the Creative Commons Attribution 4.0 License.
the Creative Commons Attribution 4.0 License.
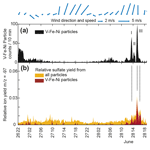
Detection of ship plumes from residual fuel operation in emission control areas using single-particle mass spectrometry
Johannes Passig
Julian Schade
Robert Irsig
Xue Li
Zhen Zhou
Thomas Adam
Ralf Zimmermann
Ships are among the main contributors to global air pollution, with substantial impacts on climate and public health. To improve air quality in densely populated coastal areas and to protect sensitive ecosystems, sulfur emission control areas (SECAs) were established in many regions of the world. Ships in SECAs operate with low-sulfur fuels, typically distillate fractions such as marine gas oil (MGO). Alternatively, exhaust gas-cleaning devices (“scrubbers”) can be implemented to remove SO2 from the exhaust, thus allowing the use of cheap high-sulfur residual fuels. Compliance monitoring is established in harbors but is difficult in open water because of high costs and technical limitations. Here we present the first experiments to detect individual ship plumes from distances of several kilometers by single-particle mass spectrometry (SPMS). In contrast to most monitoring approaches that evaluate the gaseous emissions, such as manned or unmanned surveillance flights, sniffer technologies and remote sensing, we analyze the metal content of individual particles which is conserved during atmospheric transport. We optimized SPMS technology for the evaluation of residual fuel emissions and demonstrate their detection in a SECA. Our experiments show that ships with installed scrubbers can emit PM emissions with health-relevant metals in quantities high enough to be detected from more than 10 km distance, emphasizing the importance of novel exhaust-cleaning technologies and cleaner fuels. Because of the unique and stable signatures, the method is not affected by urban background. With this study, we establish a route towards a novel monitoring protocol for ship emissions. Therefore, we present and discuss mass spectral signatures that indicate the particle age and thus the distance to the source. By matching ship transponder data, measured wind data and air mass back trajectories, we show how real-time SPMS data can be evaluated to assign distant ship passages.
- Article
(5706 KB) - Full-text XML
-
Supplement
(3210 KB) - BibTeX
- EndNote
Among the variety of air pollution sources, ships emit particularly large amounts of sulfur, carbonaceous aerosols and metals with substantial impacts on climate and public health (Corbett et al., 2007; Eyring et al., 2010; Viana et al., 2014; Jonson et al., 2020). Between 60 000 and 400 000 annual deaths by cardiopulmonary diseases and lung cancer as well as 14 million cases of childhood asthma were attributed to ship emissions (Sofiev et al., 2018). Mitigation strategies focus on the sulfur aspect, e.g., by a global 0.5 % standard for ship fuels since 2020 and by implementation of sulfur emission control areas (SECAs, <0.1 % S in fuel mass since 2015) that currently comprise the Baltic Sea, the North Sea, and most of the US and Canadian coast. Legal alternatives to the use of expensive distillate fuels in SECAs include desulfurized “hybrid” blends of low-grade residual fuels (Lähteenmäki-Uutela et al., 2019) or the installation of exhaust-cleaning devices for SO2-like flue gas scrubbers (Winnes et al., 2018; Lehtoranta et al., 2019; Winnes et al., 2020).
Several studies investigated the effect of fuel composition on ship emissions and their respective effects on climate (Lack et al., 2011; Sofiev et al., 2018; Yu et al., 2020; Corbin et al., 2019) and health (Winebrake et al., 2009; Oeder et al., 2015). Beyond the SO2 emissions, the particle-phase pollution is also specifically serious if low-grade heavy fuel oils (bunker fuels) are used (Moldanová et al., 2009; Sippula et al., 2014; Streibel et al., 2017; Di Wu et al., 2018). Within the various health effects of such combustion particles, specific risks of acute cardiovascular effects were associated with water-soluble fractions of particle-bound metals (Ye et al., 2018). Of note, iron solubility is promoted by the presence of sulfur (Fang et al., 2017), a situation that particularly arises for ship emission particles. Implementation of the sulfur regulations substantially decreased the use of residual fuels in SECAs (Jonson et al., 2019; Lähteenmäki-Uutela et al., 2019). However, scrubber operation and combustion of desulfurized residual fuels reduce PM emissions only partially (Fridell and Salo, 2016; Lehtoranta et al., 2019; Winnes et al., 2020), and there is vital interest in assessing the environmental and health effects (Winnes et al., 2018). Furthermore, compliance monitoring and emission inventories would benefit from the ability to distinguish between these options for ship operation.
Compliance monitoring on short distances is typically based on gas-phase measurements of CO2 and SO2 in the plumes of passing ships in harbors or at bridges (Kattner et al., 2015; Mellqvist et al., 2017b; Zhang et al., 2019). However, these places are known to be monitored, and onboard checks also occur frequently (Lähteenmäki-Uutela et al., 2019). Plume analysis in open water requires expensive surveillance flights (Beecken et al., 2014; Van Roy and Scheldemann, 2016). Unmanned aerial vehicles can reduce these costs but have limitations in cruising range, payload and weather conditions (Zhou et al., 2020). Optical sensing technologies that have been utilized to monitor ship plumes on distances of a few kilometers comprise light detection and ranging (lidar), ultraviolet cameras and multi-axis differential optical absorption spectroscopy (MAX-DOAS) (Balzani Lööv et al., 2014; Seyler et al., 2017).
Particles often preserve source-specific chemical signatures while being transported by the wind over large distances. Field studies on particulate matter (PM) from ship plumes can be performed by following ships at some hundreds of meters to a few kilometers' distance (Chen, 2005; Lack et al., 2009; Petzold et al., 2008; Berg et al., 2012). A different approach to characterize ship plumes is by stationary ambient measurements downwind of shipping lanes while recording the ship transponder data (automatic identification system, AIS) (Diesch et al., 2013). Recently, Ausmeel et al. (2019, 2020) measured physical and chemical properties of more than 150 ship plumes in the Baltic Sea from a distance of about 10 km, and Celik et al. (2020) characterized 252 ship plumes in the Mediterranean Sea and around the Arabian Peninsula at distances up to 40 km from a ship-based measurement station. Such methods determine the presence of a ship plume mainly by an increase in particle number and changes in its size distribution or by an increase in a marker substance in the particle ensemble. Dispersion models of ship plumes showed rapid decrease in particle number concentration within the first minutes after emission (Tian et al., 2014; Celik et al., 2020). Therefore, particle number-based methods as well as particle integrating approaches are limited to low background levels, and clear chemical indicators for ship plume presence combined with single-particle information may push the limits of stationary ambient measurements.
Established markers for aerosols from residual fuel combustion are combinations of vanadium, iron and nickel (Celo et al., 2015; Zhang et al., 2014). Single-particle mass spectrometry (SPMS) can detect these particle-bound metals in real time (Pratt and Prather, 2012; Passig and Zimmermann, 2021). Briefly, after optical sizing and introduction into vacuum, particles are exposed to intense UV laser pulses that form a microplasma (laser desorption/ionization, LDI). Both positive and negative ions are analyzed with respect to their mass-to-charge ratio (). Thus, the size and a chemical profile from individual particles are obtained. SPMS studies documented air pollution by regional transport of emissions from harbors and shipping lanes (Reinard et al., 2007; Ault et al., 2009; Arndt et al., 2017; Gaston et al., 2013; Liu et al., 2017; Wang et al., 2019). Also, individual ship plumes were analyzed by in-port studies (Healy et al., 2009; Ault et al., 2010; Xiao et al., 2018), demonstrating that SPMS can distinguish between residual fuel combustion and distillate fuel operation, predominantly by the metal signatures. All these studies were performed outside SECA zones, and, for the individual plume analyses, on short distances to the ships.
With the present study, we apply SPMS with resonant ionization of iron (Passig et al., 2020) for the detection of individual ship plumes from the distance to evaluate residual fuel combustion in SECAs, also for ships equipped with scrubbers. We show that this approach is independent of background air pollution, and we discuss the limits of detection over large distances. By examining indicators for particle ageing and the effects of inaccuracies in wind field determination, we pave the way for future open-sea monitoring of ship plumes using SPMS.
2.1 Single-particle mass spectrometer and sampling
The SPMS instrument was manufactured by Hexin Instruments Ltd., Guangzhou, China, and Photonion GmbH, Schwerin, Germany (Li et al., 2011; Zhou et al., 2016). It consists of a dual-polarity mass spectrometer in Z-TOF geometry (Pratt et al., 2009), an aerodynamic lens inlet and 75 mW continuous-wave lasers (wavelength λ=532 nm), ellipsoidal mirrors and photomultipliers for particle detection and sizing. The instrument is equipped with a KrF-excimer laser (λ=248.3 nm, PhotonEx, Photonion GmbH, Germany). The used wavelength is well-suited for resonance-enhanced laser desorption/ionization (LDI) of iron (Passig et al., 2020). Setting the lens (f=200 mm) to an off-focus position of 7 mm with respect to the particle beam, the spot size was about 150×300 µm and the resulting intensity 5 GW cm−2 at 6 mJ pulse energy. The off-focus position in conjunction with the flat-top profile of the excimer laser beam allows hit rates of around 50 % (particles producing mass spectra vs. optically detected particles) (Schade et al., 2019; Passig et al., 2020).
