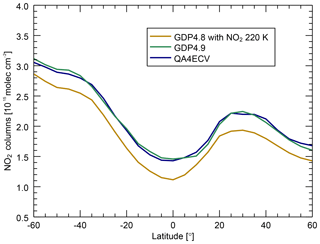
Figure 5Comparisons of monthly zonal average NO2 columns (slant columns scaled by geometric AMFs) from the operational GDP 4.8 product (but retrieved using a 220 K NO2 cross section from Vandaele et al., 2002) (brown), the improved GDP 4.9 algorithm (green), and the QA4ECV dataset (blue) over the Pacific (160–180∘ E) in February 2007 for GOME-2A.
Figure 6 presents the time series of calculated slant column errors from the three datasets, following a statistical method to analyse the NO2 slant column uncertainty for GOME-2 (Valks et al., 2011, Sect. 6.1 therein). The slant column errors, calculated as variations of NO2 measurements within small boxes () over the tropical Pacific (20∘S–20∘ N, 160–180∘ E), increase for all the three datasets as a result of instrument degradation (Dikty et al., 2011; Munro et al., 2016) until the major throughput test in September 2009 (see Sect. 4.3.1) and stabilise afterwards. Mainly driven by the use of a wider fitting window with stronger absorptions, the smallest slant column errors are found by the GDP 4.9 algorithm, e.g. 23.8 % smaller than from the GDP 4.8 and 13.5 % smaller than from the QA4ECV dataset in February 2007, with an increasing difference with time for the QA4ECV dataset (27.9 % in December 2015).
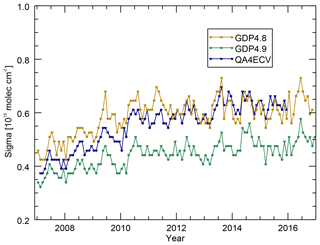
Figure 6Temporal evolution of the NO2 slant column errors from the operational GDP 4.8 product (brown, January 2007–December 2016), the improved GDP 4.9 algorithm (green, January 2007–December 2016), and the QA4ECV dataset (blue, February 2007–December 2015) for GOME-2A, using deviations of NO2 slant columns from box () mean values over the tropical Pacific (20∘ S–20∘ N, 160–180∘ E).
The calculation of tropospheric NO2 requires an estimation and removal of the stratospheric contribution to the initial total NO2 columns. In our GDP 4.9 retrieval, the stratosphere–troposphere separation algorithm STREAM (Beirle et al., 2016) has been adapted to GOME-2 measurements. Belonging to the modified reference sector method, STREAM uses initial total NO2 columns with negligible tropospheric contribution, i.e. unpolluted measurements at remote areas and cloudy measurements at medium altitudes, to derive the stratospheric NO2 columns. Based on a tropospheric NO2 climatology and the GOME-2 cloud product, STREAM calculates weighting factors for each satellite pixel to define the contribution of initial total columns to the stratospheric estimation; potentially polluted pixels are weighted low instead of being totally masked out in the GDP 4.8 spatial filtering method, cloudy observations at medium altitudes are given higher weights because they directly provide the stratospheric information, and the weights are further adjusted in a second iteration if pixels suffer from large biases in the tropospheric residues. Depending on these weighting factors, stratospheric NO2 fields are derived by weighted convolution on the daily initial total columns using convolution kernels. The convolution kernels are wider at lower latitudes due to the longitudinal homogeneity assumption of stratospheric NO2 and narrower at higher latitudes to reflect the stronger natural variations. To remove the biases in the weighted convolution resulting from the large latitudinal gradients, a latitudinal correction is applied on the initial total columns: the latitudinal dependencies of initial total NO2 are calculated over the clean Pacific, removed from the initial total NO2 before weighted convolution, and added back to the estimated stratospheric columns afterwards. However, we found that longitudinal variations of NO2 concentration resulted in biases in the latitudinal correction and hence in the stratospheric estimation. For the adaptation of STREAM to GOME-2 measurements, the performance of STREAM is analysed using synthetic GOME-2 NO2 observations (see Sect. 5.1), and an improved latitudinal correction is applied (see Sect. 5.2).
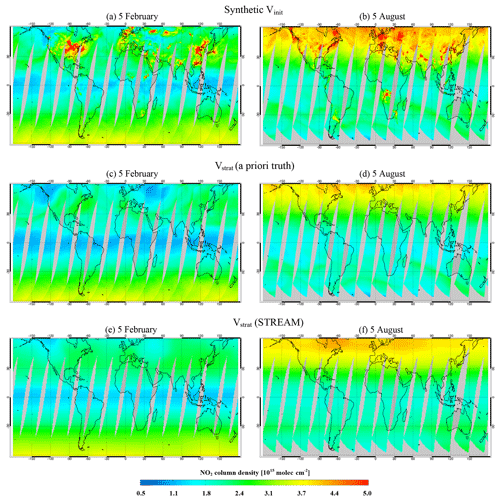
Figure 7Synthetic initial total NO2 columns (a, b), a priori stratospheric columns from C-IFS (c, d), and estimated stratospheric columns from STREAM (e, f) on 5 February (a, c, e) and 5 August (b, d, f) 2009.
5.1 Performance of STREAM
To test the performance of STREAM for GOME-2, simulated NO2 fields from the C-IFS-CB05-BASCOE (referred to as C-IFS throughout this work) experiment (Huijnen et al., 2016) are applied. The C-IFS model is a combination of tropospheric chemistry module in the Integrated Forecast System (IFS, with current version based on the Carbon Bond chemistry scheme, CB05) of the European Centre for Medium-Range Weather Forecasts (ECMWF) and stratospheric chemistry from the Belgian Assimilation System for Chemical ObsErvations (BASCOE) system. Based on 1 year of C-IFS data (2009) at a resolution of 0.75∘ long. × 0.75∘ lat., synthetic initial total columns Vinit are calculated as
(see Eq. 2). Modelled NO2 slant columns S are based on the total vertical columns Vtotal from C-IFS with interpolation to match the GOME-2 centre pixel coordinate and measurement time. Total AMFs Mtotal and stratospheric AMFs Mstrat are derived using Eqs. (3)–(5) with surface properties and cloud information from GOME-2 orbital data and with C-IFS a priori NO2 profiles for the whole atmosphere and between the tropopause (defined by a latitude-dependent parameterisation with the tropopause height ranging from 270 hPa for arctic to 92 hPa for tropics) and the top of the atmosphere, respectively. The performance of STREAM is evaluated by applying the synthetic initial total NO2 columns and comparing the estimated stratospheric NO2 columns with the a priori truth (stratospheric fields from C-IFS integrated between the tropopause and the top of the atmosphere).
Figure 7 displays the synthetic initial total columns from C-IFS, the modelled stratospheric columns, and the estimated stratospheric columns from STREAM on 5 February and 5 August 2009. The result from STREAM presents an overall smooth stratospheric pattern with a strong latitudinal and seasonal dependency resulting from photochemical changes and dynamical variabilities. Because the stratospheric values over polluted regions are taken from the clean measurements at the same latitude, the stratospheric and tropospheric contribution over polluted regions is well separated by STREAM, especially in the Northern Hemisphere. Due to the latitude-dependent definition of convolution kernels, STREAM conserves the longitudinal gradients of stratospheric NO2 at low latitudes and identifies certain strong stratospheric variations at high latitudes, e.g. in the polar vortex on 5 February. However, smaller structures in the synthetic initial total columns, for instance, resulting from the diurnal variation of NO2 across an orbital swath, are aliased into the troposphere by STREAM due to the use of convolution kernels.
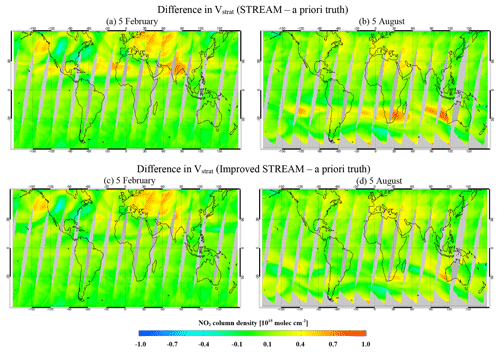
Figure 8Difference in the stratospheric NO2 columns estimated from STREAM and modelled by C-IFS on 5 February (a) and 5 August (b) 2009. Panels (c) and (d) show STREAM with improved latitudinal correction.
Figure 8a, b shows the differences in estimated (Fig. 7e, f) and a priori (Fig. 7c, d) stratospheric NO2. Overall, the stratospheric columns estimated from STREAM show good agreement with the modelled truth with a slight overestimation, e.g. by ∼1–2×1014 molec cm2 over low latitudes for both days. Larger differences are found at higher latitudes, especially in winter, e.g. by molec cm2 over eastern Europe and over the North Pacific (west of Canada) on 5 February. The strong longitudinal variations of NO2 over these regions in the a priori truth (Fig. 7c, d) can not be completely captured by STREAM (Fig. 7e, f), which is a general limitation of the modified reference sector method. Note that these larger differences are reduced to molec cm2 in monthly averages (not shown). The found deviations are in agreement with the uncertainty estimates in Beirle et al. (2016).
5.2 Improved latitudinal correction
In Fig. 8a, b, larger differences are noticeable over the subtropical regions in winter for both days, primarily related to the latitudinal correction used in STREAM. As described in the previous Sect. 5, the latitudinal correction is applied by determining the latitudinal dependencies of total NO2 over the clean Pacific, removing the latitudinal dependencies before convolution and adding it back to the estimated stratospheric columns. However, longitudinal variations of total NO2, for instance, enhanced total NO2 columns over the Pacific (compared to the Atlantic Ocean) at 15–30∘ N on 5 February 2009 (Fig. 7a), introduce biases in the stratospheric NO2 columns. Therefore, an improved latitudinal correction is introduced to reduce the biases over the subtropics. The new latitudinal correction determines the latitudinal dependencies of total NO2 based on clean measurements in the whole latitude band (the median of lowest NO2 columns for each 1∘ latitude band). Figure 8c, d shows the difference for the estimated stratospheric NO2 using the improved latitudinal correction. For both days, the application of the new latitudinal correction in STREAM largely removes the biases over the subtropics in Fig. 8a, b.
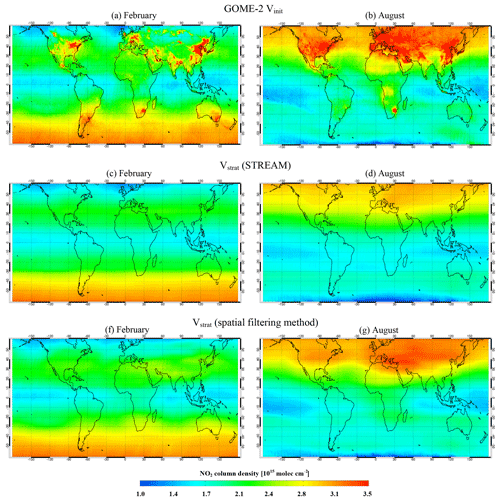
Figure 9GOME-2 initial total NO2 columns (a, b) and stratospheric NO2 columns retrieved from the improved STREAM algorithm (c, d) and from the spatial filtering method used in GDP 4.8 (e, f), measured by GOME-2A in February (a, c, e) and August (b, d, f) 2009.
Applying the improved STREAM on GOME-2 data, Fig. 9 presents the initial total columns from GOME-2 and the stratospheric NO2 calculated with STREAM and with the spatial filtering method used in the GDP 4.8 algorithm (see Sect. 3) in February and August 2009. For both months, the results calculated with STREAM and with the spatial filtering method show similar global structures. Since the spatial filtering method applies a fixed pollution mask to remove the potentially polluted regions (tropospheric NO2 larger than 1×1015 molec cm2), moderately polluted pixels with tropospheric NO2 up to 1×1015 molec cm2 still contribute to the stratospheric estimation. Therefore, enhanced stratospheric NO2 by more than 5×1014 molec cm2 is found over polluted regions, e.g. the Middle East, China, central Africa, southern Africa, and Australia in Fig. 9e, f. This overestimation is largely removed by STREAM in Fig. 9c, d.
Boersma et al. (2004)Tilstra et al. (2017b)6.1 RTM
As summarised in Table 2, updated box-AMFs are calculated using the linearised vector code VLIDORT (Spurr, 2006) version 2.7. VLIDORT applies the discrete ordinates method to generate simulated intensity and analytic intensity derivatives with respect to atmospheric and surface parameters (i.e. weighting functions). Box-AMFs ml (see Eq. 3) are determined as
with I being the simulated top-of-atmosphere radiance, the absorption optical thickness of NO2 at layer l, and term the NO2 profile weighting function. Compared to the scalar (intensity-only) LIDORT code, VLIDORT provides more realistic modelling results with a treatment of light polarisation, which affects the tropospheric AMFs by up to 4 %.
