the Creative Commons Attribution 4.0 License.
the Creative Commons Attribution 4.0 License.
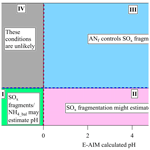
Aerosol pH indicator and organosulfate detectability from aerosol mass spectrometry measurements
Melinda K. Schueneman
Benjamin A. Nault
Pedro Campuzano-Jost
Duseong S. Jo
Douglas A. Day
Jason C. Schroder
Brett B. Palm
Alma Hodzic
Jack E. Dibb
Jose L. Jimenez
Aerosol sulfate is a major component of submicron particulate matter (PM1). Sulfate can be present as inorganic (mainly ammonium sulfate, AS) or organosulfate (OS). Although OS is thought to be a smaller fraction of total sulfate in most cases, recent literature argues that this may not be the case in more polluted environments. Aerodyne aerosol mass spectrometers (AMSs) measure total submicron sulfate, but it has been difficult to apportion AS vs. OS as the detected ion fragments are similar. Recently, two new methods have been proposed to quantify OS separately from AS with AMS data. We use observations collected during several airborne field campaigns covering a wide range of sources and air mass ages (spanning the continental US, marine remote troposphere, and Korea) and targeted laboratory experiments to investigate the performance and validity of the proposed OS methods. Four chemical regimes are defined to categorize the factors impacting sulfate fragmentation. In polluted areas with high ammonium nitrate concentrations and in remote areas with high aerosol acidity, the decomposition and fragmentation of sulfate in the AMS is influenced by multiple complex effects, and estimation of OS does not seem possible with current methods. In regions with lower acidity (pH > 0) and ammonium nitrate (fraction of total mass < 0.3), the proposed OS methods might be more reliable, although application of these methods often produced nonsensical results. However, the fragmentation of ambient neutralized sulfate varies somewhat within studies, adding uncertainty, possibly due to variations in the effect of organics. Under highly acidic conditions (when calculated pH < 0 and ammonium balance < 0.65), sulfate fragment ratios show a clear relationship with acidity. The measured ammonium balance (and to a lesser extent, the HySO SO AMS ratio) is a promising indicator of rapid estimation of aerosol pH < 0, including when gas-phase NH3 and HNO3 are not available. These results allow an improved understanding of important intensive properties of ambient aerosols.
- Article
(4208 KB) -
Supplement
(2053 KB) - BibTeX
- EndNote
PM1, or submicron particulate matter, have important impacts on visibility, climate, and environmental and human health (Dockery et al., 1996; Lighty et al., 2000; Lohmann et al., 2004; IPCC, 2013). In order to quantify the impacts of PM1 and their evolution with changes in emissions, chemistry, and climate, PM1 sources, chemistry, and composition must be understood. Field measurements are critical to that goal, and one tool used extensively in field studies since the early 2000s is the Aerodyne aerosol mass spectrometer (AMS) and more recently its simplified version, the aerosol chemical speciation monitor (ACSM) (Jayne et al., 2000; DeCarlo et al., 2006; Canagaratna et al., 2007; Ng et al., 2011a). The AMS typically quantifies the chemical composition and size distribution of sulfate, nitrate, organic aerosol (OA), ammonium, and chloride (Jayne et al., 2000; DeCarlo et al., 2006; Canagaratna et al., 2007; Jimenez et al., 2009).
Within the AMS, particles are vaporized, leading to some thermal decomposition (e.g., Docherty et al., 2015), and then ionized via 70 eV electron ionization, which leads to substantial fragmentation of the molecular ions. Despite or perhaps because of the substantial (and reproducible) decomposition and fragmentation, the relative signals of different AMS fragments have been found to be indicative of different chemical species in the aerosol. These include the presence of inorganic vs. organic nitrates (Farmer et al., 2010; Fry et al., 2013) and of several source and composition characteristics of organic aerosols (Alfarra et al., 2004; Q. Zhang et al., 2004; Cubison et al., 2011; Ng et al., 2011b; Hu et al., 2015). In contrast to nitrates, deconvolving inorganic sulfates vs. organosulfates (OSs, which include sulfonic acids, when present) are thought to be more difficult. The fragmentation pattern for one atmospherically relevant OS was similar to those of inorganic sulfates (ASs, mainly ammonium sulfate salts) in an early study, with minimal C–S-containing fragments (Farmer et al., 2010). Until recently, most studies have shown that the OS molar fraction (OSf = OS (AS + OS), calculated using only the sulfate moiety of the molecules) typically makes a small (∼ 1 %–10 %) contribution to total sulfate in PM1 (e.g., Tolocka and Turpin, 2012; Hu et al., 2015; Liao et al., 2015; Riva et al., 2016, 2019). However, for biogenic areas OSf is predicted to increase substantially in the future (Riva et al., 2019). Another important recent subject of debate are the missing sources of sulfate production in haze events in China (Wang et al., 2014; Zheng et al., 2015; Li et al., 2017), which some studies have attributed to a major contribution of OS (e.g., Song et al., 2019). It should be noted that a recent study reports that OS filter-based measurements in past scientific studies may have substantial associated positive biases, leading to an overestimate for [OS] (Brüggemann et al., 2021). It is also important to quantify OS in order to understand the chemistry of aerosol formation and aging (Surratt et al., 2007, 2008; Song et al., 2019), which impact the ability to understand how sulfate may influence various PM1 properties and processes (e.g., gas uptake, aqueous reactions). Finally, accurate AS concentrations are needed to quantify the inorganic : organic ratio (to predict the hygroscopicity of PM1, which impacts satellite and model interpretation) and to estimate aerosol pH and liquid water content from thermodynamic models as it is currently still not possible to measure the aerosol pH in the field in situ (Hennigan et al., 2015; Guo et al., 2016; Craig et al., 2018; Pye et al., 2020).
Recent AMS work has attempted to quantify OSf from the measured individual sulfate ion signals (Chen et al., 2019; Song et al., 2019). The vaporization and ionization of AS and OS in the AMS produce similar ion fragments that do not contain a carbon atom, the major ones quantified being SO+, SO, SO, HSO, and H2SO. These ions were attributed primarily to inorganic sulfate in earlier AMS analyses (e.g., Jimenez et al., 2003) but were shown to have a contribution from organosulfates by Farmer et al. (2010). Note that these are the ions detected in the AMS (following ionization and decomposition) and not the ions present in the aerosols (discussed in Sect. 3.3 and shown in Fig. 2c). However, a recent laboratory study with many OS standards found reproducible differences in the fragmentation of AS vs. OS (Chen et al., 2019). That study proposed a method using the unique AS ion fragments (H2SO and HSO) divided by the total sulfate signal (H2SO + HSO + SO + SO + SO+) to apportion OS, AS, and methanesulfonic acid (MSA, an organosulfur compound but not an organosulfate) in field datasets. It is important to note that MSA can be directly measured with the (HR-)AMS (Phinney et al., 2006; Zorn et al., 2008; Huang et al., 2017; Hodshire et al., 2019), so quantification of MSA with the method in Chen et al. (2019) is not necessary. From this method, an average OS mass concentration (COS) of 0.12 µg m−3 was estimated for the Southern Oxidant and Aerosol Study (SOAS) ground campaign in rural Alabama (Carlton et al., 2018), with OSf ∼ 4 % (Chen et al., 2019). That estimate is consistent with others for that site and region (Hu et al., 2015; Liao et al., 2015). An alternative method to estimate OSf based on the same principle was proposed by Song et al. (2019) using the observed AMS SO+ HySO and SO HySO. These authors reported OSf ∼ 17 % ± 7 % (which corresponds to [OS] ∼ 5–10 µg m−3) during winter haze episodes in China. A recent study (Dovrou et al., 2019) investigated mixtures of sodium sulfate and sodium hydroxymethanesulfonate (HMS); however, they found that HMS cannot be distinguished from AMS ions alone due to the complex ambient-aerosol mixture containing organosulfates and inorganic sulfates, which all, in part, produce the same sulfate fragments as HMS.
Another important and related analytical challenge is online quantification or estimation of ambient-aerosol acidity from real-time measurements, e.g., during field campaigns. So far, online aerosol pH measurements have only been performed in the laboratory (Rindelaub et al., 2016; Craig et al., 2018). Aerosol acidity is important because it impacts human health by decreasing lung function (Raizenne et al., 1996) and strongly impacts the equilibria and kinetics of a very large number of atmospheric physical and chemical processes (Jang et al., 2002; Meskhidze et al., 2003; Anon, 2007; Thornton et al., 2008; Bertram and Thornton, 2009; Gaston et al., 2014; Ackendorf et al., 2017; Guo et al., 2017; Losey et al., 2018). In addition, the deposition of acidic particles leads to damage to terrestrial and freshwater ecosystems, i.e., “acid rain” or more properly acid deposition (Schindler, 1988; Johnson et al., 2008). Currently, the state-of-the-art technique to quantify aerosol acidity for field data is to run an inorganic-aerosol thermodynamic model that includes the measured particle and gas inorganic concentrations as well as temperature and humidity. The Extended Aerosol Inorganics Model (E-AIM) (Clegg et al., 1998, 2003; Wexler and Clegg, 2002) is generally considered to be the reference model (Pye et al., 2020). ISORROPIA-II (Nenes et al., 1999; Fountoukis and Nenes, 2007) is a faster model utilizing look-up tables to calculate aerosol liquid water content (and thus is frequently used as part of chemical transport models) at the expense of some accuracy at different relative humidity (RH) levels (Pye et al., 2020). In general, these thermodynamic models are thought to perform best for pH estimation when gas-phase measurements of NH3 and/or HNO3 are used in the calculations and to perform less well when run only with aerosol measurements (Guo et al., 2015; Hennigan et al., 2015; Song et al., 2018).
There has been an ongoing debate about the potential relationship between the inorganic cation anion charge ratio (commonly referred to as “ammonium balance”; see Eq. 7) and aerosol acidity. Ammonia gas and its particle-phase equivalent (ammonium) are the dominant bases in the atmosphere (Dentener and Crutzen, 1994). As the most important base in PM1, a deficit of NH vs. dominant PM1 anions, SO and NO (Jimenez et al., 2009), is indicative of the concentration of H+ since the particles are (nearly) electrically neutral. Thus, in the absence of substantial non-volatile cations (e.g., Na+, K+) ammonium balance is an indicator of aerosol acidity. Ammonium balance has been shown to correlate well with pH under certain conditions, specifically when using daily averaged temperature and relative humidity (Zhang et al., 2007a), but has been criticized as being a poor surrogate of pH under other conditions (Hennigan et al., 2015). In particular, ammonium balance can be a poor surrogate of pH because changes in temperature and RH impact the aerosol liquid water in the diurnal cycle (Zhang et al., 2007a). This is especially important in the boundary layer, where almost all past pH quantification has been carried out (Pye et al., 2020), compared to the lower diurnal variance in T and RH in the free and upper troposphere. Many field studies do not include measurements of NH3 or HNO3, two species that are difficult to measure due to inlet delays caused by strong interactions with surfaces. Both species are typically present at low concentrations and thus are not routinely measured, limiting the ability to calculate aerosol pH (Hennigan et al., 2015). A more direct estimate of aerosol acidity using only ambient-particle data is highly desirable.
Here, we analyze sulfate ion fragment data from laboratory and ambient AMS observations, spanning multiple aircraft campaigns with a routinely calibrated AMS response to AS and across a wide range of chemical and meteorological environments. We use this large dataset to test the applicability of recently published methods to partition AS and OS. We investigate the feasibility of estimating pH based on AMS data as well as the regions of chemical space where the different estimation methods may work. Finally, we provide a physical interpretation for sulfate fragmentation in the AMS.
2.1 Airborne campaigns
Sulfate fragmentation data were obtained using an Aerodyne high-resolution time-of-flight aerosol mass spectrometer (AMS) (Aerodyne Research Inc., Billerica, MA, USA; DeCarlo et al., 2006). The ambient data used here are from aircraft observations from the following campaigns (Table 1): Deep Convective Clouds and Chemistry (DC3) (Barth et al., 2015); Studies of Emissions and Atmospheric Composition, Clouds and Climate Coupling by Regional Surveys (SEAC4RS) (Toon et al., 2016); Wintertime Investigation of Transport, Emissions, and Reactivity (WINTER) (Schroder et al., 2018); Korean–United States Air Quality (KORUS-AQ) (Nault et al., 2018); and Atmospheric Tomography Mission 1 and 2 (ATom-1 and ATom-2) (Guo et al., 2020; Hodzic et al., 2020). Flight paths for all six campaigns are shown in Fig. S1 in the Supplement. These campaigns span polluted urban, partially polluted biogenic, biomass burning smoke, rural, and remote regions of the atmosphere. DC3 sampled continental and rural conditions with diffuse pollution and some biomass burning events. WINTER and KORUS-AQ were airborne campaigns that focused on urbanized regions (although from different regions and times of year; Table 1); therefore, the campaigns had appreciable mass concentrations of ammonium nitrate due to anthropogenic emissions of NOx and the subsequent production of HNO3 that partitions into the aerosol with ammonia (Seinfeld and Pandis, 2006). SEAC4RS focused on regional background chemistry of the continental United States, which included impacts from biomass burning, biogenic, and pollution emissions and upper-tropospheric chemistry impacted by convection. Finally, ATom-1 and ATom-2 sampled the remote Pacific and Atlantic basins with continuous full vertical profiling in order to study the composition of the remote marine atmosphere, impacted by long-range-transported chemical species and marine emissions and far from anthropogenic sources. Not all campaigns are usable for all the analyses in this paper, depending on the quality and completeness of the data. Table 1 indicates which campaigns were usable for each analysis.
Table 1Summary of the campaigns used in this study. See Fig. S1 for flight paths. Reference label refers to the type of data used for each campaign throughout this paper, depending on the quality and completeness of the data, for the purposes of a specific analysis. A: ammonium balance; f: SO4 campaign-averaged fragments; F: SO4 campaign-averaged and time-resolved fragments; and C: pure-AS calibration data reliable and used.
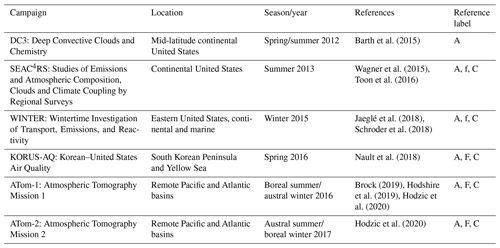
2.2 High-resolution time-of-flight aerosol mass spectrometer
The highly customized University of Colorado-Boulder aircraft AMS was used in all campaigns and has been described elsewhere (DeCarlo et al., 2008; Dunlea et al., 2009; Nault et al., 2018; Schroder et al., 2018; Guo et al., 2020), so only details relevant to this study are summarized here. Ambient air is drawn through a National Center for Atmospheric Research (NCAR) high-performance instrumented airborne platform for environmental research modular inlet (HIMIL; Stith et al., 2009) with a constant standard flow rate of 9 L min−1, and all data are reported at a constant standard temperature (T = 273 K) and pressure (P = 1013 hPa). The sampled air enters a pressure-controlled inlet (Bahreini et al., 2008) and is then introduced into an aerodynamic focusing lens (Liu et al., 1995; X. Zhang et al., 2004). Particles then impact onto an inverted-cone porous-tungsten “standard” vaporizer (SV), operated at ∼ 600 ∘C under high vacuum. The standard vaporizer is used in this study. A “capture vaporizer” has been recently demonstrated; it leads to more thermal decomposition while still retaining similar (although noisier) fragment information (Hu et al., 2017a; Zheng et al., 2020), but it is not used here. Non-refractory species, those that evaporate in less than a few seconds (such as sulfate, nitrate, ammonium, and organic material), are subsequently ionized by 70 eV electrons. Some refractory and semi-refractory species such as sea salt, lead, and potassium can be detected by the AMS in some cases (Lee et al., 2010; Salcedo et al., 2010; Ovadnevaite et al., 2012; Hodzic et al., 2020). A cryopump reduces background in the ionizer by orders of magnitude during the flights, leading to low detection limits, in particular for NH4, which is critical for acidity quantification in the remote troposphere. Data were taken at 1 Hz but were processed at both 1 Hz and 1 min resolution, and the latter product is primarily used here due to higher signal-to-noise ratios. The 1 min datasets were further filtered by removing points where the sulfate signal was below 3 times its detection limit. Detection limits were estimated continuously via the methods of Drewnick et al. (2009) and confirmed with frequent in-flight filter blanks. For the laboratory studies, everything was kept the same as on the aircraft other than no use of the HIMIL aircraft inlet. Data were processed and analyzed with the standard Squirrel and PIKA ToF-AMS data analysis software packages within Igor Pro 7 (Wavemetrics) (DeCarlo et al., 2006; Sueper, 2018).