The setup was housed in the southern periphery of the city of Rostock (population 210 000) contributing urban background aerosols in between the sampling site and the coast. Possible regional aerosol sources comprise the urban area of Rostock, forests in the northeasterly direction and farmland in the surrounding area; see Fig. 1. Ambient air was sampled directly on the roof of the laboratory building (54∘04′41.5′′ N, 12∘06′30.6′′ E), about 35 m above sea level. Because the study focuses on particles from distant sources, the sampling was optimized for larger particles 0.5 µm in size at the expense of efficiency for smaller particles, such as local traffic emissions. Therefore, an aerosol concentrator, originally designed for particles above 2 µm in size, was used (model 4240, MSP Corp., USA) (Romay et al., 2002). The multi-stage virtual impactor of this device concentrates particles from the 300 L min−1 intake airflow into a 1 L min−1 carrier gas stream, from which 0.1 L min−1 were finally guided into the SPMS instrument. The real concentration factor for ambient air particles around 0.5 µm in size was approximately 10:1, as estimated in previous experiments (Passig et al., 2020). Corrections of the inlet efficiency have not been applied.
2.2 Analysis of single-particle mass spectra
Using custom software on the Matlab platform (MathWorks Inc.), mass spectra were computed from time-of-flight data considering peak area within nominal mass resolution. Positive and negative mass spectra including the metal signatures were separately normalized, and missing negative ion spectra were set to zero. We classified the particles using the adaptive resonance theory neural network, ART-2a (Song et al., 1999), extracted from the open-source toolkit FATES (Flexible Analysis Toolkit for the Exploration of SPMS data) (Sultana et al., 2017) with a learning rate of 0.05, a vigilance factor of 0.8 and 20 iterations. Ion peak assignments correspond to the most likely ion at a given mass (). It should be noted that SPMS obtains numbers of particles with particular chemical signatures, not the mass concentration of these components.
2.3 Meteorological and ship transponder data
Air trajectories were calculated using the interactive HYSPLIT web tool from the National Oceanic and Atmospheric Administration model GDAS with 0.5∘ resolution (http://www.ready.noaa.gov/HYSPLIT.php, last access: 24 March 2021) (Stein et al., 2015). Hourly wind data were obtained from the web archive of a local meteorological station that belongs to Germany's National Meteorological Service, 12 km north of the sampling site and close to the harbor exit (https://www.dwd.de/DE/leistungen/klimadatendeutschland/klarchivstunden.html#buehneTop, last access: 12 November 2020). AIS data for all ships sailing between 54∘ N, 11.5∘ E and 55.5∘ N, 16∘ E in the measurement period (26 June–2 July 2018) were acquired from the German Federal Waterways and Shipping Administration in anonymized form, analyzed and filtered by custom software on the Matlab platform.
3.1 Chemical profile of residual fuel emission particles
During 26 June–2 July 2018, a total number of 290 144 particles were detected by the SPMS instrument, and 162 288 particles yielded mass spectra and were sized and chemically analyzed. The ART-2a algorithm produced 715 particle clusters, whereof the top 300 clusters accounting for >90 % of the particles were visually inspected. Similar clusters based on the ion signal of key species were grouped by hand into six general categories. The mass spectra of the six general particle classes can be found in the Supplement, Fig. S1, as well as the discussion on it. Here we focus on the class of ship emission particles.
The combination of signals from the transition metals V, Fe and Ni is a well-documented marker for particles from residual fuel combustion on ships (Healy et al., 2009; Ault et al., 2010; Xiao et al., 2018; Furutani et al., 2011; Reinard et al., 2007); see Fig. 2 for the mass spectrum. The relative peak intensities do not reflect the mass concentration of these species, and further metals such as Zn and Cu are less frequently detected in SPMS, despite their high concentration in the fuels and particles (Viana et al., 2009; Popovicheva et al., 2012; Moldanová et al., 2009; Streibel et al., 2017; Corbin et al., 2018). In previous studies on ship emissions, vanadium signals dominated largely over Fe and Ni peaks (Healy et al., 2009; Ault et al., 2010) and were partly treated as a singular marker (Xiao et al., 2018). Of note, the KrF-excimer laser used in our experiment resonantly ionizes particle-bound Fe, enabling a more efficient and secure detection of iron (Passig et al., 2020). This allows us to strengthen the assignment by counting only particle clusters to the V–Fe–Ni class that show either the complete peak pattern of 51V+, 56Fe+, 58Ni+, 67VO+ or 51V+, 54Fe+, 56Fe+, 67VO+.
Beyond the transition metals from residual fuel combustion, the V–Fe–Ni particles reveal Ca+ ions that can be attributed to additives of lubrication oil (Toner et al., 2006; Spencer et al., 2006), minor signals from elemental carbon (EC) and organic carbon (OC) as well as a particularly intense peak. Considering a strong signal also from other particle classes (compare Fig. S1), the sulfate can be primary and secondary.
Apart from the sea salt, the particles of all classes show comparable sizes, peaking around 400–500 nm; see Fig. S1a. One explanation lies in the setup: the optical detection of particles based on Mie scattering drops rapidly, approaching the Rayleigh limit around 150 nm (for a 532 nm scattering wavelength) (Gaie-Levrel et al., 2012). Furthermore, the aerosol concentrator is optimized for particles of 2.5–10 µm in size (Romay et al., 2002). While detailed data on its performance for small particles are not available, we could estimate an approximately 10-fold concentration for 0.5 µm particles and particle losses of at least 50 % below 0.5 µm in a previous study (Passig et al., 2020). Beyond the instrumental aspect, particles in the accumulation mode can be dominant if local emissions are of minor importance or if they rapidly grow, e.g., by condensation of secondary material (Seinfeld and Pandis, 2016).
3.2 Temporal profile of residual fuel emission particles
The measurements were performed during a period of relatively calm summer weather with light to moderate winds from mostly northern to eastern directions, representing a typical scenario for a northern European coastal region during summer. The mean PM2.5 mass was 4.0 µg cm−3 and the mean particle number density was 44 cm−3 (0.25–32 µm), as measured by a monitoring station near the coastline (Grimm EDM-180, http://www.lung.mv-regierung.de/umwelt/luft/akt_wahl.htm, last access: 25 March 2021). Figure 3a lists the regions passed by the air masses within the last 24 h before arriving at the sampling site, while the back trajectories are shown in Fig. S4. The last hour of air transport before arriving at the site can be determined from the local wind data. Air masses crossing the heavily trafficked ship lanes >20 km north of the coast (see Fig. 1) enter the site, passing the urban area of Rostock. The wind data (Fig. 3b) reveal a pronounced land/onshore circulation, with regular northern winds in the afternoon and light winds from different directions in the night and morning hours. Figure 3c shows time series of the particle numbers within the general particle classes, while Fig. 3d shows their relative contribution to total particle numbers, both with 10 min resolution.
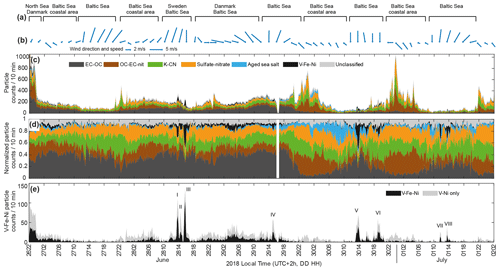
Figure 3(a) Air mass origin (top row: >12 h, bottom row <12 h) according to the HYSPLIT back-trajectory analysis (Fig. S4). (b) Measured wind data from the meteorological station at the harbor exit, 12 km north of the measurement site. (c) The time series of particle counts from the general particle classes shows regional/long-range transported air pollution (26–29 June) and night-time secondary organic aerosol formation (29 June–2 July); see the Supplement for a detailed discussion. (d) The same data as (c) but normalized to total particle counts illustrate the contribution of each particle type. (e) The temporal behavior of V–Fe–Ni particles (black) from residual fuel combustion reveals transient events (ship plumes I–VIII) and smooth background signals, predominantly during onshore winds. Apart from the short events, their contribution to total particle numbers is low. The plumes can also be recognized by evaluating only the presence of 51V+ and 67VO+ (grey area, relative peak area of 51V % and 67VO %), however with some false positive results from interference with fragments, especially during events with high counts of organic aerosols.
In the following, we discuss only the mass spectra and time series of V–Fe–Ni particles; for all other classes we refer to the Supplement. The V–Fe–Ni particles (Fig. 3e) resemble the temporal profile of the EC–OC particles, however, with additional transient features that have a width of approximately 20–60 min and occur only during wind from the north. As is apparent from Fig. 3d, the V–Fe–Ni class contributes only a small fraction to total particle numbers, while during the transient events they account for 10 %–20 %.
In order to give an estimate of the benefit of resonant Fe ionization, we performed laboratory experiments on a research ship engine. Herein, we compared the SPMS spectra and the fraction of particles showing metal signatures for the resonant Fe ionization at 248 nm versus the more common SPMS wavelength at 266 nm. The results are shown in Fig. S3. Although these are no ambient air experiments on real plumes, the results indicate an ≈15 times more frequent detection of Fe at 248 nm, which roughly corresponds to the Fe enhancements found in the ambient air study (Passig et al., 2020). Of note, V is ≈2 times and Ni ≈4 times more frequently detected; see Fig. S3. An explanation can be found in the relatively broad laser spectrum at 248 nm overlapping with further atomic absorption lines of the metals (Passig et al., 2020).