The box-AMFs ml for each layer are calculated for the midpoint wavelength of fitting window, i.e. 461 nm in our NO2 retrieval, which is representative of the window-averaged box-AMFs. Compared to the tropospheric AMFs at 440 nm (midpoint wavelength in GDP 4.8), the ones calculated at 461 nm are higher by up to 10 % for polluted situations, due to the wavelength dependency of Rayleigh scattering, in agreement with Boersma et al. (2018) (see Fig. 7 therein). Note that the uncertainty related to the wavelength dependency of the AMF is much smaller than the uncertainties introduced by surface albedo, a priori NO2 profile, cloud, and aerosol (see Sect. 6.4).
The ml value is calculated with the RTM and stored in a LUT as a function of GOME-2 viewing geometry, surface pressure, and surface albedo. Compared to the LUT used in the GDP 4.8, a new LUT is calculated with an increased number of reference points, e.g. for surface pressure (from 10 to 16) and for surface albedo (from 10 to 14), as well as vertical layers (from 24 to 60) to reduce the interpolation error (Lorente et al., 2017), leading to differences in tropospheric AMFs by up to 2 %.
6.2 Surface albedo
Surface albedo is an important parameter for an accurate retrieval of NO2 columns and cloud properties. The sensitivity of backscattered radiance to the boundary layer NO2 is strongly related to the surface albedo, especially over polluted areas. In the GDP 4.9, the surface LER climatology based on TOMS–GOME data (Boersma et al., 2004) has been replaced by one based on GOME-2 observations (Tilstra et al., 2017b). Using the degradation-corrected GOME-2 level 1 measurements, the GOME-2 surface LER is derived by matching the measurements in a pure Rayleigh scattering atmosphere without clouds. Compared to the TOMS–GOME LER climatology, the GOME-2 surface LER (version 2.1) dataset takes advantage of newer observations for 2007–2013, an increased spatial resolution of 1.0∘ long. × 1.0∘ lat. for standard grid cells and 0.25∘ long. × 0.25∘ lat. at coastlines (Tilstra et al., 2017a), and an improved treatment of cloud contaminated cells over the ocean.
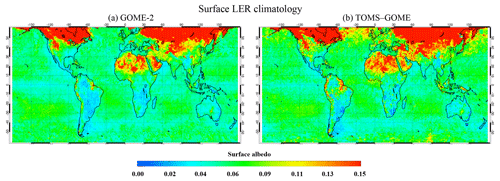
Figure 10Map of surface LER data for 440 nm in February based on GOME-2 observations for 2007–2013 (Tilstra et al., 2017b) (a) and TOMS–GOME data for 1979–1993 (b).
Figure 10 shows the surface LER data from the GOME-2 and TOMS–GOME observations for 440 nm in February. A good overall consistency is found between the two LER datasets, particularly over the ocean. Larger differences are found over certain snow or ice areas, like Russia and southern Canada, which can be attributed to changes in snow or ice cover during the different measurement periods of the two LER datasets. Increased spatial resolution for the GOME-2 LER version 2.1 dataset enables a better representation of surface features for the land–sea boundaries, e.g. coasts around western Europe and eastern China. Improvements in the GOME-2 LER algorithm (Tilstra et al., 2017b) decreases the surface LER values over regions with persistent clouds, e.g. the North Atlantic Ocean and the North Pacific Ocean at middle latitudes. Systematic differences in the LER climatologies are also caused by the different overpass time, observing geometry, and radiometric calibration of the instruments.
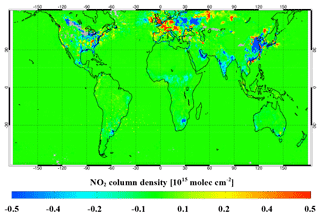
Figure 11Difference in tropospheric NO2 columns for clear-sky conditions (cloud radiance fraction smaller than 0.5) for February 2008 retrieved using the GOME-2 surface LER climatology version 2.1 and the LER climatology based on TOMS–GOME data at 440 nm.
Figure 11 illustrates the influence of the updated surface LER at 440 nm on the retrieved tropospheric NO2 columns in February 2008. The difference over the ocean is very small. Larger effects are noticed primarily under polluted conditions with positive differences, e.g. over parts of central Europe, Russia, or USA, and negative values, e.g. over parts of South Africa, India, or China. The differences in the retrieved tropospheric NO2 columns are consistent with the changes in the surface LER. For example, the GOME-2 surface LER over central Europe is ∼0.012 smaller than TOMS–GOME data, and a lower sensitivity to tropospheric NO2 is therefore assumed in the AMF calculation. This results in a decrease in the AMF and hence an increase in the retrieved tropospheric NO2 column by molec cm2 (∼12 %). Vice versa, an increase of the surface LER values by ∼0.018 over the Yangtze River region in eastern China leads to a reduction of tropospheric NO2 columns by molec cm2 (∼15 %).
As described in Sect. 6.1, the AMFs are calculated for 461 nm in the GDP 4.9 (425–497 nm wavelength window) instead of 440 nm in the GDP 4.8 (with 425–450 nm wavelength window), and therefore the corresponding surface LER values of 463 nm are used. The surface LER values at 463 nm are higher by up to 0.02 over desert areas and lower by up to 0.02 over the ocean and the snow or ice areas, which result in differences of up to 5 % in the calculated AMFs.
The surface LER climatology from Kleipool et al. (2008) derived from OMI measurements for 2004–2007 has been widely used in satellite NO2 retrievals (e.g. Boersma et al., 2011; Barkley et al., 2013; Bucsela et al., 2013). An important advantage of using the GOME-2 LER climatology with respect to the OMI LER dataset in our retrieval is the consistency with the GOME-2 NO2 observations, considering the illumination conditions, observation geometry, and instrumental characteristics. Another advantage of the GOME-2 LER climatology is the use of more recent observations to reduce the errors introduced by ignoring the interannual variability of surface albedo, which are possibly large for varying snow and ice situations. Possible corrections for the surface albedo from a climatology include the use of external information about the actual snow and ice conditions, e.g. from the Near-real-time Ice and Snow Extent (NISE) dataset (Nolin et al., 2005).
6.3 A priori vertical profiles
The retrieved tropospheric NO2 columns are sensitive to changes in the relative vertical distribution of the a priori NO2 concentrations (i.e. profile shape). Increasing the spatial and/or temporal resolution of the a priori profiles have shown to produce a more accurate NO2 retrieval (e.g. Russell et al., 2011; Heckel et al., 2011; McLinden et al., 2014; Nüß et al., 2006; Laughner et al., 2016). To improve the tropospheric AMF calculation, daily a priori NO2 profiles are obtained with a resolution of 1∘ long. lat. from the chemical transport model TM5-MP (Huijnen et al., 2010; Williams et al., 2017). The TM5-MP profiles have been used in several studies to derive AMFs and tropospheric NO2 columns (e.g. van Geffen et al., 2016; Lorente et al., 2017; Boersma et al., 2018).
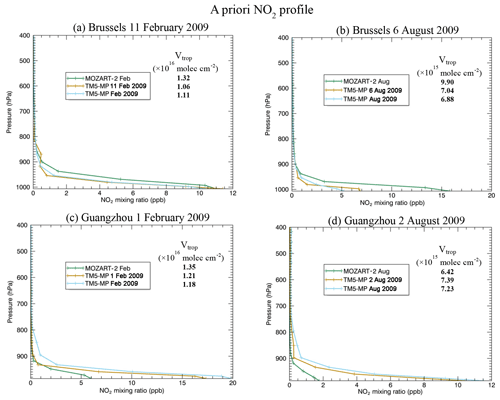
Figure 12Examples of a priori NO2 profiles for Brussels (a, b) and Guangzhou (c, d) on a given day in February (a, c) and August (b, d) 2009. Monthly profiles are shown for MOZART-2 (green), and daily profiles on the given days are shown for TM5-MP (brown) together with the monthly average profiles calculated for TM5-MP (blue). The tropospheric NO2 columns retrieved using each a priori NO2 profile are also given.
Figure 12 shows the TM5-MP and MOZART-2 a priori NO2 profiles for two pollution hot spots located in Brussels (Belgium, lat. 50.9, long. 4.4) and Guangzhou (China; lat. 23.1, long. 113.3) on 1 day in February and August 2009 as examples. Monthly profiles are shown for MOZART-2, and profiles for the given days are shown for TM5-MP. Large differences between the a priori NO2 profile shapes from TM5-MP and MOZART-2 are found for both cities. These differences are the result of the different chemical mechanism, transport scheme, and emission inventory employed by the model, the different spatial resolution, and the use of daily vs. monthly profiles. In TM5-MP, the use of an updated NOx emissions from the MACCity inventory (Granier et al., 2011) produces more realistic profiles. Improvement in the spatial resolution gives a more accurate description of the NO2 gradient and transport. The use of daily profiles provides a better description of the temporal NO2 variation, especially for regions dominated by emission and transport like Brussels and Guangzhou.
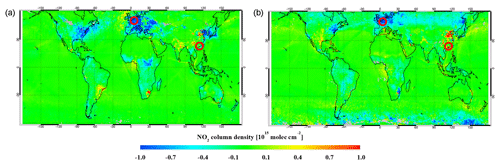
Figure 13Difference in tropospheric NO2 columns for clear-sky conditions (cloud radiance fraction smaller than 0.5) retrieved using daily TM5-MP and monthly MOZART-2 a priori NO2 profiles for February (a) and August (b) 2009. Red circles indicate locations in Fig. 12.
In Fig. 12, the tropospheric NO2 columns retrieved for the individual days using TM5-MP and MOZART-2 a priori NO2 profiles are also reported. Taking Brussels on 11 February 2009 (Fig. 12a) as an example, the smaller boundary layer concentration modelled by TM5-MP (less steep profile shape) leads to an increase in the tropospheric AMF and hence a decrease in the retrieved tropospheric NO2 columns by 2.6×1015 molec cm2 (19.7 %). Figure 13 presents a comparison of the monthly averaged tropospheric NO2 columns retrieved using daily TM5-MP and monthly MOZART-2 a priori NO2 profiles in February and August 2009. The application of the daily TM5-MP a priori NO2 profiles affects the tropospheric NO2 columns by more than 1×1015 molec cm2 mostly over polluted regions with enhanced NO2 in the boundary layer, e.g. with an increase of tropospheric NO2 over parts of China, India, and South Africa and a decrease over parts of the eastern US, Europe, and Japan.
To analyse the effect of using daily vs. monthly profiles, the tropospheric NO2 columns are also retrieved using the monthly average TM5-MP profiles, as shown in Fig. 12. Differences in the profile shape of daily and monthly profiles are mainly related to the variations in the meteorology. In agreement with Nüß et al. (2006) and Laughner et al. (2016), the use of monthly profiles changes the tropospheric NO2 columns by up to 3×1015 molec cm2 depending on the wind speed and wind direction, in particular for regions affected by transport (not shown). For the example of Brussels on 11 February 2009 (Fig. 12a), the use of monthly profiles increases the tropospheric NO2 columns by 5×1014 molec cm2 (4.7 %). A comprehensive analyse of the effect of using a priori NO2 profiles from different chemistry transport models on the retrieved tropospheric NO2 will be described in a subsequent paper.
6.4 Examples of GOME-2 tropospheric NO2
Figure 14 shows the tropospheric NO2 columns from the improved GDP 4.9 algorithm for February and August averaged for the year 2007–2016. Figure 15 shows the difference in tropospheric NO2 columns from the GDP 4.9 and GDP 4.8 product. The tropospheric NO2 columns increase globally by molec cm2 due to the improved DOAS slant column fitting and increase further by molec cm2 around moderately polluted regions benefitting from the use of new stratosphere–troposphere separation algorithm STREAM. A stronger change by more than 1×1015 molec cm2 is found mainly over polluted continents as a result of the improvements to the AMF calculation, primarily the surface albedo (which also affects the snow or ice area, e.g. southern Canada and northeastern Europe) and/or the a priori NO2 profiles (which also affect the polluted ocean, e.g. shipping lanes in southeastern Asia).