One important parameter for AMS quantification is collection efficiency (CE). CE is the probability that a particle entering the AMS is detected. It is affected by several particle properties (Huffman et al., 2005), the most important being particle bounce off the vaporizer without detection (Middlebrook et al., 2012). Bounce is controlled by particle phase (Quinn et al., 2006; Matthew et al., 2008) and is estimated for ambient particles based on their ammonium balance (acidity) and ammonium nitrate content (Middlebrook et al., 2012). This parameterization performs well for ambient particles (Middlebrook et al., 2012; Hu et al., 2017a, 2020; Guo et al., 2020). Still, potential variability in CE that is not perfectly captured by the parameterization contributes a major fraction of the AMS uncertainty for ambient-particle analysis (Bahreini et al., 2009). Alternative methods to estimate ambient CE for ambient particles are of interest; we explore a potential alternative method here.
2.3 Quantification of OS and AS using literature methods
Two methods have been proposed to quantify OS contribution to total sulfate using AMS sulfate ion fragment fractions. The first method uses different sulfate ions to attribute measured total sulfate to either OS, AS, or methanesulfonic acid (MSA). Due to the structure of OS, only non-hydrogenated sulfate ions, i.e., SO+, SO, and SO, are produced in the AMS for OS. AS does produce hydrogenated sulfate ions, i.e., H2SO and HSO, as well as the same non-hydrogenated sulfate ions produced by OS. Chen et al. (2019) proposed a “triangle method” to estimate these two species and MSA, based on the observed fragments. Note that mineral sulfates such as sodium sulfate fragment similarly to OS, and thus these methods need to be interpreted differently in regions with significant submicron mineral sulfates. MSA calibrations show variability for the fragments (Chen et al., 2019) and were not performed for all the studies in this work. Since MSA can be quantified without using the sulfate fragments, here we apply this method to estimate the fractions of OS and AS by using a one-dimensional version of the triangle (i.e., just the hypotenuse connecting pure OS to pure AS). An alternative method is based on the same assumptions but uses different equations to quantify the relative concentration of OS (Song et al., 2019).
Both literature methods for deconvolving sulfate as OS and AS assume that the main factor impacting sulfate fragmentation in the AMS is sulfate structure (OS, AS, or MSA). Chen et al. (2019) briefly mention that acidity can impact sulfate fragmentation, but this effect has not been studied and quantified. In addition, Chen et al. (2019) used pure standards to quantify the AMS fragmentation of different species but did not explore potential matrix effects in AMS fragments, which could impact internally mixed ambient particles.
2.4 Quantification of the AMS sulfate fragment ratios
To compare our field data to those analyzed in Chen et al. (2019), we use the variables defined in that study, fH2SO and fHSO, and define the normalized nfH2SO and nfHSO (normalized to the values of fH2SO and fHSO for pure AS):
It should be noted that while that study includes methanesulfonic acid (MSA) data, the impact of MSA on fH2SO and fHSO is minimal for the ATom campaigns (see Fig. S2). Additionally, one study over the western United States (representing a rural, continental region) observed MSA concentrations of ∼ 50 ng m−3 (Sorooshian et al., 2015), which results in a very small deviation in the Chen triangle and can hence be neglected for the purposes of this work. All variables were normalized to the values of the same variables for pure-AS calibrations (conducted during each field experiment) in order to eliminate some of the spread in the sulfate ions that is likely due to instrument-to-instrument or instrument-in-time variability (Fry et al., 2013; Chen et al., 2019) (Fig. S3). We also define a new AMS sulfate ion ratio, HySO SO, and create the normalized nHySO SO to reduce the influence of instrument-to-instrument or instrument-in-time variability:
The submicron aerosol molar ammonium balance (NH4_bal) is calculated as
The concentration of non-refractory chloride is only included for non-remote campaigns (KORUS-AQ, WINTER, and SEAC4RS) since it was negligible for others and strongly impacted by sea salt in the marine boundary layer. The fraction of ammonium nitrate in the particle phase (ammonium nitrate mass fraction, ANf) (by mass) is
The fraction of total AMS aerosol mass comprised of OA (OAf) is
The sulfate equivalent concentration of OS in the Song et al. (2019) paper is calculated as
where “cd” stands for “clean and dry”. Clean and dry conditions are defined in Song et al. (2019) as ambient data points where PM1 = 10 µg m−3 and RH = 30 %. Clean and dry conditions are assumed to represent nearly pure AS. M is for the molar mass of the different sulfate ions, and “obs” represents the ambient data for specific sulfate fragments. HySO (which differs from the notation used in Song et al., 2019, but is necessary to differentiate HySO between Chen et al., 2019, and Song et al., 2019) is defined in Song et al. (2019) as SOHSO + H2SO. For the Chen method, the COS is defined based on the AS-normalized nfH2SO values:
OSf, the fraction of OS : total sulfate, is defined as
where COS is calculated from Eq. (10) or (11).
2.5 Laboratory experiments
As ambient aerosols contain mixtures of chemical species, we investigated if matrix effects may impact the fragmentation of sulfate species. Different solution mixtures, composed of various amounts of AS (certified American Chemical Society (ACS), 99.7 % purity) and ammonium nitrate (AN) (certified ACS, 99.9 % purity) in water (Milli-Q-grade, R > 19 MΩ), were atomized to generate particles and size-selected using a differential mobility analyzer (DMA) (TSI Model 3081), analyzed with a condensation particle counter (CPC) (Model 3775), and electrostatic classifier (Model 3080) for mobility diameters between 350–400 nm. We investigated AS–AN mixtures, ranging from ANf = 0 % to 95 %.
In order to assess effects on the sulfate fragmentation from mixing with OA, chamber experiments, where different types of secondary organic aerosol (SOA) were formed by gas-phase reactions and condensation onto AS seeds, were investigated. SOA was formed from alkanol and toluene photooxidation under high-NOx conditions (Liu et al., 2019) as well as Δ-3-carene and α-pinene reactions with nitrate radicals (Kang et al., 2016). Experiments were initiated with 100 % AS in a dry chamber (RH < 5 %, ∼ 298 K) followed by either rapid, gradual, or stepwise increases in SOA until a maximum OA (OA + AS) ratio of ∼ 70 % was reached. Aerosol composition was monitored by the AMS, and size distributions were monitored with a scanning mobility particle sizer (SMPS; DMA was TSI Model 3081, electrostatic classifier was Model 3080, and the CPC was Model 3775). The relative ionization efficiency (RIE) of sulfate was directly calibrated with pure ammonium sulfate, while RIE * CE of the SOA produced was estimated by comparison to the SMPS-integrated volume, together with OA density estimated from the AMS-derived elemental ratios per Kuwata et al. (2012), in order to accurately quantify OA (OA + AS). Humid experiments were not considered here due to the potential of forming organosulfates.
2.6 E-AIM thermodynamic model for pH estimation
Aerosol pH was estimated using the Extended Aerosol Inorganic Model (E-AIM) IV (Clegg et al., 1998; Massucci et al., 1999; Wexler and Clegg, 2002). We input into the model (run in “forward mode”) the total nitrate (gas-phase HNO3 plus particle-phase total NO), sulfate, ammonium, relative humidity (calculated according to the parameterization of Murphy and Koop (2005), which is critical for upper-tropospheric conditions), and temperature. Total nitrate (inorganic + organic) was input as Nault et al. (2021) found that removing estimated organic nitrate does not impact the pH calculation. This was done to calculate aerosol liquid water and aerosol pH. Model IV was not run with chloride ions as their concentrations were very low, and including chloride limits the model to temperatures ≥ 263 K (Friese and Ebel, 2010), which would greatly limit the analysis of calculated pH for WINTER, ATom-1, and ATom-2. We have added the modifier “calculated” before pH for all situations where we are describing the E-AIM pH and “estimated” when we refer to pH from the empirical estimation methods from AMS measurements, introduced in this study. Also, including chloride precludes running the model under supersaturated-solution conditions, which is a closer approximation of ambient aerosol (Pye et al., 2020). All aerosol mass concentrations were from the University of Colorado at Boulder (CU) AMS. HNO3(g) was measured by the California Institute of Technology chemical ionization mass spectrometer (CIT-CIMS) (Crounse et al., 2006), which was flown in all of these missions (excluding WINTER, where the chemical ionization mass spectrometer of the University of Washington (UW-CIMS) was used for the HNO3 measurements) (Lee et al., 2014, 2018). Results are generally similar when using the soluble acidic gases and aerosols (SAGA) mist chamber measurement for total nitrate (Nault et al., 2020). The forward mode is less sensitive to uncertainties in measurements than the “reverse mode,” which only uses particle composition and T and RH as inputs (Hennigan et al., 2015). Also, due to lack of NH3(g) measurements, the model was run iteratively until convergence in modeled NH3 occurred, similarly to Guo et al. (2016). Performance for calculated pH was investigated by comparing model-calculated HNO3 and NO to measurements as the partitioning of nitrate between gas and particle phase is sensitive to calculated pH under acidic conditions (Guo et al., 2016). For all campaigns included herein (DC3, WINTER, SEAC4RS, KORUS-AQ, ATom-1, and ATom-2), the slopes of HNO3 (measured vs. predicted) are within the uncertainty in the measurements and with good correlations (Fig. S4). For NO, the slopes are within the measurement uncertainty for five of the six campaigns. For ATom-2, the NO slopes were low; however, for this campaign, the measured NO mass concentrations were extremely low (mean = 0.02 µg sm−3), and the calculated pH was also very low (mean = −0.5), leading to very little NO in the aerosol phase (see Fig. S4).
In addition, other bases present in the atmosphere (such as amines) were examined. Prior studies have shown that amines were less than a maximum concentration of 30 ng m−3 at the ocean surface (Gibb et al., 1999; Facchini et al., 2008; Müller et al., 2009; Frossard et al., 2014; van Pinxteren et al., 2015; Youn et al., 2015). Another study by Sorooshian et al. (2009) found that amine mass concentration dropped off quickly with altitude to concentrations less than 25 ng m−3 at an altitude between 200 and 300 m, which is the approximate minimum altitude flown on the DC-8 during the ATom campaigns. As the 1 min detection limit for the AMS data for amines is typically 10 ng m−3, we expect the amine signal to generally be below the limit of detection and thus outside of our quantification capabilities. This was observed for AMS data from the ATom campaigns, using characteristic ions identified in past studies (Murphy et al., 2007; Ge et al., 2014). It was found that amine ions cannot be distinguished from background for many ATom flights. Only during one flight in ATom-1 did we observe an amine signal (C2H6N+ = 44) above the background (see Fig. S5). During this flight, amines (from the contribution of CH4N, C2H6N, and C3H8N) only accounted for 0.7 ng m−3of aerosol, whereas ammonium accounted for 19 ng m−3. Amines can produce the same fragments as ammonium, but this is only the case for a few percent of the amine fragments (Ge et al., 2014). In this case, the ammonium concentration is 25 times that of the amines. Since amines were even lower during other flights, we assume the effect of amines to the pH calculation is very small and can be ignored for E-AIM calculations.
2.7 GEOS-Chem model
We used a global chemical transport model (GEOS-Chem 12.6.1; https://doi.org/10.5281/zenodo.3520966; Bey et al., 2001) to investigate modeled global distributions of ammonium nitrate mass fraction (ANf) and calculated aerosol pH across different regions. GEOS-Chem was driven by assimilated meteorological fields from the Modern-Era Retrospective analysis for Research and Applications version 2 (MERRA2) (Gelaro et al., 2017) for the year of 2010. The simulation was conducted at 2∘ (latitude) × 2.5∘ (longitude) with 47 vertical layers up to 0.01 hPa and ∼ 30 layers under 200 hPa. We used the Community Emissions Data System (CEDS) inventory for global anthropogenic emissions (Hoesly et al., 2018) and the Global Fire Emissions Database version 4 (GFED4) for biomass burning emissions (Giglio et al., 2013). Aerosol pH and gas-particle partitioning of inorganic aerosols were calculated online using the ISORROPIA-II model within GEOS-Chem (Fountoukis and Nenes, 2007; Pye et al., 2020). Similarly to Jo et al. (2019), sea salt aerosol was excluded from pH calculations based on a better agreement with the observationally constrained calculated-pH values as suggested by Nault et al. (2020). Oceanic NHx emissions were also included in this model based on recent work (Paulot et al., 2015; Nault et al., 2020).
3.1 Lab quantification of AMS data
Application of the one-dimensional Chen method to laboratory data is shown in Fig. 1. Data are expected to lie inside the triangular region and be apportioned depending on the relative distance to the three vertices. For example, data lying at [0.5,0.5] on the line between the OS and AS points would represent a sample with ∼ 50 % OS and ∼ 50 % AS. If data cluster around the [1,1] point, where pure AS resides, all of the sulfate is attributed to AS. From applying this method, it is clear that none of the campaign averages or laboratory data fall between the [0,0] and [1,1] points, suggesting that there may be additional factors (other than sulfate composition) impacting the location of data in this triangular region.
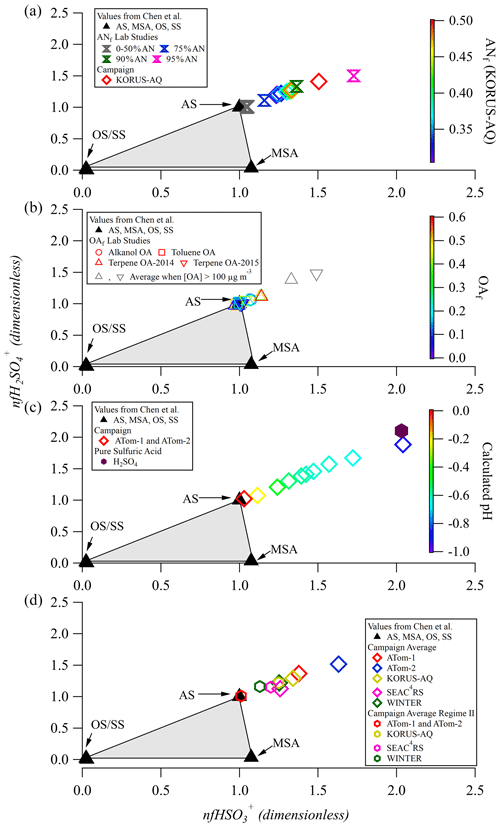
Figure 1Laboratory and field data for sulfate fragmentation shown in the triangle diagram proposed by Chen et al. (2019). (a) Data split into 10 quantiles of ANf value for the full KORUS-AQ campaign as well as for different laboratory internal mixtures of AS and AN. (b) Data from two chamber experiments, split into five quantiles of OAf. Data with very high OA (> 100 µg m−3) are shown as gray triangles. The average of OAf for the very high OA data in 2014 and 2015 is 0.8. Two separate datasets of monoterpene SOA chamber experiments are labeled as “2014” and “2015”. (c) Data split into 10 quantiles by calculated pH for ATom-1 and ATom-2, colored by calculated pH from E-AIM. (d) Averages for five aircraft campaigns for the full campaign and a subset of each campaign where pH > 0 and ANf < 0.3.