The particle identification via ART-2a clustering that recognizes the full pattern of V, Ni and Fe can be evaluated by a comparison with an ion-marker screening for only 51V+ and 67VO+, as shown by the grey area in Fig. 3e. 51V+ and 67VO+ may interfere with major organic fragments (and 56Fe+ with 56CaO+), as apparent for periods with high contributions from organic particles in Fig. 3. Higher signal thresholds for marker ions can mitigate that problem at the cost of sensitivity.
3.3 Background particles and particles from transient events
To elucidate the sources and atmospheric processing of the V–Fe–Ni particles, we separately analyzed the mass spectra and temporal behavior of the 12 most abundant clusters out of the total 15 clusters assigned to the V–Fe–Ni class. Figure 4a lists the clusters according to their labels in the full cluster analysis (first row) in the order of their respective particle counts (second row). The average mass spectra of the clusters (Fig. 4b and c) show signals from EC, OC, Ca+ and Na+ as well as the metal signatures. Generally, all clusters with negative ion spectra reveal a dominant peak (with additional EC signals for clusters 161 and 164 and nitrate for cluster 226).
Time series of the particle clusters are depicted in Fig. 4d. An important finding here is that the cluster algorithm was exclusively applied to chemical particle data, but it also yielded two distinct groups according to the particle's temporal behavior, as discussed in the following. The first group of V–Fe–Ni particles, comprising the clusters 97, 111, 127, 138, 196 and 226, shows rather smooth time series, comparable to the EC–OC class in Fig. 3c. The mass spectra of this group reveal either no negative ions or comparably small signals from sulfate (fourth row in Fig. 4a) or secondary nitrate (cluster 226). Also, the positive ion signals apart from transition metals are weak. The smooth temporal behavior (Fig. 4d) gives rise to the term “background group”. These particles are predominantly observed during phases of light onshore winds, also from northwesterly directions, where heavily trafficked ship routes towards the North Sea and the Atlantic Ocean are located. Such particles most likely originate from distant shipping lanes.
The second group identified by mass spectral signatures contributes the transient events and is formed by the clusters 110, 150, 151, 161, 164 and 183. All of these particle clusters show negative ions and, with the exception of cluster 150, also remarkable signals of EC, OC, Ca+ and Na+ in the positive ion mass spectra. We term these particles the “transient group”, as their temporal behavior points to individual, less distant sources. Cluster 111 combines both properties. Because of the biased aerosol concentration and sampling in our study, the particle size distribution is rather uniform and allows no differentiation between local and distant emissions.
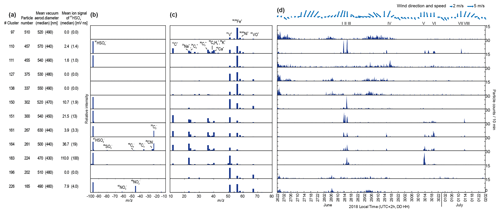
Figure 4Results of the ART-2a clustering for 12 of the 15 clusters showing signatures of residual fuel combustion. (a) Cluster number according to their labels in the full cluster analysis (see the Supplement), particle number, vacuum aerodynamic diameter and average sulfate signal of the clusters. (b) Weight matrices (spectra of the cluster center) of negative ions and (c) positive ions corresponding to average mass spectra. (d) Top: measured wind data and ship plume numbers; bottom: time series of particle counts with 10 min resolution. Note the different y-axis scales. While only mass spectral data were considered in the clustering algorithm, the time series of the resulting clusters also reveal two distinct groups: the transient group with bipolar mass spectra and short events as well as the background group with dominant metal signatures and smooth time series.
The main difference between the two groups is the limited number of negative ion signals and the weaker positive signatures from EC, OC, Ca+ and Na+ for the background particles. The absence of negative ion signals in SPMS was commonly associated with water uptake during aerosol ageing (Neubauer et al., 1998; Moffet et al., 2008; Ault et al., 2010), but suppression effects on positive ions were also documented (Neubauer et al., 1997; Dall'Osto et al., 2006). Hatch et al. (2014) found that laser absorption and particle ablation in LDI are reduced from coatings of secondary species, finally affecting mass spectra for both polarities.
Previous studies on ship emission particles have discussed the lack of negative ion mass spectra (Ault et al., 2009), the balance between sulfate and nitrate (Liu et al., 2017) or solely the presence of nitrate signals (Wang et al., 2019) as indicators of atmospheric ageing. However, a suppression of positive ions through ageing was not reported in these studies, although the mass spectra of substantially aged ship particles shown by Ault et al. (2009) also reveal a low relative intensity of EC, OC, Ca+ and Na+ compared to the freshly emitted particles documented by in-port studies (Healy et al., 2009; Ault et al., 2010; Liu et al., 2017; Xiao et al., 2018). Generally, the formation of negative ions by electron capture requires previous generation of positive ions and is therefore more prone to suppression effects. In consequence, the relative heights of positive ion peaks apart from the transition metals should be considered a further estimate for the amount of atmospheric ageing of ship particles. The respective mass spectral indicators for ageing of V–Fe–Ni particles are summarized in Table 1.
3.4 Metal signatures of V–Fe–Ni particles
In contrast to anions and EC, OC as well as alkali cations, the transition metal signals are more stable and remain also after long-range transport (Furutani et al., 2011; Ault et al., 2009). A difference to all previous SPMS studies on ship emissions is the strong Fe+ signal and the remarkable Ni+ signal in most particles. Therefore, it appears feasible to evaluate whether signal ratios may be indicative for a specific source, e.g., as a result of different fuel composition (Viana et al., 2009). However, from Fig. 4c and d, it becomes apparent that the same transient events or sources contribute particle clusters with very different ratios. On the other hand, these ratios do not differ much among the plumes, as summarized in Table 2. Only plume V stands out by higher V+ signals in relation to the other metals and also by a particularly high sulfate signal.
Table 2Ship plumes according to Figs. 3, 4 and 5, their main properties as well as the median of relative signal ratios for metals and sulfate signals.
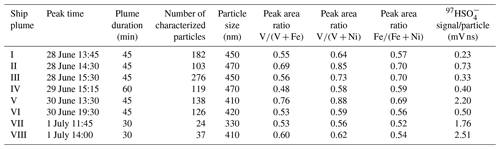
In general, background particles tend to show higher Fe and lower V signals compared to the transient group, but due to a number of exceptions (clusters 110 and 196), we cannot draw clear conclusions here. Note that the LDI signal strength in SPMS does not necessarily indicate the component's mass concentration. Beyond the transition metals, Ca+ signals appear in all clusters of the transient group. Strong calcium signals from lubrication oil additives were frequently observed in SPMS studies on diesel engines (Toner et al., 2008; Shields et al., 2007), mainly because of its low ionization potential, and calcium was also found in particulate emissions from ships using residual fuels (Moldanová et al., 2009; Streibel et al., 2017).
3.5 Sulfate signals
As is apparent from the fourth row in Fig. 4a, there are considerable differences in sulfate signals, both between the clusters of the transient group and between the plumes; see Table 2. Although sulfate can also be secondary, fresh plumes from sulfur-rich fuel combustion have particular high sulfate contents from gas-particle conversion of SO2 (Murphy et al., 2009; Ault et al., 2010; Healy et al., 2009). In this regard, the temporal trend of the sulfate ion yield from all particles is plotted in Fig. 5b (yellow area), while the time series of all V–Fe–Ni particles are again shown in Fig. 5a for comparison. The sulfate yield shows slightly elevated background for marine air during northern winds and some smaller features that are not correlated with the number of V–Fe–Ni particles. However, for some of the transients from V–Fe–Ni particles, we find coinciding features of sulfate levels within the full particle ensemble. Comparison with the sulfate ion yield from only V–Fe–Ni particles (brown) reveals that this particle type contributes the main fraction of sulfate during these incidents.
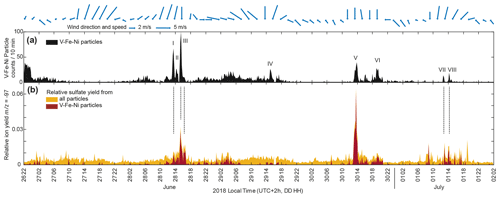
Figure 5(a) Time series of V–Fe–Ni particles (same as Fig. 3e). (b) Yellow area: the ion yield of normalized to the particle number per 10 min reveals coinciding sulfate events for some of the V–Fe–Ni features. The majority of sulfate is detected on the V–Fe–Ni particles themselves (brown area). Most of these particles belong to cluster 183 in Fig. 4.
