the Creative Commons Attribution 4.0 License.
the Creative Commons Attribution 4.0 License.
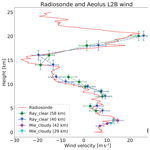
Validation of Aeolus wind products above the Atlantic Ocean
Alina Herzog
Birgit Heese
Kevin Ohneiser
Karsten Hanbuch
Julian Hofer
Zhenping Yin
Ronny Engelmann
Ulla Wandinger
In August 2018, the first Doppler wind lidar in space called Atmospheric Laser Doppler Instrument (ALADIN) was launched on board the satellite Aeolus by the European Space Agency (ESA). Aeolus measures profiles of one horizontal wind component (i.e., mainly the west–east direction) in the troposphere and lower stratosphere on a global basis. Furthermore, profiles of aerosol and cloud properties can be retrieved via the high spectral resolution lidar (HSRL) technique. The Aeolus mission is supposed to improve the quality of weather forecasts and the understanding of atmospheric processes.
We used the opportunity to perform a unique validation of the wind products of Aeolus by utilizing the RV Polarstern cruise PS116 from Bremerhaven to Cape Town in November/December 2018. Due to concerted course modifications, six direct intersections with the Aeolus ground track could be achieved in the Atlantic Ocean west of the African continent. For the validation of the Aeolus wind products, we launched additional radiosondes and used the EARLINET/ACTRIS lidar PollyXT for atmospheric scene analysis. The six analyzed cases prove that Aeolus is able to measure horizontal wind speeds in the nearly west–east direction. Good agreements with the radiosonde observations could be achieved for both Aeolus wind products – the winds observed in clean atmospheric regions called Rayleigh winds and the winds obtained in cloud layers called Mie winds (according to the responsible scattering regime). Systematic and statistical errors of the Rayleigh winds were less than 1.5 and 3.3 m s−1, respectively, when compared to radiosonde values averaged to the vertical resolution of Aeolus. For the Mie winds, a systematic and random error of about 1 m s−1 was obtained from the six comparisons in different climate zones. However, it is also shown that the coarse vertical resolution of 2 km in the upper troposphere, which was set in this early mission phase 2 months after launch, led to an underestimation of the maximum wind speed in the jet stream regions. In summary, promising first results of the first wind lidar space mission are shown and prove the concept of Aeolus for global wind observations.
On 22 August 2018, the European Space Agency (ESA) launched the Earth Explorer Core mission Aeolus. This mission aims to demonstrate significant improvements in weather forecasting by measuring height-resolved wind profiles in the troposphere and lower stratosphere in order to advance the understanding of atmospheric dynamics and climate processes (ESA, 2019a; Straume et al., 2020). The satellite Aeolus has one instrument on board, namely the Atmospheric Laser Doppler Instrument (ALADIN). ALADIN is the first lidar (LIght Detection And Ranging) instrument on a European satellite. It is also the first space-borne instrument capable of measuring vertical profiles of one horizontal wind component on a global basis. Next to wind measurements, aerosol properties can be obtained as a spin-off product (Ansmann et al., 2007; Flamant et al., 2008) via the high spectral resolution lidar (HSRL) technique (Wandinger, 1998; Eloranta, 2005), which is a space-borne novelty as well. Thus, one of the mission goals is to prove the concept of the new technology in space.
For precise weather forecasts, the numerical weather prediction (NWP) models rely on the data assimilation of worldwide meteorological observations. However, the global meteorological observing system does not provide equally distributed wind observations in time and space. The global wind observations that were assimilated at the European Centre for Medium-Range Weather Forecasts (ECMWF) in late 2016 (ECMWF, 2018) are mainly obtained by aircrafts and radiosondes and via atmospheric motion vectors (AMVs). AMVs describe the method of observing the movement of objects (like clouds or water vapor fields) from space and derive the wind velocity from this movement (e.g., Bormann et al., 2003). However, the coverage of AMVs in the lower stratosphere is poor, and there are only a few aircraft and radiosonde measurements in the lower stratosphere. Furthermore, the main input of aircraft measurements is obtained in Europe and the United States of America and is not globally distributed. The global meteorological observing system is therefore suffering from a shortage of observations in specific regions, especially in the Southern Hemisphere, in the lower stratosphere, and over the oceans. The aim of Aeolus is to fill these gaps by providing global horizontal wind profiles at altitudes from 0 to 30 km that are ready for data assimilation in NWP models (Horányi et al., 2015a, b).
Within the German initiative EVAA (Experimental Validation and Assimilation of Aeolus observations; e.g., Baars et al., 2020; Geiß et al., 2019), calibration/validation (CAL/VAL) activities of this space mission have been performed by the Ludwig Maximilian University of Munich (LMU), the Leibniz Institute of Tropospheric Research (TROPOS), the German Meteorological Service (Deutscher Wetterdienst, DWD), and the DLR (Deutsches Zentrum für Luft- und Raumfahrt). The aim of EVAA is to validate the wind and aerosol products of Aeolus and to quantify the benefits of these new measurements for weather forecasting by assimilation experiments.
As one part of these activities, the regular participation of TROPOS on RV Polarstern (Knust, 2017) cruises within the OCEANET project (Macke et al., 2010; Kanitz et al., 2013; Rittmeister et al., 2017; Bohlmann et al., 2018) offered the unique opportunity to perform ground-based validation above the Atlantic Ocean, a region for which only limited observational data are available. The RV Polarstern cruise PS116 from Bremerhaven, Germany, to Cape Town, Republic of South Africa, took place from 10 November 2018 to 11 December 2018 (Hanfland and König, 2019), shortly after the launch of the satellite. Starting in the northern midlatitudes and ending in the southern subtropical region at a latitude of −33.92∘, PS116 covered the northern midlatitude region with frequent westerly winds, the subtropical jet stream region, the trade winds region, and the Intertropical Convergence Zone (ITCZ), before finally ending up in the subtropical region around Cape Town.
The wind validation could be realized using radiosonde launches provided by the German Meteorological Service DWD on RV Polarstern (Schmithüsen, 2019). We also utilized the multiwavelength Raman polarization lidar PollyXT (Engelmann et al., 2016; Baars et al., 2016) in order to characterize the atmospheric state above RV Polarstern, which is part of the European Research Infrastructure EARLINET/ACTRIS (European Aerosol Research Lidar Network/European Research Infrastructure for the Observation of Aerosol, Clouds and Trace Gases).
In 1999, ESA selected the Atmospheric Dynamics Mission (ADM; Stoffelen et al., 2005) as the second Earth Explorer Core mission. The name Aeolus was inspired by the keeper of the wind in Greek mythology (Ingmann and Straume, 2016). ALADIN, the instrument on board, is a high-spectral-resolution (HSR) elastic backscatter lidar with a Nd:YAG laser operating at a wavelength of around 355 nm (Andersson et al., 2008; Reitebuch, 2012; Ingmann and Straume, 2016; Lux et al., 2020; Witschas et al., 2020). The laser pulses are circularly polarized and are emitted with a frequency of 50.5 Hz. The wind profiles are obtained from backscattering processes of the laser light pulses at moving air molecules and particles (Stoffelen et al., 2006; Tan et al., 2008; Reitebuch, 2012; Rennie et al., 2020). The signals are separately detected by two different receiver channels: the Rayleigh channel for backscattering from molecules and the Mie channel for backscattering from particles. As a consequence, two independent wind measurements can be obtained. Furthermore, it provides the possibility to measure the particle extinction and the particle backscatter coefficients independently (Flamant et al., 2017, 2008; Ansmann et al., 2007; Martinet et al., 2018; Flament et al., 2019).
Aeolus has a weekly repeating, polar-sun-synchronous orbit with an inclination of 97∘ and a mean altitude of 320 km (Kanitz et al., 2019a; Straume et al., 2020). One orbit period has a duration of about 90 min (ESA, 2018; Straume et al., 2020; von Bismarck et al., 2019). The ground track velocity is about 7200 m s−1. The line of sight (LOS) describes the field of view in which the backscattered light from the emitted laser pulses can be collected by the lidar telescope. It has an angle of 35∘ versus nadir to be able to measure the horizontal line-of-sight (HLOS) wind velocity. It should be mentioned that this angle changes to 37.6∘ on the measurement ground point due to the earth's curvature (Reitebuch et al., 2014).
Besides in strong convection cases, the vertical wind velocity is small compared to the horizontal wind. Thus, the vertical wind component is neglected when calculating the horizontal wind speed from the Aeolus LOS observations. Furthermore, the LOS is orthogonal to the flight direction in order to minimize the effect of the satellite's velocity on the wind measurements. The orbit is aligned such that Aeolus flies along the day/night border facing towards the night side to minimize the solar background radiation (Kanitz et al., 2019a). Thus, the overpasses are either in the morning (descending orbit) at around 06:00 local solar time or in the evening (ascending orbit) at around 18:00 local solar time. Passing from north to south in the morning, Aeolus' viewing direction has an azimuth angle of around 100∘. This leads to a measurement of the mainly horizontal west–east wind component, having a positive sign for easterly winds along the HLOS. Consequently, the sign is opposite for the Aeolus track from south to north, having an azimuth angle of around 260∘.
For the Aeolus mission, the accumulation of the return signal of 19 outgoing laser pulses is defined as one measurement and corresponds to a horizontal length of ≈2.85 km. One observation is the average of several measurements and aims to be about 30 measurements at ≈87 km horizontal resolution for Rayleigh wind observations. The number of measurements included in one observation can be modified depending on the desired integration length. The receiver has 24 vertical range bins, and wind profiles can be obtained between 0 and 30 km with a vertical resolution between 250 and 2 km (Reitebuch et al., 2014).