The effect of internally mixed ammonium nitrate (AN) is shown in Fig. 1a. For mixtures containing ANf < 50 %, data center around the pure-AS point in the Chen triangle. When ANf is increased past 0.50, there is an increase in both nfHySO ions, even when all of the particulate sulfate is inorganic. As the particle ANf increases up to ANf = 0.95, the OSf estimation becomes increasingly inaccurate. The method may estimate OSf = 0 % in the latter situation, when OSf is actually 50 %. While OSf = 0 % may be reasonable in some parts of the atmosphere, and one may be inclined to accept this result as it is non-negative, it is actually incorrect due to the effect of particulate AN. Thus for laboratory data, the Chen method should not be used on mixtures containing ANf > 0.50.
The effect of OA internally mixed with AS on the sulfate fragmentation pattern was also explored with toluene, alkanol, and monoterpene SOA (Figs. 1b and S6). For the alkanol SOA experiments we found that the presence of even a small coating of alkanol SOA (which is thought to be liquid; Liu et al., 2019) shifts the normalized AS [1,1] point to ∼ [1.08,1.08], but increases in the fraction of OA (OAf) from 0.1 to 0.3 lead to no further changes in nfHySO (Fig. 1b). This means that for a sample containing a mixture of AS and alkanol SOA, the calculated OSf would be −15 % (Chen et al., 2019). In contrast, toluene SOA, which spans 0 < OAf < 0.5, shows no clear change in the nfHySO ions, indicating that OAf would not bias the Chen method for this example. The monoterpene SOA, from two different experimental datasets (2014 and 2015) using different AMSs, show more varied results than the previous two studies. Overall, the 2014 data show a very small increase in the “pure” AS value in the OAf range 0–0.50, whereas the 2015 monoterpene data show a consistent and constant 10 %–20 % increase in nfHySO compared to the pure-AS calibration point (similarly to the alkanol SOA). However, when OAf is in the range of 0.50 < OAf < 0.70, 30 %–40 % increases are observed for the 2014 and 2015 data. This result is only applicable to a few of the experiments (see Fig. S6), potentially due to very high SOA loadings (up to 300 µg m−3). These high OA concentrations could potentially lead to a change in the particle phase due to condensation of more volatile and liquid species, potentially altering the interactions of the particles and the vaporizer surfaces. These experiments collectively suggest that a “pure” AS calibration point of [1.15,1.15] may be more appropriate when applying the Chen et al. (2019) method to some mixed aerosol at typical OA concentrations observed in the atmosphere; this is discussed further in Sect. 3.2.
Chen et al. (2019) briefly discussed the potential impact of acidity on their OS quantification method. This is explored here with pure-sulfuric-acid lab calibrations (Fig. 1c). Pure sulfuric acid shows a large deviation from the pure-AS triangle point (similar to increasing ANf), nearly doubling the values for the nfHySO ions. This implies that a particle containing sulfuric acid would produce a strong negative bias in the estimate of OS by the Chen method.
3.2 Evaluation of the Chen method with aircraft field studies
The results of applying the Chen et al. (2019) method to five aircraft campaigns are shown in Fig. 1. The effect of internally mixed ammonium nitrate (AN) was explored in Fig. 1a and Sect. 3.1 (for laboratory studies). Here we explore the effect for field data from KORUS-AQ (near Seoul, South Korea), where AN was often a major aerosol component (average ANf ∼ 0.18). As discussed in Sect. 3.1, as the percent of AN in laboratory mixtures of AS–AN increases, so do the nfHySO ions. The same effect is observed for the KORUS-AQ campaign, although the departure from the AS vertex is observed at substantially lower AN fractions for the field data (ANf ∼ 0.30). When field data are affected by AN, the Chen method might be applicable for situations with ANf < 0.30. At higher fractions, a correction could potentially be developed but with increased resulting uncertainty.
The effect of OA (shown in Fig. 1b for laboratory data) on sulfate fragmentation in ambient data is less clear due to the lack of data that have a lower ANf, higher pH, and little or no OS (see Table S1 for average campaign OAf). In the presence of any one of those factors, the sulfate fragmentation will be affected. It is especially challenging to confirm the absence of OS due to the lack of direct total OS measurements available. In Fig. S7, we isolate a subset of the KORUS-AQ dataset (where ANf < 0.3 and pH > 0, defined as “regime II” and discussed in detail in Sect. 3.4) to see if there is an offset in the AS under these chemical conditions as observed in the laboratory data shown in Fig. 1b. Similarly to the lab data, there appears to be a ∼ 10 % offset between the pure-AS fHySO values from calibrations and the KORUS-AQ data that occupy regime II (average OAf ∼ 43 %). This offset is smaller than some of the offsets observed in the laboratory data (Figs. 1b and S6) but may hinder the ability of the Chen OSf quantification method to estimate [OS] even in conditions where the pH > 0 and the ANf < 0.3.
In Fig. 1d, average values for each campaign in regime II, defined as ANf < 0.3 and calculated pH > 0, are shown. For less acidic aerosols and in the absence of OS or ANf effects, it is expected that the data would fall on top of the [1,1] pure-AS point in the 1D triangle plot, but this is not observed. This shift suggests that there are other factors (such as the presence of organics) that affect the location of the pure-AS point. In addition, the average values for the different campaigns vary substantially, so it is unlikely that a “corrected” pure-AS point can be used for all campaign and/or lab data.
To further look into the potential effect of acidity, we consider the ATom campaigns in Fig. 1c. ATom focused on remote oceanic air, with very low ANf (< 0.01). This is expected as AN is semivolatile (DeCarlo et al., 2008; Hennigan et al., 2008; Nault et al., 2018), and for the very-low-calculated-pH conditions during ATom (∼ −1 to 1, average of −0.6), most of the nitrate will be in the form of HNO3(g) (Guo et al., 2016). The Particle Analysis by Laser Mass Spectrometry (PALMS) instrument independently reports OSf ∼ 0.3 %–0.7 % for ATom (depending on the pH). The results for ATom span the range between pure AS and pure H2SO4, following a monotonic trend as acidity increases, consistent with the laboratory results and the results from the WINTER campaign in Chen et al. (2019). We hypothesize that high acidity is leading to the observed departure from the Chen triangle. Hence, the ATom results suggest that all of the sulfate sampled is inorganic, and if the Chen method is applied, then OSf = −26 % to +4 %. Thus the Chen method is insufficient to describe the trends observed for very acidic aerosols until calculated pH increases to ∼ 0 (where the ATom data start to converge onto the pure-AS data point). For campaigns containing particles of calculated pH > 0, the Chen method might be applicable.
To further illustrate that the ATom and KORUS-AQ campaigns are representative of the range of air masses in the troposphere, Fig. 1d shows results for two additional campaigns that focused on the continental US. SEAC4RS and WINTER represent chemical regimes that are not extremely acidic (average calculated-pH SEAC4RS ∼ −0.2, WINTER calculated pH ∼ 1.2). SEAC4RS had low ANf (∼ 0.04), while WINTER had high ANf (∼ 0.25). It is observed that every single campaign average falls outside of the triangle (for the full campaign and non-acidic, low ANf), indicating that the Chen et al. (2019) method, as proposed, is not applicable to many regions of the atmosphere. Average ANf, OAf, and calculated-pH values for different campaigns are shown in Table S1.
3.3 Physical interpretation of the sulfate fragmentation trends
We note that this section (Sect. 3.3) should be of most interest for AMS and/or ACSM users and can probably be skipped by others. It is useful to provide a physical interpretation of the trends that are likely driving the observed sulfate fragmentation changes based on the physicochemical details of the AMS detection and those of the particles being sampled. In Fig. 2a, a simplified diagram of the AMS detection process is shown, highlighting important details that are thought to give rise to the observed trends.
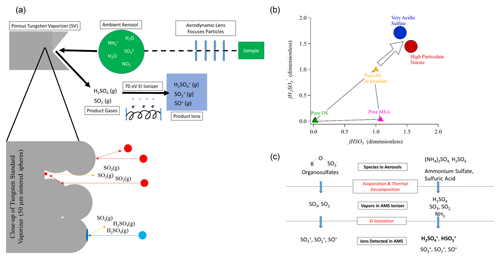
Figure 2(a) Simplified schematic of the AMS detection process, including a close-up of the tungsten standard vaporizer surface and the different species produced by AS and OS. (b) Conceptual model of the position of particles of different compositions in the Chen et al. (2019) triangle plot. As particles become more acidic or higher in particulate nitrate, the ratio of the AMS hydrogenated to total sulfate fragments increases. When sulfate is present as AS (or mixtures of AS and ammonium bisulfate), the sulfate fragmentation is mainly impacted by OS vs. AS vs. MSA relative concentrations inside the Chen triangle. (c) Schematic of the transformations during the AMS detection process for OS and AS.
Ambient particles containing AS, OS, and other species are sampled into the AMS through a focusing lens. Following a series of differential pumping steps through the instrument, the particles impact on a porous-tungsten standard vaporizer. The time spent under vacuum from sampling to detection is of the order of 15 ms. A fraction of the more viscous particles may bounce from the vaporizer without detection. Non-refractory species in the particles that stick to the vaporizer (such as OS and AS) are heated by heat transfer from the vaporizer surface. Some species may evaporate in the form in which they are present in the particle, while others may thermally decompose to other species, which then evaporate. For example, ammonium sulfate may evaporate to H2SO4(g) and NH3(g), but it may also thermally decompose to SO2(g), SO3(g), and H2O(g) (Hu et al., 2017b). Finally, these gaseous thermal-decomposition products undergo electron ionization to become positively charged species. Since the electrons used in electron impact (EI) have far more energy (70 eV) than typical bonds in a molecule (∼ 6 eV for S=O), the initial ions may fragment into smaller ions if the ionization process results in absorption of > 6 eV of internal energy by the molecule, beyond the ionization energy (Lambert, 1998). Some of the evaporated H2SO4(g) may remain as H2SO after ionization, or it may fragment to HSO or SO ions. SO2(g) can only produce SO ions. Thus the mixture of fragments observed will retain some memory of the species that evaporated from the particles. If the mixture of evaporating species is influenced by the particle composition (e.g., pH, AN, OA, or OSf), then it may be possible to calibrate the observed relationship to estimate an intensive chemical property of the particle.
Figure 2a also shows a schematic close-up of the SV surface, which is the main point in the instrument that controls ammonium sulfate fragmentation. In this diagram, we show a non-smooth surface with pores, consistent with the fabrication of the vaporizer by sintering 50 µm tungsten spheres. The interaction of a particle with this porous surface is dependent on the particle phase and/or viscosity. The red particles represent rigid (more solid-like) particles. These rigid particles can simply bounce off of the vaporizer, leading to no detection. AS-dominated particles are likely to be rigid (due to the solid phase of pure AS), thus increasing bounce and lowering the AMS CE (Matthew et al., 2008; Middlebrook et al., 2012). AS particles can also become trapped in the porous surface. When trapped, they are heated by conduction from the vaporizer surface and by radiation from surrounding surfaces. They reach higher temperatures that lead to more thermal decomposition and a lower H2SO4(g) SOx(g) ratio. Consistent with this interpretation, it was shown that the H2SO SO+ fragment ratio increased as the vaporizer temperature was reduced while sampling ambient air, while the SO SO+ ratio did not change (Fig. S5 in Docherty et al., 2015). In addition, molecules that evaporate as H2SO4(g) from these trapped particles are likely to collide with tungsten surfaces on their way out to the ionization region, leading to additional thermal decomposition (Hu et al., 2017b) and further reducing the H2SO4(g) SOx(g) ratio for the gases reaching the EI region and thus the HySO SO ion ratio.
The second case (blue particle) represents the situation where the particle is less rigid and/or viscous or liquid. Acidic sulfate particles (with a lower fraction of the sulfate ions neutralized by NH), particles with high ANf, or particles coated with a large water or liquid organic layer are more likely to deform upon impact and not bounce. This leads to an increased CE (Matthew et al., 2008; Middlebrook et al., 2012; Hu et al., 2017a). There are several effects that will lead to a higher H2SO4(g) SOx(g) ratio reaching the ionization region in this situation: (a) evaporated H2SO4(g) from particles that impact the front of the vaporizer and do not bounce can now escape without further collisions with the tungsten surface; (b) the increased surface area from impact deformation and the lower viscosity allow more H2SO4(g) molecules to escape the particle before those molecules are heated to temperatures that would lead to thermal decomposition.
In Fig. 2b, we show a conceptual model of the impact of these phenomena on the Chen triangle. For very acidic sulfate (approximately a calculated pH < 0), the liquid character of the particles leads to less bounce in the vaporizer. It also leads to faster evaporation, which reduces the internal temperature for the particles and that of the evaporated molecules, leading to less fragmentation. In this part of the atmosphere, OSf cannot be estimated, but pH may be as long as it can be assumed (or shown by additional measurements from the AMS or other instruments) that OSf and non-volatile cations are small. As an air mass becomes more neutralized by NH, the particles become less acidic and more rigid and/or viscous, leading to more thermal decomposition of the evaporated species, and the fragmentation of ammonium sulfate occurs at the upper vertex of the triangle. In this part of the atmosphere, methods such as Chen et al. (2019) may be applicable to estimate OSf as long as there are no other effects that interfere with the sulfate fragments detected (such as substantial non-volatile cations or variations in possible OA effects). As more ammonia is added to an air mass, the acidity of the particles decreases, and the higher pH favors the partitioning of HNO3(g) to the particle phase, forming ammonium nitrate. If ANf becomes high enough (> 0.3), the particles again become less rigid and/or viscous, and the fragmentation shifts again outside the Chen triangle for the same reasons discussed for the acidic particles. Finally, Fig. 2c shows the differences in the detection process and the fragments produced in the AMS for OS, AS, and H2SO4.
3.4 Specification of aerosol chemical regimes for feasibility of OSf quantification
In Fig. 3a, we introduce a plot of ANf vs. calculated pH that can be used to evaluate the applicability of the OSf methods to different datasets. Data for five different campaigns (those with AS calibrations, labeled “C” in Table 1) are shown, along with the campaign averages. Regime I (“highly acidic, low AN”) occupies the bottom left quadrant, where ANf < 0.3 and calculated pH < 0. Campaigns sampling the more remote atmosphere (e.g., ATom-1, 89 % of data points; ATom-2, 80 %) and a fraction of the data from continental campaigns (SEAC4RS, 13 %; DC3, 40 %) fall in this regime. For remote regions, emissions (such as NH3 and NOx) are generally low. Remote oceanic regions are relatively isolated from the major continental ammonia sources (Paulot et al., 2015). Therefore, less ammonia is available to balance the hydronium ions from H2SO4, leading to high acidity (Quinn et al., 1988; Keene, 2002; Nault et al., 2020). Highly acidic aerosols and lack of NH3 shift HNO3 to the gas phase, so low ANf is observed. In contrast, for sampling in polluted source regions with strong HNO3 formation and substantial NH3 emissions, a much smaller fraction of the data fall in this regime (e.g., only 4 % for KORUS-AQ). In Sect. 3.5 we discuss the potential to estimate pH from AMS data in regime I.
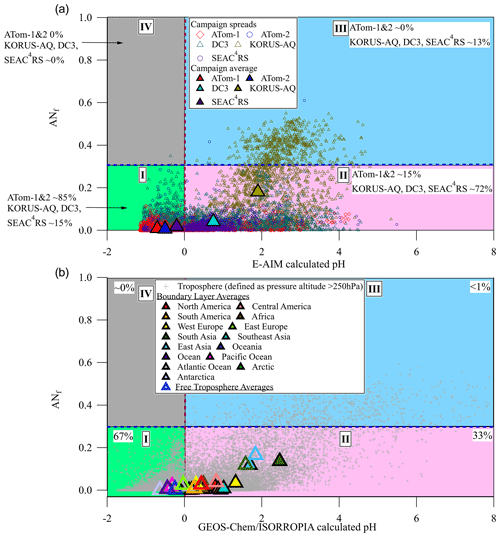
Figure 3(a) Location of the aircraft campaign 1 min data points on the chemical regimes defined in this paper (ANf, from AMS measurements) vs. E-AIM pH. SEAC4RS, WINTER, and KORUS-AQ are averaged to one value for brevity but defined individually in Sect. 3.4. (b) Location of global GEOS-Chem v12 results in the chemical regimes diagram. Yearlong averages shown as large triangles.