3.6 Assignment to ships
Land-based sources can be excluded for the transient group particles, because there are no refineries or chemical industry plants in the town, and the local coal power plant was not in operation during measurements. There are two possible source regions of V–Fe–Ni particles: the main shipping lane (Kadet channel, >50 000 passages per year), about 40 km north of the sampling site, and the harbor of Rostock (≈7000 approaches, 75 % ferries and roll-on-roll-off ships), located about 10 km north of the site; see Fig. 6a. The complete Baltic Sea is a SECA, with a 0.1 % limit for sulfur in fuel mass. Several studies assessed the compliance of ships to more than 95 % (International Transport Forum Policy Papers, 2016; Lähteenmäki-Uutela et al., 2019). Thus, it appears unlikely to detect many ship plumes from operation with conventional high-sulfur bunker fuel within about 36 h with northern wind in our study. However, an increasing number of ships is currently equipped with scrubbers (Winnes et al., 2018), efficiently removing SO2 from the exhaust with moderate effects on the PM emissions (Fridell and Salo, 2016; Lehtoranta et al., 2019). Several ships with scrubbers regularly approach the port of Rostock. The ferry route to Denmark is operated with a pair of hybrid ferries (ferry A and B), being equipped with scrubbers and using batteries for in-port manoeuvring. The diesel engines are started at the harbor exit, directly east of the meteorological station and 12 km north of the measurement site; see the enlarged view in Fig. 6a. On the way back, the engines are stopped at the same position. The typical turnaround times between two departures are 2 h and 15 min, matching the delay between two major transient events during afternoon on 28 and 30 June; see Fig. 4d.
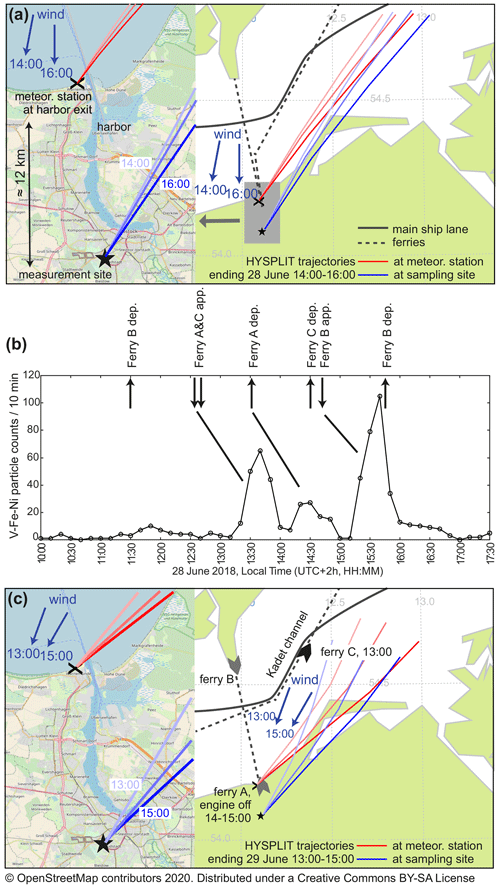
Figure 6Assignment of transient particle events to distant ship passages. (a) For the first transients, observed on 28 June during a period of northern wind, the HYSPLIT trajectories indicate transport of particles from distant sea areas to the sampling site (star). However, the measured wind directions (blue arrows) in conjunction with ship transponder data reveal ferries with scrubbers as the probable source. (b) Time series of particles from the transient group during northern winds on 28 June. The particle events follow the approach and departure times of the ferries (arrows) with a delay of about 45 min during optimum wind directions. (c) On 29 June, the wind direction is north-northeast, and only one weak transient event appears (see Fig. 4). However, a broader feature of aged V–Fe–Ni particles at 15:00 indicates a distant source, and ferry C is underway in the Kadet channel in the respective time frame (and six further ships). The difference between trajectories and measured wind data emphasizes the importance of accurate meteorological data in potential future monitoring systems.
For the period with northerly wind on 28 June, the times when the ferries pass the harbor exit, where the engines are typically started and stopped, are derived from AIS data; see Fig. 6b. These passages of the harbor exit are followed by strong events of particles from the transient group with a delay of 45–60 min, in agreement with the wind speed of about 4 m s−1. There was a further ferry “C” with a scrubber (no hybrid), approaching Rostock around 12:45 and leaving the port at 14:30, whose signals interfere with ferries A and B. For earlier and later departures and arrivals, the wind direction was unfavorable on this day.
From the HYSPLIT back trajectories, shown by the red and blue lines in Fig. 6a, it appears unlikely that the V–Fe–Ni particle transients stem from the harbor area, as they indicate distant source regions further east. However, there is substantial difference between the trajectories and the measured wind data; see blue arrows in Fig. 6. Using the measured wind data, the group of transient particle events on 28 June is in agreement with AIS data from the ferries.
On the next day (29 June), there is no transient feature of comparable intensity, because the wind turned rapidly to northeast. However, a broader event lasting about 1 h around 15:00 can be noticed for particles of cluster 111 (Fig. 4). This cluster shows a different chemical profile, with solely sulfate in negative mode and marginal signals of EC, OC and alkali metals in positive mode, which we associated with ageing and a more distant source; see Sect. 3.3. Both the trajectories as well as the measured wind data shown in Fig. 6c reveal a northeasterly direction, guiding air masses from the eastern Kadet channel to the site. Among six cargo ships, ferry C passed this stretch between 12:30 and 14:00 on its way from a different harbor to Sweden. This ferry is also equipped with a scrubber and therefore legally operated with heavy fuel oil, contributing a possible source of the detected V–Fe–Ni particles. Its position at 13:00 is indicated in Fig. 6c, while the hybrid ferries A and B were in the harbor (engines off) in the relevant time between 14:00 and 15.00 and can therefore be excluded as a source. Therefore, it appears likely that the event of cluster 111 on 29 June at 15:00 results from regional transport from the Kadet channel (>30 km distance). In conjunction with the strong transient signals from 28 June, this suggests that long-range detection of residual fuel operation might be possible if the wind field is captured correctly and if the traffic is not too dense.
On 30 June, during straight northerly winds, Fig. 4d shows several transient V–Fe–Ni particle events, including a small dual-peak pattern for cluster 110 with 2 h 15 min delay, matching AIS-derived departures of ferries A and B, respectively. The time series of sulfate signals in Fig. 5b reveals a strong increase that is mainly contributed by particles from cluster 183; compare Fig. 4. The event coincides with departure of ferry A and may be associated with scrubber malfunction or delayed onset of its operation. High sulfate emissions from ships with installed scrubbers have previously been reported (Mellqvist et al., 2017b, a). However, a different source, such as a further passing ship, cannot be excluded.
The background particle signals can be associated with general ship traffic, which is also supported by their increase during wind from the western Baltic Sea and the North Sea. The high levels at the beginning of the measurements followed a period of air mass stagnation in the western Baltic Sea, where emissions have probably been enriched. Ships can emit V–Fe–Ni particles for three reasons: (I) under operation with residual fuels by using scrubbers or (II) if non-compliant fuels or (III) desulfurized hybrid fuels are used. Antturi et al. (2016) estimated that only 136 of about 5000 ships sailing in the Baltic Sea had installed a scrubber, and the large majority of ships use distillate fuels such as MGO. Therefore, a further source is conceivable: as shown in Fig. 4, all particle clusters that belong to the transient group show calcium signals from lubrication oil. While the size distribution of freshly emitted soot particles from diesel engines peaks well below 100 nm (Streibel et al., 2017; Oeder et al., 2015), the majority of particles in the accumulation modes show signatures of lube oil (Lyyränen et al., 1999; Sakurai et al., 2003; Toner et al., 2006). Because many ships run on residual fuels outside the SECAs and switch to low-sulfur distillates when entering them (Van Roy and Scheldemann, 2016; Lähteenmäki-Uutela et al., 2019), their lubrication oil contains metals from previous residual fuel operation that can consequently be emitted in small amounts also during operation in SECAs, as shown by our measurements on a research ship engine; see the Supplement. Recently, Zanatta et al. (2020) also found a limited number of V–Fe particles in the marine boundary layer along ship lanes in the Baltic Sea using an aircraft-based SPMS.
Our analysis of AIS data revealed that a total number of 470 cargo ships, tankers and passenger ships of all sizes were sailing the major ship lane during our measurement period. It should be noted that the ships running on distillate fuels such as MGO cannot be separated from other fuel combustion sources by our approach. Considering the typical compliance rate and the small number of ships with scrubbers, it can be estimated that less than 10 % of the particles from ships are V–Fe–Ni particles. Consequently, a substantial fraction of the EC–OC particles may also stem from ships, which is supported by the increased number of EC–OC particles during onshore winds; see Fig. 3.
With the present study, we demonstrated the chemical detection of individual ship plumes from more than 10 km distance. It could be shown that ships with installed scrubbers can also be detected by their PM emissions, indicating that the emissions of toxic transition metals from residual fuel combustion are not sufficiently abated by scrubbers. This emphasizes the need for additional cleaning technologies and cleaner fuels. By using chemical indicators on a single-particle basis for the presence of ship plumes, we extended the approach to perform stationary measurements at some distance downwind of shipping lanes (Ausmeel et al., 2019; Celik et al., 2020). Of note, this renders the method independent of background aerosols as long as source-specific and detectable markers exist. We analyzed mass spectral signatures for ageing of particles from residual fuel combustion and recommend considering the suppression of positive ions apart from transition metals as an additional ageing indicator for this particle class. Our results furthermore suggest that the signal ratio between transition metals is not a suitable marker for individual ship assignment with SPMS.