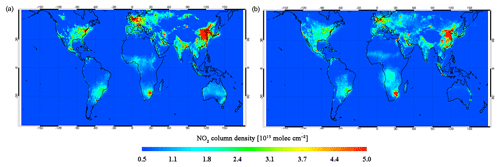
Figure 14Monthly average tropospheric NO2 columns from GDP 4.9 for clear-sky conditions (cloud radiance fraction smaller than 0.5), measured by GOME-2A in February (a) and August (b) 2007–2016.
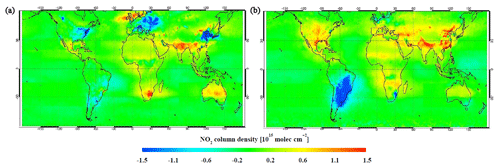
Figure 15Difference in tropospheric NO2 columns from GDP 4.9 and GDP 4.8 for clear-sky conditions (cloud radiance fraction smaller than 0.5) in February (a) and August (b) 2007–2016 for GOME-2A.
Over central northern Europe, the tropospheric NO2 columns are reduced by molec cm2 for GDP 4.9 in winter and molec cm2 in summer. A larger number of negative values in GDP 4.8, possibly related to the overestimated stratospheric NO2 around polar vortex areas, is largely corrected in GDP 4.9 by improving the stratosphere–troposphere separation algorithm. Over eastern China and eastern US, the seasonal variation is consistent between GDP 4.8 and 4.9, with reduced values in winter (by more than 1×1015 molec cm2) and enlarged values in summer (by more than 1×1015 molec cm2 for eastern China and 5×1014 molec cm2 for eastern US) for GDP 4.9 due to the combined impact of the algorithm changes, mainly the AMF calculation. Over India and its surrounding areas, a systematic increase in tropospheric NO2 columns by molec cm2 for GDP 4.9 benefits from the use of STREAM.
6.5 Uncertainty estimates for GOME-2 total and tropospheric NO2
The uncertainty in our GDP 4.9 NO2 slant columns is 4.4×1014 molec cm2, calculated from the average slant column error using a statistical method described in Sect. 4.5. The uncertainty in the GOME-2 stratospheric columns is ∼4–5×1014 molec cm2 for polluted conditions based on the daily synthetic GOME-2 data and ∼1–2×1014 molec cm2 for monthly averages. The uncertainty in the GDP 4.9 AMF calculation is likely reduced, considering the improved surface albedo climatology and a priori NO2 profiles, which are the main causes of AMF structural uncertainty (Lorente et al., 2017). In addition, the AMF uncertainty is substantially driven by the cloud parameters and the aerosol correction approach.
The largest cloud-related uncertainty in NO2 retrieval is introduced by the surface albedo–cloud fraction error correlation, as analysed by Boersma et al. (2018) for OMI using the OMCLDO2 cloud product, which requires a surface albedo climatology as input in the cloud fraction retrieval. But this uncertainty is likely smaller for OCRA and ROCINN cloud algorithms, since the surface albedo is treated differently in OCRA's cloud fraction calculation. Retrieved by separating a spectral scene into cloudy contribution and cloud-free background, the cloud fraction from OCRA is affected by surface albedo through the cloud-free map construction with a larger impact over bright surfaces like snow or ice cover, particularly during snowfall (higher background) or melting (lower background), which has been corrected by interpolating towards a daily value between two monthly cloud-free maps in OCRA (Lutz et al., 2016).
The uncertainty introduced by aerosol in GDP 4.9 is ∼50 % for high aerosol loading, in agreement with Lorente et al. (2017). With direct impact on NO2 AMF calculation and indirect impact via cloud parameter retrieval, the aerosol effect has been considered for OMI implicitly through the cloud correction (Boersma et al., 2004, 2011) or explicitly with additional aerosol information for regional studies (Lin et al., 2014, 2015; Kuhlmann et al., 2015; Castellanos et al., 2015; Chimot et al., 2018), leading to an increase or decrease of NO2 AMF by up to 40 % depending on NO2 distribution and aerosol properties and distribution. Since aerosol is highly variable in space and time due to the dependency on emission sources, transports, and atmospheric processes (Holben et al., 1991), explicit aerosol correction will be applied in our AMF calculation when reliable observations or model outputs of aerosol optical properties and vertical distributions are available. To conclude, the uncertainty in the AMF calculation is estimated to be in the 10 %–45 % range for polluted conditions, leading to a total uncertainty in the tropospheric NO2 columns likely in the range of 30 %–70 %.
The validation of NO2 data derived from the GOME-2 GDP algorithm is part of the validation activities done at BIRA-IASB in the AC-SAF context (Hassinen et al., 2016). An end-to-end validation approach is usually performed for each main release and summarised in validation reports that can be found on AC-SAF validation website (http://cdop.aeronomie.be/validation/valid-reports, last access: 1 February 2019). This includes several steps, such as
-
the DOAS analysis results, cloud property retrievals, and AMF evaluations by confrontation of GOME-2 retrievals to other established satellite retrievals and AMF evaluations;
-
the stratospheric reference evaluation by comparison with correlative observations from ground-based zenith-looking DOAS spectrometers and from other nadir-looking satellites; and
-
the tropospheric and total NO2 column data evaluation by comparison with correlative observations from ground-based MAXDOAS and direct-sun spectrometers (Pinardi et al., 2014).
In this paper, we focus on the last point: the validation of tropospheric data with BIRA-IASB ground-based MAXDOAS data. The MAXDOAS instruments collect scattered sky light in a series of line-of-sight angular directions extending from the horizon to the zenith. High sensitivity towards absorbers near the surface is obtained for the smallest elevation angles, while measurements at higher elevations provide information on the rest of the column. This technique allows the determination of vertically resolved abundances of atmospheric trace species in lowermost troposphere (Hönninger et al., 2004; Wagner et al., 2004; Wittrock et al., 2004; Heckel et al., 2005). Here the bePRO retrieval code (Clémer et al., 2010; Hendrick et al., 2014; Vlemmix et al., 2015) is used to retrieve tropospheric columns and low tropospheric profiles (up to 3.5 km with about 2 to 3 degrees of freedom).
As summarised in Table 3, a set of MAXDOAS stations (Beijing, Bujumbura, Observatoire de Haute-Provence (OHP), Réunion, Uccle, and Xianghe) is providing interesting test cases for GOME-2 sensitivity to tropospheric NO2. Indeed Beijing and Uccle are typical urban stations, Xianghe is a suburban station (∼60 km from Beijing), Bujumbura and Réunion are small cities in remote regions, and OHP is largely rural but occasionally influenced by polluted air masses transported from neighbouring cities. These different station types are important in the validation context as it is generally expected that urban stations are underestimated by the satellite data, due to the averaging of a local source over a pixel size (80 km ×40 km and 40 km ×40 km for GOME-2) larger than the horizontal sensitivity of the ground-based measurements which is about a few to tens of kilometres (Irie et al., 2011; Wagner et al., 2011; Ortega et al., 2015). In this context, MAXDOAS data are already better than in situ measurements with an extended horizontal and vertical sensitivity, more similar to the satellite sensitivity, but differences in sampling and sensitivity still remain and explain part of the biases highlighted by validation exercises. Several validation studies show a significant underestimation of tropospheric trace gases, such as NO2, from satellite observations over regions with strong spatial gradients in tropospheric pollution (e.g. Celarier et al., 2008; Kramer et al., 2008; Chen et al., 2009; Irie et al., 2012; Ma et al., 2013; Wu et al., 2013; Kanaya et al., 2014; Wang et al., 2017; Drosoglou et al., 2017, 2018). Other possible explanations include the uncertainties in the applied satellite retrieval assumptions, such as the choices of surface albedo, a priori NO2 profiles, or cloud and aerosol treatment (Boersma et al., 2004, 2011; Leitão et al., 2010; Heckel et al., 2011; Lin et al., 2014, 2015). The best agreement is generally obtained in the case of suburban and remote stations, but difficulties may arise when small local sources are present in a remote location, such as Réunion island or Bujumbura (Pinardi et al., 2015; Gielen et al., 2017).
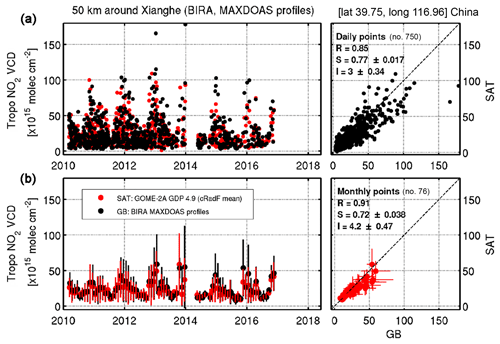
Figure 16Daily (a) and monthly mean (b) time series and scatter plots of GOME-2A and MAXDOAS tropospheric NO2 columns (mean value of all the pixels within 50 km around Xianghe).
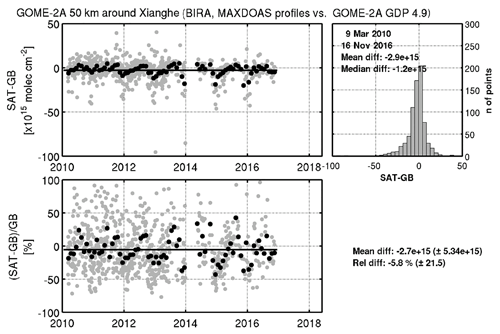
Figure 17Daily (grey dots) and monthly mean (back dots) absolute and relative GOME-2A and MAXDOAS time series differences for the Xianghe station. The histogram of the daily differences is also given, with the mean and median difference, and the total time-series absolute and relative monthly differences are given outside the panels.
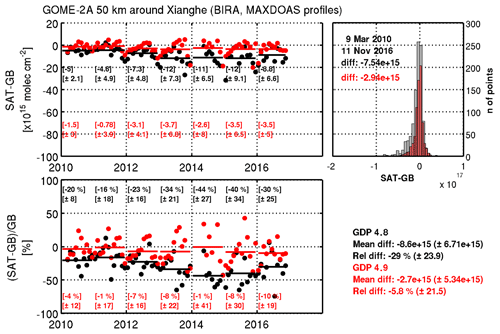
Figure 18Absolute and relative differences of GOME-2A and MAXDOAS tropospheric NO2 columns. The time series presents the monthly mean differences for GDP 4.8 (black) and GDP 4.9 (red). The total mean difference values and standard deviations are given, as well as the yearly values. The histogram presents the daily differences over the whole time series for the two products (grey for GDP 4.8 and red for GDP 4.9).
The same methodology as in the GDP 4.8 validation report (Pinardi et al., 2015) is used for the validation of this improved GDP 4.9 tropospheric NO2 dataset; the satellite data are filtered for clouds (cloud radiance fraction smaller than 0.5), and the mean value of all the valid pixels within 50 km of the stations is compared to the ground-based value. The original ground-based MAXDOAS data usually retrieve NO2 columns all day long every 20 to 30 min, and these values are linearly interpolated to the GOME-2 overpass time (09:30 LT) if original data exist within ±1 h.
Figure 16 shows an example of the time series and scatter plot of the daily and monthly mean comparison between GDP 4.9 tropospheric NO2 columns and ground-based MAXDOAS measurements in Xianghe, including the statistical information on the number of points, correlation coefficient, slope, and intercept of orthogonal regression analysis. Figure 17 presents the daily and monthly mean absolute and relative differences of GDP 4.9 and ground-based measurements. As can be seen in Figs. 16 and 17, the seasonal variation in the tropospheric NO2 columns is similarly captured by both observation systems, with differences on average within ±3 ×1015 molec cm2 (median difference of molec cm2). Larger differences are observed on some days and months, particularly in winter when NO2 and aerosol loadings are large. A relatively compact scatter is found, with a correlation coefficient of 0.91 and a slope of 0.72±0.04 for the orthogonal regression fit. These results are qualitatively similar to those obtained in previous validation exercises (Celarier et al., 2008; Kramer et al., 2008; Chen et al., 2009; Irie et al., 2012; Ma et al., 2013; Wu et al., 2013; Kanaya et al., 2014; Wang et al., 2017; Drosoglou et al., 2017, 2018). Similar figures for GDP 4.8 can be found on the AC-SAF validation website (http://cdop.aeronomie.be/validation/valid-results, last access: 1 February 2019).