Regime II (lower right) involves less acidic conditions (calculated pH > 0) and lower ANf (< 0.3). In this region sulfate fragmentation in the AMS is not strongly impacted by either ANf or acidity. In principle, in this regime the recently proposed sulfate deconvolution methods could be applicable. The geographical regions studied in Chen et al. (2019) and Song et al. (2019) generally fall in this regime, and this might explain the lack of large negative OSf values in those studies in contrast to our observations for other regions. About half of our campaign data are located in this regime, more so for the continental campaigns and much less so for the remote campaigns. Specifically, 65 % of KORUS-AQ, 60 % of DC3, 87 % of SEAC4RS, 11 % of ATom-1, and 20 % of ATom-2 fall in this regime. We have applied the 1D version of the Chen method to each field campaign after filtering it by the ANf and calculated-pH constraints for regime II. OSf is nominally slightly greater than 0 for ATom-1, OSf ∼ 3 %, of the order of the 0.3 % estimate in regime II from PALMS (for ATom-1 and ATom-2, estimated by only considering the sulfate moiety from the isoprene-derived epoxydiol (IEPOX) or glycolic acid sulfate (GAS) OS, neither of which was detected in the supermicron aerosol; Froyd et al., 2009, 2019; Liao et al., 2015) (see Fig. S8). However, OSf is much less than 0 for ATom-2 (OSf ∼ −23 %) and KORUS-AQ (OSf ∼ −26 %). These unreasonable results may be due to the effect of OA on sulfate fragmentation in the AMS (discussed in Sect. 3.2). For this reason, strong caution is advised in applying OSf estimation methods to ambient data, even in regime II. In addition, estimating OS with sulfate ions may be susceptible to errors due to inaccuracies in AS calibrations, noise present in the ambient data, or other factors.
We also show results from applying the Song et al. (2019) method in regime II (which is based on similar principles to the Chen method) in Fig. S9. Similarly to the Chen method, we see that most OSf values are predicted to be less than 0. For the entire atmosphere, shown in Fig. S10, the distribution for OSf looks similar to Fig. S9.
Regime III is characterized by high ANf (> 0.3) and lower acidity (calculated pH > 0). This chemical regime primarily exists in polluted continental regions near large source regions such as megacities and agricultural regions as high NOx and NH3 emissions can lead to increased particulate AN and an increase in aerosol pH (Pye et al., 2020). In this regime, there are strong variations in the AMS sulfate fragments that are driven by ANf. OSf cannot be estimated with the AMS sulfate fragmentation methods proposed so far unless they are further modified to account for the ANf effect. Around 31 % of KORUS-AQ data fall in this regime, but almost none of the data from the rural and/or remote campaigns fall in this region as AN typically evaporates as the air is diluted during advection away from polluted regions (DeCarlo et al., 2008).
Finally, regime IV in the top left quadrant has high AN (ANf > 0.3) and high acidity (calculated pH < 0). This chemical regime is unlikely to be observed in the real atmosphere, and indeed there are very few points in that region for our campaigns. Sulfate is ubiquitous (Zhang et al., 2007b; Hodzic et al., 2020), and nitrate is not thermodynamically stable in the aerosol phase together with acidic sulfate for calculated pH < 0 (Guo et al., 2016). For all campaigns we observe ∼ 0 % of points occupying this regime. Very unusual data points can be observed when ammonium-nitrate-containing particles are externally mixed with acidic-sulfate-containing particles in an air mass.
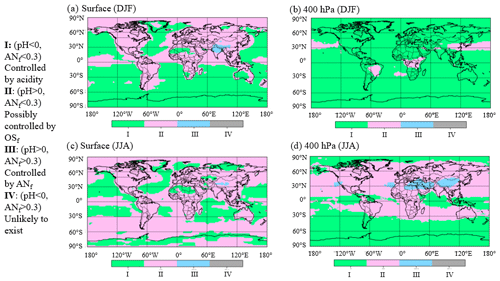
Figure 4Areas characterized by different chemical regimes according to results from GEOS-Chem v12. (a) Surface for December, January, and February (DJF); (b) 400 hPa for DJF; (c) surface for June, July, and August (JJA); (d) 400 hPa for JJA. Roman numerals correspond to regimes in Fig. 3.
Since the field studies analyzed here targeted large regions but did not sample many others, it is of interest to evaluate the fraction of the troposphere located in each one of the chemical regimes. The results of the GEOS-Chem v12 model are used for this purpose in Fig. 3b and shown as a global map in Figs. 4 and S11. About 67 % of the model troposphere exists in regime I (calculated pH < 0). In addition, ∼ 33 % of the global troposphere exists in regime II, where it may be feasible to estimate OSf from AMS fragments. Less than 1 % of the modeled atmosphere exists in regime III (upper right quadrant), where ammonium nitrate strongly influences sulfate fragmentation, consistent with the relatively small, very polluted geographical regions with very large ANf. Finally, none of the data fell in regime IV, consistent with aerosols being assumed to be internally mixed in GEOS-Chem. At the surface during December, January, and February (DJF) (Fig. 4a), most of the remote oceans fall in regime I (calculated pH < 0 and ANf < 0.3), while regime II (calculated pH > 0 and ANf < 0.3) is dominant over continental regions. At the surface in June, July, and August (JJA) (Fig. 4c), most of the globe is in regime II. Very little of the data fall in regime III, except parts of Asia, regardless of season. A similar pattern is observed in the free troposphere (Fig. 4b and d), with some geographical differences. Regime III (calculated pH > 0 and ANf > 0.3), which represents pollution hotspots, is observed in a large region in Asia during the summer months, whereas the winter months are dominated by regime I (low pH). The summer months in the free troposphere are also mostly in regime II, especially over continental regions. Due to averaging of an entire year as well as the limited spatial resolution of the GEOS-Chem model, locations and periods of high-ANf hotspots are not as prominent in these results, even when the data are divided by season.
3.5 Potential pH estimation from AMS measurements
3.5.1 Estimation of pH from AMS sulfate fragments
In Sect. 3.4, we introduced chemical regime I with low calculated pH and low ANf. In this regime, which encompasses about half of the campaign data and two-thirds of the modeled global troposphere, PALMS data show that the overwhelming majority of the sulfate is inorganic, with OSf contributing ∼ 0.7 % to total sulfate by mass during ATom-1 and ATom-2 when calculated pH < 0 (in regime I; see Fig. S8). This removes sulfate fragmentation changes caused by AN and sulfate type (OS vs. AS), indicating that sulfate fragmentation is almost exclusively controlled by the acidity of the aerosol. Figure 1c shows that fH2SO and fHSO, i.e., the number of sulfate fragments retaining one or two hydrogens (H2SO and HSO) relative to the total sulfate fragments (H2SO, HSO, SO, SO, and SO+), increase as calculated pH decreases.
In Fig. 5 we show the relationship between HySO SO and aerosol pH. As the relationship is noisy for individual data points, we show the results for 5 % quantiles of the data. HySO SO appears to show a proportional relationship with decreasing calculated pH for the ATom campaigns, for which much of the data are in regime I. The KORUS-AQ data, of which very few fall in the regime I, do not show a relationship between these variables, as expected. A fitted equation to the ATom relationship may allow the real-time estimation of pH for different air masses for campaigns in regime I as
As shown in the histogram in Fig. 5b, this relationship is applicable to a substantial fraction of ambient observations. This estimation equation likely needs to be calibrated for each instrument (e.g., by sampling sulfate particles with different acidities) since the sulfate fragmentation does vary with instrument (Chen et al., 2019) and potentially also in time for a given instrument.
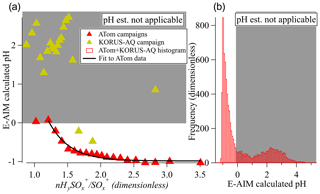
Figure 5(a) Calculated pH vs. sulfate fragmentation indicator (HySO SO) for the ATom and KORUS-AQ campaigns and binned by nHySO SO. The black line is an exponential fit to ATom data (see text) when calculated pH < 0. (b) Histogram of the calculated pH for the 1 min data points from the ATom-1, ATom-2, and KORUS-AQ datasets. In both panels, the white (gray) area shows the regime where calculated pH can (and cannot) be estimated from the sulfate fragmentation.
Although an estimation equation that apparently works for only one unit of pH may seem of limited value, two caveats apply: first, it is of high value to know that the estimated pH < 0 for a certain air mass (as opposed to, e.g., estimated pH = 2 or 3, which is frequently encountered). Second, the range of estimated pH below 0 is limited here due to not considering the activity coefficient. If that coefficient were included, the predicted estimated-pH range in this regime would be ∼ −4 to 0.
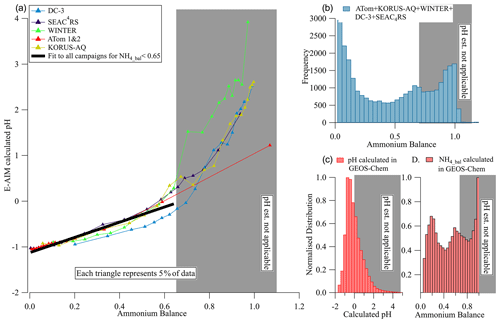
Figure 6(a) Calculated pH vs. ammonium balance for multiple campaigns. Quantiles of the data are used to reduce the impact of noise. The black line is an orthogonal distance regression (ODR) fit to the campaign data for values with NH4_Bal < 0.65. (b) Histogram of measured ammonium balance for the six campaigns. (c, d) Calculated pH and ammonium balance from GEOS-Chem (pH calculated with ISORROPIA). In all panels the white (gray) areas encompass the data points for which calculated pH can (and cannot) be estimated from the measured ammonium balance.
3.5.2 Estimation of pH from ammonium balance
Ammonium balance (NH4_bal) (Eq. 7) is often used as a qualitative indicator of acidity. Zhang et al. (2007a) showed that calculated pH under constant temperature and RH was well correlated with ammonium balance, but much more scatter was observed when the instantaneous T and RH were used. Several studies have argued that ammonium balance cannot be used to estimate ambient pH (e.g., Guo et al., 2015, 2016; Hennigan et al., 2015; Weber et al., 2016); however, those studies were all performed at continental ground sites that were in the less-acidic chemical regimes (II and III) and where daily temperature and humidity changes were strong. As shown in Fig. 6, NH4_bal and calculated pH for the aircraft studies show a strong and consistent relationship in regime I (calculated pH < 0), providing another potential method for estimating pH (all one needs to use this method is the ammonium balance, and if it is < 0.65, the method should be applicable). As ammonium balance increases, so does calculated pH across the six campaigns studied. These data are generally outside of the continental boundary layer, where temperature and RH change less in a diurnal cycle, reducing the impact of those changes on pH. For data in regimes II–III (calculated pH > 0), some proportionality of pH and NH4_bal is still observed on average but with more dispersion across campaigns. Given the similarity of the results for regime I, the fitting equation of calculated pH vs. ammonium balance may be used to provide a near-real-time estimate of pH (for NH4_bal < 0.65).
As shown in the histogram in Fig. 6b–d, this relationship is also applicable to a substantial fraction of ambient regions. This estimation equation should be tested with other studies. An advantage of this relationship (vs. the one based on HySO SO) is that it is likely to be less instrument-dependent as long as careful calibrations of RIE and RIE have been performed. Conditions where non-volatile cations (e.g., Na+, K+, Ca2+) are important for submicron particles could lead to deviations from this relationship (Guo et al., 2020). However, such conditions are infrequent in remote air (Nault et al., 2020) and can be diagnosed by concurrent supermicron or filter measurements.
3.5.3 Application of pH estimation methods to ambient data
As discussed above, ammonium balance and HySO SO are two measurements that may be used to estimate aerosol acidity in parts of the atmosphere. In Fig. 7 these two methods are applied to one flight during ATom-1 and an SO2 plume sampled during WINTER. In Fig. 7a, both HySO SO and NH4_bal follow the trend for E-AIM calculated pH during most periods when calculated pH < 0, even at 1 min time resolution.
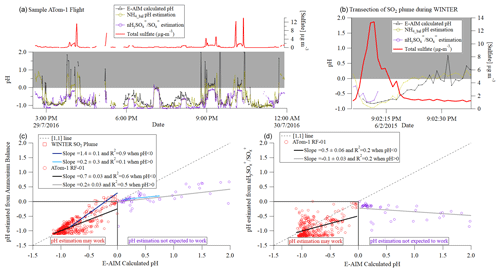
Figure 7(a) Time series of sulfate, pH calculated from E-AIM and estimated from HySO SO, and NH4_Bal for one flight during ATom-1 (at 1 min. resolution, filtered to remove points where sulfate was less than 3 times its detection limit). (b) Time series of sulfate and pH for a large power plant plume sampled during WINTER; only a few data points are shown for pH estimated from nHySO SO because sulfate in the AMS evaporated slowly during the second half of the plume transect, leading to altered sulfate fragmentation, and this effect cannot be corrected for due to infrequent backgrounds in aircraft fast-acquisition mode. (c) Scatterplot of estimated pH predicted from NH4_Bal- vs. E-AIM calculated pH for the data above. (d) Scatterplot of estimated pH predicted from nHySO SO- vs. E-AIM calculated pH for the ATom flight.
As expected from Fig. 6, NH4_bal is a less noisy, more robust metric for estimating pH at 1 min time resolution. Unlike HySO SO, NH4_bal appears to be able to capture basic calculated-pH trends at the full range of calculated-pH values observed during this flight in ATom-1. NH4_bal also matches the E-AIM calculated pH well for the WINTER power plant plume. For RF01 in ATom-1 (WINTER), NH4_bal estimated pH has an R2 ∼ 0.6 (0.9) for pH < 0 (Fig. 7c–d). This shows that in the remote atmosphere (like in ATom) or in an SO2 plume, NH4_bal has the potential to allow fast estimation of pH, even under relatively low sulfate concentrations, albeit not perfectly. More scatter is observed for the estimate based on HySO SO, indicating that longer averages are needed for this method. The error is typically within ±0.5 estimated-pH units, which is thought to be the accuracy of thermodynamic pH estimation models.
3.6 Possibility of estimating collection efficiency (CE) from sulfate fragmentation
From the previous discussion it is clear that sulfate fragmentation changes due to some of the same factors (acidity, ANf) that influence ambient AMS CE. It is of interest to explore whether a quantitative estimate of ambient-particle CE could be derived from the measured sulfate fragments, at least under some conditions, as it could provide a complementary characterization to the CE estimates from the Middlebrook et al. (2012) parameterization. In Fig. 8 we show the CE estimated from Middlebrook et al. (2012) vs. HySO SO for ATom and KORUS-AQ. CE does show some relationship with HySO SO, with most sensitivity around CE ∼ 0.8–0.9. A substantial level of noise is observed in the high-time-resolution data, and the trend varies between the two campaigns (where variations in CE are controlled by two different effects, acidity vs. ANf). Further research would be necessary to evaluate whether this method could be used to estimate CE.