From analysis of transient particle events, wind data and ship transponder signals, it becomes apparent that accuracy in wind data, possible mixing of different plumes during high traffic and prevailing wind directions are key limiting factors rather than chemical detection limits or background air pollution. Consequently, SPMS-based monitoring systems should acquire local wind data, and small-scale plume dispersion models should be integrated (Matthias et al., 2018; Badeke et al., 2021). The possible operation time is mainly limited by the prevailing wind directions, which should be perpendicular to the ship lane to avoid plume mixing and straight to the monitoring site. However, this limitation can be overcome by installation of two monitoring stations. Favorable places are opposite sides of straits covering the main wind directions, at islands near major shipping routes and waterways to large ports. Mobile ship-based units could complement such monitoring networks.
While our approach can detect ship plumes from residual fuel operation, it is not applicable for monitoring of ship emissions from distillate fuels, because in its present form, it does not unambiguously separate ships running on MGO from land-based traffic emissions. Novel markers for ship emissions beyond the metal signatures have been identified, including source-specific signatures of polycyclic aromatic hydrocarbons (PAHs) (Czech et al., 2017a, b). Recent developments in SPMS allow us to acquire detailed PAH profiles from individual particles (Passig et al., 2017; Schade et al., 2019), and therefore they open a perspective towards a comprehensive monitoring protocol for ship emissions and individual plumes.
Data are available on request from Johannes Passig (johannes.passig@uni-rostock.de).
The supplement related to this article is available online at: https://doi.org/10.5194/amt-14-4171-2021-supplement.
JP designed the experiments, analyzed data, prepared the figures and wrote the manuscript with contributions from all the authors. JS and RI developed software and performed the experiments. LL, XL and ZZ provided the SPMS instrument. TA and RZ assisted with technical support, data interpretation and manuscript writing.
The authors declare that they have no conflict of interest.
Instrumental and technical support was provided by Photonion GmbH, Schwerin, Germany. We thank the German Federal Waterways and Shipping Administration for providing AIS data, Germany's National Meteorological Service “Deutscher Wetterdienst” for wind data and the State Agency for the Environment, Nature Conservation and Geology Mecklenburg-Vorpommern for PM2.5 data. The authors are grateful for the NOAA Air Resources Laboratory (ARL) for the provision of the HYSPLIT transport and dispersion model and READY website (https://www.ready.noaa.gov, last access: 20 November 2020) used in this publication.
This research has been supported by the Deutsche Forschungsgemeinschaft (grant no. ZI 764/6-1), the Bundesministerium für Wirtschaft und Energie (grant no. ZF4402101 ZG7), and the Helmholtz-Gemeinschaft (International Lab Aerohealth and Virtual Institute of Complex Molecular Systems in Environmental Health, HICE).
This paper was edited by Markus Rapp and reviewed by two anonymous referees.
Antturi, J., Hänninen, O., Jalkanen, J.-P., Johansson, L., Prank, M., Sofiev, M., and Ollikainen, M.: Costs and benefits of low-sulphur fuel standard for Baltic Sea shipping, J. Environ. Manag., 184, 431–440, https://doi.org/10.1016/j.jenvman.2016.09.064, 2016.
Arndt, J., Sciare, J., Mallet, M., Roberts, G. C., Marchand, N., Sartelet, K., Sellegri, K., Dulac, F., Healy, R. M., and Wenger, J. C.: Sources and mixing state of summertime background aerosol in the north-western Mediterranean basin, Atmos. Chem. Phys., 17, 6975–7001, https://doi.org/10.5194/acp-17-6975-2017, 2017.
Ault, A. P., Moore, M. J., Furutani, H., and Prather, K. A.: Impact of Emissions from the Los Angeles Port Region on San Diego Air Quality during Regional Transport Events, Environ. Sci. Technol., 43, 3500–3506, https://doi.org/10.1021/es8018918, 2009.
Ault, A. P., Gaston, C. I., Wang, Y., Dominguez, G., Thiemens, M. H., and Prather, K. A.: Characterization of the single particle mixing state of individual ship plume events measured at the Port of Los Angeles, Environ. Sci. Technol., 44, 1954–1961, https://doi.org/10.1021/es902985h, 2010.
Ausmeel, S., Eriksson, A., Ahlberg, E., and Kristensson, A.: Methods for identifying aged ship plumes and estimating contribution to aerosol exposure downwind of shipping lanes, Atmos. Meas. Tech., 12, 4479–4493, https://doi.org/10.5194/amt-12-4479-2019, 2019.
Ausmeel, S., Eriksson, A., Ahlberg, E., Sporre, M. K., Spanne, M., and Kristensson, A.: Ship plumes in the Baltic Sea Sulfur Emission Control Area: chemical characterization and contribution to coastal aerosol concentrations, Atmos. Chem. Phys., 20, 9135–9151, https://doi.org/10.5194/acp-20-9135-2020, 2020.
Badeke, R., Matthias, V., and Grawe, D.: Parameterizing the vertical downward dispersion of ship exhaust gas in the near field, Atmos. Chem. Phys., 21, 5935–5951, https://doi.org/10.5194/acp-21-5935-2021, 2021.
Balzani Lööv, J. M., Alfoldy, B., Gast, L. F. L., Hjorth, J., Lagler, F., Mellqvist, J., Beecken, J., Berg, N., Duyzer, J., Westrate, H., Swart, D. P. J., Berkhout, A. J. C., Jalkanen, J.-P., Prata, A. J., van der Hoff, G. R., and Borowiak, A.: Field test of available methods to measure remotely SOx and NOx emissions from ships, Atmos. Meas. Tech., 7, 2597–2613, https://doi.org/10.5194/amt-7-2597-2014, 2014.
Beecken, J., Mellqvist, J., Salo, K., Ekholm, J., and Jalkanen, J.-P.: Airborne emission measurements of SO2, NOx and particles from individual ships using a sniffer technique, Atmos. Meas. Tech., 7, 1957–1968, https://doi.org/10.5194/amt-7-1957-2014, 2014.
Berg, N., Mellqvist, J., Jalkanen, J.-P., and Balzani, J.: Ship emissions of SO2 and NO2: DOAS measurements from airborne platforms, Atmos. Meas. Tech., 5, 1085–1098, https://doi.org/10.5194/amt-5-1085-2012, 2012.
Celik, S., Drewnick, F., Fachinger, F., Brooks, J., Darbyshire, E., Coe, H., Paris, J.-D., Eger, P. G., Schuladen, J., Tadic, I., Friedrich, N., Dienhart, D., Hottmann, B., Fischer, H., Crowley, J. N., Harder, H., and Borrmann, S.: Influence of vessel characteristics and atmospheric processes on the gas and particle phase of ship emission plumes: in situ measurements in the Mediterranean Sea and around the Arabian Peninsula, Atmos. Chem. Phys., 20, 4713–4734, https://doi.org/10.5194/acp-20-4713-2020, 2020.
Celo, V., Dabek-Zlotorzynska, E., and McCurdy, M.: Chemical Characterization of Exhaust Emissions from Selected Canadian Marine Vessels: The Case of Trace Metals and Lanthanoids, Environ. Sci. Technol., 49, 5220–5226, https://doi.org/10.1021/acs.est.5b00127, 2015.
Chen, G., Huey, L. G., Trainer, M., Nicks, D., Corbett, J., Ryerson, T., Parrish, D., Neuman, J. A. Nowak, J., Tanner, D., Holloway, J., Brock, C., Crawford, J., Olson, J. R., Sullivan, A., Weber, R., Schauffler, S., Donnelly, S., Atlas, E., Roberts, J., Flocke, F., Hübler, G., and Fehsenfeld, F.: An investigation of the chemistry of ship emission plumes during ITCT 2002, J. Geophys. Res., 110, D10S90, https://doi.org/10.1029/2004JD005236, 2005.
Corbett, J. J., Winebrake, J. J., Green, E. H., Kasibhatla, P., Eyring, V., and Lauer, A.: Mortality from Ship Emissions: A Global Assessment, Environ. Sci. Technol., 41, 8512–8518, https://doi.org/10.1021/es071686z, 2007.
Corbin, J. C., Mensah, A. A., Pieber, S. M., Orasche, J., Michalke, B., Zanatta, M., Czech, H., Massabò, D., Buatier de Mongeot, F., Mennucci, C., El Haddad, I., Kumar, N. K., Stengel, B., Huang, Y., Zimmermann, R., Prévôt, A. S. H., and Gysel, M.: Trace Metals in Soot and PM2.5 from Heavy-Fuel-Oil Combustion in a Marine Engine, Environ. Sci. Technol., 52, 6714–6722, https://doi.org/10.1021/acs.est.8b01764, 2018.
Corbin, J. C., Czech, H., Massabò, D., Mongeot, F. B. de, Jakobi, G., Liu, F., Lobo, P., Mennucci, C., Mensah, A. A., Orasche, J., Pieber, S. M., Prévôt, A. S. H., Stengel, B., Tay, L.-L., Zanatta, M., Zimmermann, R., El Haddad, I., and Gysel, M.: Infrared-absorbing carbonaceous tar can dominate light absorption by marine-engine exhaust, npj Climate and Atmospheric Science, 2, 12, https://doi.org/10.1038/s41612-019-0069-5, 2019.