Aeolus, i.e., ALADIN, is able to retrieve wind from the Doppler shift caused by particles – these are the so-called Mie winds – but also in clean atmosphere due to the Doppler shift caused by molecules – these are the so-called Rayleigh winds. The technique on board the satellite and the respective algorithms to retrieve the wind are described in Stoffelen et al. (2006), Andersson et al. (2008), Tan et al. (2008), Reitebuch et al. (2009), Reitebuch (2012), Ingmann and Straume (2016), Reitebuch et al. (2014), and Rennie et al. (2020).
Products of Aeolus are delivered at several data levels (Reitebuch et al., 2014; Ingmann and Straume, 2016; Flamant et al., 2017). For the end user, only Level 2 is of interest, which is where all necessary calibration and instrument corrections have been performed. The calibrated and fully processed HLOS wind is delivered in the Level 2B (L2B) data (Rennie et al., 2020; Ingmann and Straume, 2016). This is the main wind product of Aeolus. There are also Level 2C data which are vector wind data resulting from ECMWF model analysis after the assimilation of Level 2B profiles. In Level 2A (L2A), the aerosol and cloud spin-off products (optical properties) are delivered (Ansmann et al., 2007; Flamant et al., 2008, 2017; Flament et al., 2019) but will not be discussed in this paper.
The observational requirements (Ingmann and Straume, 2016) for the Aeolus mission are that the vertical resolution should achieve 500 m in the planetary boundary layer (PBL), 1 km in the troposphere, and 2 km in the lower stratosphere. The requirements for the horizontal integration length per observation depend on the measurement type and altitude. The precision of the HLOS component aims to be 1 m s−1 within the PBL, 2.5 m s−1 for the troposphere, and 3 m s−1 for the lower stratosphere. As the goal is to use the Aeolus observations to improve the weather forecast by data assimilation, the data need to be available within 3 h after the measurement has been taken (Reitebuch et al., 2014).
For the validation of the Aeolus wind products, Level 2B is the product of choice for comparisons with the radiosonde measurements. These are the fully calibrated and processed HLOS winds ready for data assimilation in NWP models. The output of the product includes different classifications and quality parameters which need to be chosen correctly. The use of these parameters is described in the following.
3.1 Atmospheric classification
The Level 2B product provides four separate wind profiles for one atmospheric scene according to the atmospheric classification performed in the processor chain (Rennie et al., 2020). These four wind “types” are as follows.
-
Rayleighclear is wind derived in atmospheric regions without any particle backscatter, thus in clear skies using the Rayleigh methodology.
-
Rayleighcloudy is wind derived from measurements with non-zero particle backscatter, thus in a cloudy or particle-loaded environment using the Rayleigh methodology.
-
Mieclear is wind derived in atmospheric regions with zero particle backscatter using the Mie methodology, which, since in clear-sky conditions no Mie wind should be detectable, is only possible if the classification failed to detect particle backscatter.
-
Miecloudy is wind derived in atmospheric regions with non-zero particle backscatter using the Mie methodology.
Each range bin of the measurements (about 2.85 km horizontal scale) in the observation (87 km horizontal scale equals 30 measurements) is analyzed individually for the atmospheric scene classification (Rennie and Isaksen, 2020). The classification can be done by using the scattering ratio, a feature-finding algorithm, or the particle extinction coefficient as criteria (Rennie et al., 2020). The currently applied method by ESA is the use of the scattering ratio which is determined as part of the Level 1B (L1B) processing (Reitebuch et al., 2014) and used as input for the L2B processing (Rennie et al., 2020; de Kloe et al., 2016). For this, a predefined scattering ratio threshold value as a function of altitude is used. If the scattering ratio is higher than the threshold value, particle scattering is considered to be dominant. Below the threshold, only molecular scattering is assumed. The range bins assigned to the same classification type are accumulated within the corresponding observation. This accumulation of the measurements improves the signal-to-noise ratio and provides a large-scale wind observation which is ready for the NWP data assimilation (Rennie et al., 2020). The Rayleigh and Mie wind retrieval algorithms are then each applied to both classes within the observation. Thus, one observation comprises four different wind types for each range bin, namely Rayleighcloudy, Rayleighclear, Miecloudy, and Mieclear.
To sum up, each observation with a horizontal length of 87 km consists of individual measurements with a horizontal length of about 3 km. Within the observation, the measurements are grouped into the four different classifications, namely Rayleighcloudy, Rayleighclear, Miecloudy, and Mieclear. As the cloud and aerosol situation is usually not homogeneous within the 87 km, only the measurements which are useful for the respective classification are taken into account for the wind retrieval. If, for example, a cloud layer exists in the first 21 km of the observation, the Miecloudy wind product considers only the measurements of these first 21 km. As this procedure is not only applied to the profiles but for each vertical range bin individually, the coordinates of the Aeolus observation profiles given at a certain range bin can be different. While, for example, at 4 km altitude a cloud is observed for the first 21 km, another one is observed at 7 km altitude in the last 30 km of the 87 km horizontal path. Then the coordinates given for the Rayleighcloudy and Miecloudy winds at 4 km altitude are the mean coordinates of the first 21 km, while for 7 km height, the mean coordinates of the last 30 km are used.
To make it even more difficult, in principle, the Mie and Rayleigh wind observations can have a different horizontal resolution. In this work, however, we analyzed early mission data obtained shortly after launch during the commissioning phase of Aeolus, and at this time the horizontal resolution for both Rayleigh and Mie winds was equal and about 87 km. As Miecloudy winds benefit from strong backscatter from cloud particles, the horizontal resolution has in the meantime increased to 12 km due to the significantly higher signal-to-noise ratio of this wind “type”. The Rayleigh horizontal resolution is, however, kept at 87 km.
Two out of this four wind products, namely the Rayleighclear and the Miecloudy winds, are the main targets for the operational use of Aeolus data in NWP. For an accurate Mie wind measurement, a strong particle backscatter is required, whereas the best quality of the Rayleigh measurements is achieved in clear-sky conditions. The Rayleighcloudy products may also deliver usable wind measurements, but contamination of Mie scattering needs to be corrected first, which is still at an experimental stage. Thus, we will use only Rayleighclear and Miecloudy products for our analysis.
3.2 Error threshold and validity flag
The Level 2B product provides a HLOS error estimation for each range bin in the observation profiles. We only consider wind data with errors of less than 8 m s−1 for the Rayleighclear and of less than 5 m s−1 for the Miecloudy winds. These error thresholds are recommendations from ESA/DISC (Aeolus Data Science and Innovation Cluster) (Reitebuch et al., 2019; Stoffelen et al., 2019; Rennie and Isaksen, 2019; Isaksen and Rennie, 2019) for the Aeolus CAL/VAL teams. These thresholds are chosen subjectively based on the compromise between the number of observations that pass quality control and the overall quality of the data set (Rennie and Isaksen, 2020).
The validity flag (de Kloe et al., 2016) considers the validity of the products. Several different technical, instrumental, and retrieving checks account for this flag. It has either the value 1 (valid) or 0 (not valid). We only use Aeolus products with a validity flag of 1.
3.3 Hot pixels
During the commissioning phase of Aeolus, it was noticed that pixels with an increased dark current occurred in the memory zone of both ACCDs (accumulation charge coupled devices) in the detector unit of ALADIN (Reitebuch et al., 2020; Kanitz et al., 2019b). These pixels are called hot pixels, and their increased dark current can have a changing magnitude with time. As no correction procedure was available at the early mission period we focused on, we skipped all height bins in which a hot pixel occurred as they significantly bias the Aeolus wind and aerosol products. For our analyzed data period, these are range bins 2, 13, 16, and 24 of the Mie products and range bins 5, 11, and 15 of the Rayleigh products (note that according to ESA's nomenclature, range bin 1 is the highest and range bin 24 the lowest of the profile). It is worth noting that in the meantime, a hot pixel correction has been in place for Aeolus data since 14 June 2019.
3.4 Observation geometry
As Aeolus provides only the wind along the HLOS, which is mainly the west–east wind component, the radiosonde measurements are projected to the HLOS of Aeolus using the following formula:
where vRS describes the total horizontal wind velocity measured by the radiosonde, φRS is the wind direction measured with the radiosonde, and φAeolus is the azimuth angle of Aeolus which is obtained from the Level 2B data and differs depending on range bin and global position.
The ship-borne validation took place during the RV Polarstern cruise PS116 (10 November 2018 to 11 December 2018) from Bremerhaven, Germany, to Cape Town, Republic of South Africa (Hanfland and König, 2019). Figure 1 shows the ground tracks of Aeolus obtained with ESA's Earth observation Swath and Orbit Visualization (ESOV) tool along the track of the ship (white thick line). Each color indicates a different weekday of the Aeolus overpass. Along the cruise, six points of intersection with the ground tracks of Aeolus within a 150 km radius around RV Polarstern were possible for which additional radiosondes could be launched (yellow circles).
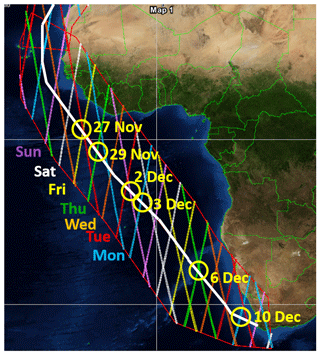
Figure 1Ground tracks of Aeolus (thick colored lines). Each color represents another weekday as indicated in the plot. The thick white line represents the ship track of RV Polarstern. The yellow circles indicate the points of intersection of RV Polarstern and the Aeolus ground track for which additional radiosondes were launched.