The presence of organosulfates in particles is a topic of much recent interest, but there is a lack of online methods to quantify them. Two methods have been proposed to use widely available AMS data to quantify OSf (Chen et al., 2019; Song et al., 2019). These methods have only been applied to ground continental datasets to our knowledge. We show using both laboratory and field data that both high acidity (regime I) and high ANf (regime III) result in major changes in sulfate fragmentation, which often lead to nonsensical results for the OSf methods. Regime I accounts for around two-thirds of the global troposphere, while regime III can be important in polluted regions (e.g., Seoul region), and thus it is critical to avoid applying the proposed OSf estimation methods in these regimes. In regime II, with lower acidity and lower nitrate (calculated pH > 0, ANf < 0.3), OSf estimation methods may be applicable if no other effects (e.g., significant non-volatile cations or variations in OA effects) confound the sulfate fragmentation. For the ambient data analyzed here, even in regime II the OSf estimation produced nonsensical results. Extreme caution is recommended to anyone who chooses to apply the OSf estimation methods. For reasons not fully understood, fragmentation of the sulfate ions in the lab vs. ambient data differs at times.
We investigated two different methods to estimate pH in real time in regime I (calculated pH < 0 and ANf < 0.3) based on the AMS HySO SO fragment ratio and the ammonium balance, respectively, without the need to run a thermodynamic model and without the need for gas-phase NH3 or HNO3 measurements. Low OSf and non-volatile cations need to be assumed or confirmed from AMS and other measurements. The ammonium balance method shows better performance. These in situ and direct pH estimation methods should be applicable in the remote atmosphere (oceanic regions and often the continental free troposphere when not recently impacted by surface sources). Both the OSf and pH estimations require careful instrument calibration for a given campaign, and the methods based on sulfate fragments are expected to be instrument-dependent, including for the same instrument in time when filaments or the vaporizer are replaced or when the instrument is re-tuned. Both methods should be further evaluated with data from other studies.
We propose a conceptual model to explain the observed sulfate fragmentation changes with changing particle chemical composition. As particles become more acidic or higher in AN, a higher fraction of H2SO4(g) can reach the ionization region, leading to changes in the observed ion population. Since AMS CE is thought to be controlled by the same effects, we explore whether it can be estimated from the observed sulfate fragmentation and find that, while changes in HySO SO do correlate to changes in CE, the relationship is not the same across different campaigns. Further investigation of this relationship, especially when direct CE measurements are available via internal AMS light scattering, would be of interest.
We have not explored the application of these methods to ACSM data. ACSM data are unit mass resolution, and the interferences between species at a given unit mass are estimated using a fragmentation table approach (Allan et al., 2004). This approach introduces more uncertainties, as exemplified by Hu et al. (2015) for similar fragment-based methods.
DC3 data are available at https://doi.org/10.5067/Aircraft/DC3/DC8/Aerosol-TraceGas (DC3 Science Team, 2012). SEAC4RS data are available at https://doi.org/10.5067/Aircraft/SEAC4RS/Aerosol-TraceGas-Cloud (SEAC4RS Science Team, 2013). WINTER data are available at https://data.eol.ucar.edu/master_lists/generated/winter/ (WINTER Science Team, 2015). KORUS-AQ data are available at https://doi.org/10.5067/Suborbital/KORUSAQ/DATA01 (KORUS-AQ Science Team, 2016). ATom-1 and ATom-2 data are available at https://doi.org/10.3334/ORNLDAAC/158 (Hall et al., 1996). GEOS-Chem 12.6.1 data are available at https://doi.org/10.5281/zenodo.3520966 (The International GEOS-Chem User Community, 2019).
The supplement related to this article is available online at: https://doi.org/10.5194/amt-14-2237-2021-supplement.
MKS, BAN, and JLJ conceived the study. BAN, PCJ, DAD, JCS, and BBP collected the AMS data. AH provided data from GEOS-Chem, and JED provided SAGA measurements for the inputs of the E-AIM model. MKS, BAN, PCJ, DAD, DSJ, and JLJ analyzed the data. MKS wrote the paper, with assistance from BAN, DSJ, and JLJ. All authors reviewed and provided comments for the manuscript.
The authors declare that they have no conflict of interest.
We thank the members of the Jimenez group, the AMS user community, Weiwei Hu, Amber Ortega, and Patrick Hayes for help with data acquisition during SEAC4RS and DC-3; Jason St. Clair, Alex Teng, Michelle Kim, John Crounse, and Paul Wennberg for providing CIT-CIMS HNO3 data; Joel Thornton, Felipe Lopez-Hilfiker, and Ben Lee for providing UW-CIMS HNO3 data during WINTER; Karl Froyd, Gregory P. Schill, and Daniel Murphy for providing PALMS organosulfate data for ATom campaigns; and Glenn Diskin for providing DLH H2O data. We acknowledge high-performance computing support from Cheyenne (https://doi.org/10.5065/D6RX99HX, Computational and Information Systems Laboratory, 2019) provided by NCAR's Computational and Information Systems Laboratory, sponsored by the National Science Foundation.
This work was supported by NASA grants NNX15AH33A, NNX15AJ23G, 80NSSC18K0630, 80NSSC19K0124, and 19-EARTH20-0193 and a CIRES fellowship to Melinda K. Schueneman.
This paper was edited by Hang Su and reviewed by two anonymous referees.
Ackendorf, J. M., Ippolito, M. G., and Galloway, M. M.: pH Dependence of the Imidazole-2-carboxaldehyde Hydration Equilibrium: Implications for Atmospheric Light Absorbance, Environ. Sci. Technol. Lett., 4, 551–555, https://doi.org/10.1021/acs.estlett.7b00486, 2017.
Alfarra, M. R., Coe, H., Allan, J. D., Bower, K. N., Boudries, H., Canagaratna, M. R., Jimenez, J. L., Jayne, J. T., Garforth, A. A., Li, S.-M., and Worsnop, D. R.: Characterization of urban and rural organic particulate in the Lower Fraser Valley using two Aerodyne Aerosol Mass Spectrometers, Atmos. Environ., 38, 5745–5758, https://doi.org/10.1016/j.atmosenv.2004.01.054, 2004.
Allan, J. D., Delia, A. E., Coe, H., Bower, K. N., Alfarra, M. R., Jimenez, J. L., Middlebrook, A. M., Drewnick, F., Onasch, T. B., Canagaratna, M. R., Jayne, J. T., and Worsnop, D. R.: A generalised method for the extraction of chemically resolved mass spectra from Aerodyne aerosol mass spectrometer data, J. Aerosol Sci., 35, 909–922, https://doi.org/10.1016/j.jaerosci.2004.02.007, 2004.
Anon: Atmospheric chemistry and physics: from air pollution to climate change, Choice Reviews Online, 44, 4512–4512, https://doi.org/10.5860/CHOICE.44-4512, 2007.
Bahreini, R., Dunlea, E. J., Matthew, B. M., Simons, C., Docherty, K. S., DeCarlo, P. F., Jimenez, J. L., Brock, C. A., and Middlebrook, A. M.: Design and Operation of a Pressure-Controlled Inlet for Airborne Sampling with an Aerodynamic Aerosol Lens, Aerosol Sci. Technol., 42, 465–471, https://doi.org/10.1080/02786820802178514, 2008.
Bahreini, R., Ervens, B., Middlebrook, A. M., Warneke, C., de Gouw, J. A., DeCarlo, P. F., Jimenez, J. L., Brock, C. A., Neuman, J. A., Ryerson, T. B., Stark, H., Atlas, E., Brioude, J., Fried, A., Holloway, J. S., Peischl, J., Richter, D., Walega, J., Weibring, P., Wollny, A. G., and Fehsenfeld, F. C.: Organic aerosol formation in urban and industrial plumes near Houston and Dallas, Texas, J. Geophys. Res., 114, 1185, https://doi.org/10.1029/2008JD011493, 2009.
Barth, M. C., Cantrell, C. A., Brune, W. H., Rutledge, S. A., Crawford, J. H., Huntrieser, H., Carey, L. D., MacGorman, D., Weisman, M., Pickering, K. E., Bruning, E., Anderson, B., Apel, E., Biggerstaff, M., Campos, T., Campuzano-Jost, P., Cohen, R., Crounse, J., Day, D. A., Diskin, G., Flocke, F., Fried, A., Garland, C., Heikes, B., Honomichl, S., Hornbrook, R., Huey, L. G., Jimenez, J. L., Lang, T., Lichtenstern, M., Mikoviny, T., Nault, B., O'Sullivan, D., Pan, L. L., Peischl, J., Pollack, I., Richter, D., Riemer, D., Ryerson, T., Schlager, H., St. Clair, J., Walega, J., Weibring, P., Weinheimer, A., Wennberg, P., Wisthaler, A., Wooldridge, P. J., and Ziegler, C.: The Deep Convective Clouds and Chemistry (DC3) Field Campaign, B. Am. Meteorol. Soc., 96, 1281–1309, https://doi.org/10.1175/BAMS-D-13-00290.1, 2015.
Bertram, T. H. and Thornton, J. A.: Toward a general parameterization of N2O5 reactivity on aqueous particles: the competing effects of particle liquid water, nitrate and chloride, Atmos. Chem. Phys., 9, 8351–8363, https://doi.org/10.5194/acp-9-8351-2009, 2009.
Bey, I., Jacob, D. J., Yantosca, R. M., Logan, J. A., Field, B. D., Fiore, A. M., Li, Q., Liu, H. Y., Mickley, L. J., and Schultz, M. G.: Global modeling of tropospheric chemistry with assimilated meteorology: Model description and evaluation, J. Geophys. Res., 106, 23073–23095, https://doi.org/10.1029/2001JD000807, 2001.
Brock, C.: Interactive comment on “Aerosol sizedistributions during the Atmospheric Tomography(ATom) mission: methods, uncertainties, and dataproducts”, Atmos. Meas. Tech. Discuss., https://doi.org/10.5194/amt-2019-44-ac1, 2019.
Brüggemann, M., Riva, M., Perrier, S., Poulain, L., George, C., and Herrmann, H.: Overestimation of Monoterpene Organosulfate Abundance in Aerosol Particles by Sampling in the Presence of SO2, Environ. Sci. Technol. Lett., 8, 206–211, https://doi.org/10.1021/acs.estlett.0c00814, 2021.
Canagaratna, M. R., Jayne, J. T., Jimenez, J. L., Allan, J. D., Alfarra, M. R., Zhang, Q., Onasch, T. B., Drewnick, F., Coe, H., Middlebrook, A., Delia, A., Williams, L. R., Trimborn, A. M., Northway, M. J., DeCarlo, P. F., Kolb, C. E., Davidovits, P., and Worsnop, D. R.: Chemical and microphysical characterization of ambient aerosols with the aerodyne aerosol mass spectrometer, Mass Spectrom. Rev., 26, 185–222, https://doi.org/10.1002/mas.20115, 2007.
Carlton, A. G., de Gouw, J., Jimenez, J. L., Ambrose, J. L., Attwood, A. R., Brown, S., Baker, K. R., Brock, C., Cohen, R. C., Edgerton, S., Farkas, C. M., Farmer, D., Goldstein, A. H., Gratz, L., Guenther, A., Hunt, S., Jaeglé, L., Jaffe, D. A., Mak, J., McClure, C., Nenes, A., Nguyen, T. K., Pierce, J. R., de Sa, S., Selin, N. E., Shah, V., Shaw, S., Shepson, P. B., Song, S., Stutz, J., Surratt, J. D., Turpin, B. J., Warneke, C., Washenfelder, R. A., Wennberg, P. O., and Zhou, X.: Synthesis of the Southeast Atmosphere Studies: Investigating Fundamental Atmospheric Chemistry Questions, B. Am. Meteorol. Soc., 99, 547–567, https://doi.org/10.1175/BAMS-D-16-0048.1, 2018.
Chen, Y., Xu, L., Humphry, T., Hettiyadura, A. P. S., Ovadnevaite, J., Huang, S., Poulain, L., Schroder, J. C., Campuzano-Jost, P., Jimenez, J. L., Herrmann, H., O'Dowd, C., Stone, E. A., and Ng, N. L.: Response of the Aerodyne Aerosol Mass Spectrometer to Inorganic Sulfates and Organosulfur Compounds: Applications in Field and Laboratory Measurements, Environ. Sci. Technol., 53, 5176–5186, https://doi.org/10.1021/acs.est.9b00884, 2019.
Clegg, S. L., Brimblecombe, P., and Wexler, A. S.: Thermodynamic Model of the System H+-NH-SO -NO-H2O at Tropospheric Temperatures, J. Phys. Chem. A, 102, 2137–2154, https://doi.org/10.1021/jp973042r, 1998.
Clegg, S. L., Seinfeld, J. H., and Edney, E. O.: Thermodynamic modelling of aqueous aerosols containing electrolytes and dissolved organic compounds. II. An extended Zdanovskii–Stokes–Robinson approach, J. Aerosol Sci., 34, 667–690, https://doi.org/10.1016/S0021-8502(03)00019-3, 2003.
Computational and Information Systems Laboratory: Cheyenne: HPE/SGI ICE XA System (University Community Computing), Boulder, CO: National Center for Atmospheric Research, https://doi.org/10.5065/D6RX99HX, 2019.
Craig, R. L., Peterson, P. K., Nandy, L., Lei, Z., Hossain, M. A., Camarena, S., Dodson, R. A., Cook, R. D., Dutcher, C. S., and Ault, A. P.: Direct Determination of Aerosol pH: Size-Resolved Measurements of Submicrometer and Supermicrometer Aqueous Particles, Anal. Chem., 90, 11232–11239, https://doi.org/10.1021/acs.analchem.8b00586, 2018.
Crounse, J. D., McKinney, K. A., Kwan, A. J., and Wennberg, P. O.: Measurement of gas-phase hydroperoxides by chemical ionization mass spectrometry, Anal. Chem., 78, 6726–6732, https://doi.org/10.1021/ac0604235, 2006.
Cubison, M. J., Ortega, A. M., Hayes, P. L., Farmer, D. K., Day, D., Lechner, M. J., Brune, W. H., Apel, E., Diskin, G. S., Fisher, J. A., Fuelberg, H. E., Hecobian, A., Knapp, D. J., Mikoviny, T., Riemer, D., Sachse, G. W., Sessions, W., Weber, R. J., Weinheimer, A. J., Wisthaler, A., and Jimenez, J. L.: Effects of aging on organic aerosol from open biomass burning smoke in aircraft and laboratory studies, Atmos. Chem. Phys., 11, 12049–12064, https://doi.org/10.5194/acp-11-12049-2011, 2011.
DC3 Science Team: DC3 data, NASA, https://doi.org/10.5067/Aircraft/DC3/DC8/Aerosol-TraceGas, 2012.
DeCarlo, P. F., Kimmel, J. R., Trimborn, A., Northway, M. J., Jayne, J. T., Aiken, A. C., Gonin, M., Fuhrer, K., Horvath, T., Docherty, K. S., Worsnop, D. R., and Jimenez, J. L.: Field-deployable, high-resolution, time-of-flight aerosol mass spectrometer, Anal. Chem., 78, 8281–8289, https://doi.org/10.1021/ac061249n, 2006.
DeCarlo, P. F., Dunlea, E. J., Kimmel, J. R., Aiken, A. C., Sueper, D., Crounse, J., Wennberg, P. O., Emmons, L., Shinozuka, Y., Clarke, A., Zhou, J., Tomlinson, J., Collins, D. R., Knapp, D., Weinheimer, A. J., Montzka, D. D., Campos, T., and Jimenez, J. L.: Fast airborne aerosol size and chemistry measurements above Mexico City and Central Mexico during the MILAGRO campaign, Atmos. Chem. Phys., 8, 4027–4048, https://doi.org/10.5194/acp-8-4027-2008, 2008.
Dentener, F. J. and Crutzen, P. J.: A three-dimensional model of the global ammonia cycle, J. Atmos. Chem., 19, 331–369, https://doi.org/10.1007/BF00694492, 1994.
Docherty, K. S., Lewandowski, M., and Jimenez, J. L.: Effect of Vaporizer Temperature on Ambient Non-Refractory Submicron Aerosol Composition and Mass Spectra Measured by the Aerosol Mass Spectrometer, Aerosol Sci. Technol., 49, 485–494, https://doi.org/10.1080/02786826.2015.1042100, 2015.