Czech, H., Schnelle-Kreis, J., Streibel, T., and Zimmermann, R.: New directions: Beyond sulphur, vanadium and nickel – About source apportionment of ship emissions in emission control areas, Atmos. Environ., 163, 190–191, https://doi.org/10.1016/j.atmosenv.2017.05.017, 2017a.
Czech, H., Stengel, B., Adam, T., Sklorz, M., Streibel, T., and Zimmermann, R.: A chemometric investigation of aromatic emission profiles from a marine engine in comparison with residential wood combustion and road traffic: Implications for source apportionment inside and outside sulphur emission control areas, Atmos. Environ., 167, 212–222, https://doi.org/10.1016/j.atmosenv.2017.08.022, 2017b.
Dall'Osto, M., Harrison, R. M., Beddows, D. C. S., Freney, E. J., Heal, M. R., and Donovan, R. J.: Single-Particle Detection Efficiencies of Aerosol Time-of-Flight Mass Spectrometry during the North Atlantic Marine Boundary Layer Experiment, Environ. Sci. Technol., 40, 5029–5035, https://doi.org/10.1021/es050951i, 2006.
Di Wu, Li, Q., Ding, X., Sun, J., Li, D., Fu, H., Teich, M., Ye, X., and Chen, J.: Primary Particulate Matter Emitted from Heavy Fuel and Diesel Oil Combustion in a Typical Container Ship: Characteristics and Toxicity, Environ. Sci. Technol., 52, 12943–12951, https://doi.org/10.1021/acs.est.8b04471, 2018.
Diesch, J.-M., Drewnick, F., Klimach, T., and Borrmann, S.: Investigation of gaseous and particulate emissions from various marine vessel types measured on the banks of the Elbe in Northern Germany, Atmos. Chem. Phys., 13, 3603–3618, https://doi.org/10.5194/acp-13-3603-2013, 2013.
Eyring, V., Isaksen, I. S., Berntsen, T., Collins, W. J., Corbett, J. J., Endresen, O., Grainger, R. G., Moldanova, J., Schlager, H., and Stevenson, D. S.: Transport impacts on atmosphere and climate: Shipping, Atmos. Environ., 44, 4735–4771, https://doi.org/10.1016/j.atmosenv.2009.04.059, 2010.
Fang, T., Guo, H., Zeng, L., Verma, V., Nenes, A., and Weber, R. J.: Highly Acidic Ambient Particles, Soluble Metals, and Oxidative Potential: A Link between Sulfate and Aerosol Toxicity, Environ. Sci. Technol., 51, 2611–2620, https://doi.org/10.1021/acs.est.6b06151, 2017.
Fridell, E. and Salo, K.: Measurements of abatement of particles and exhaust gases in a marine gas scrubber, Proceedings of the IMechE, 230, 154–162, https://doi.org/10.1177/1475090214543716, 2016.
Furutani, H., Jung, J., Miura, K., Takami, A., Kato, S., Kajii, Y., and Uematsu, M.: Single-particle chemical characterization and source apportionment of iron-containing atmospheric aerosols in Asian outflow, J. Geophys. Res., 116, D18204, https://doi.org/10.1029/2011JD015867, 2011.
Gaie-Levrel, F., Perrier, S., Perraudin, E., Stoll, C., Grand, N., and Schwell, M.: Development and characterization of a single particle laser ablation mass spectrometer (SPLAM) for organic aerosol studies, Atmos. Meas. Tech., 5, 225–241, https://doi.org/10.5194/amt-5-225-2012, 2012.
Gaston, C. J., Quinn, P. K., Bates, T. S., Gilman, J. B., Bon, D. M., Kuster, W. C., and Prather, K. A.: The impact of shipping, agricultural, and urban emissions on single particle chemistry observed aboard the R/V Atlantis during CalNex, J. Geophys. Res., 118, 5003–5017, https://doi.org/10.1002/jgrd.50427, 2013.
Hatch, L. E., Pratt, K. A., Huffman, J. A., Jimenez, J. L., and Prather, K. A.: Impacts of Aerosol Aging on Laser Desorption/Ionization in Single-Particle Mass Spectrometers, Aerosol Sci. Technol., 48, 1050–1058, https://doi.org/10.1080/02786826.2014.955907, 2014.
Healy, R. M., O'Connor, I. P., Hellebust, S., Allanic, A., Sodeau, J. R., and Wenger, J. C.: Characterisation of single particles from in-port ship emissions, Atmos. Environ., 43, 6408–6414, https://doi.org/10.1016/j.atmosenv.2009.07.039, 2009.
International Transport Forum Policy Papers: Reducing Sulphur Emissions from Ships: The Impact of International Regulation, Organisation for Economic Cooperation and Development (OECD) iLibrary, ISSN: 24108871, https://doi.org/10.1787/24108871, 2016.
Jonson, J. E., Gauss, M., Jalkanen, J.-P., and Johansson, L.: Effects of strengthening the Baltic Sea ECA regulations, Atmos. Chem. Phys., 19, 13469–13487, https://doi.org/10.5194/acp-19-13469-2019, 2019.
Jonson, J. E., Gauss, M., Schulz, M., Jalkanen, J.-P., and Fagerli, H.: Effects of global ship emissions on European air pollution levels, Atmos. Chem. Phys., 20, 11399–11422, https://doi.org/10.5194/acp-20-11399-2020, 2020.
Kattner, L., Mathieu-Üffing, B., Burrows, J. P., Richter, A., Schmolke, S., Seyler, A., and Wittrock, F.: Monitoring compliance with sulfur content regulations of shipping fuel by in situ measurements of ship emissions, Atmos. Chem. Phys., 15, 10087–10092, https://doi.org/10.5194/acp-15-10087-2015, 2015.
Lack, D. A., Corbett, J. J., Onasch, T., Lerner, B., Massoli, P., Quinn, P. K., Bates, T. S., Covert, D. S., Coffman, D., Sierau, B., Herndon, S., Allan, J., Baynard, T., Lovejoy, E., Ravishankara, A. R., and Williams, E.: Particulate emissions from commercial shipping: Chemical, physical, and optical properties, J. Geophys. Res., 114, D00F04, https://doi.org/10.1029/2008JD011300, 2009.
Lack, D. A., Cappa, C. D., Langridge, J., Bahreini, R., Buffaloe, G., Brock, C., Cerully, K., Coffman, D., Hayden, K., Holloway, J., Lerner, B., Massoli, P., Li, S.-M., McLaren, R., Middlebrook, A. M., Moore, R., Nenes, A., Nuaaman, I., Onasch, T. B., Peischl, J., Perring, A., Quinn, P. K., Ryerson, T., Schwartz, J. P., Spackman, R., Wofsy, S. C., Worsnop, D., Xiang, B., and Williams, E.: Impact of Fuel Quality Regulation and Speed Reductions on Shipping Emissions: Implications for Climate and Air Quality, Environ. Sci. Technol., 45, 9052–9060, https://doi.org/10.1021/es2013424, 2011.
Lähteenmäki-Uutela, A., Yliskylä-Peuralahti, J., Repka, S., and Mellqvist, J.: What explains SECA compliance: rational calculation or moral judgment?, WMU Journal of Maritime Affairs, 18, 61–78, https://doi.org/10.1007/s13437-019-00163-1, 2019.
Lehtoranta, K., Aakko-Saksa, P., Murtonen, T., Vesala, H., Ntziachristos, L., Rönkkö, T., Karjalainen, P., Kuittinen, N., and Timonen, H.: Particulate Mass and Nonvolatile Particle Number Emissions from Marine Engines Using Low-Sulfur Fuels, Natural Gas, or Scrubbers, Environ. Sci. Technol., 53, 3315–3322, https://doi.org/10.1021/acs.est.8b05555, 2019.
Li, L., Huang, Z., Dong, J., Li, M., Gao, W., Nian, H., Fu, Z., Zhang, G., Bi, X., Cheng, P., and Zhou, Z.: Real time bipolar time-of-flight mass spectrometer for analyzing single aerosol particles, Int. J. Mass Spectrom., 303, 118–124, https://doi.org/10.1016/j.ijms.2011.01.017, 2011.
Liu, Z., Lu, X., Feng, J., Fan, Q., Zhang, Y., and Yang, X.: Influence of Ship Emissions on Urban Air Quality: A Comprehensive Study Using Highly Time-Resolved Online Measurements and Numerical Simulation in Shanghai, Environ. Sci. Technol., 51, 202–211, https://doi.org/10.1021/acs.est.6b03834, 2017.
Lyyränen, J., Jokiniemi, J., Kauppinen, E. I., and Joutsensaari, J.: Aerosol characterisation in medium-speed diesel engines operating with heavy fuel oils, J. Aerosol Sci., 30, 771–784, https://doi.org/10.1016/S0021-8502(98)00763-0, 1999.