Figure 18 reports the monthly mean absolute and relative differences for both GDP 4.8 and GDP 4.9 for Xianghe station. The daily differences are also reported through the histogram panel, where the reduction in the spread of the daily comparison points is clearly visible for GDP 4.9. The reduction of the bias, which is smaller and more stable in time, is seen in the absolute and relative monthly mean bias time series. A total of 3 years show a standard deviation of the monthly biases larger for GDP 4.9 than for GDP 4.8 (±12 % instead of ±8 % in 2010, ±12 % instead of ±8 % in 2013, and ±41 % instead of ±27 % in 2014) but with a strongly reduced mean bias (−4 % instead of −20 %, −8 % instead of −34 %, and −1 % instead of −44 %).
Similar figures as Figs. 16 and 18 for all the stations are gathered in Figs. S1 to S4 in the Supplement, and all the statistics are summarised in Tables 4 and 5 for GOME-2A and GOME-2B, respectively. Figures S1 and S2 in the Supplement present the time series and scatter plots for GDP 4.9, while Figs. S3 and S4 in the Supplement present the differences for both GDP 4.9 and GDP 4.8 comparisons. As discussed in Pinardi et al. (2015), for background stations (here Bujumbura, Réunion, and OHP), the mean bias is considered the best indicator of the validation results, due to the relatively small variability in the measured NO2. In urban (Beijing and Uccle) and suburban (Xianghe) situations, the NO2 variability is large enough and in this case, the correlation coefficient is a good indication of the linearity or coherence of the satellite and ground-based dataset, although a larger difference in terms of slope (closer to 0.5 than to 1 for urban cases) and mean bias can be expected because satellite measurements (and especially GOME-2 80 km ×40 km and 40 km ×40 km pixels) smooth out the local NO2 hot spots. This can be seen, e.g. in the cases of Beijing and Xianghe for GOME-2A (see Fig. S1a in the Supplement and Fig. 16, respectively), where very high correlations (R=0.94 and 0.91, respectively) are obtained from GDP 4.9, showing the very consistent behaviour of both datasets for small and large NO2 columns, while their slopes (S=0.4 and 0.72, respectively) show almost a factor of 2 difference, with a smaller slope in the Beijing case, where the MAXDOAS instrument is in the city centre and thus much more subject to local emission smeared out by the GOME-2 large pixel. This last effect is also seen through the bias values (RD = −47 % and −5.8 %, respectively) that are strongly reduced when moving the MAXDOAS outside the city in a suburban location like Xianghe. A slope of 0.47 (similar to the 0.4 of Beijing) is also obtained in Uccle, another urban site, where the MAXDOAS is affected by local emissions.
In remote cases such as OHP, Bujumbura, or Réunion island, as discussed above, the variation of the NO2 columns is small and the statistical analysis on the regression is not very representative of the situation, with a cloud of points giving small slopes and low correlation coefficients (see e.g. Fig. S1b–d in the Supplement and Table 4 for GOME-2A). In those cases, GOME-2 is lower than the ground-based measurements, with sometimes almost no seasonal variation, e.g. Bujumbura and Réunion, and in other cases, like OHP, some of the daily peaks are captured by GOME-2 (as days in the winter of 2014 and 2015), and the seasonal patterns and the orders of magnitude of both datasets are similar. In these cases, it is best to look at the absolute biases (as relative biases are large due to the division with small ground-based columns), as presented in e.g. Fig. S3b–d and Table 4. Mean absolute differences for GDP 4.9 are about molec cm2 for Bujumbura, molec cm2 for OHP, and molec cm2 for Réunion, which are all smaller than their respective GDP 4.8 values. The daily differences presented in the histograms of those figures also show reduced spread of GDP 4.9 comparisons when superposed to the GDP 4.8 results. Similar differences are also found for GOME-2B.
To conclude, although the Xianghe case presented in Figs. 16 to 18 is the best case (due to its suburban location and its long time series), better seasonal agreement between GDP 4.9 and MAXDOAS data is found for urban and suburban cases like Beijing, Uccle, and Xianghe, compared to results with GDP 4.8. In remote locations such as OHP, which is occasionally influenced by polluted air masses transported from neighbouring cities, the comparison is also meaningful (e.g. with a mean bias reduced from −45 % for GDP 4.8 to −25 % for GDP 4.9 for GOME-2A), while cases such as Bujumbura and Réunion are quite challenging for satellite validation, with specific local conditions (Bujumbura is in a valley on the side of Lake Tanganyika, while the MAXDOAS at Réunion is in St-Denis, on the coast of the 65 km long and 50 km wide island in the Indian Ocean, containing a mountain massif with summits above 2740 m a.s.l.). In both cases the MAXDOAS instrument is located in small cities surrounded by specific orography, difficult for satellite retrievals and challenging for validation. The absolute and relative differences show, however, a clear improvement for all the stations when comparing to GDP 4.8 results for both daily and monthly mean biases. The daily biases and spreads are all reduced.
To summarise, the impact of the improvement of the algorithm (as seen in Tables 4 and 5 and in Figs. S3 and S4 in the Supplement) leads to a decrease of the relative differences in urban conditions such as in Beijing or Uccle from % for GDP 4.8 to % for GDP 4.9 for GOME-2A and from −54 % to −40 % for GOME-2B. In suburban conditions such as in Xianghe, the differences go from −30 % to −6 % for GOME-2A and from −26 % to −2 % for GOME-2B. In remote (difficult) cases such as in Bujumbura or Réunion, the differences go from % to % for GOME-2A and from % to % for GOME-2B, while in background cases such as in OHP, the differences decrease from −45 % to −25 % for GOME-2A and from −42 % to −17 % for GOME-2B. The differences in numbers for GOME-2A and GOME-2B are due to the different time-series lengths of both comparisons (e.g. March 2010–November 2016 for GOME-2A and December 2012–November 2016 for GOME-2B in Xianghe), the different sampling of the atmosphere by GOME-2A and GOME-2B (slight time delay between both overpasses and reduced swath pixels for GOME-2A since July 2013), and the impact of the decreasing quality of the satellite in time, i.e. the GOME-2A degradation (Dikty et al., 2011; Munro et al., 2016). This lead, e.g. for Xianghe, to −2 % bias and 0.49 slope for GOME-2B compared to −6 % and 0.72 for GOME-2A for GDP 4.9.
These comparisons results aim at showing how the final GDP 4.9 product is improved compared to its predecessor, and not to summarise the improvements of each of the changes discussed in previous sections. In addition, the specific validation method could be improved or at least better characterised (including results uncertainties), by, e.g. changing the colocation method (averaging the MAXDOAS within 1 h of the satellite overpass or selecting the closest satellite pixel, or only considering the pixels containing the station, etc.), but this is out of the scope of the present paper that wants to compare to standard validation results performed routinely on GDP 4.8 (and publicly available at http://cdop.aeronomie.be/validation/valid-results, last access: 1 February 2019).
Table 4Averaged absolute differences (AD, SAT-GB in 1015 molec cm2), relative differences (RD, (SAT-GB)/GB in %), standard deviation (SD), correlation coefficient R, and regression parameters (slope S and intercept I) of the orthogonal regression for the monthly means GOME-2A tropospheric NO2 product when comparing to MAXDOAS data. Values for GDP 4.9 (this study) are given and the values for GDP 4.8 are reported in brackets for comparison. Results for both the original comparisons and the smoothed comparisons (smo.) are reported.
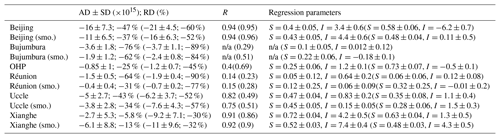
n/a denotes values that are not applicable.
For most stations, in addition of the tropospheric columns, MAXDOAS retrieved NO2 profiles can also be exploited with satellite column averaging kernels (AKs) to further investigate the impact of the satellite a priori NO2 profiles in the comparison differences (Eskes and Boersma, 2003). The satellite AK describes the vertical sensitivity of measurements to NO2 concentrations and relates the MAXDOAS profiles to satellite column measurements by calculating the “smoothed MAXDOAS columns” as
The smoothed MAXDOAS NO2 columns VMAXDOAS,smoothed are derived for each day by convolving the layer (l)-dependent daily profile (interpolated to the satellite overpass time) xMAXDOAS expressed in partial columns with the satellite column averaging kernel AKsat.
The comparisons of satellite and smoothed MAXDOAS columns for the different stations are reported in the supplement (Figs. S5 and S6 in the Supplement) and Tables 4 and 5. The different impact of MAXDOAS smoothing on the 2 GDP products results from the different AKs as parameters like surface albedo or a priori NO2 profiles used in both satellite retrievals are quite different (see Sect. 6). In general, the use of smoothing reduces the MAXDOAS columns and thus reduces both the daily and monthly differences of satellite and MAXDOAS columns. When the average kernels are used to remove the contribution of a priori NO2 profile shape, as seen in Tables 4 and 5 and in Figs. S5 and S6 in the Supplement, the relative differences in urban conditions such as in Beijing or Uccle decrease from % for GDP 4.8 to % for GDP 4.9 for GOME-2A and from −56 % to −29 % for GOME-2B. The differences go from −32 % to −13 % for GOME-2A and from −27 % to −11 % for GOME-2B for suburban conditions such as in Xianghe and go from −77 % to −31 % for GOME-2A and from −64 % to −7 % for GOME-2B for remote conditions such as in Réunion.
The results obtained here are coherent with other validation exercises at different stations and with other satellite products, where the NO2 levels are underestimated by the satellite sensors, e.g. with differences of 5 % to 25 % over China (Ma et al., 2013; Wu et al., 2013; Wang et al., 2017; Drosoglou et al., 2018), mostly explained by the relatively low sensitivity of space-borne measurements near the surface, the gradient-smoothing effect, and the aerosol shielding effect. These effects are often inherent to the different measurements types or the specific conditions of the validation sites (as seen for the different results for Beijing and Xianghe sites in this paper), but also to the remaining impact of structural uncertainties (Boersma et al., 2016), such as the impact of the choices of the a priori NO2 profiles and/or the albedo database assumed for the satellite AMF calculations (see Sect. 6). Lorente et al. (2017) estimated, e.g. the AMF structural uncertainty to be on average 42 % over polluted regions and 31 % over unpolluted regions, mostly driven by substantial differences in the a priori trace gas profiles, surface albedo and cloud parameters used to represent the state of the atmosphere. However, the differences in Bujumbura are still of −62 %, because of the peculiar condition with the MAXDOAS being in a valley, close to Lake Tanganyika, which always leads to a higher surface pressure for the satellite pixels due to the information coming from the a priori model. This is leading to large representation errors and uncertainties in the comparisons (Boersma et al., 2016) that needs to be investigated in more details.
NO2 columns retrieved from measurements of the GOME-2 aboard the MetOp-A and MetOp-B platforms have been successfully applied in many studies. The abundance of NO2 is retrieved from the narrow band absorption structures of NO2 in the backscattered and reflected radiation in the visible spectral region. The current operational retrieval algorithm (GDP 4.8) for total and tropospheric NO2 from GOME-2 was first introduced by Valks et al. (2011), and an improved algorithm (GDP 4.9) is described in this paper.
To calculate the NO2 slant columns, a larger 425–497 nm wavelength fitting window is used in the DOAS fit to increase the signal-to-noise ratio. Absorption cross sections are updated and a linear intensity offset correction is applied. The long-term and in-orbit variations of GOME-2 slit function are corrected by deriving effective slit functions with a stretched preflight GOME-2 slit function and by including a resolution correction function as a pseudo absorber cross section in the DOAS fit, respectively. Compared to the GDP 4.8 algorithm, the NO2 columns from GDP 4.9 are higher by ∼1–3×1014 molec cm2 (up to 27 %) and the NO2 slant column noise is lower by ∼24 %. In addition, the effect of using a new version (6.1) of the GOME-2 level 1b data has been analysed in our NO2 algorithm. The application of new GOME-2 level 1b data largely reduces the offset between GOME-2A and GOME-2B NO2 columns by removing calibration artefacts in the GOME-2B irradiances (due to Xe-line contaminations in the calibration key data). Compared to the GOME-2 NO2 product from the QA4ECV project, the NO2 columns from GDP 4.9 show good consistency and the NO2 slant column noise is ∼14 %–28 % smaller, indicating a good overall quality of the improved DOAS retrieval.