Table 1Overview of Aeolus validation cases performed on board RV Polarstern with radiosonde (RS) launches during cruise PS116. The date, the location of RV Polarstern during the RS launch, the launch time, the time of the exact Aeolus overpass, and the distance between the radiosonde and the closest Aeolus wind profile are given. The last column indicates whether Aeolus had an ascending node during local evening or an descending node during local morning.
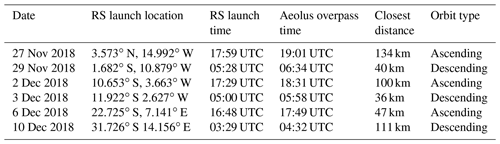
The radius was chosen as a compromise between the number of possible points of intersection and a reasonable limit for the distance between the two individual measurements (radiosonde versus Aeolus profiles). For the wind validation, radiosondes of the type RS41 (Jauhiainen et al., 2014; Jensen et al., 2016) produced by the company Vaisala (Finland) and provided by the German Meteorological Service DWD (Schmithüsen, 2019) with a vertical range up to 30 km were launched 1 h prior to the Aeolus overpass. An overview of the six obtained validation cases for the cruise PS116 is given in Table 1.
For the presented validation, we have used the Aeolus operational product (baseline 2B02 with processor version 03.01) available at the time of the overpass. For this product, a correction of the hot pixels as mentioned above was not yet performed; thus, we skip the respective height bins.
4.1 Case studies for the validation of the Aeolus wind products
In the following, the performance of Aeolus will be discussed intensively by means of two dedicated case studies. The other four validation cases are presented afterwards to provide an overview of the measurements as all are used for a statistical analysis presented in the respective section. An overview of all six validation cases is given in Table 1.
4.1.1 Case study 1: 29 November 2018
In the first case study, the observations of 29 November 2018 (see Fig. 1) which are representative of a tropical wind regime are discussed. For this case, the Aeolus ground track could be reached within a distance of ≈40 km (Table 1) after RV Polarstern had just passed the Equator.
On board RV Polarstern was also the portable multiwavelength Raman polarization lidar PollyXT of the OCEANET facility (Engelmann et al., 2016). With this lidar, aerosol and cloud properties can be classified by shape, size, and absorption behavior (Baars et al., 2016, 2017). The observations with this EARLINET/ACTRIS lidar are used to characterize the atmospheric state above RV Polarstern. Figure 2 shows the temporal evolution of the attenuated backscatter coefficient (calibrated range-corrected signal) to get an impression of the atmospheric scenery for the time around overpass at 06:30 UTC. A cloud layer at around 2 km was observed exactly during the Aeolus overpass (red rectangle). The lidar could not penetrate this optically thick cloud. Below this cloud, the marine boundary layer (BL) was located as indicated by moderate backscattering (green colors). No signal from heights below 400 m can be received due to the incomplete overlap between the receiver field of view and the laser beam of the lidar. Having a look at the period without cloud occurrence after the Aeolus overpass (after ca. 07:25 UTC), an aerosol layer up to around 4 km is visible (greenish-bluish colors).
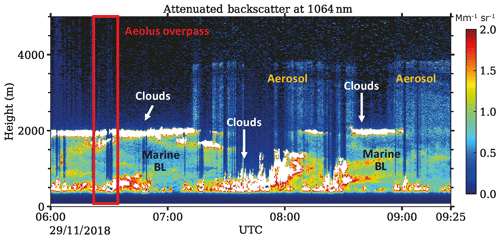
Figure 2Time–height plot of the attenuated backscatter coefficient at 1064 nm of the Raman polarization lidar PollyXT on board RV Polarstern around the time of the Aeolus overpass (red rectangle) on 29 November 2018.
As Aeolus is not nadir-pointing but is taking measurements 35∘ off-nadir, the horizontal distance of the Aeolus observations to RV Polarstern is different for the different heights in the Aeolus wind profile. Also, the radiosonde drifts along the wind direction; thus, the distance between the Aeolus measurements and the radiosonde changes during the ascent. The effect of both is illustrated for this case study in Fig. 3 for the two closest Rayleigh (green and blue) and Mie (purple and cyan) observation profiles. While the horizontal distance to the Miecloudy profiles varies between 10 and 55 km in the lower 5 km (remember the accumulation of measurements within one observation as discussed above), the distance to the Rayleighclear profile has only minor changes. These variations in the distance are not only the result of the radiosonde drift but in particular because of the Aeolus classification algorithm, as discussed above. The mean distances averaged over all heights are those indicated in the legend of Fig. 3.
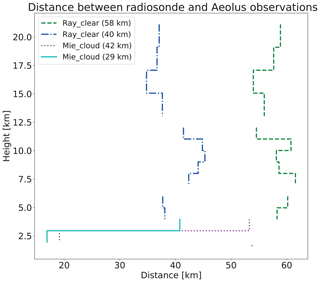
Figure 3The distance between the radiosonde and the geolocation of the single wind observations for the two closest Miecloudy (magenta and cyan) and Rayleighclear (green and blue) profiles.
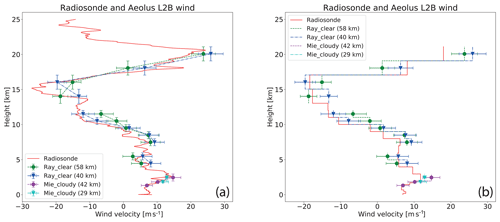
Figure 4Wind velocity profiles measured by the radiosonde (red) with the two closest Aeolus Level 2B (L2B) Rayleighclear (green and blue) and Miecloudy (magenta and cyan) profiles on 29 November 2018. The radiosonde profile is shown with its highest resolution (a) and with an adjusted resolution to the Aeolus range bin width (b). The radiosonde measurements are projected to the HLOS of Aeolus.
Figure 4 shows the HLOS wind velocity profiles measured by the radiosonde (red) and the two closest Aeolus Rayleighclear (green and blue) and Miecloudy (magenta and cyan) profiles. Figure 4a provides the radiosonde profile with its highest vertical resolution, while in Fig. 4b, the vertical resolution of the radiosonde measurements is aggregated in order to match exactly the Aeolus range bin setting. The given distance in the legend of Fig. 4 is the mean distance regarding all range bins. The uncertainty estimation of the radiosonde wind velocity profile is based on calculations of the Global Climate Observing System Reference Upper-Air Network (GRUAN) which estimated an uncertainty between 0.4 and 1 m s−1 for the wind velocity and 1∘ for the wind direction (Dirksen et al., 2014). Even though this reference considers the Vaisala radiosonde type RS92 and not RS41, which was used on RV Polarstern, there is no significant difference in the uncertainty as both radiosonde types are based on the same technique to derive wind velocity and direction (Jensen et al., 2016).
Regarding the Miecloudy profiles in Fig. 4, only measurements at the altitudes of the cloud layer between 1.5 and 2 km (see Fig. 2) were obtained. Below the cloud, ALADIN could not receive any signal as the cloud layer was optically too thick to be penetrated by the laser beam. In Fig. 4a, it can be seen that the Miecloudy measurements are in very good agreement with the radiosonde measurements. Considering the horizontal distance of both observations, one can assume that the cloud observed above RV Polarstern was horizontally homogeneous, as well as the horizontal winds in the lowermost troposphere. In Fig. 4b, a deviation of the adapted low-resolution radiosonde observations to the Miecloudy measurements centered at an altitude of 2.5 km is obvious. The reason for this seems to be the small-scale vertical wind variations observed by the radiosonde (short and rapid decrease in the wind velocity between 2.1 and 2.3 km and a step increase above). The disagreement arises because, with the Aeolus Mie algorithm, the wind speed at cloud top is measured, but, due to the range bin thickness of 1 km, the top height of this cloud cannot be correctly assigned. Thus, the Mie wind speed measured at cloud top is assigned to the center of the 1 km thick range bin disregarding the true top height of the cloud. As a consequence, the agreement to the high-resolution radiosonde profile is much better (almost identical values at 2.5 km) than to the radiosonde profiles binned to Aeolus resolution. The presence of cloud or aerosol layers in the measurement bins was already discussed prior to launch by Sun et al. (2014), and it was shown that biases of more than 0.4 m s−1 can occur when the cloud top is not in the center of the range bin. This statement is confirmed by our observations and shows that a higher vertical resolution is in principle preferable and valuable.
Regarding the Rayleighclear profiles, a good agreement was found for the winds between 4 and 6 km, while a positive bias (systematic error) in the region between 7.5 and 12 km was observed for the two closest observations. Above 12 km, a good agreement is found, considering also the extent of the range bins at this altitude of about 2 km. Below 4 km, no measurements are available due to the low signal-to-noise ratio and the cloud layer at 2.5 km. In summary, the Rayleighclear winds follow well the shape of the wind profile from 4 to 20 km.
Nevertheless, as can be seen in Fig. 4a, the maximum wind velocity occurs just below the tropopause at around 15 km, having an opposite direction (westerly winds) than in the lower troposphere (easterly winds). A maximum absolute wind velocity higher than 25 m s−1 was observed in this height region according to the high-resolution radiosonde profile. However, the Rayleighclear wind measurements of Aeolus are not able to detect such high wind speeds. This is in principle no measurement error of Aeolus. The reason for the disagreement is the coarse range resolution of the Aeolus measurements in the higher troposphere/lower stratosphere at this time of the mission, as can been seen in Fig. 4b. Here it becomes obvious that the resolution is simply too coarse to recognize the strong wind velocity in a vertically narrow atmospheric layer. In this height region, the high-resolution radiosonde wind speed (Fig. 4a) is about 8 m s−1 higher than compared to the radiosonde velocity aggregated to the range bin setting of Aeolus (Fig. 4b).