Matthias, V., Arndt, J. A., Aulinger, A., Bieser, J., Denier van der Gon, Hugo, Kranenburg, R., Kuenen, J., Neumann, D., Pouliot, G., and Quante, M.: Modeling emissions for three-dimensional atmospheric chemistry transport models, J. Air Waste Manage., 68, 763–800, https://doi.org/10.1080/10962247.2018.1424057, 2018.
Mellqvist, J., Beecken, J., Conde, V., and Ekholm, J.: Surveillance of sulphur emissions from ships in Danish waters, Report to the Danish Environmental Protection Agency, Report no. 500251, available at: https://research.chalmers.se/publication/500251 (last access: 24 November 2020), 2017a.
Mellqvist, J., Conde, V., Beecken, J., and Ekholm, J.: Fixed remote surveillance of fuel sulfur content in ships from fixed sites in the Göteborg ship channel and Öresund bridge,Chalmers University of Technology, Report no. 500248, available at: https://research.chalmers.se/en/publication/500248 (last access: 24 November 2020), 2017b.
Moffet, R. C., Qin, X., Rebotier, T., Furutani, H., and Prather, K. A.: Chemically segregated optical and microphysical properties of ambient aerosols measured in a single-particle mass spectrometer, J. Geophys. Res., 113, D12213, https://doi.org/10.1029/2007JD009393, 2008.
Moldanová, J., Fridell, E., Popovicheva, O., Demirdjian, B., Tishkova, V., Faccinetto, A., and Focsa, C.: Characterisation of particulate matter and gaseous emissions from a large ship diesel engine, Atmos. Environ., 43, 2632–2641, https://doi.org/10.1016/j.atmosenv.2009.02.008, 2009.
Murphy, S. M., Agrawal, H., Sorooshian, A., Padró, L. T., Gates, H., Hersey, S., Welch, W. A., Jung, H., Miller, J. W., Cocker, D. R., Nenes, A., Jonsson, H. H., Flagan, R. C., and Seinfeld, J. H.: Comprehensive Simultaneous Shipboard and Airborne Characterization of Exhaust from a Modern Container Ship at Sea, Environ. Sci. Technol., 43, 4626–4640, https://doi.org/10.1021/es802413j, 2009.
Neubauer, K. R., Johnston, M. V., and Wexler, A. S.: On-line analysis of aqueous aerosols by laser desorption ionization, Int. J. Mass. Spectrom. Ion Process., 163, 29–37, https://doi.org/10.1016/S0168-1176(96)04534-X, 1997.
Neubauer, K. R., Johnston, M. V., and Wexler, A. S.: Humidity effects on the mass spectra of single aerosol particles, Atmos. Environ., 32, 2521–2529, https://doi.org/10.1016/S1352-2310(98)00005-3, 1998.
Oeder, S., Kanashova, T., Sippula, O., Sapcariu, S. C., Streibel, T., Arteaga-Salas, J. M., Passig, J., Dilger, M., Paur, H.-R., Schlager, C., Mülhopt, S., Diabaté, S., Weiss, C., Stengel, B., Rabe, R., Harndorf, H., Torvela, T., Jokiniemi, J. K., Hirvonen, M.-R., Schmidt-Weber, C., Traidl-Hoffmann, C., BéruBé, K. A., Wlodarczyk, A. J., Prytherch, Z., Michalke, B., Krebs, T., Prévôt, A. S. H., Kelbg, M., Tiggesbäumker, J., Karg, E., Jakobi, G., Scholtes, S., Schnelle-Kreis, J., Lintelmann, J., Matuschek, G., Sklorz, M., Klingbeil, S., Orasche, J., Richthammer, P., Müller, L., Elsasser, M., Reda, A., Gröger, T., Weggler, B., Schwemer, T., Czech, H., Rüger, C. P., Abbaszade, G., Radischat, C., Hiller, K., Buters, J. T. M., Dittmar, G., and Zimmermann, R.: Particulate matter from both heavy fuel oil and diesel fuel shipping emissions show strong biological effects on human lung cells at realistic and comparable in vitro exposure conditions, PloS ONE, 10, e0126536, https://doi.org/10.1371/journal.pone.0126536, 2015.
Passig, J. and Zimmermann, R.: Laser Ionization in Single-Particle Mass Spectrometry, in: Photoionization and Photo-induced Processes in Mass Spectrometry: Fundamentals and Applications, edited by: Hanley, L. and Zimmermann, R., Wiley-VCH, Weinheim, 359–411, https://doi.org/10.1002/9783527682201.ch11, 2021.
Passig, J., Schade, J., Oster, M., Fuchs, M., Ehlert, S., Jäger, C., Sklorz, M., and Zimmermann, R.: Aerosol Mass Spectrometer for Simultaneous Detection of Polyaromatic Hydrocarbons and Inorganic Components from Individual Particles, Anal. Chem., 89, 6341–6345, https://doi.org/10.1021/acs.analchem.7b01207, 2017.
Passig, J., Schade, J., Rosewig, E. I., Irsig, R., Kröger-Badge, T., Czech, H., Sklorz, M., Streibel, T., Li, L., Li, X., Zhou, Z., Fallgren, H., Moldanova, J., and Zimmermann, R.: Resonance-enhanced detection of metals in aerosols using single-particle mass spectrometry, Atmos. Chem. Phys., 20, 7139–7152, https://doi.org/10.5194/acp-20-7139-2020, 2020.
Petzold, A., Hasselbach, J., Lauer, P., Baumann, R., Franke, K., Gurk, C., Schlager, H., and Weingartner, E.: Experimental studies on particle emissions from cruising ship, their characteristic properties, transformation and atmospheric lifetime in the marine boundary layer, Atmos. Chem. Phys., 8, 2387–2403, https://doi.org/10.5194/acp-8-2387-2008, 2008.
Popovicheva, O., Kireeva, E., Persiantseva, N., Timofeev, M., Bladt, H., Ivleva, N. P., Niessner, R., and Moldanová, J.: Microscopic characterization of individual particles from multicomponent ship exhaust, J. Environ. Monit., 14, 3101–3110, https://doi.org/10.1039/c2em30338h, 2012.
Pratt, K. A. and Prather, K. A.: Mass spectrometry of atmospheric aerosols–recent developments and applications. Part II: On-line mass spectrometry techniques, Mass Spectrom. Rev., 31, 17–48, https://doi.org/10.1002/mas.20330, 2012.
Pratt, K. A., Mayer, J. E., Holecek, J. C., Moffet, R. C., Sanchez, R. O., Rebotier, T. P., Furutani, H., Gonin, M., Fuhrer, K., Su, Y., Guazzotti, S., and Prather, K. A.: Development and Characterization of an Aircraft Aerosol Time-of-Flight Mass Spectrometer, Anal. Chem., 81, 1792–1800, https://doi.org/10.1021/ac801942r, 2009.
Reinard, M. S., Adou, K., Martini, J. M., and Johnston, M. V.: Source characterization and identification by real-time single particle mass spectrometry, Atmos. Environ., 41, 9397–9409, https://doi.org/10.1016/j.atmosenv.2007.09.001, 2007.
Romay, F. J., Roberts, D. L., Marple, V. A., Liu, B. Y. H., and Olson, B. A.: A High-Performance Aerosol Concentrator for Biological Agent Detection, Aerosol Sci. Technol., 36, 217–226, https://doi.org/10.1080/027868202753504074, 2002.
Sakurai, H., Tobias, H. J., Park, K., Zarling, D., Docherty, K. S., Kittelson, D. B., McMurry, P. H., and Ziemann, P. J.: On-line measurements of diesel nanoparticle composition and volatility, Atmos. Environ., 37, 1199–1210, https://doi.org/10.1016/S1352-2310(02)01017-8, 2003.
Schade, J., Passig, J., Irsig, R., Ehlert, S., Sklorz, M., Adam, T., Li, C., Rudich, Y., and Zimmermann, R.: Spatially Shaped Laser Pulses for the Simultaneous Detection of Polycyclic Aromatic Hydrocarbons as well as Positive and Negative Inorganic Ions in Single Particle Mass Spectrometry, Anal. Chem., 91, 10282–10288, https://doi.org/10.1021/acs.analchem.9b02477, 2019.
Seinfeld, J. H. and Pandis, S. N.: Atmospheric Chemistry and Physics: From Air Pollution to Climate Change, 3rd edn., Wiley, s.l., 2185 pp., ISBN: 978-1-118-94740-1, 2016.
Seyler, A., Wittrock, F., Kattner, L., Mathieu-Üffing, B., Peters, E., Richter, A., Schmolke, S., and Burrows, J. P.: Monitoring shipping emissions in the German Bight using MAX-DOAS measurements, Atmos. Chem. Phys., 17, 10997–11023, https://doi.org/10.5194/acp-17-10997-2017, 2017.
Shields, L. G., Suess, D. T., and Prather, K. A.: Determination of single particle mass spectral signatures from heavy-duty diesel vehicle emissions for PM2.5 source apportionment, Atmos. Environ., 41, 3841–3852, https://doi.org/10.1016/j.atmosenv.2007.01.025, 2007.
Sippula, O., Stengel, B., Sklorz, M., Streibel, T., Rabe, R., Orasche, J., Lintelmann, J., Michalke, B., Abbaszade, G., Radischat, C., Gröger, T., Schnelle-Kreis, J., Harndorf, H., and Zimmermann, R.: Particle emissions from a marine engine: chemical composition and aromatic emission profiles under various operating conditions, Environ. Sci. Technol., 48, 11721–11729, https://doi.org/10.1021/es502484z, 2014.