Dockery, D. W., Cunningham, J., Damokosh, A. I., Neas, L. M., Spengler, J. D., Koutrakis, P., Ware, J. H., Raizenne, M., and Speizer, F. E.: Health effects of acid aerosols on North American children: respiratory symptoms, Environ, Health Perspect., 104, 500–505, https://doi.org/10.1289/ehp.96104500, 1996.
Dovrou, E., Lim, C. Y., Canagaratna, M. R., Kroll, J. H., Worsnop, D. R., and Keutsch, F. N.: Measurement techniques for identifying and quantifying hydroxymethanesulfonate (HMS) in an aqueous matrix and particulate matter using aerosol mass spectrometry and ion chromatography, Atmos. Meas. Tech., 12, 5303–5315, https://doi.org/10.5194/amt-12-5303-2019, 2019.
Drewnick, F., Hings, S. S., Alfarra, M. R., Prevot, A. S. H., and Borrmann, S.: Aerosol quantification with the Aerodyne Aerosol Mass Spectrometer: detection limits and ionizer background effects, Atmos. Meas. Tech., 2, 33–46, https://doi.org/10.5194/amt-2-33-2009, 2009.
Dunlea, E. J., DeCarlo, P. F., Aiken, A. C., Kimmel, J. R., Peltier, R. E., Weber, R. J., Tomlinson, J., Collins, D. R., Shinozuka, Y., McNaughton, C. S., Howell, S. G., Clarke, A. D., Emmons, L. K., Apel, E. C., Pfister, G. G., van Donkelaar, A., Martin, R. V., Millet, D. B., Heald, C. L., and Jimenez, J. L.: Evolution of Asian aerosols during transpacific transport in INTEX-B, Atmos. Chem. Phys., 9, 7257–7287, https://doi.org/10.5194/acp-9-7257-2009, 2009.
Facchini, M. C., Decesari, S., Rinaldi, M., Carbone, C., Finessi, E., Mircea, M., Fuzzi, S., Moretti, F., Tagliavini, E., Ceburnis, D., and O'Dowd, C. D.: Important source of marine secondary organic aerosol from biogenic amines, Environ. Sci. Technol., 42, 9116–9121, https://doi.org/10.1021/es8018385, 2008.
Farmer, D. K., Matsunaga, A., Docherty, K. S., Surratt, J. D., Seinfeld, J. H., Ziemann, P. J., and Jimenez, J. L.: Response of an aerosol mass spectrometer to organonitrates and organosulfates and implications for atmospheric chemistry, P. Natl. Acad. Sci. USA, 107, 6670–6675, https://doi.org/10.1073/pnas.0912340107, 2010.
Fountoukis, C. and Nenes, A.: ISORROPIA II: a computationally efficient thermodynamic equilibrium model for K+–Ca2+–Mg2+–NH–Na+–SO–NO–Cl−–H2O aerosols, Atmos. Chem. Phys., 7, 4639–4659, https://doi.org/10.5194/acp-7-4639-2007, 2007.
Friese, E. and Ebel, A.: Temperature Dependent Thermodynamic Model of the System H-NH4-Na-SO42–NO3–Cl–H2O, J. Phys. Chem. A, 114, 11595–11631, https://doi.org/10.1021/jp101041j, 2010.
Frossard, A. A., Russell, L. M., Burrows, S. M., Elliott, S. M., Bates, T. S. and Quinn, P. K.: Sources and Composition of Submicron Organic Mass in Marine Aerosol Particles, J. Geophys. Res.-Atmos., 119, 977–13, 003, https://doi.org/10.1002/2014JD021913, 2014.
Froyd, K. D., Murphy, D. M., Sanford, T. J., Thomson, D. S., Wilson, J. C., Pfister, L., and Lait, L.: Aerosol composition of the tropical upper troposphere, Atmos. Chem. Phys., 9, 4363–4385, https://doi.org/10.5194/acp-9-4363-2009, 2009.
Froyd, K. D., Murphy, D. M., Brock, C. A., Campuzano-Jost, P., Dibb, J. E., Jimenez, J.-L., Kupc, A., Middlebrook, A. M., Schill, G. P., Thornhill, K. L., Williamson, C. J., Wilson, J. C., and Ziemba, L. D.: A new method to quantify mineral dust and other aerosol species from aircraft platforms using single-particle mass spectrometry, Atmos. Meas. Tech., 12, 6209–6239, https://doi.org/10.5194/amt-12-6209-2019, 2019.
Fry, J. L., Draper, D. C., Zarzana, K. J., Campuzano-Jost, P., Day, D. A., Jimenez, J. L., Brown, S. S., Cohen, R. C., Kaser, L., Hansel, A., Cappellin, L., Karl, T., Hodzic Roux, A., Turnipseed, A., Cantrell, C., Lefer, B. L., and Grossberg, N.: Observations of gas- and aerosol-phase organic nitrates at BEACHON-RoMBAS 2011, Atmos. Chem. Phys., 13, 8585–8605, https://doi.org/10.5194/acp-13-8585-2013, 2013.
Gaston, C. J., Riedel, T. P., Zhang, Z., Gold, A., Surratt, J. D., and Thornton, J. A.: Reactive Uptake of an Isoprene-Derived Epoxydiol to Submicron Aerosol Particles, Environ. Sci. Technol., 48, 11178–11186, https://doi.org/10.1021/es5034266, 2014.
Ge, X., Shaw, S. L., and Zhang, Q.: Toward Understanding Amines and Their Degradation Products from Postcombustion CO2 Capture Processes with Aerosol Mass Spectrometry, Environ. Sci. Technol., 48, 5066–5075, https://doi.org/10.1021/es4056966, 2014.
Gelaro, R., McCarty, W., Suárez, M. J., Todling, R., Molod, A., Takacs, L., Randles, C. A., Darmenov, A., Bosilovich, M. G., Reichle, R., Wargan, K., Coy, L., Cullather, R., Draper, C., Akella, S., Buchard, V., Conaty, A., da Silva, A. M., Gu, W., Kim, G.-K., Koster, R., Lucchesi, R., Merkova, D., Nielsen, J. E., Partyka, G., Pawson, S., Putman, W., Rienecker, M., Schubert, S. D., Sienkiewicz, M., and Zhao, B.: The Modern-Era Retrospective Analysis for Research and Applications, Version 2 (MERRA-2), J. Climate, 30, 5419–5454, https://doi.org/10.1175/JCLI-D-16-0758.1, 2017.
Gibb, S. W., Mantoura, R. F. C., and Liss, P. S.: Ocean-atmosphere exchange and atmospheric speciation of ammonia and methylamines in the region of the NW Arabian Sea, Global Biogeochem. Cy., 13, 161–178, https://doi.org/10.1029/98gb00743, 1999.
Giglio, L., Randerson, J. T., and van der Werf, G. R.: Analysis of daily, monthly, and annual burned area using the fourth-generation global fire emissions database (GFED4), J. Geophys. Res.-Biogeosci., 118, 317–328, 2013.
Guo, H., Xu, L., Bougiatioti, A., Cerully, K. M., Capps, S. L., Hite Jr., J. R., Carlton, A. G., Lee, S.-H., Bergin, M. H., Ng, N. L., Nenes, A., and Weber, R. J.: Fine-particle water and pH in the southeastern United States, Atmos. Chem. Phys., 15, 5211–5228, https://doi.org/10.5194/acp-15-5211-2015, 2015.
Guo, H., Sullivan, A. P., Campuzano-Jost, P., Schroder, J. C., Lopez-Hilfiker, F. D., Dibb, J. E., Jimenez, J. L., Thornton, J. A., Brown, S. S., Nenes, A. and Others: Fine particle pH and the partitioning of nitric acid during winter in the northeastern United States, J. Geophys. Res.-Atmos., 121, 10355–10376, 2016.
Guo, H., Liu, J., Froyd, K. D., Roberts, J. M., Veres, P. R., Hayes, P. L., Jimenez, J. L., Nenes, A., and Weber, R. J.: Fine particle pH and gas–particle phase partitioning of inorganic species in Pasadena, California, during the 2010 CalNex campaign, Atmos. Chem. Phys., 17, 5703–5719, https://doi.org/10.5194/acp-17-5703-2017, 2017.
Guo, H., Campuzano-Jost, P., Nault, B. A., Day, D. A., Schroder, J. C., Dibb, J. E., Dollner, M., Weinzierl, B., and Jimenez, J. L.: The Importance of Size Ranges in Aerosol Instrument Intercomparisons: A Case Study for the ATom Mission, Atmos. Meas. Tech. Discuss. [preprint], https://doi.org/10.5194/amt-2020-224, in review, 2020.
Hall, F. G., Huemmrich, K. F., Strebel, D. E., Goetz, S. J., Nickeson, J. E., and Woods, K. D.: NWS Daily Climatology Data: 1972 (SNF), ORNL DAAC, Oak Ridge, Tennessee, USA, https://doi.org/10.3334/ORNLDAAC/158, 1996.
Hennigan, C. J., Sullivan, A. P., Fountoukis, C. I., Nenes, A., Hecobian, A., Vargas, O., Peltier, R. E., Case Hanks, A. T., Huey, L. G., Lefer, B. L., Russell, A. G., and Weber, R. J.: On the volatility and production mechanisms of newly formed nitrate and water soluble organic aerosol in Mexico City, Atmos. Chem. Phys., 8, 3761–3768, https://doi.org/10.5194/acp-8-3761-2008, 2008.
Hennigan, C. J., Izumi, J., Sullivan, A. P., Weber, R. J., and Nenes, A.: A critical evaluation of proxy methods used to estimate the acidity of atmospheric particles, Atmos. Chem. Phys., 15, 2775–2790, https://doi.org/10.5194/acp-15-2775-2015, 2015.
Hodshire, A. L., Campuzano-Jost, P., Kodros, J. K., Croft, B., Nault, B. A., Schroder, J. C., Jimenez, J. L., and Pierce, J. R.: The potential role of methanesulfonic acid (MSA) in aerosol formation and growth and the associated radiative forcings, Atmos. Chem. Phys., 19, 3137–3160, https://doi.org/10.5194/acp-19-3137-2019, 2019.
Hodzic, A., Campuzano-Jost, P., Bian, H., Chin, M., Colarco, P. R., Day, D. A., Froyd, K. D., Heinold, B., Jo, D. S., Katich, J. M., Kodros, J. K., Nault, B. A., Pierce, J. R., Ray, E., Schacht, J., Schill, G. P., Schroder, J. C., Schwarz, J. P., Sueper, D. T., Tegen, I., Tilmes, S., Tsigaridis, K., Yu, P., and Jimenez, J. L.: Characterization of organic aerosol across the global remote troposphere: a comparison of ATom measurements and global chemistry models, Atmos. Chem. Phys., 20, 4607–4635, https://doi.org/10.5194/acp-20-4607-2020, 2020.
Hoesly, R. M., Smith, S. J., Feng, L., Klimont, Z., Janssens-Maenhout, G., Pitkanen, T., Seibert, J. J., Vu, L., Andres, R. J., Bolt, R. M., Bond, T. C., Dawidowski, L., Kholod, N., Kurokawa, J.-I., Li, M., Liu, L., Lu, Z., Moura, M. C. P., O'Rourke, P. R., and Zhang, Q.: Historical (1750–2014) anthropogenic emissions of reactive gases and aerosols from the Community Emissions Data System (CEDS), Geosci. Model Dev., 11, 369–408, https://doi.org/10.5194/gmd-11-369-2018, 2018.
Hu, W., Campuzano-Jost, P., Day, D. A., Croteau, P., Canagaratna, M. R., Jayne, J. T., Worsnop, D. R., and Jimenez, J. L.: Evaluation of the new capture vaporizer for aerosol mass spectrometers (AMS) through field studies of inorganic species, Aerosol Sci. Technol., 51, 735–754, https://doi.org/10.1080/02786826.2017.1296104, 2017a.
Hu, W., Campuzano-Jost, P., Day, D. A., Croteau, P., Canagaratna, M. R., Jayne, J. T., Worsnop, D. R., and Jimenez, J. L.: Evaluation of the new capture vapourizer for aerosol mass spectrometers (AMS) through laboratory studies of inorganic species, Atmos. Meas. Tech., 10, 2897–2921, https://doi.org/10.5194/amt-10-2897-2017, 2017b.
Hu, W., Campuzano-Jost, P., Day, D. A., Nault, B., Park, T., Lee, T., Pajunoja, A., Virtanen, A., Croteau, P. L., Canagaratna, M. R., Jayne, J. T., Worsnop, D. R., and Jimenez, J. L.: Ambient quantification and size distributions for organic aerosol (OA) in aerosol mass spectrometer (AMS) instruments with the new capture vaporizer (CV), ACS Earth Space Chem., 4, 676–689, https://doi.org/10.1021/acsearthspacechem.9b00310, 2020.
Hu, W. W., Campuzano-Jost, P., Palm, B. B., Day, D. A., Ortega, A. M., Hayes, P. L., Krechmer, J. E., Chen, Q., Kuwata, M., Liu, Y. J., de Sá, S. S., McKinney, K., Martin, S. T., Hu, M., Budisulistiorini, S. H., Riva, M., Surratt, J. D., St. Clair, J. M., Isaacman-Van Wertz, G., Yee, L. D., Goldstein, A. H., Carbone, S., Brito, J., Artaxo, P., de Gouw, J. A., Koss, A., Wisthaler, A., Mikoviny, T., Karl, T., Kaser, L., Jud, W., Hansel, A., Docherty, K. S., Alexander, M. L., Robinson, N. H., Coe, H., Allan, J. D., Canagaratna, M. R., Paulot, F., and Jimenez, J. L.: Characterization of a real-time tracer for isoprene epoxydiols-derived secondary organic aerosol (IEPOX-SOA) from aerosol mass spectrometer measurements, Atmos. Chem. Phys., 15, 11807–11833, https://doi.org/10.5194/acp-15-11807-2015, 2015.
Huang, S., Poulain, L., van Pinxteren, D., van Pinxteren, M., Wu, Z., Herrmann, H., and Wiedensohler, A.: Latitudinal and Seasonal Distribution of Particulate MSA over the Atlantic using a Validated Quantification Method with HR-ToF-AMS, Environ. Sci. Technol., 51, 418–426, https://doi.org/10.1021/acs.est.6b03186, 2017.
Huffman, J. A., Jayne, J. T., Drewnick, F., Aiken, A. C., Onasch, T., Worsnop, D. R., and Jimenez, J. L.: Design, Modeling, Optimization, and Experimental Tests of a Particle Beam Width Probe for the Aerodyne Aerosol Mass Spectrometer, Aerosol Sci. Technol., 39, 1143–1163, https://doi.org/10.1080/02786820500423782, 2005.
IPCC: IPCC 2013: Climate Change 2013: The Physical Science Basis. Contribution of Working Group I to the Fifth Assessment Report of the Intergovernmental Panel on Climate Change, edited by: Stocker, T. F., Qin, D., Plattner, G. K., Tignor, M., Allen, S. K., Bex, V., and Midgley, P. M., Cambridge University Press, Cambridge, UK and New York, NY, USA, 2013.
Jaeglé, L., Shah, V., Thornton, J. A., Lopez-Hilfiker, F. D., Lee, B. H., McDuffie, E. E., Fibiger, D., Brown, S. S., Veres, P., Sparks, T., Ebben, C., Wooldridge, P. J., Kenagy, H. S., Cohen, R. C., Weinheimer, A. J., Campos, T. L., Montzka, D. D., Digangi, J. P., Wolfe, G. M., Hanisco, T., Schroder, J. C., Campuzano-Jost, P., Day, D. A., Jimenez, J. L., Sullivan, A. P., Guo, H., and Weber, R. J.: Nitrogen Oxides Emissions, Chemistry, Deposition, and Export Over the Northeast United States During the WINTER Aircraft Campaign, J. Geophys. Res.-Atmos., 123, 12368–12393, https://doi.org/10.1029/2018JD029133, 2018.