The stratosphere–troposphere separation algorithm STREAM, which was designed for TROPOMI, was optimised for GOME-2 instrument. Compared to the spatial filtering method used in the GDP 4.8, STREAM provides an improved treatment of polluted and cloudy pixels by defining weighting factors for each measurement depending on polluted situation and cloudy information. For the adaption to GOME-2 measurements, the performance of STREAM is analysed by applying it to synthetic GOME-2 data and by comparing the difference between estimated and original stratospheric fields. Applied to synthetic GOME-2 data calculated by a RTM using C-IFS model data, the estimated stratospheric NO2 columns from STREAM show good consistency with the a priori truth. A slight overestimation by ∼1–2×1014 molec cm2 is found over lower latitudes, and larger differences of up to molec cm2 are found at higher latitudes. To reduce the biases over the subtropical regions in winter, an improved latitudinal correction is used in STREAM. Applied to GOME-2 measurements, the updated STREAM successfully separates the stratospheric and tropospheric contribution over polluted regions, especially in the Northern Hemisphere. Compared to the current method in the GDP 4.8, the use of STREAM slightly decreases the stratospheric NO2 columns by molec cm2 in general and largely reduces the overestimation over polluted areas.
To improve the calculation of NO2 AMF, a new box-AMF LUT was generated using the latest version of the VLIDORT RTM with an increased number of reference points and vertical layers to reduce interpolation errors. The new GOME-2 surface LER climatology (Tilstra et al., 2017b) used in this study is derived with a high resolution of 1∘ long. lat. (0.25∘ long. lat. at coastlines) and an improved LER algorithm based on observations for 2007–2013. Daily a priori NO2 profiles, obtained from the chemistry transport model TM5-MP, capture the short-term variability in the NO2 fields with a resolution of 1∘ long. × 1∘ lat. A large impact on the retrieved tropospheric NO2 columns (more than 10 %) is found over polluted areas.
The uncertainty in our GDP 4.9 NO2 slant columns is 4.4×1014 molec cm2, calculated from the average slant column error using a statistical method described in Sect. 4.5. The uncertainty in the GOME-2 stratospheric columns is ∼4–5×1014 molec cm2 for polluted conditions based on the daily synthetic GOME-2 data and ∼1–2×1014 molec cm2 for monthly averages. The uncertainty in the tropospheric AMFs is estimated to be in the 10 %–45 % range, considering the use of updated box-AMF LUT and improved surface albedo climatology and a priori NO2 profiles, resulting in a total uncertainty in the tropospheric NO2 columns likely in the range of 30 %–70 % for polluted conditions.
An end-to-end validation of the improved GOME-2 GDP 4.9 dataset was performed by comparing the GOME-2 tropospheric NO2 columns with BIRA-IASB ground-based MAXDOAS measurements. The validation was illustrated for different MAXDOAS stations (Beijing, Bujumbura, OHP, Réunion, Uccle, and Xianghe) covering urban, suburban, and background situations. Taking Xianghe station as an example, the GDP 4.9 dataset shows a similar seasonal variation in the tropospheric NO2 columns as the MAXDOAS measurements with a relative difference of −5.8 % (i.e. molec cm2 in absolute) and a correlation coefficient of 0.91 for GOME-2A, indicating good agreement. The Xianghe site, by its suburban nature, is the best site for validation. At the other sites, mean biases range from (−47 %; molec cm2) for Beijing, (−76 %, −74 %; molec cm2, molec cm2) for Bujumbura, (−25 %, −17 %; molec cm2, molec cm2) for OHP, (−64 %, −47 %; molec cm2, molec cm2) for Réunion, and (−43 %, −40 %; molec cm2, molec cm2) for Uccle. Réunion and Bujumbura are difficult sites for validation, due to their valley and mountain nature, while urban sites Beijing and Uccle show similar relative results. A smaller absolute bias is found at the rural OHP station. Compared to the current operational GDP 4.8 product, the GDP 4.9 dataset is a significant improvement. Although GOME-2 measurements are still underestimating the tropospheric NO2 columns with respect to the ground data, the absolute and relative differences with the different MAXDOAS stations are smaller, both for the original comparisons and for the comparisons with the smoothed MAXDOAS columns.
In the future, the AMF calculation will be further improved, since uncertainty in AMF is one dominating source of errors in the tropospheric NO2 retrieval, especially over polluted areas. The surface bidirectional reflectance distribution function (BRDF) effect will be included using a direction-dependent LER climatology from GOME-2 (L. Gijsbert Tilstra, personal communication, 2018) to describe the angular distribution of the surface reflectance. Aerosol properties will be considered explicitly in the RTM calculation using ground-based aerosol observations from, e.g. MAXDOAS instruments, Mie scattering lidars, or sun photometers operated by the AErosol RObotic NETwork (AERONET). A priori NO2 profiles from different global and regional models will help to analyse the effect of spatial resolution, temporal resolution, and emissions on the tropospheric NO2 retrieval for GOME-2. Furthermore, the NO2 algorithm will be adapted to measurements from the TROPOMI instrument with a spatial resolution as high as 7 km ×3.5 km.
The current operational (GDP 4.8) NO2 data from GOME-2 can be ordered via the FTP server and the EUMETSAT Data Centre (https://acsaf.org/, last access: 1 February 2019). The improved (GDP 4.9) dataset is currently available upon request.
The supplement related to this article is available online at: https://doi.org/10.5194/amt-12-1029-2019-supplement.
The authors declare that they have no conflict of interest.
This work is funded by the DLR-DAAD Research Fellowships 2015 (57186656)
programme with reference number 91585186 and is undertaken in the framework
of the EUMETSAT AC-SAF project. We acknowledge the Belgian Science Policy
Office (BELSPO) supporting part of this work through the PRODEX project
B-ACSAF. We thank EUMETSAT for the ground segment interfacing work and for
the provision of GOME-2 level 1 products. We thank the UPAS team for the
development work on the UPAS system at DLR. We are thankful to
Rüdiger Lang (EUMETSAT) for providing the GOME-2 level 1b testing data,
Vincent Huijnen (KNMI) for providing the C-IFS model data, Gijsbert Tilstra
(KNMI) for discussions on surface albedo, and Henk Eskes (KNMI) for creating
the TM5-MP a priori NO2 profiles. We acknowledge the free use of
GOME-2 NO2 column data from the QA4ECV project available at
http://www.qa4ecv.eu. We also acknowledge the free use of the GOME-2
surface LER database created by KNMI and provided through the AC-SAF of
EUMETSAT.
The article processing charges for
this open-access
publication were covered by a Research
Centre of
the Helmholtz Association.
Edited by: Jun Wang
Reviewed by: Kai Yang and one anonymous referee
Azam, F., Richter, A., Weber, M., Noë, S., and Burrows, J.: GOME-2 on MetOp-B Follow-on analysis of GOME2 in orbit degradation, Tech. rep., EUM/CO/09/4600000696/RM, Final Report, IUP University of Bremen, 2015. a, b, c
Barkley, M. P., Smedt, I. D., Van Roozendael, M., Kurosu, T. P., Chance, K., Arneth, A., Hagberg, D., Guenther, A., Paulot, F., Marais, E., and Mao, J.: Top-down isoprene emissions over tropical South America inferred from SCIAMACHY and OMI formaldehyde columns, J. Geophys. Res.-Atmos., 118, 6849–6868, 2013. a
Beirle, S., Hörmann, C., Jöckel, P., Liu, S., Penning de Vries, M., Pozzer, A., Sihler, H., Valks, P., and Wagner, T.: The STRatospheric Estimation Algorithm from Mainz (STREAM): estimating stratospheric NO2 from nadir-viewing satellites by weighted convolution, Atmos. Meas. Tech., 9, 2753–2779, https://doi.org/10.5194/amt-9-2753-2016, 2016. a, b, c
Beirle, S., Lampel, J., Lerot, C., Sihler, H., and Wagner, T.: Parameterizing the instrumental spectral response function and its changes by a super-Gaussian and its derivatives, Atmos. Meas. Tech., 10, 581–598, https://doi.org/10.5194/amt-10-581-2017, 2017. a, b
Boersma, K., Bucsela, E., Brinksma, E., and Gleason, J.: OMI algorithm theoretical basis document: OMI trace gas algorithms, vol. 4, edited by: Chance, K., Rep. ATBD-OMI, p. 19, 2002. a, b
Boersma, K., Eskes, H., and Brinksma, E.: Error analysis for tropospheric NO2 retrieval from space, J. Geophys. Res.-Atmos., 109, https://doi.org/10.1029/2003JD003962, 2004. a, b, c, d, e, f, g
Boersma, K. F., Eskes, H. J., Richter, A., De Smedt, I., Lorente, A., Beirle, S., van Geffen, J. H. G. M., Zara, M., Peters, E., Van Roozendael, M., Wagner, T., Maasakkers, J. D., van der A, R. J., Nightingale, J., De Rudder, A., Irie, H., Pinardi, G., Lambert, J.-C., and Compernolle, S. C.: Improving algorithms and uncertainty estimates for satellite NO2 retrievals: results from the quality assurance for the essential climate variables (QA4ECV) project, Atmos. Meas. Tech., 11, 6651–6678, doi:10.5194/amt-11-6651-2018, 2018. a, b, c, d
Boersma, K. F., Eskes, H. J., Dirksen, R. J., van der A, R. J., Veefkind, J. P., Stammes, P., Huijnen, V., Kleipool, Q. L., Sneep, M., Claas, J., Leitão, J., Richter, A., Zhou, Y., and Brunner, D.: An improved tropospheric NO2 column retrieval algorithm for the Ozone Monitoring Instrument, Atmos. Meas. Tech., 4, 1905–1928, https://doi.org/10.5194/amt-4-1905-2011, 2011. a, b, c, d
Boersma, K. F., Vinken, G. C. M., and Eskes, H. J.: Representativeness errors in comparing chemistry transport and chemistry climate models with satellite UV-Vis tropospheric column retrievals, Geosci. Model Dev., 9, 875–898, https://doi.org/10.5194/gmd-9-875-2016, 2016. a, b
Bovensmann, H., Burrows, J., Buchwitz, M., Frerick, J., Rozanov, V., Chance, K., and Goede, A.: SCIAMACHY: Mission objectives and measurement modes, J. Atmos. Sci., 56, 127–150, 1999. a
Brion, J., Chakir, A., Charbonnier, J., Daumont, D., Parisse, C., and Malicet, J.: Absorption spectra measurements for the ozone molecule in the 350–830 nm region, J. Atmos. Chem., 30, 291–299, 1998. a
Bucsela, E. J., Krotkov, N. A., Celarier, E. A., Lamsal, L. N., Swartz, W. H., Bhartia, P. K., Boersma, K. F., Veefkind, J. P., Gleason, J. F., and Pickering, K. E.: A new stratospheric and tropospheric NO2 retrieval algorithm for nadir-viewing satellite instruments: applications to OMI, Atmos. Meas. Tech., 6, 2607–2626, https://doi.org/10.5194/amt-6-2607-2013, 2013. a