At that time of the mission, i.e., shortly after the launch, the Aeolus range bins had a resolution of 250 m up to 2 km height to perform necessary ground echo characterizations. Above this height, the vertical resolution was 1 km up to the altitude of 13 km, and then it was set to 2 km for higher altitudes as a consequence of the limitations of 24 range bins in total. Thus, considering the vertical binning, the Aeolus observations are correct, while they miss important information on the tropical jet stream speed as impressively shown here in this one example. As a consequence, the range bins were changed to a resolution of 1 km up to an altitude of 19 km on 26 February 2019 to provide the NWP models with much more detailed wind information in a height region which is very important for weather forecasts while accepting the drawback of an increased random error.
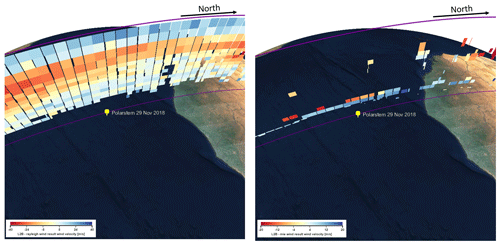
Figure 5Aeolus HLOS winds on 29 November 2018. Rayleighclear (left) and Miecloudy (right) HLOS winds are shown for the overpass around 06:34 UTC. The location of RV Polarstern 800 km off the shore of Liberia is indicated by the yellow pin. The figure was created with the Aeolus visualization tool VirES (https://aeolus.services/).
Figure 5 provides an overview of the Aeolus wind profiles along the ground track during the overpass on 29 November 2018 as visualized with ESA's VirES tool (https://aeolus.services/, last access: 8 May 2020, Santillan et al., 2019). The location of RV Polarstern during the radiosonde launch is indicated by the yellow pin. On the left side, the Rayleighclear wind observations are shown, while on the right side, the Miecloudy observations are plotted.
Noticeable is the good coverage of the Rayleighclear winds at altitudes higher than 3–4 km above sea level (a.s.l.) along the whole track. The pronounced tropical jet with westerly winds as observed by the radiosonde is seen in all Rayleighclear observations as a prominent feature (reddish colors). In the lower troposphere, easterly winds prevail (bluish colors) throughout the whole region. Miecloudy winds are available only in the lowermost 3 km where low-level clouds occurred and sporadically at high altitudes most probably due to the occurrence of cirrus clouds. The Miecloudy winds show steady easterly winds at the cloud layer at around 2.5 km, which is in agreement with the Rayleighclear winds, as discussed above. However, a short statement is needed for the obviously strong westerly winds just above these easterly winds at an altitude of 3 km. These westerly winds are simply an artifact caused by the hot pixel at range bin 13 which was left out in the analysis presented in Fig. 4 but is visualized by VirES in Fig. 5. Thus, these wind measurements at this altitude (indicated by reddish colors just above the bluish colors in the lowermost profile) should be neglected until the hot pixel correction is in place.
4.1.2 Case study 2: 6 December 2018
The second case study discussed in this paper is from 6 December 2018 when RV Polarstern was west of Namibia (see Fig. 1) and thus already in the subtropical region. The radiosonde was launched around 50 km away from the Aeolus ground track.
The lidar observations shown in Fig. 6 indicate no clouds at all but aerosol up to 800 m around the overpass at about 17:50 UTC. Low clouds with a bottom height at around 750 m a.s.l. were observed before 15:00 UTC and after 20:30 UTC.
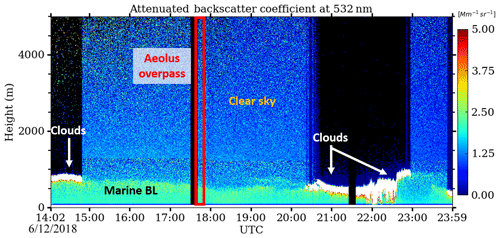
Figure 6Time–height plot of the attenuated backscatter coefficient at 532 nm around the time of the Aeolus overpass (red rectangle) on 6 December 2018.
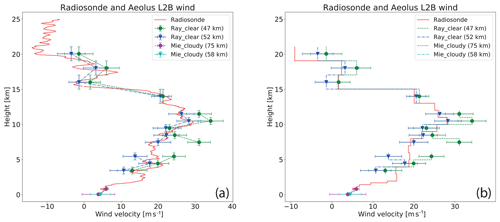
Figure 7Wind velocity profiles measured by the radiosonde (red) with the two closest Aeolus L2B Rayleighclear (green and blue) and Miecloudy (magenta and cyan) profiles on 6 December 2018. The radiosonde profile is shown with its highest resolution (a) and with an adjusted resolution to the Aeolus range bin width (b). The radiosonde measurements are projected to the HLOS of Aeolus.
These clouds might be the reason for the two obtained Miecloudy observations below 1 km a.s.l. as presented in Fig. 7. As described above, if during the 87 km horizontal accumulation distance some measurements are classified as cloudy, a valid Miecloudy wind is obtained for the whole observation. Thus, considering the distance of RV Polarstern to the Aeolus ground track and the Aeolus horizontal resolution, together with the cloud occurrence before and after the overpass as detected with the lidar, it is quite obvious that clouds were partly existent in the Aeolus observational domain and could be used for the Mie wind retrieval.
The winds obtained with the Mie methodology in atmospheric regions classified as cloudy agree perfectly with the HLOS wind obtained from the radiosondes. Also, the vertically aggregated radiosonde velocities as shown in Fig. 7b do match with the Miecloudy winds due to the relatively high vertical resolution of Aeolus in the lowermost 2 km of the atmosphere.
Valid wind observations retrieved with the Rayleigh methodology are available for altitude ranges between 4 and 21 km, having its maximum at an altitude of around 10.5 km. As RV Polarstern crossed the Aeolus ground track in the evening, the positive wind speed values in Fig. 7 indicate westerly winds. Again, the issue concerning the low resolution of Aeolus at higher altitudes is obvious in this comparison. Even though the low-resolved radiosonde measurements fit with the Aeolus ones, the high-resolved radiosonde profile (Fig. 7a) shows many more and stronger changes in wind velocity, e.g., at 17 km height compared to the low-resolution one (Fig. 7b).
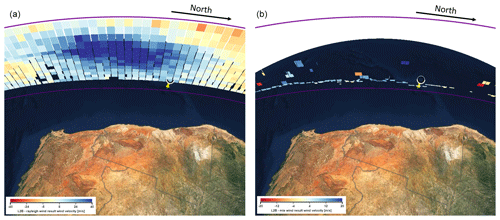
Figure 8Aeolus HLOS winds on 6 December 2018 off the shore of Namibia. Rayleighclear (left) and Miecloudy (right) HLOS winds are shown for the overpass around 17:50 UTC. The location of RV Polarstern is indicated by the yellow pin. The figure was created with the Aeolus visualization tool VirES (https://aeolus.services/).
It is interesting to note that in the case of the Rayleighclear observations, the profile with the further distance (blue line) to RV Polarstern is in better agreement with the radiosonde measurements than the closer one (green line). Especially between 7 and 12 km, it is very similar to the radiosonde profile. Figure 8 shows the wind profiles along the Aeolus track close to RV Polarstern. There was a region with strong winds at higher altitudes just south of the research vessel – namely the subtropical jet. Obviously, there was a significant horizontal (north–south) gradient in high-altitude winds at the time of the overpass, as seen in Fig. 8. Nevertheless, the profile represented by the green line in Fig. 7 was measured more southwardly along the Aeolus track than the “blue profile”. As the radiosonde drifted about 20 km to the north during its ascent, it is a logical consequence that the Aeolus profile measured more northerly (blue) fits better with the radiosonde. Therefore, this analysis confirms that Aeolus is able to capture well horizontal wind gradients at several heights with its Rayleigh (see Fig. 8a) and also Mie techniques (see Fig. 8b).
4.1.3 Case studies 3–6
In order to provide a final overview of the validation cases obtained during the cruise, four remaining overpass cases are presented in Fig. 9. These cases are less favorable than the ones already presented due to larger distances in time and space between the research vessel location and the Aeolus observations, but they are still very valuable for the Aeolus validation in an area where almost no ground-truth observations exist. In addition, they are considered for a statistical analysis presented below.
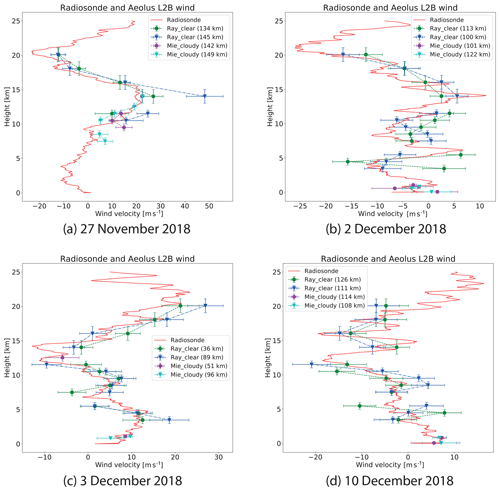
Figure 9Wind velocity profiles measured by the radiosonde (red) with the two closest Aeolus L2B Rayleighclear (green and blue) and Miecloudy (magenta and cyan) profiles of all four remaining validation cases obtained during the RV Polarstern cruise (see Fig. 1).