Jang, M., Czoschke, N. M., Lee, S., and Kamens, R. M.: Heterogeneous atmospheric aerosol production by acid-catalyzed particle-phase reactions, Science, 298, 814–817, https://doi.org/10.1126/science.1075798, 2002.
Jayne, J. T., Leard, D. C., Zhang, X., Davidovits, P., Smith, K. A., Kolb, C. E., and Worsnop, D. R.: Development of an Aerosol Mass Spectrometer for Size and Composition Analysis of Submicron Particles, Aerosol Sci. Technol., 33, 49–70, https://doi.org/10.1080/027868200410840, 2000.
Jimenez, J. L., Jayne, J. T., Shi, Q., Kolb, C. E., Worsnop, D. R., Yourshaw, I., Seinfeld, J. H., Flagan, R. C., Zhang, X. F., Smith, K. A., Morris, J. W., and Davidovits, P.: Ambient aerosol sampling using the Aerodyne Aerosol Mass Spectrometer, J. Geophys. Res., 108, 8425–8425, https://doi.org/10.1029/2001JD001213, 2003.
Jimenez, J. L., Canagaratna, M. R., Donahue, N. M., Prevot, A. S. H., Zhang, Q., Kroll, J. H., DeCarlo, P. F., Allan, J. D., Coe, H., Ng, N. L., Aiken, A. C., Docherty, K. S., Ulbrich, I. M., Grieshop, A. P., Robinson, A. L., Duplissy, J., Smith, J. D., Wilson, K. R., Lanz, V. A., Hueglin, C., Sun, Y. L., Tian, J., Laaksonen, A., Raatikainen, T., Rautiainen, J., Vaattovaara, P., Ehn, M., Kulmala, M., Tomlinson, J. M., Collins, D. R., Cubison, M. J., Dunlea, E. J., Huffman, J. A., Onasch, T. B., Alfarra, M. R., Williams, P. I., Bower, K., Kondo, Y., Schneider, J., Drewnick, F., Borrmann, S., Weimer, S., Demerjian, K., Salcedo, D., Cottrell, L., Griffin, R., Takami, A., Miyoshi, T., Hatakeyama, S., Shimono, A., Sun, J. Y., Zhang, Y. M., Dzepina, K., Kimmel, J. R., Sueper, D., Jayne, J. T., Herndon, S. C., Trimborn, A. M., Williams, L. R., Wood, E. C., Middlebrook, A. M., Kolb, C. E., Baltensperger, U., and Worsnop, D. R.: Evolution of organic aerosols in the atmosphere, Science, 326, 1525–1529, https://doi.org/10.1126/science.1180353, 2009.
Jo, D. S., Hodzic, A., Emmons, L. K., Marais, E. A., Peng, Z., Nault, B. A., Hu, W., Campuzano-Jost, P., and Jimenez, J. L.: A simplified parameterization of isoprene-epoxydiol-derived secondary organic aerosol (IEPOX-SOA) for global chemistry and climate models: a case study with GEOS-Chem v11-02-rc, Geosci. Model Dev., 12, 2983–3000, https://doi.org/10.5194/gmd-12-2983-2019, 2019.
Johnson, K. S., Laskin, A., Jimenez, J. L., Shutthanandan, V., Molina, L. T., Salcedo, D., Dzepina, K., and Molina, M. J.: Comparative analysis of urban atmospheric aerosol by particle-induced X-ray emission (PIXE), proton elastic scattering analysis (PESA), and aerosol mass spectrometry (AMS), Environ. Sci. Technol., 42, 6619–6624, https://doi.org/10.1021/es800393e, 2008.
Kang, H., Day, D. A., Krechmer, J. E., Ayres, B. R., Keehan, N. I., Thompson, S. L., Hu, W., Campuzano-Jost, P., Schroder, J. C., Stark, H., Ranney, A., Ziemann, P., Zarzana, K. J., Wild, R. J., Dubé, W., Brown, S. S., Fry, J., and Jimenez, J. L.: A33E-0280: Secondary organic aerosol mass yields from the dark NO3 oxidation of α-pinene and-carene: effect of RO2 radical fate, American Geophysical Union Fall Meeting, 12–16 December 2016, San Francisco, CA, USA, 2016.
Keene, W. C.: Variation of marine aerosol acidity with particle size, Geophys. Res. Lett., 29, 5-1–5-4, https://doi.org/10.1029/2001GL013881, 2002.
KORUS-AQ Science Team: KORUS-AQ data, NASA, https://doi.org/10.5067/Suborbital/KORUSAQ/DATA01, 2016.
Kuwata, M., Zorn, S. R., and Martin, S. T.: Using elemental ratios to predict the density of organic material composed of carbon, hydrogen, and oxygen, Environ. Sci. Technol., 46, 787–794, https://doi.org/10.1021/es202525q, 2012.
Lambert, J. B., Gronert, S., Shurvell, H. F., and Lightner, D. A.: Organic structural spectroscopy, 2nd Edn., edited by: Lambert, J. B., Pearson College Division, New York City, NY, USA, 1998.
Lee, B. H., Lopez-Hilfiker, F. D., Mohr, C., Kurtén, T., Worsnop, D. R., and Thornton, J. A.: An iodide-adduct high-resolution time-of-flight chemical-ionization mass spectrometer: application to atmospheric inorganic and organic compounds, Environ. Sci. Technol., 48, 6309–6317, https://doi.org/10.1021/es500362a, 2014.
Lee, B. H., Lopez-Hilfiker, F. D., Veres, P. R., McDuffie, E. E., Fibiger, D. L., Sparks, T. L., Ebben, C. J., Green, J. R., Schroder, J. C., Campuzano-Jost, P., and Others: Flight deployment of a high-resolution time-of-flight chemical ionization mass spectrometer: Observations of reactive halogen and nitrogen oxide species, J. Geophys. Res.-Atmos., 123, 7670–7686, 2018.
Lee, T., Sullivan, A. P., Mack, L., Jimenez, J. L., Kreidenweis, S. M., Onasch, T. B., Worsnop, D. R., Malm, W., Wold, C. E., Hao, W. M., and Collett, J. L.: Chemical Smoke Marker Emissions During Flaming and Smoldering Phases of Laboratory Open Burning of Wildland Fuels, Aerosol Sci. Technol., 44, i–v, https://doi.org/10.1080/02786826.2010.499884, 2010.
Li, G., Bei, N., Cao, J., Huang, R., Wu, J., Feng, T., Wang, Y., Liu, S., Zhang, Q., Tie, X., and Molina, L. T.: A possible pathway for rapid growth of sulfate during haze days in China, Atmos. Chem. Phys., 17, 3301–3316, https://doi.org/10.5194/acp-17-3301-2017, 2017.
Liao, J., Froyd, K. D., Murphy, D. M., Keutsch, F. N., Yu, G., Wennberg, P. O., St. Clair, J. M., Crounse, J. D., Wisthaler, A., Mikoviny, T., Jimenez, J. L., Campuzano‐Jost, P., Day, D. A., Hu, W., Ryerson, T. B., Pollack, I. B., Peischl, J., Anderson, B. E., Ziemba, L. D., Blake, D. R., Meinardi, S., and Diskin, G.: Airborne measurements of organosulfates over the continental US, J. Geophys. Res.-Atmos., 120, 2990–3005, 2015
Lighty, J. S., Veranth, J. M., and Sarofim, A. F.: Combustion aerosols: factors governing their size and composition and implications to human health, JAPCA J. Air Waste Ma., 50, 1565–618, 2000.
Liu, P., Ziemann, P. J., Kittelson, D. B., and McMurry, P. H.: Generating Particle Beams of Controlled Dimensions and Divergence: II. Experimental Evaluation of Particle Motion in Aerodynamic Lenses and Nozzle Expansions, Aerosol Sci. Technol., 22, 314–324, https://doi.org/10.1080/02786829408959749, 1995.
Liu, X., Day, D. A., Krechmer, J. E., Brown, W., Peng, Z., Ziemann, P. J., and Jimenez, J. L.: Direct measurements of semi-volatile organic compound dynamics show near-unity mass accommodation coefficients for diverse aerosols, Commun. Chem., 2, 98, https://doi.org/10.1038/s42004-019-0200-x, 2019.
Lohmann, U., Broekhuizen, K., Leaitch, R., Shantz, N., and Abbatt, J.: How efficient is cloud droplet formation of organic aerosols?, Geophys. Res. Lett., 31, L05108, https://doi.org/10.1029/2003GL018999, 2004.
Losey, D. J., Ott, E.-J. E., and Freedman, M. A.: Effects of High Acidity on Phase Transitions of an Organic Aerosol, J. Phys. Chem. A, 122, 3819–3828, https://doi.org/10.1021/acs.jpca.8b00399, 2018.
Massucci, M., Clegg, S. L., and Brimblecombe, P.: Equilibrium Partial Pressures, Thermodynamic Properties of Aqueous and Solid Phases, and Cl2 Production from Aqueous HCl and HNO3 and Their Mixtures, J. Phys. Chem. A, 103, 4209–4226, https://doi.org/10.1021/jp9847179, 1999.
Matthew, B. M., Middlebrook, A. M., and Onasch, T. B.: Collection Efficiencies in an Aerodyne Aerosol Mass Spectrometer as a Function of Particle Phase for Laboratory Generated Aerosols, Aerosol Sci. Technol., 42, 884–898, https://doi.org/10.1080/02786820802356797, 2008.
Meskhidze, N., Chameides, W. L., Nenes, A., and Chen, G.: Iron mobilization in mineral dust: Can anthropogenic SO2 emissions affect ocean productivity?, Geophys. Res. Lett., 30, 2085, https://doi.org/10.1029/2003GL018035, 2003.
Middlebrook, A. M., Bahreini, R., Jimenez, J. L., and Canagaratna, M. R.: Evaluation of Composition-Dependent Collection Efficiencies for the Aerodyne Aerosol Mass Spectrometer using Field Data, Aerosol Sci. Technol., 46, 258–271, https://doi.org/10.1080/02786826.2011.620041, 2012.
Müller, C., Iinuma, Y., Karstensen, J., van Pinxteren, D., Lehmann, S., Gnauk, T., and Herrmann, H.: Seasonal variation of aliphatic amines in marine sub-micrometer particles at the Cape Verde islands, Atmos. Chem. Phys., 9, 9587–9597, https://doi.org/10.5194/acp-9-9587-2009, 2009.
Murphy, D. M. and Koop, T.: Review of the vapour pressures of ice and supercooled water for atmospheric applications, Quart. J. Roy. Meteor. Soc., 131), 1539–1565, https://doi.org/10.1256/qj.04.94, 2005.
Murphy, S. M., Sorooshian, A., Kroll, J. H., Ng, N. L., Chhabra, P., Tong, C., Surratt, J. D., Knipping, E., Flagan, R. C., and Seinfeld, J. H.: Secondary aerosol formation from atmospheric reactions of aliphatic amines, Atmos. Chem. Phys., 7, 2313–2337, https://doi.org/10.5194/acp-7-2313-2007, 2007.
Nault, B. A., Campuzano-Jost, P., Day, D. A., Schroder, J. C., Anderson, B., Beyersdorf, A. J., Blake, D. R., Brune, W. H., Choi, Y., Corr, C. A., de Gouw, J. A., Dibb, J., DiGangi, J. P., Diskin, G. S., Fried, A., Huey, L. G., Kim, M. J., Knote, C. J., Lamb, K. D., Lee, T., Park, T., Pusede, S. E., Scheuer, E., Thornhill, K. L., Woo, J.-H., and Jimenez, J. L.: Secondary organic aerosol production from local emissions dominates the organic aerosol budget over Seoul, South Korea, during KORUS-AQ, Atmos. Chem. Phys., 18, 17769–17800, https://doi.org/10.5194/acp-18-17769-2018, 2018.
Nault, B. A., Campuzano-Jost, P., Jo, D., Day, D., Bahreini, R., Bian, H., Chin, M., Clegg, S., Colarco, P., Kodros, J., Lopez-Hilfiker, F., Marais, E., Middlebrook, A., Neuman, A., Nowak, J., Pierce, J., Thornton, J., Tsigaridis, K., and Jimenez, J. and the ATom Science Team: Global Survey of Aerosol Acidity from Polluted to Remote Locations: Measurements and Comparisons with Global Models, EGU General Assembly 2020, Online, 4–8 May 2020, EGU2020-11366, https://doi.org/10.5194/egusphere-egu2020-11366, 2020.
Nault, B. A., Campuzano-Jost, P., Day, D. A., Jo, D. S., Schroder, J. S., Allen, H. M., Bahreini, R., Bian, H., Blake, D. R., Chin, M., Clegg, S. L., Colarco, P. R., Crounse, J. D., Cubison, M. J., DeCarlo, P. F., Dibb, J. E., Diskin, G. S., Hodzic, A., Hu, W., Katich, J., Kim, M. J., Kodros, J. K., Kupc, A., Lopez-Hilfiker, F. D., Marais, E. A., Middlebrook, A. M., Neuman, J. A., Nowak, J. B., Palm, B. B., Paulot, F., Pierce, J. R., Schill, G. P., Scheuer, E., Thornton, J. A., Tsigaridis, K., Wennberg, P. O., Williamson, C. J., and Jimenez, J. L.: Models underestimate the increase of acidity with remoteness biasing radiative impact calculations, Commun. Earth Environ. submitted, 2021.
Nenes, A., Pandis, S. N., and Pilinis, C.: Continued development and testing of a new thermodynamic aerosol module for urban and regional air quality models, Atmos. Environ., 33, 1553–1560, https://doi.org/10.1016/S1352-2310(98)00352-5, 1999.
Ng, N. L., Herndon, S. C., Trimborn, A., Canagaratna, M. R., Croteau, P. L., Onasch, T. B., Sueper, D., Worsnop, D. R., Zhang, Q., Sun, Y. L., and Jayne, J. T.: An Aerosol Chemical Speciation Monitor (ACSM) for Routine Monitoring of the Composition and Mass Concentrations of Ambient Aerosol, Aerosol Sci. Technol., 45, 780–794, https://doi.org/10.1080/02786826.2011.560211, 2011a.
Ng, N. L., Canagaratna, M. R., Jimenez, J. L., Chhabra, P. S., Seinfeld, J. H., and Worsnop, D. R.: Changes in organic aerosol composition with aging inferred from aerosol mass spectra, Atmos. Chem. Phys., 11, 6465–6474, https://doi.org/10.5194/acp-11-6465-2011, 2011b.
Ovadnevaite, J., Ceburnis, D., Canagaratna, M., Berresheim, H., Bialek, J., Martucci, G., Worsnop, D. R. and O'Dowd, C.: On the effect of wind speed on submicron sea salt mass concentrations and source fluxes, J. Geophys. Res.-Atmos., 117, D16201, https://doi.org/10.1029/2011JD017379, 2012.
Paulot, F., Jacob, D. J., Johnson, M. T., Bell, T. G., Baker, A. R., Keene, W. C., Lima, I. D., Doney, S. C., and Stock, C. A.: Global oceanic emission of ammonia: Constraints from seawater and atmospheric observations, Global Biogeochem. Cy., 29, 1165–1178, https://doi.org/10.1002/2015GB005106, 2015.
Phinney, L., Richard Leaitch, W., Lohmann, U., Boudries, H., Worsnop, D. R., Jayne, J. T., Toom-Sauntry, D., Wadleigh, M., Sharma, S., and Shantz, N.: Characterization of the aerosol over the sub-arctic north east Pacific Ocean, Deep Sea Res. Pt. 2, 53, 2410–2433, https://doi.org/10.1016/j.dsr2.2006.05.044, 2006.