Burrows, J. P., Weber, M., Buchwitz, M., Rozanov, V., Ladstätter-Weißenmayer, A., Richter, A., DeBeek, R., Hoogen, R., Bramstedt, K., Eichmann, K.-U., Eisinger, M., and Perner, D.: The global ozone monitoring experiment (GOME): Mission concept and first scientific results, J. Atmos. Sci., 56, 151–175, 1999. a
Callies, J., Corpaccioli, E., Eisinger, M., Hahne, A., and Lefebvre, A.: GOME-2-Metop's second-generation sensor for operational ozone monitoring, ESA bulletin, 102, 28–36, 2000. a
Castellanos, P., Boersma, K. F., Torres, O., and de Haan, J. F.: OMI tropospheric NO2 air mass factors over South America: effects of biomass burning aerosols, Atmos. Meas. Tech., 8, 3831–3849, https://doi.org/10.5194/amt-8-3831-2015, 2015. a, b
Celarier, E. A., Brinksma, E. J., Gleason, J. F., Veefkind, J. P., Cede, A., Herman, J. R., Ionov, D., Goutail, F., Pommereau, J.-P., Lambert, J.-C., van Roozendael, M., Pinardi, G., Wittrock, F., Schönhardt, A., Richter, A., Ibrahim, O. W., Wagner, T., Bojkov, B., Mount, G., Spinei, E., Chen, C. M., Pongetti, T. J., Sander, S. P., Bucsela, E. J., Wenig, M. O., Swart, D. P. J., Volten, H., Kroon, M., and Levelt, P. F.: Validation of Ozone Monitoring Instrument nitrogen dioxide columns, J. Geophys. Res. Atmos., 113, https://doi.org/10.1029/2007JD008908, 2008. a, b
Chance, K. and Kurucz, R.: An improved high-resolution solar reference spectrum for earth's atmosphere measurements in the ultraviolet, visible, and near infrared, J. Quant. Spectrosc. Ra., 111, 1289–1295, 2010. a, b
Chen, D., Zhou, B., Beirle, S., Chen, L. M., and Wagner, T.: Tropospheric NO2 column densities deduced from zenith-sky DOAS measurements in Shanghai, China, and their application to satellite validation, Atmos. Chem. Phys., 9, 3641–3662, https://doi.org/10.5194/acp-9-3641-2009, 2009. a, b
Chimot, J., Veefkind, J. P., de Haan, J. F., Stammes, P., and Levelt, P. F.: Minimizing aerosol effects on the OMI tropospheric NO2 retrieval – An improved use of the 477 nm O2–O2 band and an estimation of the aerosol correction uncertainty, Atmos. Meas. Tech. Discuss., https://doi.org/10.5194/amt-2018-247, in review, 2018. a
Clémer, K., Van Roozendael, M., Fayt, C., Hendrick, F., Hermans, C., Pinardi, G., Spurr, R., Wang, P., and De Mazière, M.: Multiple wavelength retrieval of tropospheric aerosol optical properties from MAXDOAS measurements in Beijing, Atmos. Meas. Tech., 3, 863–878, https://doi.org/10.5194/amt-3-863-2010, 2010. a
Danckaert, T., Fayt, C., Van Roozendael, M., De Smedt, I., Letocart, V., Merlaud, A., and Pinardi, G.: QDOAS software user manual 2.109, IASB/BIRA, Uccle, Belgium, available at: http://uvvis.aeronomie.be/software/QDOAS (last access: 19 September 2015), 2015. a
De Smedt, I., Van Roozendael, M., Stavrakou, T., Müller, J.-F., Lerot, C., Theys, N., Valks, P., Hao, N., and van der A, R.: Improved retrieval of global tropospheric formaldehyde columns from GOME-2/MetOp-A addressing noise reduction and instrumental degradation issues, Atmos. Meas. Tech., 5, 2933–2949, https://doi.org/10.5194/amt-5-2933-2012, 2012. a
Dikty, S., Richter, A., Weber, M., Noël, S., Bovensmann, H., Wittrock, F., and Burrows, J.: GOME-2 on MetOp-A Support for Analysis of GOME-2 In-Orbit Degradation and Impacts on Level 2 Data Products–Final Report, Tech. rep., ITT 09/10000262, IUP University of Bremen, 2011. a, b, c
Ding, J., Miyazaki, K., van der A, R. J., Mijling, B., Kurokawa, J.-I., Cho, S., Janssens-Maenhout, G., Zhang, Q., Liu, F., and Levelt, P. F.: Intercomparison of NOx emission inventories over East Asia, Atmos. Chem. Phys., 17, 10125–10141, https://doi.org/10.5194/acp-17-10125-2017, 2017. a
Doicu, A., Trautmann, T., and Schreier, F.: Numerical regularization for atmospheric inverse problems, Springer Science & Business Media, 2010. a
Drosoglou, T., Bais, A. F., Zyrichidou, I., Kouremeti, N., Poupkou, A., Liora, N., Giannaros, C., Koukouli, M. E., Balis, D., and Melas, D.: Comparisons of ground-based tropospheric NO2 MAX-DOAS measurements to satellite observations with the aid of an air quality model over the Thessaloniki area, Greece, Atmos. Chem. Phys., 17, 5829–5849, https://doi.org/10.5194/acp-17-5829-2017, 2017. a, b
Drosoglou, T., Koukouli, M. E., Kouremeti, N., Bais, A. F., Zyrichidou, I., Balis, D., van der A, R. J., Xu, J., and Li, A.: MAX-DOAS NO2 observations over Guangzhou, China; ground-based and satellite comparisons, Atmos. Meas. Tech., 11, 2239–2255, https://doi.org/10.5194/amt-11-2239-2018, 2018. a, b, c
Eskes, H. J. and Boersma, K. F.: Averaging kernels for DOAS total-column satellite retrievals, Atmos. Chem. Phys., 3, 1285–1291, https://doi.org/10.5194/acp-3-1285-2003, 2003. a
EUMETSAT: GOME-2 Products Guide, Tech. rep., Ref.: EUM/OPSEPS/MAN/07/0445, Issue: v2D, 2009. a, b
EUMETSAT: GOME-2/Metop-A Level 1B Product Validation Report No. 5: Status at Reprocessing, Tech. rep., EUM/OPS/EPS/REP/09/0619, v1F, 2012. a
EUMETSAT: GOME-2 Newsletter Archive, Tech. rep., EUM/OPS-EPS/TEN/09/0552, 2015. a
European Commission: The EU Environmental Implementation Review: Common challenges and how to combine efforts to deliver better results, Tech. rep., Ref.: SWD(2017)33-60 fina, 2017. a
Gielen, C., Hendrick, F., Pinardi, G., De Smedt, I., Fayt, C., Hermans, C., Stavrakou, T., Bauwens, M., Müller, J.-F., Ndenzako, E., Nzohabonayo, P., Akimana, R., Niyonzima, S., Van Roozendael, M., and De Mazière, M.: Characterisation of Central-African aerosol and trace-gas emissions based on MAX-DOAS measurements and model simulations over Bujumbura, Burundi, Atmos. Chem. Phys. Discuss., https://doi.org/10.5194/acp-2016-1104, in review, 2017. a
Granier, C., Bessagnet, B., Bond, T., D'Angiola, A., van Der Gon, H. D., Frost, G. J., Heil, A., Kaiser, J. W., Kinne, S., Klimont, Z., Kloster, S., Lamarque, J.-F., Liousse, C., Masui, T., Meleux, F., Mieville, A., Ohara, T., Raut, J.-C., Riahi, K., Schultz, M. G., Smith, S. J., Thompson, A., van Aardenne, J., van der Werf, G. R., and van Vuuren, D. P.: Evolution of anthropogenic and biomass burning emissions of air pollutants at global and regional scales during the 1980–2010 period, Clim. Change, 109, p. 163, 2011. a
Gu, D., Wang, Y., Smeltzer, C., and Boersma, K. F.: Anthropogenic emissions of NOx over China: Reconciling the difference of inverse modeling results using GOME-2 and OMI measurements, J. Geophys. Res.-Atmos., 119, 7732–7740, 2014. a
Hassinen, S., Balis, D., Bauer, H., Begoin, M., Delcloo, A., Eleftheratos, K., Gimeno Garcia, S., Granville, J., Grossi, M., Hao, N., Hedelt, P., Hendrick, F., Hess, M., Heue, K.-P., Hovila, J., Jønch-Sørensen, H., Kalakoski, N., Kauppi, A., Kiemle, S., Kins, L., Koukouli, M. E., Kujanpää, J., Lambert, J.-C., Lang, R., Lerot, C., Loyola, D., Pedergnana, M., Pinardi, G., Romahn, F., van Roozendael, M., Lutz, R., De Smedt, I., Stammes, P., Steinbrecht, W., Tamminen, J., Theys, N., Tilstra, L. G., Tuinder, O. N. E., Valks, P., Zerefos, C., Zimmer, W., and Zyrichidou, I.: Overview of the O3M SAF GOME-2 operational atmospheric composition and UV radiation data products and data availability, Atmos. Meas. Tech., 9, 383–407, https://doi.org/10.5194/amt-9-383-2016, 2016. a
Heckel, A., Richter, A., Tarsu, T., Wittrock, F., Hak, C., Pundt, I., Junkermann, W., and Burrows, J. P.: MAX-DOAS measurements of formaldehyde in the Po-Valley, Atmos. Chem. Phys., 5, 909–918, https://doi.org/10.5194/acp-5-909-2005, 2005. a
Heckel, A., Kim, S.-W., Frost, G. J., Richter, A., Trainer, M., and Burrows, J. P.: Influence of low spatial resolution a priori data on tropospheric NO2 satellite retrievals, Atmos. Meas. Tech., 4, 1805–1820, https://doi.org/10.5194/amt-4-1805-2011, 2011. a, b
Hendrick, F., Müller, J.-F., Clémer, K., Wang, P., De Maziére, M., Fayt, C., Gielen, C., Hermans, C., Ma, J. Z., Pinardi, G., Stavrakou, T., Vlemmix, T., and Van Roozendael, M.: Four years of ground-based MAX-DOAS observations of HONO and NO2 in the Beijing area, Atmos. Chem. Phys., 14, 765–781, https://doi.org/10.5194/acp-14-765-2014, 2014. a
Hilboll, A., Richter, A., and Burrows, J. P.: Long-term changes of tropospheric NO2 over megacities derived from multiple satellite instruments, Atmos. Chem. Phys., 13, 4145–4169, https://doi.org/10.5194/acp-13-4145-2013, 2013. a
Hilboll, A., Richter, A., and Burrows, J. P.: NO2 pollution over India observed from space – the impact of rapid economic growth, and a recent decline, Atmos. Chem. Phys. Discuss., https://doi.org/10.5194/acp-2017-101, in review, 2017. a, b
Holben, B., Eck, T., and Fraser, R.: Temporal and spatial variability of aerosol optical depth in the Sahel region in relation to vegetation remote sensing, Int. J. Remote Sens., 12, 1147–1163, 1991. a
Hönninger, G., von Friedeburg, C., and Platt, U.: Multi axis differential optical absorption spectroscopy (MAX-DOAS), Atmos. Chem. Phys., 4, 231–254, https://doi.org/10.5194/acp-4-231-2004, 2004. a
Horowitz, L. W., Walters, S., Mauzerall, D. L., Emmons, L. K., Rasch, P. J., Granier, C., Tie, X., Lamarque, J.-F., Schultz, M. G., Tyndall, G. S., Orlando, J. J., and Brasseur, G. P.: A global simulation of tropospheric ozone and related tracers: Description and evaluation of MOZART, version 2, J. Geophys. Res. Atmos., 108, https://doi.org/10.1029/2002JD002853, 2003. a
Huijnen, V., Williams, J., van Weele, M., van Noije, T., Krol, M., Dentener, F., Segers, A., Houweling, S., Peters, W., de Laat, J., Boersma, F., Bergamaschi, P., van Velthoven, P., Le Sager, P., Eskes, H., Alkemade, F., Scheele, R., Nédélec, P., and Pätz, H.-W.: The global chemistry transport model TM5: description and evaluation of the tropospheric chemistry version 3.0, Geosci. Model Dev., 3, 445–473, https://doi.org/10.5194/gmd-3-445-2010, 2010. a
Huijnen, V., Flemming, J., Chabrillat, S., Errera, Q., Christophe, Y., Blechschmidt, A.-M., Richter, A., and Eskes, H.: C-IFS-CB05-BASCOE: stratospheric chemistry in the Integrated Forecasting System of ECMWF, Geosci. Model Dev., 9, 3071–3091, https://doi.org/10.5194/gmd-9-3071-2016, 2016. a
Ingmann, P., Veihelmann, B., Langen, J., Lamarre, D., Stark, H., and Courrèges-Lacoste, G. B.: Requirements for the GMES Atmosphere Service and ESA's implementation concept: Sentinels-4/-5 and-5p, Remote Sens. Environ., 120, 58–69, 2012. a