On 27 November 2018 (Fig. 9a), the overpass region was exactly inside the ITCZ where enhanced vertical turbulence can occur. These vertical velocities have been so far neglected in the retrieval of the Aeolus HLOS, as explained above, and thus might lead to higher errors in the retrieved wind speed. Furthermore, the Aeolus ground track was relatively far away from the position of RV Polarstern (134 and 149 km distance at an altitude of 10 km). Valid Miecloudy measurements were observed at altitudes higher than 8 km due to the existence of high clouds. Considering the large horizontal distance between the radiosonde and Aeolus profiles, as well as the strong convection within the ITCZ, a reasonable agreement is found even though parts of the Miecloudy observations deviate significantly from the radiosonde observations (at around 9 km a.s.l.). The Rayleighclear winds agree in shape with the radiosonde observation, but the Aeolus observation at 14 km differs significantly from the radiosonde. From the available information, it is not possible to conclude if this strong wind speed change within a horizontal distance of 150 km is an atmospheric feature or if there are issues in the Aeolus wind retrievals. For these reasons, we excluded this case from the statistical analysis presented below.
On 2 December 2018 (Fig. 9b), the mean distance between the radiosonde and the Aeolus observations was 100 to 122 km. The radiosonde profile shows a stronger vertical fluctuation in the horizontal wind velocity and direction than in the previously discussed case studies. Especially between 12 to 16 km, large and fast changes in wind direction and thus the projected HLOS were observed by the radiosonde. Due to its coarse vertical resolution, Aeolus is only partly able to detect these rapid changes. Nevertheless, considering the vertical heterogeneity in the wind field, the agreement is acceptable for most Rayleighclear wind observations. Aeolus-derived winds mostly follow the shape and magnitude of the radiosonde winds except for a large deviation at around 4 km (green profile). The reason for that is unclear. Probably the atmospheric classification of Aeolus was not working properly for this scene, and thus cross talk of cloud signals could have led to the deviation in the derived Rayleighclear winds. The observed Miecloudy winds, however, all agree well on this day. Miecloudy winds were observed at around 1 km where partly stratiform clouds were present according to the lidar measurements (not shown). Miecloudy winds could also be retrieved very close to the surface, and they agree very well with the radiosonde observation, taking into account the estimated uncertainty and the distance between the two measurements.
On 3 December 2018 (Fig. 9c), the mean distances between the closest Aeolus profiles and the radiosonde location were less than 100 km. A good agreement between the two measurements was achieved on this day. For the last point of intersection on 10 December 2018 (Fig. 9d), RV Polarstern was more than 100 km away from the Aeolus track. Like in the second case study, the Rayleighclear profile which was further away is partly in better agreement with the radiosonde profile than the closer one. Also, the small-scale structures in wind speed could not be resolved by Aeolus, as discussed above. Nevertheless, within the uncertainty range, a satisfying agreement was achieved for the two last case studies.
4.2 Statistical analysis
In this subsection, the performed comparisons are statistically analyzed. The offset between Aeolus and the radiosonde – the so-called bias – which represents the systematic error of the Aeolus wind measurements is of special interest. For this analysis, the Aeolus wind observation values are plotted against the corresponding values of the radiosondes averaged to the Aeolus height resolution (as discussed above) to focus on the instrumental behavior of Aeolus only. We hereby assume that the atmospheric variability between the two measurements will not cause a bias but will only increase noise, i.e., the random error. Nevertheless, the validation case of 27 November 2018 is not included in the statistics due to the large horizontal distance of the two measurements, together with the fact that the observations were taken directly inside the ITCZ.
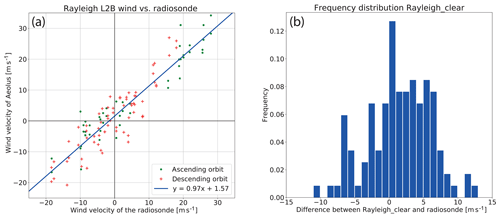
Figure 10(a) The L2B Rayleighclear winds versus the radiosonde measurements made during the ship-borne validation except for 27 November 2018. Green represents measurements of an ascending orbit, while red represents measurements of a descending orbit. (b) Frequency distribution of the difference between Rayleighclear and radiosonde wind speeds for the same data set. Radiosonde data are aggregated to the Aeolus vertical resolution and projected to the HLOS of Aeolus.
The respective correlation plot of the Rayleighclear wind is shown in Fig. 10a, together with the retrieved linear regression. A linear trend between the Aeolus and the radiosonde observations is clearly seen.
The trend line has a slope of 0.97 with an offset (i.e., a bias) of 1.57 m s−1. The different colors indicate whether Aeolus had an ascending node (green) or descending node (red), i.e., if the measurement was taken at local evening or local morning, respectively. This separation is done because the first long-term CAL/VAL activities showed significant differences in the determined biases of Aeolus wind measurements between the two different modes (Rennie and Isaksen, 2020; Geiß et al., 2019; Krisch and the Aeolus DISC, 2020). However, from our observations on board RV Polarstern in the early mission phase of Aeolus, we do not observe a significant difference between the two modes with respect to the Rayleighclear winds.
Figure 10b shows the normalized frequency distribution of the deviation between the Rayleighclear and radiosonde wind observations. When calculating the mean value of this distribution, one gets 1.52 m s−1 as the bias for the Rayleighclear wind observations. If one uses the median of the distribution for the bias calculation, one gets a bias of 1.47 m s−1 which is thus a little less than that calculated from the mean. If one forces the linear regression to have a slope of 1, the retrieved offset is practically the same as the mean deviation between the radiosonde and Aeolus. As this is expected for a Gaussian distribution, one could assume, in accordance with the shape of the distribution shown in Fig. 10b, a normally distributed behavior of the Rayleighclear wind deviations.
To conclude, a bias (systematic error) of 1.47–1.57 m s−1 was derived from the five radiosonde ascents for the Rayleighclear winds regardless of the calculation method. The median absolute deviation (MAD) of the distribution is used to calculate the random error of the Aeolus wind observations (Lux et al., 2020; Witschas et al., 2020) because it is less sensitive to outliers than the standard deviation. It is 67.4 % of the standard deviation, or, the other way around, the scaled MAD (MAD × 1.4826) is identical to the standard deviation for a perfectly Gaussian distribution. The scaled MAD is thus used as an indicator for the random error for Aeolus observations. The MAD is, in the case of the Rayleighclear winds, 3.26 m s−1, and the scaled MAD is correspondingly 4.84 m s−1.
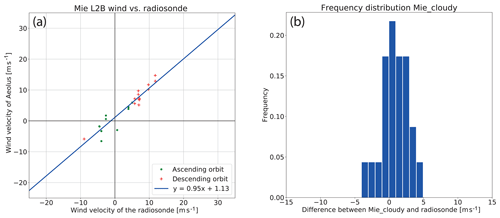
Figure 11(a) L2B Miecloudy winds versus the radiosonde measurements made during the ship-borne validation except for 27 November 2018. (b) Frequency distribution of the difference between Miecloudy and radiosonde wind speeds for the same data set. Radiosonde data are aggregated to the Aeolus vertical resolution and projected to the HLOS of Aeolus.
The same statistics are shown for the Miecloudy winds in Fig. 11. Here a much smaller number of points for comparison could be used as Miecloudy winds are only available at heights where clouds occur. Regardless of the low number of values, it is already obvious that the bias is less than for the Rayleighclear winds. The bias obtained with the linear regression is relatively high at 1.13 compared to the bias obtained from the median and mean (0.95 and 0.95 m s−1, respectively) of the frequency distribution of the differences between Aeolus and the radiosonde (Fig. 11b). Nevertheless, forcing the slope to be unity, the same offset (i.e., bias or systematic error) as for the mean of the distribution is retrieved, which again confirms that the deviations of Miecloudy winds are normally (Gaussian) distributed. As for the Rayleighclear winds, no difference in the Aeolus performance between ascending and descending orbits is found (Fig. 11a).
Figure 11b shows that the absolute deviations are much lower than for the Rayleighclear winds, which is reflected in the much smaller MAD of 1.06 m s−1 (corresponding to a scaled MAD of 1.58 m s−1 ). All absolute deviations are below 5 m s−1 compared to values up to 13 m s−1 for the Rayleighclear winds. This is mainly caused by the generally lower signal-to-noise ratio (SNR) of the Rayleigh return signal compared to the Mie channel, in addition to the different measurement and retrieval techniques. Thus, one can conclude for a user's perspective that Miecloudy winds are more accurate than Rayleighclear winds (less systematic and less random error) and should be used if available. The Rayleighclear winds, on the other hand, provide a better coverage of the atmosphere.
Table 2Overview of derived biases (systematic errors), the median absolute deviation (MAD), and the scaled MAD (which is an indicator for the random error) for both the Rayleighclear and Miecloudy winds obtained from the comparison with the five radiosonde launches. All values are in meters per second (m s−1).
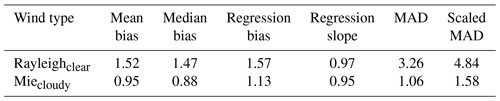
An overview of the derived values for the systematic and random errors of Aeolus from the ship-borne validation is given in Table 2. The systematic and random errors observed are higher than those demanded in the mission requirements (Ingmann and Straume, 2016). The aim is to achieve a systematic error of less than 0.7 m s−1 with an overall precision of 1 m s−1 in the PBL, 2.5 m s−1 in the troposphere, and 3–5 m s−1 in the stratosphere. Despite the fact that the mission requirements could not yet be achieved, the mission can be seen as a success as it has already demonstrated that winds are globally observable from space by active remote sensing with sufficient quality to achieve a positive impact in NWP (Rennie and Isaksen, 2020; Martin et al., 2020). However, it is worth mentioning again that the Aeolus data which were used are not yet the finalized data set for this space mission. Meanwhile, it was found that slight temperature variations over the receiving telescope area are one of the main reasons for biases in the Rayleigh winds (Rennie and Isaksen, 2020; Krisch and the Aeolus DISC, 2020; Reitebuch et al., 2020). This effect and some other instrumental challenges, like the hot pixels issue, have not yet been compensated for in the data of the early mission stage. Processor updates with several improvements have been taking place in the meantime, and more are expected in the future to correct such effects, after which a reprocessing of the early Aeolus data set is foreseen.