Pye, H. O. T., Nenes, A., Alexander, B., Ault, A. P., Barth, M. C., Clegg, S. L., Collett Jr., J. L., Fahey, K. M., Hennigan, C. J., Herrmann, H., Kanakidou, M., Kelly, J. T., Ku, I.-T., McNeill, V. F., Riemer, N., Schaefer, T., Shi, G., Tilgner, A., Walker, J. T., Wang, T., Weber, R., Xing, J., Zaveri, R. A., and Zuend, A.: The acidity of atmospheric particles and clouds, Atmos. Chem. Phys., 20, 4809–4888, https://doi.org/10.5194/acp-20-4809-2020, 2020.
Quinn, P. K., Charlson, R. J., and Bates, T. S.: Simultaneous observations of ammonia in the atmosphere and ocean, Nature, 335, 336–338, https://doi.org/10.1038/335336a0, 1988.
Quinn, P. K., Bates, T. S., Coffman, D., Onasch, T. B., Worsnop, D., Baynard, T., De Gouw, J. A., Goldan, P. D., Kuster, W. C., Williams, E., and Others: Impacts of sources and aging on submicrometer aerosol properties in the marine boundary layer across the Gulf of Maine, J. Geophys. Res.-Atmos., 111, D23S36, https://doi.org/10.1029/2006JD007582, 2006.
Raizenne, M., Neas, L. M., Damokosh, A. I., Dockery, D. W., Spengler, J. D., Koutrakis, P., Ware, J. H., and Speizer, F. E.: Health effects of acid aerosols on North American children: pulmonary function, Environ. Health Perspect., 104, 506–514, https://doi.org/10.1289/ehp.96104506, 1996.
Rindelaub, J. D., Craig, R. L., Nandy, L., Bondy, A. L., Dutcher, C. S., Shepson, P. B., and Ault, A. P.: Direct Measurement of pH in Individual Particles via Raman Microspectroscopy and Variation in Acidity with Relative Humidity, J. Phys. Chem. A, 120, 911–917, https://doi.org/10.1021/acs.jpca.5b12699, 2016.
Riva, M., Budisulistiorini, S. H., Chen, Y., Zhang, Z., D'Ambro, E. L., Zhang, X., Gold, A., Turpin, B. J., Thornton, J. A., Canagaratna, M. R., and Surratt, J. D.: Chemical Characterization of Secondary Organic Aerosol from Oxidation of Isoprene Hydroxyhydroperoxides, Environ. Sci. Technol., 50, 9889–9899, https://doi.org/10.1021/acs.est.6b02511, 2016.
Riva, M., Chen, Y., Zhang, Y., Lei, Z., Olson, N. E., Boyer, H. C., Narayan, S., Yee, L. D., Green, H. S., Cui, T., Zhang, Z., Baumann, K., Fort, M., Edgerton, E., Budisulistiorini, S. H., Rose, C. A., Ribeiro, I. O., E Oliveira, R. L., Dos Santos, E. O., Machado, C. M. D., Szopa, S., Zhao, Y., Alves, E. G., de Sá, S. S., Hu, W., Knipping, E. M., Shaw, S. L., Duvoisin Junior, S., de Souza, R. A. F., Palm, B. B., Jimenez, J.-L., Glasius, M., Goldstein, A. H., Pye, H. O. T., Gold, A., Turpin, B. J., Vizuete, W., Martin, S. T., Thornton, J. A., Dutcher, C. S., Ault, A. P., and Surratt, J. D.: Increasing Isoprene Epoxydiol-to-Inorganic Sulfate Aerosol Ratio Results in Extensive Conversion of Inorganic Sulfate to Organosulfur Forms: Implications for Aerosol Physicochemical Properties, Environ. Sci. Technol., 53, 8682–8694, https://doi.org/10.1021/acs.est.9b01019, 2019.
Salcedo, D., Onasch, T. B., Aiken, A. C., Williams, L. R., de Foy, B., Cubison, M. J., Worsnop, D. R., Molina, L. T., and Jimenez, J. L.: Determination of particulate lead using aerosol mass spectrometry: MILAGRO/MCMA-2006 observations, Atmos. Chem. Phys., 10, 5371–5389, https://doi.org/10.5194/acp-10-5371-2010, 2010.
Schindler, D. W.: Effects of Acid rain on freshwater ecosystems, Science, 239, 149–157, https://doi.org/10.1126/science.239.4836.149, 1988.
Schroder, J. C., Campuzano-Jost, P., Day, D. A., Shah, V., Larson, K., Sommers, J. M., Sullivan, A. P., Campos, T., Reeves, J. M., Hills, A., Hornbrook, R. S., Blake, N. J., Scheuer, E., Guo, H., Fibiger, D. L., McDuffie, E. E., Hayes, P. L., Weber, R. J., Dibb, J. E., Apel, E. C., Jaeglé, L., Brown, S. S., Thornton, J. A., and Jimenez, J. L.: Sources and Secondary Production of Organic Aerosols in the Northeastern United States during WINTER, J. Geophys. Res.-Atmos., 42, 4478, https://doi.org/10.1029/2018JD028475, 2018.
SEAC4RS Science Team: SEAC4RS Data, NASA, https://doi.org/10.5067/Aircraft/SEAC4RS/Aerosol-TraceGas-Cloud, 2013.
Seinfeld, J. H. and Pandis, S. N.: Atmospheric Chemistry and Physics, 2nd edn., John Wiley & Sons, Inc., New York, USA, 2006.
Song, S., Gao, M., Xu, W., Shao, J., Shi, G., Wang, S., Wang, Y., Sun, Y., and McElroy, M. B.: Fine-particle pH for Beijing winter haze as inferred from different thermodynamic equilibrium models, Atmos. Chem. Phys., 18, 7423–7438, https://doi.org/10.5194/acp-18-7423-2018, 2018.
Song, S., Gao, M., Xu, W., Sun, Y., Worsnop, D. R., Jayne, J. T., Zhang, Y., Zhu, L., Li, M., Zhou, Z., Cheng, C., Lv, Y., Wang, Y., Peng, W., Xu, X., Lin, N., Wang, Y., Wang, S., Munger, J. W., Jacob, D. J., and McElroy, M. B.: Possible heterogeneous chemistry of hydroxymethanesulfonate (HMS) in northern China winter haze, Atmos. Chem. Phys., 19, 1357–1371, https://doi.org/10.5194/acp-19-1357-2019, 2019.
Sorooshian, A., Padró, L. T., Nenes, A., Feingold, G., McComiskey, A., Hersey, S. P., Gates, H., Jonsson, H. H., Miller, S. D., Stephens, G. L., Flagan, R. C., and Seinfeld, J. H.: On the link between ocean biota emissions, aerosol, and maritime clouds: Airborne, ground, and satellite measurements off the coast of California, Global Biogeochem. Cy., 23, GB4007, https://doi.org/10.1029/2009GB003464, 2009.
Sorooshian, A., Crosbie, E., Maudlin, L. C., Youn, J.-S., Wang, Z., Shingler, T., Ortega, A. M., Hersey, S.m and Woods, R. K.: Surface and airborne measurements of organosulfur and methanesulfonate over the western United States and coastal areas, J. Geophys. Res.-Atmos., 120, 8535–8548, https://doi.org/10.1002/2015JD023822, 2015.
Stith, J. L., Ramanathan, V., Cooper, W. A., Roberts, G. C., DeMott, P. J., Carmichael, G., Hatch, C. D., Adhikary, B., Twohy, C. H., Rogers, D. C., Baumgardner, D., Prenni, A. J., Campos, T., Gao, R., Anderson, J.m and Feng, Y.: An overview of aircraft observations from the Pacific Dust Experiment campaign, J. Geophys. Res., 114, D05207, https://doi.org/10.1029/2008JD010924, 2009.
Sueper, D.: ToF-AMS Data Analysis Software Webpage, available at: http://cires1.colorado.edu/jimenez-group/wiki/index.php/ToF-AMS_Analysis_Software (last access: 5 May 2018), 2018.
Surratt, J. D., Kroll, J. H., Kleindienst, T. E., Edney, E. O., Claeys, M., Sorooshian, A., Ng, N. L., Offenberg, J. H., Lewandowski, M., Jaoui, M., Flagan, R. C., and Seinfeld, J. H.: Evidence for organosulfates in secondary organic aerosol, Environ. Sci. Technol., 41, 517–527, https://doi.org/10.1021/es062081q, 2007.
Surratt, J. D., Gómez-González, Y., Chan, A. W. H., Vermeylen, R., Shahgholi, M., Kleindienst, T. E., Edney, E. O., Offenberg, J. H., Lewandowski, M., Jaoui, M., Maenhaut, W., Claeys, M., Flagan, R. C., and Seinfeld, J. H.: Organosulfate formation in biogenic secondary organic aerosol, J. Phys. Chem. A, 112, 8345–8378, https://doi.org/10.1021/jp802310p, 2008.
The International GEOS-Chem User Community: geoschem/geos-chem: GEOS-Chem 12.6.1 (Version 12.6.1), Zenodo, https://doi.org/10.5281/zenodo.3520966, 2019.
Thornton, J. A., Jaeglé, L., and McNeill, V. F.: Assessing known pathways for HO2 loss in aqueous atmospheric aerosols: Regional and global impacts on tropospheric oxidants, J. Geophys. Res.-Atmos., 113, D05303, https://doi.org/10.1029/2007JD009236, 2008.
Tolocka, M. P. and Turpin, B.: Contribution of organosulfur compounds to organic aerosol mass, Environ. Sci. Technol., 46, 7978–7983, https://doi.org/10.1021/es300651v, 2012.
Toon, O. B., Maring, H., Dibb, J., Ferrare, R., Jacob, D. J., Jensen, E. J., Luo, Z. J., Mace, G. G., Pan, L. L., Pfister, L. and Others: Planning, implementation, and scientific goals of the Studies of Emissions and Atmospheric Composition, Clouds and Climate Coupling by Regional Surveys (SEAC4RS) field mission, J. Geophys. Res.-Atmos., 121 4967–5009, 2016.
van Pinxteren, M., Fiedler, B., van Pinxteren, D., Iinuma, Y., Körtzinger, A., and Herrmann, H.: Chemical characterization of sub-micrometer aerosol particles in the tropical Atlantic Ocean: marine and biomass burning influences, J. Atmos. Chem., 72, 105–125, https://doi.org/10.1007/s10874-015-9307-3, 2015.
Wagner, N. L., Brock, C. A., Angevine, W. M., Beyersdorf, A., Campuzano-Jost, P., Day, D., de Gouw, J. A., Diskin, G. S., Gordon, T. D., Graus, M. G., Holloway, J. S., Huey, G., Jimenez, J. L., Lack, D. A., Liao, J., Liu, X., Markovic, M. Z., Middlebrook, A. M., Mikoviny, T., Peischl, J., Perring, A. E., Richardson, M. S., Ryerson, T. B., Schwarz, J. P., Warneke, C., Welti, A., Wisthaler, A., Ziemba, L. D., and Murphy, D. M.: In situ vertical profiles of aerosol extinction, mass, and composition over the southeast United States during SENEX and SEAC4RS: observations of a modest aerosol enhancement aloft, Atmos. Chem. Phys., 15, 7085–7102, https://doi.org/10.5194/acp-15-7085-2015, 2015.
Wang, Y., Zhang, Q., Jiang, J., Zhou, W., Wang, B., He, K., Duan, F., Zhang, Q., Philip, S., and Xie, Y.: Enhanced sulfate formation during China's severe winter haze episode in January 2013 missing from current models, J. Geophys. Res.-Atmos., 119, 10425–10440, 2014.
Weber, R. J., Guo, H., Russell, A. G., and Nenes, A.: High aerosol acidity despite declining atmospheric sulfate concentrations over the past 15 years, Nat. Geosci., 9, 282, https://doi.org/10.1038/ngeo2665, 2016.
Wexler, A. S. and Clegg, S. L.: Atmospheric aerosol models for systems including the ions H+, NH4+, Na+, SO42−, NO3−, Cl−, Br−, and H2O, J. Geophys. Res.-Atmos., 107, ACH 14-1–ACH 14-14, https://doi.org/10.1029/2001JD000451, 2002.
WINTER Science Team: WINTER data, available at: https://data.eol.ucar.edu/master_lists/generated/winter/ (last access: 27 April 2019), 2015.
Youn, J.-S., Crosbie, E., Maudlin, L. C., Wang, Z., and Sorooshian, A.: Dimethylamine as a major alkyl amine species in particles and cloud water: Observations in semi-arid and coastal regions, Atmos. Environ., 122, 250–258, https://doi.org/10.1016/j.atmosenv.2015.09.061, 2015.
Zhang, Q., Stanier, C. O., Canagaratna, M. R., Jayne, J. T., Worsnop, D. R., Pandis, S. N., and Jimenez, J. L.: Insights into the chemistry of new particle formation and growth events in Pittsburgh based on aerosol mass spectrometry, Environ. Sci. Technol., 38, 4797–4809, https://doi.org/10.1021/es035417u, 2004.
Zhang, Q., Jimenez, J. L., Worsnop, D. R., and Canagaratna, M.: A case study of urban particle acidity and its influence on secondary organic aerosol, Environ. Sci. Technol., 41, 3213–3219, https://doi.org/10.1021/Es061812j, 2007a.
Zhang, Q., Jimenez, J. L., Canagaratna, M. R., Allan, J. D., Coe, H., Ulbrich, I., Alfarra, M. R., Takami, A., Middlebrook, A. M., Sun, Y. L., Dzepina, K., Dunlea, E., Docherty, K., DeCarlo, P. F., Salcedo, D., Onasch, T., Jayne, J. T., Miyoshi, T., Shimono, A., Hatakeyama, S., Takegawa, N., Kondo, Y., Schneider, J., Drewnick, F., Borrmann, S., Weimer, S., Demerjian, K., Williams, P., Bower, K., Bahreini, R., Cottrell, L., Griffin, R. J., Rautiainen, J., Sun, J. Y., Zhang, Y. M., and Worsnop, D. R.: Ubiquity and dominance of oxygenated species in organic aerosols in anthropogenically-influenced Northern Hemisphere midlatitudes, Geophys. Res. Lett., 34, L13801, https://doi.org/10.1029/2007gl029979, 2007.
Zhang, X., Smith, K. A., Worsnop, D. R., Jimenez, J. L., Jayne, J. T., Kolb, C. E., Morris, J., and Davidovits, P.: Numerical Characterization of Particle Beam Collimation: Part II Integrated Aerodynamic-Lens–Nozzle System, Aerosol Sci. Technol., 38, 619–638, https://doi.org/10.1080/02786820490479833, 2004.
Zheng, B., Zhang, Q., Zhang, Y., He, K. B., Wang, K., Zheng, G. J., Duan, F. K., Ma, Y. L., and Kimoto, T.: Heterogeneous chemistry: a mechanism missing in current models to explain secondary inorganic aerosol formation during the January 2013 haze episode in North China, Atmos. Chem. Phys., 15, 2031–2049, https://doi.org/10.5194/acp-15-2031-2015, 2015.
Zheng, Y., Cheng, X., Liao, K., Li, Y., Li, Y. J., Huang, R.-J., Hu, W., Liu, Y., Zhu, T., Chen, S., Zeng, L., Worsnop, D. R., and Chen, Q.: Characterization of anthropogenic organic aerosols by TOF-ACSM with the new capture vaporizer, Atmos. Meas. Tech., 13, 2457–2472, https://doi.org/10.5194/amt-13-2457-2020, 2020.
Zorn, S. R., Drewnick, F., Schott, M., Hoffmann, T., and Borrmann, S.: Characterization of the South Atlantic marine boundary layer aerosol using an aerodyne aerosol mass spectrometer, Atmos. Chem. Phys., 8, 4711–4728, https://doi.org/10.5194/acp-8-4711-2008, 2008.