Irie, H., Takashima, H., Kanaya, Y., Boersma, K. F., Gast, L., Wittrock, F., Brunner, D., Zhou, Y., and Van Roozendael, M.: Eight-component retrievals from ground-based MAX-DOAS observations, Atmos. Meas. Tech., 4, 1027–1044, https://doi.org/10.5194/amt-4-1027-2011, 2011. a
Irie, H., Boersma, K. F., Kanaya, Y., Takashima, H., Pan, X., and Wang, Z. F.: Quantitative bias estimates for tropospheric NO2 columns retrieved from SCIAMACHY, OMI, and GOME-2 using a common standard for East Asia, Atmos. Meas. Tech., 5, 2403–2411, https://doi.org/10.5194/amt-5-2403-2012, 2012. a, b
Kanaya, Y., Irie, H., Takashima, H., Iwabuchi, H., Akimoto, H., Sudo, K., Gu, M., Chong, J., Kim, Y. J., Lee, H., Li, A., Si, F., Xu, J., Xie, P.-H., Liu, W.-Q., Dzhola, A., Postylyakov, O., Ivanov, V., Grechko, E., Terpugova, S., and Panchenko, M.: Long-term MAX-DOAS network observations of NO2 in Russia and Asia (MADRAS) during the period 2007–2012: instrumentation, elucidation of climatology, and comparisons with OMI satellite observations and global model simulations, Atmos. Chem. Phys., 14, 7909–7927, https://doi.org/10.5194/acp-14-7909-2014, 2014. a, b
Kleipool, Q., Dobber, M., de Haan, J., and Levelt, P.: Earth surface reflectance climatology from 3 years of OMI data, J. Geophys. Res.-Atmos., 113, https://doi.org/10.1029/2008JD010290, 2008. a
Kramer, L. J., Leigh, R. J., Remedios, J. J., and Monks, P. S.: Comparison of OMI and ground-based in situ and MAX-DOAS measurements of tropospheric nitrogen dioxide in an urban area, J. Geophys. Res.-Atmos., 113, https://doi.org/10.1029/2007JD009168, 2008. a, b
Krotkov, N. A., Lamsal, L. N., Celarier, E. A., Swartz, W. H., Marchenko, S. V., Bucsela, E. J., Chan, K. L., Wenig, M., and Zara, M.: The version 3 OMI NO2 standard product, Atmos. Meas. Tech., 10, 3133–3149, https://doi.org/10.5194/amt-10-3133-2017, 2017. a
Kuhlmann, G., Lam, Y. F., Cheung, H. M., Hartl, A., Fung, J. C. H., Chan, P. W., and Wenig, M. O.: Development of a custom OMI NO2 data product for evaluating biases in a regional chemistry transport model, Atmos. Chem. Phys., 15, 5627–5644, https://doi.org/10.5194/acp-15-5627-2015, 2015. a
Lambert, J., Granville, J., Van Roozendael, M., Sarkissian, A., Goutail, F., Müller, J., Pommereau, J., and Russell III, J.: A climatology of NO2 profile for improved air mass factors for ground-based vertical column measurements, in: Proceedings of the 5th European Symposium Strat. Ozone, Saint Jean de Luz, p. 703, 1999. a
Lampel, J., Pöhler, D., Tschritter, J., Frieß, U., and Platt, U.: On the relative absorption strengths of water vapour in the blue wavelength range, Atmos. Meas. Tech., 8, 4329–4346, https://doi.org/10.5194/amt-8-4329-2015, 2015. a
Laughner, J. L., Zare, A., and Cohen, R. C.: Effects of daily meteorology on the interpretation of space-based remote sensing of NO2, Atmos. Chem. Phys., 16, 15247–15264, https://doi.org/10.5194/acp-16-15247-2016, 2016. a, b
Laughner, J. L., Zhu, Q., and Cohen, R. C.: The Berkeley High Resolution Tropospheric NO2 product, Earth Syst. Sci. Data, 10, 2069–2095, https://doi.org/10.5194/essd-10-2069-2018, 2018. a
Leitão, J., Richter, A., Vrekoussis, M., Kokhanovsky, A., Zhang, Q. J., Beekmann, M., and Burrows, J. P.: On the improvement of NO2 satellite retrievals – aerosol impact on the airmass factors, Atmos. Meas. Tech., 3, 475–493, https://doi.org/10.5194/amt-3-475-2010, 2010. a
Levelt, P., Van den Oord, G., Dobber, M., Malkki, A., Visser, H., de Vries, J., Stammes, P., Lundell, J., and Saari, H.: The Ozone Monitoring Instrument, IEEE T. Geosci. Remote Sens., 44, 1093–1101, 2006. a
Lin, J.-T., Martin, R. V., Boersma, K. F., Sneep, M., Stammes, P., Spurr, R., Wang, P., Van Roozendael, M., Clémer, K., and Irie, H.: Retrieving tropospheric nitrogen dioxide from the Ozone Monitoring Instrument: effects of aerosols, surface reflectance anisotropy, and vertical profile of nitrogen dioxide, Atmos. Chem. Phys., 14, 1441–1461, https://doi.org/10.5194/acp-14-1441-2014, 2014. a, b, c
Lin, J.-T., Liu, M.-Y., Xin, J.-Y., Boersma, K. F., Spurr, R., Martin, R., and Zhang, Q.: Influence of aerosols and surface reflectance on satellite NO2 retrieval: seasonal and spatial characteristics and implications for NOx emission constraints, Atmos. Chem. Phys., 15, 11217–11241, https://doi.org/10.5194/acp-15-11217-2015, 2015. a, b
Liu, F., Beirle, S., Zhang, Q., van der A, R. J., Zheng, B., Tong, D., and He, K.: NOx emission trends over Chinese cities estimated from OMI observations during 2005 to 2015, Atmos. Chem. Phys., 17, 9261–9275, https://doi.org/10.5194/acp-17-9261-2017, 2017. a
Lorente, A., Folkert Boersma, K., Yu, H., Dörner, S., Hilboll, A., Richter, A., Liu, M., Lamsal, L. N., Barkley, M., De Smedt, I., Van Roozendael, M., Wang, Y., Wagner, T., Beirle, S., Lin, J.-T., Krotkov, N., Stammes, P., Wang, P., Eskes, H. J., and Krol, M.: Structural uncertainty in air mass factor calculation for NO2 and HCHO satellite retrievals, Atmos. Meas. Tech., 10, 759–782, https://doi.org/10.5194/amt-10-759-2017, 2017. a, b, c, d, e, f
Loyola, D., Thomas, W., Livschitz, Y., Ruppert, T., Albert, P., and Hollmann, R.: Cloud properties derived from GOME/ERS-2 backscatter data for trace gas retrieval, IEEE T. Geosci. Remote Sens., 45, 2747–2758, 2007. a
Loyola, D., Koukouli, M., Valks, P., Balis, D., Hao, N., Van Roozendael, M., Spurr, R., Zimmer, W., Kiemle, S., Lerot, C., et al.: The GOME-2 total column ozone product: Retrieval algorithm and ground-based validation, J. Geophys. Res.-Atmos., 116, https://doi.org/10.1029/2010JD014675, 2011. a
Loyola, D. G., Pedergnana, M., and García, S. G.: Smart sampling and incremental function learning for very large high dimensional data, Neural Networks, 78, 75–87, 2016. a
Loyola, D. G., Gimeno García, S., Lutz, R., Argyrouli, A., Romahn, F., Spurr, R. J. D., Pedergnana, M., Doicu, A., Molina García, V., and Schüssler, O.: The operational cloud retrieval algorithms from TROPOMI on board Sentinel-5 Precursor, Atmos. Meas. Tech., 11, 409–427, https://doi.org/10.5194/amt-11-409-2018, 2018. a
Lutz, R., Loyola, D., Gimeno García, S., and Romahn, F.: OCRA radiometric cloud fractions for GOME-2 on MetOp-A/B, Atmos. Meas. Tech., 9, 2357–2379, https://doi.org/10.5194/amt-9-2357-2016, 2016. a, b
Ma, J. Z., Beirle, S., Jin, J. L., Shaiganfar, R., Yan, P., and Wagner, T.: Tropospheric NO2 vertical column densities over Beijing: results of the first three years of ground-based MAX-DOAS measurements (2008–2011) and satellite validation, Atmos. Chem. Phys., 13, 1547–1567, https://doi.org/10.5194/acp-13-1547-2013, 2013. a, b, c
McLinden, C. A., Fioletov, V., Boersma, K. F., Kharol, S. K., Krotkov, N., Lamsal, L., Makar, P. A., Martin, R. V., Veefkind, J. P., and Yang, K.: Improved satellite retrievals of NO2 and SO2 over the Canadian oil sands and comparisons with surface measurements, Atmos. Chem. Phys., 14, 3637–3656, https://doi.org/10.5194/acp-14-3637-2014, 2014. a
Miyazaki, K., Eskes, H., Sudo, K., Boersma, K. F., Bowman, K., and Kanaya, Y.: Decadal changes in global surface NOx emissions from multi-constituent satellite data assimilation, Atmos. Chem. Phys., 17, 807–837, https://doi.org/10.5194/acp-17-807-2017, 2017. a
Müller, J.-P., Kharbouche, S., Gobron, N., Scanlon, T., Govaerts, Y., Danne, O., Schultz, J., Lattanzio, A., Peters, E., De Smedt, I., Beirle, S., Lorente, A., Coheur, P., George, M., Wagner, T., Hilboll, A., Richter, A., Van Roozendael, M., and Boersma, K.: Recommendations (scientific) on best practices for retrievals for Land and Atmosphere ECVs, Tech. rep., Deliverable 4.2 – version 1.0, 2016. a, b
Munro, R., Lang, R., Klaes, D., Poli, G., Retscher, C., Lindstrot, R., Huckle, R., Lacan, A., Grzegorski, M., Holdak, A., Kokhanovsky, A., Livschitz, J., and Eisinger, M.: The GOME-2 instrument on the Metop series of satellites: instrument design, calibration, and level 1 data processing – an overview, Atmos. Meas. Tech., 9, 1279–1301, https://doi.org/10.5194/amt-9-1279-2016, 2016. a, b, c, d, e, f, g
Nolin, A., Armstrong, R., and Maslanik, J.: Near real-time SSM/I EASE-grid daily global ice concentration and snow extent, Digital Media, National Snow and Ice Data Center, Boulder, CO, USA, 2005. a
Nüß, H., Richter, A., Valks, P., and Burrows, J.: Improvement of the NO2 total column retrieval for GOME-2, O3M SAF Visiting Scientist Activity, Final Report, IUP University of Bremen, 2006. a, b, c
Olivier, J. G., Bouwman, A., Berdowski, J., Veldt, C., Bloos, J., Visschedijk, A., Zandveld, P., and Haverlag, J.: Description of EDGAR Version 2.0: A set of global emission inventories of greenhouse gases and ozone-depleting substances for all anthropogenic and most natural sources on a per country basis and on 1 degree × 1 degree grid, RIVM report no. 771060 002/TNO-MEP report no. R96/119, https://www.rivm.nl/bibliotheek/rapporten/771060002.pdf (last access: 1 February 2019), 1996. a
Ortega, I., Koenig, T., Sinreich, R., Thomson, D., and Volkamer, R.: The CU 2-D-MAX-DOAS instrument – Part 1: Retrieval of 3-D distributions of NO2 and azimuth-dependent OVOC ratios, Atmos. Meas. Tech., 8, 2371–2395, https://doi.org/10.5194/amt-8-2371-2015, 2015. a
Penning de Vries, M. J. M., Beirle, S., Hörmann, C., Kaiser, J. W., Stammes, P., Tilstra, L. G., Tuinder, O. N. E., and Wagner, T.: A global aerosol classification algorithm incorporating multiple satellite data sets of aerosol and trace gas abundances, Atmos. Chem. Phys., 15, 10597–10618, https://doi.org/10.5194/acp-15-10597-2015, 2015. a
Peters, E., Wittrock, F., Richter, A., Alvarado, L. M. A., Rozanov, V. V., and Burrows, J. P.: Liquid water absorption and scattering effects in DOAS retrievals over oceans, Atmos. Meas. Tech., 7, 4203–4221, https://doi.org/10.5194/amt-7-4203-2014, 2014. a, b
Pinardi, G., Van Roozendael, M., Lambert, J.-C., Granville, J., Hendrick, F., Tack, F., Yu, H., Cede, A., Kanaya, Y., Irie, I., Goutail, F., Pommereau, J.-P., Pazmino, A., Wittrock, F., Richter, A., Wagner, T., Gu, M., Remmers, J., Friess, U., Vlemmix, T., Piters, A., Hao, N., Tiefengraber, M., Herman, J., Abuhassan, N., Bais, A., Kouremeti, N., Hovila, J., Holla, R., Chong, J., Postylyakov, O., and Ma, J.: GOME-2 total and tropospheric NO2 validation based on zenith-sky, direct-sun and multi-axis DOAS network observations, in: Proc. of the 2014 EUMETSAT Meteorological Satellite Conference, Geneva, Swizerland, 22–26 September 2014, EUMETSAT, 2014. a
Pinardi, G., Lambert, J.-C., Granville, J., Yu, H., De Smedt, I., van Roozendael, M., and Valks, P.: O3M-SAF validation report, Tech. rep., SAF/O3M/IASB/VR/NO2/TN-IASB-GOME2-O3MSAF-NO2-2015, 1/1, 2015. a, b, c