Considering that only five radiosonde launches were used, the observed biases are in agreement with other CAL/VAL teams of this mission (ESA, 2019b). At the first Aeolus CAL/VAL workshop, independent comparisons (not publicly accessible) of several CAL/VAL teams showed global biases in the range of less than 1 up to 3.3 m s−1 using different observation periods and NWP models (e.g. Rennie and Isaksen, 2019).
Lux et al. (2020) compared the Aeolus Rayleighclear wind observations to winds measured with the airborne demonstrator during WindVal III in central Europe from 17 November to 5 December 2018 and thus in the same time period as the validation measurements obtained on board RV Polarstern. The authors also validated these winds against wind data from the ECMWF model. They report a bias of 1.6 m s−1 with random errors of 2.5 m s−1 (comparison against ECMWF model winds) and a 2.53 m s−1 bias and 3.57 m s−1 random error for the comparison against the airborne demonstrator.
Witschas et al. (2020) reported slightly different biases (systematic errors) of 2.1 and 2.3 m s−1 for Rayleighclear and Miecloudy winds during the same campaign (WindVal III) using an airborne 2 µm Doppler wind lidar. Random errors were about 4 and 2.2 m s−1 for Rayleighclear and Miecloudy, respectively, in this study. For another campaign, namely AVATARE (Aeolus Validation Through Airborne Lidars in Europe) in central Europe in spring 2019, however, the authors found negative biases of −4.6 m s−1 for Rayleighclear winds and −0.2 m s−1 for Miecloudy and an increased random error of 4.4 and 2.2 m s−1 for Rayleighclear and Miecloudy, respectively. They conclude that the shift in the bias could be a result of the inadequate and constant calibration used during the L2B processing until 16 May 2019 not accounting for instrumental drifts that were observed since launch (Reitebuch et al., 2020). Meanwhile, the calibration has been updated more regularly, and instrument drifts are under investigation to be corrected in future processor updates. For further details, the reader may refer to the stated references.
Khaykin et al. (2020) analyzed one wind profile of Aeolus with the Doppler lidar at Observatoire de Haute-Provence and found a good agreement between the two measurements, but below 5 km a.g.l. (above ground level), a stronger deviation was observed which was considered to be caused by horizontal heterogeneity of the atmosphere. In our study, however, we could almost never observe any Rayleighclear wind profile below 4 km, which prohibits the discussion of this issue raised by Khaykin et al. (2020). Nevertheless, it could already be an indicator that the laser energy, which has been lower than expected (Kanitz et al., 2019b; Reitebuch et al., 2020), leads to less accuracy and therefore more invalid wind observations close to the ground (further away from the lidar on board Aeolus).
To summarize, the statistics obtained during cruise PS116 with RV Polarstern, even if only consisting of five comparisons with radiosondes, do agree well with findings from other CAL/VAL teams and give an insight into the Aeolus performance shortly after launch – thus still in the commissioning phase. It also shows that Aeolus is able to measure horizontal wind speeds from space and that the retrieved data are reliable within a given uncertainty range and thus ready for data assimilation. The first data assimilation experiments have already shown a positive impact, e.g., as announced by ECMWF (ECMWF, 2019a, b).
Wind products from the first wind lidar in space, ALADIN, on board the European satellite Aeolus were validated against wind profiles obtained from radiosonde launches on board the German RV Polarstern during cruise PS116 in autumn 2018 across the Atlantic Ocean. Six points of intersection were reached within a radius of 150 km for which additional radiosondes could be launched in time. These unique validation measurements across the Atlantic Ocean are a valuable contribution to the – until now – mainly model-based validations of Aeolus in that region of the Earth.
With the analysis of dedicated case studies, it was shown that Aeolus is able to measure accurately atmospheric wind profiles of the nearly west–east wind component. Due to its HSRL technique, Aeolus is able to measure wind speed in both clear, particle-free atmospheric regions and regions where clouds or dense aerosol layers occur. The corresponding products are the Rayleighclear and Miecloudy winds, respectively.
Aeolus, i.e., ALADIN, is able to obtain the shape of the wind profile and the magnitude of the wind speed with sufficient accuracy, also taking into account the representativeness error introduced by the horizontal distance between the radiosonde and Aeolus ground track and the low horizontal (87 km) and vertical resolution (0.5–2 km) of Aeolus. A proof of concept of the HSR Doppler wind lidar technique in space to measure global wind profiles was therefore already demonstrated. Nevertheless, it was also shown that the height resolution which was set during the commissioning phase was not sufficient to capture events of strong vertical wind shear such as near the tropical jet stream. The coarse resolution of Aeolus of 2 km at altitudes above 13 km caused a significant underestimation of the maximum wind speed. Thus, considering the vertical binning, the Aeolus observations were correct, but important information on the tropical jet stream speed was missing. As a consequence, the range bins were changed to a resolution of 1 km up to an altitude of 19 km on 26 February 2019 to provide the NWP models with much more detailed wind information in such an important atmospheric region.
It has also been discussed that Rayleighclear winds in the free troposphere have a larger offset, i.e., systematic error, than the corresponding Miecloudy winds, leading to a slight overestimation of the true HLOS wind speed. Miecloudy winds are only available at atmospheric regions with clouds, but the comparison to the radiosonde profiles shows that the Miecloudy winds were very accurate with lower systematic and random errors than the Rayleighclear winds and should be used when available in favor of the Rayleighclear winds. Nevertheless, the Rayleighclear winds especially are a highlight of the Aeolus mission as they could close a gap for clear-air observations in the global atmospheric observing system which is not covered by atmospheric motion vectors obtained in cloudy regions only. In fact, Rayleighclear winds have proven more beneficial for NWP than Miecloudy winds so far (Rennie and Isaksen, 2020).
The statistical analysis based on only five radiosondes reveals the good performance of Aeolus in this early phase of the mission, having a systematic error (bias) of about 1.5 m s−1 and random error of 4.84 m s−1 (scaled MAD) for the Rayleighclear winds. The Miecloudy winds were more accurate with about 1 m s−1 systematic error and a random error of 1.58 m s−1. This is yet higher than claimed in the mission requirements, but it should be noted that the data used for validation here are not the final wind data set of Aeolus. Some instrumental effects and calibration imperfections discovered in the meantime were not considered in the retrieval algorithms used for the autumn 2018 data set. The main challenges of the Aeolus mission are the occurrence of hot pixels, varying telescope temperatures, the laser energy development, and the lower atmospheric return signal resulting in a larger Rayleigh random error. ESA is steadily working on the improvements of the wind retrievals and processor updates. Several reprocessing steps of the existing data will take place in the future delivering data with even higher accuracy than the data set delivered in the commissioning phase of Aeolus. Once a final reprocessing has taken place, it could be worthwhile to use the existing RV Polarstern data set to quantify the improvements of the algorithm updates.
To summarize, the validation efforts performed with radiosondes launched during cruise PS116 of RV Polarstern give an insight into Aeolus' performance shortly after launch and thus still in its commissioning phase. It shows that Aeolus is able to measure one horizontal wind component from space and that the retrieved data are reliable within a given uncertainty range and usable for data assimilation. As announced by ECMWF (ECMWF, 2019b), the first data assimilation experiments have already shown a positive impact. For such experiments, the systematic errors obtained during the CAL∕VAL efforts are a prerequisite because they need to be corrected and show the importance of independent CAL/VAL activities. Since the beginning of 2020, Aeolus data have even been operationally assimilated at ECMWF (ECMWF, 2020a), and a positive impact on weather prediction has been demonstrated (Rennie and Isaksen, 2019; Isaksen and Rennie, 2019). The recent global shutdown due to the COVID-19 epidemic has even shown that Aeolus is able to partly replace the missing aircraft measurements in the global data assimilation system (ECMWF, 2020b).
Radiosonde data are available at the PANGAEA Data Center: https://doi.org/10.1594/PANGAEA.903888 (Schmithüsen, 2019). The Aeolus data set used in this publication is not publicly available, but, after reprocessing in the future, an updated data set will become publicly available via ESA services.
All authors have contributed to the paper preparation. HB and AH have performed the data analysis with the support of ZY, RE, and JH. AH, KH, and KO performed the measurements on board RV Polarstern. UW and BH have contributed to the discussion with their expertise in remote sensing and meteorology. HB has led the paper preparation based on the master thesis of AH.
The authors declare that they have no conflict of interest.
The presented work includes preliminary data (not fully calibrated/validated and not yet publicly released) of the Aeolus mission that is part of the European Space Agency (ESA) Earth Explorer Program. This includes wind products from before the public data release in May 2020 and/or aerosol and cloud products which have not yet been publicly released. The preliminary Aeolus wind products will be reprocessed during 2020 and 2021, which will include in particular a significant L2B product wind bias reduction and improved L2A radiometric calibration. Aerosol and cloud products will become publicly available by spring 2021. The processor development, improvement, and product reprocessing preparation are performed by the Aeolus DISC (Data, Innovation and Science Cluster), which involves DLR, DoRIT, ECMWF, KNMI, CNRS, S&T, ABB, and Serco, in close cooperation with the Aeolus PDGS (Payload Data Ground Segment). The analysis has been performed in the frame of the Aeolus Scientific Calibration and Validation Team (ACVT).