Sofiev, M., Winebrake, J. J., Johansson, L., Carr, E. W., Prank, M., Soares, J., Vira, J., Kouznetsov, R., Jalkanen, J.-P., and Corbett, J. J.: Cleaner fuels for ships provide public health benefits with climate tradeoffs, Nat. Commun., 9, 406, https://doi.org/10.1038/s41467-017-02774-9, 2018.
Song, X.-H., Hopke, P. K., Fergenson, D. P., and Prather, K. A.: Classification of Single Particles Analyzed by ATOFMS Using an Artificial Neural Network, ART-2A, Anal. Chem., 71, 860–865, https://doi.org/10.1021/ac9809682, 1999.
Spencer, M. T., Shields, L. G., Sodeman, D. A., Toner, S. M., and Prather, K. A.: Comparison of oil and fuel particle chemical signatures with particle emissions from heavy and light duty vehicles, Atmos. Environ., 40, 5224–5235, https://doi.org/10.1016/j.atmosenv.2006.04.011, 2006.
Stein, A. F., Draxler, R. R., Rolph, G. D., Stunder, B. J. B., Cohen, M. D., and Ngan, F.: NOAA's HYSPLIT Atmospheric Transport and Dispersion Modeling System, B. Am. Meteorol. Soc., 96, 2059–2077, https://doi.org/10.1175/BAMS-D-14-00110.1, 2015.
Streibel, T., Schnelle-Kreis, J., Czech, H., Harndorf, H., Jakobi, G., Jokiniemi, J., Karg, E., Lintelmann, J., Matuschek, G., Michalke, B., Müller, L., Orasche, J., Passig, J., Radischat, C., Rabe, R., Reda, A., Rüger, C., Schwemer, T., Sippula, O., Stengel, B., Sklorz, M., Torvela, T., Weggler, B., and Zimmermann, R.: Aerosol emissions of a ship diesel engine operated with diesel fuel or heavy fuel oil, Environ. Sci. Pollut. Res. Int., 24, 10976–10991, https://doi.org/10.1007/s11356-016-6724-z, 2017.
Sultana, C. M., Cornwell, G. C., Rodriguez, P., and Prather, K. A.: FATES: a flexible analysis toolkit for the exploration of single-particle mass spectrometer data, Atmos. Meas. Tech., 10, 1323–1334, https://doi.org/10.5194/amt-10-1323-2017, 2017.
Tian, J., Riemer, N., West, M., Pfaffenberger, L., Schlager, H., and Petzold, A.: Modeling the evolution of aerosol particles in a ship plume using PartMC-MOSAIC, Atmos. Chem. Phys., 14, 5327–5347, https://doi.org/10.5194/acp-14-5327-2014, 2014.
Toner, S. M., Sodeman, D. A., and Prather, K. A.: Single Particle Characterization of Ultrafine and Accumulation Mode Particles from Heavy Duty Diesel Vehicles Using Aerosol Time-of-Flight Mass Spectrometry, Environ. Sci. Technol., 40, 3912–3921, https://doi.org/10.1021/es051455x, 2006.
Toner, S. M., Shields, L. G., Sodeman, D. A., and Prather, K. A.: Using mass spectral source signatures to apportion exhaust particles from gasoline and diesel powered vehicles in a freeway study using UF-ATOFMS, Atmos. Environ., 42, 568–581, https://doi.org/10.1016/j.atmosenv.2007.08.005, 2008.
Van Roy, W. and Scheldemann, K.: Results MARPOL Annex VI Monitoring Report Belgian Sniffer campaign 2016, CompMon, available at: https://arkisto.trafi.fi/filebank/a/1482762219/4ba0baf93df900f6ac151919f527e2bc/23540-Results_Belgian_Sniffer_Campagin_2016-consealed.pdf, last access: 23 November 2020), 2016.
Viana, M., Amato, F., Alastuey, A., Querol, X., Moreno, T., García Dos Santos, S., Herce, M. D., and Fernández-Patier, R.: Chemical Tracers of Particulate Emissions from Commercial Shipping, Environ. Sci. Technol., 43, 7472–7477, https://doi.org/10.1021/es901558t, 2009.
Viana, M., Hammingh, P., Colette, A., Querol, X., Degraeuwe, B., de Vlieger, I., and van Aardenne, J.: Impact of maritime transport emissions on coastal air quality in Europe, Atmos. Environ., 90, 96–105, https://doi.org/10.1016/j.atmosenv.2014.03.046, 2014.
Wang, X., Shen, Y., Lin, Y., Pan, J., Zhang, Y., Louie, P. K. K., Li, M., and Fu, Q.: Atmospheric pollution from ships and its impact on local air quality at a port site in Shanghai, Atmos. Chem. Phys., 19, 6315–6330, https://doi.org/10.5194/acp-19-6315-2019, 2019.
Winebrake, J. J., Corbett, J. J., Green, E. H., Lauer, A., and Eyring, V.: Mitigating the Health Impacts of Pollution from Oceangoing Shipping: An Assessment of Low-Sulfur Fuel Mandates, Environ. Sci. Technol., 43, 4776–4782, https://doi.org/10.1021/es803224q, 2009.
Winnes, H., Granberg, M., Magnusson, K., Malmaeus, K., Mellin, A., Stripple, H., Yaramenka, K., and Zhang, Y.: Scrubbers: Closing the loop; Activity 3. Summary, IVL Swedish Environmental Research Institute, available at: https://www.ivl.se/publikationer/publikation.html?id=5737 (last access: 30 November 2020), 2018.
Winnes, H., Fridell, E., and Moldanová, J.: Effects of Marine Exhaust Gas Scrubbers on Gas and Particle Emissions, J. Mar. Sci. Eng., 8, 299, https://doi.org/10.3390/jmse8040299, 2020.
Xiao, Q., Li, M., Liu, H., Fu, M., Deng, F., Lv, Z., Man, H., Jin, X., Liu, S., and He, K.: Characteristics of marine shipping emissions at berth: profiles for particulate matter and volatile organic compounds, Atmos. Chem. Phys., 18, 9527–9545, https://doi.org/10.5194/acp-18-9527-2018, 2018.
Ye, D., Klein, M., Mulholland, J. A., Russell, A. G., Weber, R., Edgerton, E. S., Chang, H. H., Sarnat, J. A., Tolbert, P. E., and Ebelt Sarnat, S.: Estimating Acute Cardiovascular Effects of Ambient PM2.5 Metals, Environ. Health Perspect., 126, 27007, https://doi.org/10.1289/EHP2182, 2018.
Yu, C., Pasternak, D., Lee, J., Yang, M., Bell, T., Bower, K., Wu, H., Liu, D., Reed, C., Bauguitte, S., Cliff, S., Trembath, J., Coe, H., and Allan, J. D.: Characterizing the Particle Composition and Cloud Condensation Nuclei from Shipping Emission in Western Europe, Environ. Sci. Technol., 54, 15604–15612, https://doi.org/10.1021/acs.est.0c04039, 2020.
Zanatta, M., Bozem, H., Köllner, F., Schneider, J., Kunkel, D., Hoor, P., Faria, J. de, Petzold, A., Bundke, U., Hayden, K., Staebler, R. M., Schulz, H., and Herber, A. B.: Airborne survey of trace gases and aerosols over the Southern Baltic Sea: from clean marine boundary layer to shipping corridor effect, Tellus B, 72, 1–24, https://doi.org/10.1080/16000889.2019.1695349, 2020.
Zhang, F., Chen, Y., Tian, C., Wang, X., Huang, G., Fang, Y., and Zong, Z.: Identification and quantification of shipping emissions in Bohai Rim, China, Sci. Total Environ., 497–498, 570–577, https://doi.org/10.1016/j.scitotenv.2014.08.016, 2014.
Zhang, Y., Deng, F., Man, H., Fu, M., Lv, Z., Xiao, Q., Jin, X., Liu, S., He, K., and Liu, H.: Compliance and port air quality features with respect to ship fuel switching regulation: a field observation campaign, SEISO-Bohai, Atmos. Chem. Phys., 19, 4899–4916, https://doi.org/10.5194/acp-19-4899-2019, 2019.
Zhou, F., Hou, L., Zhong, R., Chen, W., Ni, X., Pan, S., Zhao, M., and An, B.: Monitoring the compliance of sailing ships with fuel sulfur content regulations using unmanned aerial vehicle (UAV) measurements of ship emissions in open water, Atmos. Meas. Tech., 13, 4899–4909, https://doi.org/10.5194/amt-13-4899-2020, 2020.
Zhou, Y., Huang, X. H., Griffith, S. M., Li, M., Li, L., Zhou, Z., Wu, C., Meng, J., Chan, C. K., Louie, P. K., and Yu, J. Z.: A field measurement based scaling approach for quantification of major ions, organic carbon, and elemental carbon using a single particle aerosol mass spectrometer, Atmos. Environ., 143, 300–312, https://doi.org/10.1016/j.atmosenv.2016.08.054, 2016.