Platt, U. and Stutz, J.: Differential Optical Absorption Spectroscopy, Springer, 2008. a
Pope, R. M. and Fry, E. S.: Absorption spectrum (380–700 nm) of pure water. II. Integrating cavity measurements, Appl. Opt., 36, 8710–8723, 1997. a, b
Richter, A. and verification team: S5P/TROPOMI Science Verification Report, Tech. rep., S5P-IUP-L2-ScVR-RP issue 2.1, 2015. a
Richter, A., Burrows, J. P., Nüß, H., Granier, C., and Niemeier, U.: Increase in tropospheric nitrogen dioxide over China observed from space, Nature, 437, p. 129, 2005. a
Richter, A., Begoin, M., Hilboll, A., and Burrows, J. P.: An improved NO2 retrieval for the GOME-2 satellite instrument, Atmos. Meas. Tech., 4, 1147–1159, https://doi.org/10.5194/amt-4-1147-2011, 2011. a, b, c
Rothman, L., Gordon, I., Barber, R., Dothe, H., Gamache, R., Goldman, A., Perevalov, V., Tashkun, S., and Tennyson, J.: HITEMP, the high-temperature molecular spectroscopic database, J. Quant. Spectrosc. Ra., 111, 2139–2150, 2010. a
Russell, A. R., Perring, A. E., Valin, L. C., Bucsela, E. J., Browne, E. C., Wooldridge, P. J., and Cohen, R. C.: A high spatial resolution retrieval of NO2 column densities from OMI: method and evaluation, Atmos. Chem. Phys., 11, 8543–8554, https://doi.org/10.5194/acp-11-8543-2011, 2011. a
Safieddine, S., Clerbaux, C., George, M., Hadji-Lazaro, J., Hurtmans, D., Coheur, P.-F., Wespes, C., Loyola, D., Valks, P., and Hao, N.: Tropospheric ozone and nitrogen dioxide measurements in urban and rural regions as seen by IASI and GOME-2, J. Geophys. Res.-Atmos., 118, 10555–10566, https://doi.org/10.1002/jgrd.50669, 2013. a
Seinfeld, J. H., Pandis, S., and Noone, K.: Atmospheric chemistry and physics: from air pollution to climate change, Wiley, 1998. a
Solomon, S.: Stratospheric ozone depletion: A review of concepts and history, Rev. Geophys., 37, 275–316, 1999. a
Spurr, R., Kurosu, T., and Chance, K.: A linearized discrete ordinate radiative transfer model for atmospheric remote-sensing retrieval, J. Quant. Spectrosc. Ra., 68, 689–735, 2001. a
Spurr, R. J.: VLIDORT: A linearized pseudo-spherical vector discrete ordinate radiative transfer code for forward model and retrieval studies in multilayer multiple scattering media, J. Quant. Spectrosc. Ra., 102, 316–342, 2006. a
Thalman, R. and Volkamer, R.: Temperature dependent absorption cross-sections of O2–O2 collision pairs between 340 and 630 nm and at atmospherically relevant pressure, Phys. Chem. Chem. Phys., 15, 15371–15381, 2013. a
Tikhonov, A. and Arsenin, V.: Solutions of ill-posed problems, V. H. Winston & Sons, Washington, 1977. a
Tilstra, L., Tuinder, O., and Stammes, P.: GOME-2 surface LER product – Algorithm Theoretical Basis Document, Tech. rep., KNMI Report O3MSAF/KNMI/ATBD/003, Issue 2.2, 2017a. a
Tilstra, L., Wang, P., and Stammes, P.: Surface reflectivity climatologies from UV to NIR determined from Earth observations by GOME-2 and SCIAMACHY, J. Geophys. Res.-Atmos., 122, 4084–4111, 2017b. a, b, c, d, e
Valks, P., Pinardi, G., Richter, A., Lambert, J.-C., Hao, N., Loyola, D., Van Roozendael, M., and Emmadi, S.: Operational total and tropospheric NO2 column retrieval for GOME-2, Atmos. Meas. Tech., 4, 1491–1514, https://doi.org/10.5194/amt-4-1491-2011, 2011. a, b, c, d
Valks, P., Loyola, D., Hao, N., Hedelt, P., Slijkhuis, S., Grossi, M., Begoin, M., Gimeno Garcia, S., and Lutz, R.: Algorithm Theoretical Basis Document for GOME-2 Total Column Products of Ozone, NO2, BrO, SO2, H2O, HCHO and Cloud Properties (GDP 4.8 for AC SAF OTO and NTO), Tech. rep., SAF/AC/DLR/ATBD/01, Iss./Rev.: 3/A/2, 2017. a, b, c
van Geffen, J. H. G. M., Boersma, K. F., Van Roozendael, M., Hendrick, F., Mahieu, E., De Smedt, I., Sneep, M., and Veefkind, J. P.: Improved spectral fitting of nitrogen dioxide from OMI in the 405–465 nm window, Atmos. Meas. Tech., 8, 1685–1699, https://doi.org/10.5194/amt-8-1685-2015, 2015. a, b
van Geffen, J., Boersma, K., Eskes, H., Maasakkers, J., and Veefkind, J.: TROPOMI ATBD of the total and tropospheric NO2 data products, Tech. rep., S5P-KNMI-L2-0005-RP issue 1.0.0. KNMI, De Bilt, the Netherlands, 2016. a, b
Vandaele, A. C., Hermans, C., Fally, S., Carleer, M., Colin, R., Merienne, M.-F., Jenouvrier, A., and Coquart, B.: High-resolution Fourier transform measurement of the NO2 visible and near-infrared absorption cross sections: Temperature and pressure effects, J. Geophys. Res.-Atmos., 107, https://doi.org/10.1029/2001JD000971, 2002. a, b, c
van der A, R. J., Mijling, B., Ding, J., Koukouli, M. E., Liu, F., Li, Q., Mao, H., and Theys, N.: Cleaning up the air: effectiveness of air quality policy for SO2 and NOx emissions in China, Atmos. Chem. Phys., 17, 1775–1789, https://doi.org/10.5194/acp-17-1775-2017, 2017. a
Vasilkov, A., Qin, W., Krotkov, N., Lamsal, L., Spurr, R., Haffner, D., Joiner, J., Yang, E.-S., and Marchenko, S.: Accounting for the effects of surface BRDF on satellite cloud and trace-gas retrievals: a new approach based on geometry-dependent Lambertian equivalent reflectivity applied to OMI algorithms, Atmos. Meas. Tech., 10, 333–349, https://doi.org/10.5194/amt-10-333-2017, 2017. a
Veefkind, J., Aben, I., McMullan, K., Förster, H., De Vries, J., Otter, G., Claas, J., Eskes, H., De Haan, J., Kleipool, Q., van Weele, M., Hasekamp, O., Hoogeveen, R., Landgraf, J., Snel, R., Tol, P., Ingmann, P., Voors, R., Kruizinga, B., Vink, R., Visser, H., and Levelt, P.: TROPOMI on the ESA Sentinel-5 Precursor: A GMES mission for global observations of the atmospheric composition for climate, air quality and ozone layer applications, Remote Sens. Environ., 120, 70–83, 2012. a
Veefkind, J. P., de Haan, J. F., Sneep, M., and Levelt, P. F.: Improvements to the OMI O2–O2 operational cloud algorithm and comparisons with ground-based radar–lidar observations, Atmos. Meas. Tech., 9, 6035–6049, https://doi.org/10.5194/amt-9-6035-2016, 2016. . a
Vlemmix, T., Hendrick, F., Pinardi, G., De Smedt, I., Fayt, C., Hermans, C., Piters, A., Wang, P., Levelt, P., and Van Roozendael, M.: MAX-DOAS observations of aerosols, formaldehyde and nitrogen dioxide in the Beijing area: comparison of two profile retrieval approaches, Atmos. Meas. Tech., 8, 941–963, https://doi.org/10.5194/amt-8-941-2015, 2015. a
Vountas, M., Richter, A., Wittrock, F., and Burrows, J. P.: Inelastic scattering in ocean water and its impact on trace gas retrievals from satellite data, Atmos. Chem. Phys., 3, 1365–1375, https://doi.org/10.5194/acp-3-1365-2003, 2003. a
Vrekoussis, M., Wittrock, F., Richter, A., and Burrows, J. P.: GOME-2 observations of oxygenated VOCs: what can we learn from the ratio glyoxal to formaldehyde on a global scale?, Atmos. Chem. Phys., 10, 10145–10160, https://doi.org/10.5194/acp-10-10145-2010, 2010. a
Wagner, T., Dix, B. V., Friedeburg, C. V., Frieß, U., Sanghavi, S., Sinreich, R., and Platt, U.: MAX-DOAS O4 measurements: A new technique to derive information on atmospheric aerosols – Principles and information content, J. Geophys. Res.-Atmos., 109, https://doi.org/10.1029/2004JD004904, 2004. a
Wagner, T., Beirle, S., Brauers, T., Deutschmann, T., Frieß, U., Hak, C., Halla, J. D., Heue, K. P., Junkermann, W., Li, X., Platt, U., and Pundt-Gruber, I.: Inversion of tropospheric profiles of aerosol extinction and HCHO and NO2 mixing ratios from MAX-DOAS observations in Milano during the summer of 2003 and comparison with independent data sets, Atmos. Meas. Tech., 4, 2685–2715, https://doi.org/10.5194/amt-4-2685-2011, 2011. a
Wang, Y., Beirle, S., Lampel, J., Koukouli, M., De Smedt, I., Theys, N., Li, A., Wu, D., Xie, P., Liu, C., Van Roozendael, M., Stavrakou, T., Müller, J.-F., and Wagner, T.: Validation of OMI, GOME-2A and GOME-2B tropospheric NO2, SO2 and HCHO products using MAX-DOAS observations from 2011 to 2014 in Wuxi, China: investigation of the effects of priori profiles and aerosols on the satellite products, Atmos. Chem. Phys., 17, 5007–5033, https://doi.org/10.5194/acp-17-5007-2017, 2017. a, b, c
Williams, J. E., Boersma, K. F., Le Sager, P., and Verstraeten, W. W.: The high-resolution version of TM5-MP for optimized satellite retrievals: description and validation, Geosci. Model Dev., 10, 721–750, https://doi.org/10.5194/gmd-10-721-2017, 2017. a
Wittrock, F., Oetjen, H., Richter, A., Fietkau, S., Medeke, T., Rozanov, A., and Burrows, J. P.: MAX-DOAS measurements of atmospheric trace gases in Ny-Ålesund – Radiative transfer studies and their application, Atmos. Chem. Phys., 4, 955–966, https://doi.org/10.5194/acp-4-955-2004, 2004. a
Wu, F. C., Xie, P. H., Li, A., Chan, K. L., Hartl, A., Wang, Y., Si, F. Q., Zeng, Y., Qin, M., Xu, J., Liu, J. G., Liu, W. Q., and Wenig, M.: Observations of SO2 and NO2 by mobile DOAS in the Guangzhou eastern area during the Asian Games 2010, Atmos. Meas. Tech., 6, 2277–2292, https://doi.org/10.5194/amt-6-2277-2013, 2013. a, b, c
Zara, M., Boersma, K. F., De Smedt, I., Richter, A., Peters, E., van Geffen, J. H. G. M., Beirle, S., Wagner, T., Van Roozendael, M., Marchenko, S., Lamsal, L. N., and Eskes, H. J.: Improved slant column density retrieval of nitrogen dioxide and formaldehyde for OMI and GOME-2A from QA4ECV: intercomparison, uncertainty characterisation, and trends, Atmos. Meas. Tech., 11, 4033–4058, https://doi.org/10.5194/amt-11-4033-2018, 2018. a
Zien, A. W., Richter, A., Hilboll, A., Blechschmidt, A.-M., and Burrows, J. P.: Systematic analysis of tropospheric NO2 long-range transport events detected in GOME-2 satellite data, Atmos. Chem. Phys., 14, 7367–7396, https://doi.org/10.5194/acp-14-7367-2014, 2014. a
Note that the derived slant columns are scaled by geometric AMFs to correct for the angular dependencies of GOME-2 measurements in this section.
- Abstract
- Introduction
- Instrument and measurements
- Total and tropospheric NO2 retrieval for GDP 4.8
- Improved DOAS slant column retrieval
- New stratosphere–troposphere separation
- Improvements to NO2 AMF calculation
- End-to-end GOME-2 NO2 validation
- Conclusions
- Data availability
- Competing interests
- Acknowledgements
- References
- Supplement
- Abstract
- Introduction
- Instrument and measurements
- Total and tropospheric NO2 retrieval for GDP 4.8
- Improved DOAS slant column retrieval
- New stratosphere–troposphere separation
- Improvements to NO2 AMF calculation
- End-to-end GOME-2 NO2 validation
- Conclusions
- Data availability
- Competing interests
- Acknowledgements
- References
- Supplement