The authors also acknowledge support through ACTRIS-2 under grant agreement no. 654109 from the European Union's Horizon 2020 research and innovation program. We thank the Alfred-Wegener Institute (AWI), the RV Polarstern crew, and the cruise leaders Claudia Hanfland and Bjela König for their incredible effort in making those measurements possible (acknowledgment no. AWI_PS116_00). Furthermore, we thank Holger Schmithüsen (AWI) and the German Meteorological Service (DWD) for the support in launching the radiosondes during the cruise. We also appreciate very much the fruitful discussions within the EVAA consortium (LMU, DWD, DLR) and with ESA.
This research has been supported by the German Federal Ministry for Economic Affairs and Energy (BMWi) (grant no. 50EE1721C).
The publication of this article was funded by the
Open-Access Fund of the Leibniz Association.
This paper was edited by Ad Stoffelen and reviewed by two anonymous referees.
Andersson, E., Dabas, A., Endemann, M., Ingmann, P., Källén, E., Offiler, D., and Stoffelen, A.: ADM-Aeolus Science Report, available at: https://earth.esa.int/documents/10174/1590943/AEOL002.pdf (last access: 8 May 2020), 2008. a, b
Ansmann, A., Wandinger, U., Le Rille, O., Lajas, D., and Straume, A. G.: Particle backscatter and extinction profiling with the spaceborne high-spectral-resolution Doppler lidar ALADIN: methodology and simulations, Appl. Optics, 46, 6606–6622, https://doi.org/10.1364/AO.46.006606, 2007. a, b, c
Baars, H., Kanitz, T., Engelmann, R., Althausen, D., Heese, B., Komppula, M., Preißler, J., Tesche, M., Ansmann, A., Wandinger, U., Lim, J.-H., Ahn, J. Y., Stachlewska, I. S., Amiridis, V., Marinou, E., Seifert, P., Hofer, J., Skupin, A., Schneider, F., Bohlmann, S., Foth, A., Bley, S., Pfüller, A., Giannakaki, E., Lihavainen, H., Viisanen, Y., Hooda, R. K., Pereira, S. N., Bortoli, D., Wagner, F., Mattis, I., Janicka, L., Markowicz, K. M., Achtert, P., Artaxo, P., Pauliquevis, T., Souza, R. A. F., Sharma, V. P., van Zyl, P. G., Beukes, J. P., Sun, J., Rohwer, E. G., Deng, R., Mamouri, R.-E., and Zamorano, F.: An overview of the first decade of PollyNET: an emerging network of automated Raman-polarization lidars for continuous aerosol profiling, Atmos. Chem. Phys., 16, 5111–5137, https://doi.org/10.5194/acp-16-5111-2016, 2016. a, b
Baars, H., Seifert, P., Engelmann, R., and Wandinger, U.: Target categorization of aerosol and clouds by continuous multiwavelength-polarization lidar measurements, Atmos. Meas. Tech., 10, 3175–3201, https://doi.org/10.5194/amt-10-3175-2017, 2017. a
Baars, H., Geiß, A., Wandinger, U., Herzog, A., Engelmann, R., Bühl, J., Radenz, M., Seifert, P., Althausen, D., Heese, B., Ansmann, A., Martin, A., Leinweber, R., Lehmann, V., Weissmann, M., Cress, A., Filioglou, M., Komppula, M., and Reitebuch, O.: First results from the German Cal/Val activities for Aeolus, EPJ Web Conf., 237, 01008, https://doi.org/10.1051/epjconf/202023701008, 2020. a
Bohlmann, S., Baars, H., Radenz, M., Engelmann, R., and Macke, A.: Ship-borne aerosol profiling with lidar over the Atlantic Ocean: from pure marine conditions to complex dust–smoke mixtures, Atmos. Chem. Phys., 18, 9661–9679, https://doi.org/10.5194/acp-18-9661-2018, 2018. a
Bormann, N., Saarinen, S., Kelly, G., and Thèpaut, J.-N.: The Spatial Structure of Observation Errors in Atmospheric Motion Vectors from Geostationary Satellite Data, Mon. Weather Rev., 131, 706–718, https://doi.org/10.1175/1520-0493(2003)131<0706:TSSOOE>2.0.CO;2, 2003. a
de Kloe, J., Stoffelen, A., Tan, D., Andersson, E., Rennie, M., Dabas, A., Poli, P., and Huber, D.: ADM-Aeolus Level-2B/2C Processor Input/Output Data Definitions Interface Control Document, available at: https://earth.esa.int/pi/esa?type=file&table=aotarget&cmd=image&alias=ADM_Aeolus_L2B_Input_Output_DD_ICD (last access: 8 May 2020), 2016. a, b
Dirksen, R. J., Sommer, M., Immler, F. J., Hurst, D. F., Kivi, R., and Vömel, H.: Reference quality upper-air measurements: GRUAN data processing for the Vaisala RS92 radiosonde, Atmos. Meas. Tech., 7, 4463–4490, https://doi.org/10.5194/amt-7-4463-2014, 2014. a
ECMWF: Improving forecasts with new wind data from ESA's Aeolus mission, available at: https://www.ecmwf.int/en/about/media-centre/science-blog/2018/improving-forecasts-new-wind-data-esas-aeolus-mission (last access: 8 May 2020), 2018. a
ECMWF: Aeolus data impact tests confirm potential of new wind data for NWP, available at: https://www.ecmwf.int/en/about/media-centre/news/2019/aeolus-data-impact-tests-confirm-potential-new-wind-data-nwp (last access: 8 May 2020), 2019a. a
ECMWF: Tests show positive impact of new Aeolus wind data on forecasts, available at: https://www.ecmwf.int/en/about/media-centre/news/2019/tests-show-positive-impact-new-aeolus-wind-data-forecasts (last access: 8 May 2020), 2019b. a, b
ECMWF: ECMWF starts assimilating Aeolus wind data, available at: https://www.ecmwf.int/en/about/media-centre/news/2020/ecmwf-starts-assimilating-aeolus-wind-data (last access: 8 May 2020), 2020a. a
ECMWF: Drop in aircraft observations could have impact on weather forecasts, available at: https://www.ecmwf.int/en/about/media-centre/news/2020/drop-aircraft-observations-could-have-impact-weather-forecasts (last access: 8 May 2020), 2020b. a
Eloranta, E. W.: High Spectral Resolution Lidar, in: Lidar: Range-Resolved Optical Remote Sensing of the Atmosphere, edited by: Weitkamp, C., Springer, 143–163, https://doi.org/10.1007/b106786, 2005. a
Engelmann, R., Kanitz, T., Baars, H., Heese, B., Althausen, D., Skupin, A., Wandinger, U., Komppula, M., Stachlewska, I. S., Amiridis, V., Marinou, E., Mattis, I., Linné, H., and Ansmann, A.: The automated multiwavelength Raman polarization and water-vapor lidar PollyXT: the neXT generation, Atmos. Meas. Tech., 9, 1767–1784, https://doi.org/10.5194/amt-9-1767-2016, 2016. a, b
ESA: Aeolus Mission Summary, available at: https://earth.esa.int/web/guest/missions/esa-operational-eo-missions/aeolus/mission-summary (last access: 8 May 2020), 2018. a
ESA: Introducing Aeolus, available at: https://www.esa.int/Our_Activities/Observing_the_Earth/Aeolus/Introducing_Aeolus (last access: 8 May 2020), 2019a. a
ESA: Aeolus CAL/VAL and Science Workshop, 26–29 March 2019, Frascati (Rome), Italy, available at: https://nikal.eventsair.com/QuickEventWebsitePortal/aeolus-calval-and-science-workshop-2019/aeolus (last access: 8 May 2020), 2019b. a
Flamant, P., Cuesta, J., Denneulin, M.-L., Dabas, A., and Huber, D.: ADM-Aeolus retrieval algorithms for aerosol and cloud products, Tellus A, 60, 273–286, https://doi.org/10.1111/j.1600-0870.2007.00287.x, 2008. a, b, c
Flamant, P. H., Lever, V., Martinet, P., Flament, t., Cuesta, J., Dabas, A., Olivier, M., and Huber, D.: ADM-Aeolus L2A Algorithm Theoretical Baseline Document, Particle spin-off products, available at: https://earth.esa.int/pi/esa?type=file&table=aotarget&cmd=image&alias=ADM_Aeolus_L2A_Algorithm_TBD (last access: 8 May 2020), 2017. a, b, c
Flament, T., Stieglitz, H., Dabas, A., and Huber, D.: Aeolus L2A aerosol products: principle and first glimpse on performances, in: ESA Living Planet Symposium 2019, 13–17 May 2019, Milan, Italy, available at: https://lps19.esa.int/NikalWebsitePortal/living-planet-symposium-2019/lps19/Agenda/AgendaItemDetail?id=1a3d272c-f7d1-4847-b1c4-08c452f9405f (last access: 8 May 2020), 2019. a, b
Geiß, A., Lehmann, V., Leinweber, R., Reitebuch, O., and Weissmann, M.: Methodology and Case Studies for the Validation of Aeolus Observations by means of Radar Wind Profilers, in: ESA Living Planet Symposium 2019, 13–17 May 2019, Milan, Italy, available at: https://lps19.esa.int/NikalWebsitePortal/living-planet-symposium-2019/lps19/Agenda/AgendaItemDetail?id=64570099-bea7-4b8f-a54b-5b6ad81fa342 (last access: 8 May 2020), 2019. a, b
Hanfland, C. and König, B.: The Expedition PS116 of the Research Vessel POLARSTERN to the Atlantic Ocean in 2018, https://doi.org/10.2312/BzPM_0731_2019, 2019. a, b
Horányi, A., Cardinali, C., Rennie, M., and Isaksen, L.: The assimilation of horizontal line-of-sight wind information into the ECMWF data assimilation and forecasting system. Part I: The assessment of wind impact, Q. J. Roy. Meteor. Soc., 141, 1223–1232, https://doi.org/10.1002/qj.2430, 2015a. a
Horányi, A., Cardinali, C., Rennie, M., and Isaksen, L.: The assimilation of horizontal line-of-sight wind information into the ECMWF data assimilation and forecasting system. Part II: The impact of degraded wind observations, Q. J. Roy. Meteor. Soc., 141, 1233–1243, https://doi.org/10.1002/qj.2551, 2015b. a
Ingmann, P. and Straume, A. G.: ADM-Aeolus Mission Requirements Document, available at: https://earth.esa.int/pi/esa?type=file&table=aotarget&cmd=image&alias=Aeolus_MRD (last access: 8 May 2020), 2016. a, b, c, d, e, f, g
Isaksen, L. and Rennie, M.: A preliminary evaluation of using Aeolus L2B Winds in ECMWF's NWP system, with focus on the tropical region, in: ESA Living Planet Symposium 2019, 13–17 May 2019, Milan, Italy, available at: https://lps19.esa.int/NikalWebsitePortal/living-planet-symposium-2019/lps19/Agenda/AgendaItemDetail?id=64570099-bea7-4b8f-a54b-5b6ad81fa342 (last access: 8 May 2020), 2019. a, b
Jauhiainen, H., Survo, P., Lehtinen, R., and Lentonen, J.: Radiosonde RS41 and RS92 key differences and comparison test results in different locations and climates, in: TECO-2014, WMO Technical Conference on Meteorological and Environmental Instruments and Methods of Observations, 7–9 July 2014, Saint Petersburg, Russian Federation, available at: https://www.wmo.int/pages/prog/www/IMOP/publications/IOM-116_TECO-2014/Session 3/P3_16_Juhiainen_Radiosonde_RS41_RS92_Key_Differences_Comparison_TestResults.pdf (last access: 8 May 2020), 2014. a
Jensen, M. P., Holdridge, D. J., Survo, P., Lehtinen, R., Baxter, S., Toto, T., and Johnson, K. L.: Comparison of Vaisala radiosondes RS41 and RS92 at the ARM Southern Great Plains site, Atmos. Meas. Tech., 9, 3115–3129, https://doi.org/10.5194/amt-9-3115-2016, 2016. a, b
Kanitz, T., Ansmann, A., Seifert, P., Engelmann, R., Kalisch, J., and Althausen, D.: Radiative effect of aerosols above the northern and southern Atlantic Ocean as determined from shipborne lidar observations, J. Geophys. Res.-Atmos., 118, 12556–12565, https://doi.org/10.1002/2013JD019750, 2013. a
Kanitz, T., Lochard, J., Marshall, J., McGoldrick, P., Lecrenier, O., Bravetti, P., Reitebuch, O., Rennie, M., Wernham, D., and Elfving, A.: Aeolus first light: first glimpse, in: International Conference on Space Optics – ICSO 2018, 9–12 October 2018, Chania, Greece, vol. 11180, 659–664, https://doi.org/10.1117/12.2535982, 2019a. a, b
Kanitz, T., Straume, A. G., Marshall, J., Lecrenier, O., Sachhieri, V., Reitebuch, O., Rennie, M., and Wernham, D.: Aeolus – 1 Year After Launch, in: IGARSS 2019 – 2019 IEEE International Geoscience and Remote Sensing Symposium, 28 July–2 August 2019, Yokohama, Japan, 4769–4770, https://doi.org/10.1109/IGARSS.2019.8898100, 2019b. a, b
Khaykin, S. M., Hauchecorne, A., Wing, R., Keckhut, P., Godin-Beekmann, S., Porteneuve, J., Mariscal, J.-F., and Schmitt, J.: Doppler lidar at Observatoire de Haute-Provence for wind profiling up to 75 km altitude: performance evaluation and observations, Atmos. Meas. Tech., 13, 1501–1516, https://doi.org/10.5194/amt-13-1501-2020, 2020. a, b
Knust, R.: Polar research and supply vessel POLARSTERN operated by the Alfred-Wegener-Institute, J. Large-Scale Res. Fac., 3, A119, https://doi.org/10.17815/jlsrf-3-163, 2017. a
Krisch, I. and the Aeolus DISC: Data quality of Aeolus wind measurements, EGU General Assembly 2020, Online, 4–8 May 2020, EGU2020-9471, https://doi.org/10.5194/egusphere-egu2020-9471, 2020. a, b
Lux, O., Lemmerz, C., Weiler, F., Marksteiner, U., Witschas, B., Rahm, S., Geiß, A., and Reitebuch, O.: Intercomparison of wind observations from the European Space Agency's Aeolus satellite mission and the ALADIN Airborne Demonstrator, Atmos. Meas. Tech., 13, 2075–2097, https://doi.org/10.5194/amt-13-2075-2020, 2020. a, b, c
Macke, A., Kalisch, J., Zoll, Y., and Bumke, K.: Radiative effects of the cloudy atmosphere from ground and satellite based observations, EPJ Web of Conferences, 9, 83–94, https://doi.org/10.1051/epjconf/201009006, 2010. a
Martin, A., Geiss, A., Cress, A., and Weissmann, M.: Experimental Validation and Assimilation of Aeolus Wind Observations, EGU General Assembly 2020, Online, 4–8 May 2020, EGU2020-18186, https://doi.org/10.5194/egusphere-egu2020-18186, 2020 a
Martinet, P., Flament, T., and Dabas, A.: Aerosol optical properties retrieved from the future space lidar mission ADM-aeolus, in: 28th International Laser Radar Conference (ILRC 28), 25–30 June 2017, Bucharest, Romania, EDP Sciences, vol. 176, 02019, https://doi.org/10.1051/epjconf/201817602019, 2018. a
Reitebuch, O.: The Spaceborne Wind Lidar Mission ADM-Aeolus, in: Atmospheric Physics: Background – Methods – Trends, edited by: Schumann, U., Springer, 815–827, https://doi.org/10.1007/978-3-642-30183-4_49, 2012. a, b, c
Reitebuch, O., Lemmerz, C., Nagel, E., Paffrath, U., Durand, Y., Endemann, M., Fabre, F., and Chaloupy, M.: The Airborne Demonstrator for the Direct-Detection Doppler Wind Lidar ALADIN on ADM-Aeolus. Part I: Instrument Design and Comparison to Satellite Instrument, J. Atmos. Ocean. Tech., 26, 2501–2515, https://doi.org/10.1175/2009JTECHA1309.1, 2009. a
Reitebuch, O., Huber, D., and Nikolaus, I.: Algorithm Theoretical Basis Document ATBD: ADM-Aeolus Level 1B Products, available at: https://earth.esa.int/pi/esa?type=file&table=aotarget&cmd=image&alias=ADM_Aeolus_L1B_Algorithm_TBD (last access: 8 May 2020), 2014. a, b, c, d, e, f
Reitebuch, O., Marksteiner, U., Weiler, F., Lemmerz, C., Witschas, B., Lux, O., Meringer, M., Schmidt, K., Huber, D., Dabas, A., Flament, T., Stieglitz, H., Mahfouf, J.-F., Isaksen, L., Rennie, M., Stoffelen, A., Marseille, G.-J., de Kloe, J., Donovan, D., Perron, G., Jupin-Langlois, S., Smeets, J., Niemeijer, S., and Di Lodovico, I.: The Aeolus Data Innovation and Science Cluster DISC – Overview and First Results, in: ESA Living Planet Symposium 2019, 13–17 May 2019, Milan, Italy, available at: https://lps19.esa.int/NikalWebsitePortal/living-planet-symposium-2019/lps19/Agenda/AgendaItemDetail?id=1a3d272c-f7d1-4847-b1c4-08c452f9405f (last access: 8 May 2020), 2019. a
Reitebuch, O., Lemmerz, C., Lux, O., Marksteiner, U., Rahm, S., Weiler, F., Witschas, B., Meringer, M., Schmidt, K., Huber, D., Nikolaus, I., Geiß, A., Vaughan, M., Dabas, A., Flament, T., Stieglitz, H., Isaksen, L., Rennie, M., de Kloe, J., Marseille, G.-J., Stoffelen, A., Wernham, D., Kanitz, T., Straume, A.-G., Fehr, T., von Bismark, J., Floberghagen, R., and Parrinello, T.: Initial assessment of the performance of the first Wind Lidar in space on Aeolus, EPJ Web Conf., 237, 01010, https://doi.org/10.1051/epjconf/202023701010, 2020. a, b, c, d
Rennie, M. P. and Isaksen, L.: Investigations Into the Quality of Aeolus L2B Winds Using the ECMWF Model and Initial NWP Impact Assessment, in: ESA Living Planet Symposium 2019, 13–17 May 2019, Milan, Italy, available at: https://lps19.esa.int/NikalWebsitePortal/living-planet-symposium-2019/lps19/Agenda/AgendaItemDetail?id=1a3d272c-f7d1-4847-b1c4-08c452f9405f (last access: 8 May 2020), 2019. a, b, c
Rennie, M. P. and Isaksen, L.: The NWP impact of Aeolus Level-2B Winds at ECMWF, https://doi.org/10.21957/alift7mhr, 2020. a, b, c, d, e, f
Rennie, M. P., Tan, D., Andersson, E., Poli, P., Dabas, A., De Kloe, J., Marseille, G.-J., and Stoffelen, A.: Aeolus Level-2B Algorithm Theoretical Basis Document (Mathematical Description of the Aeolus L2B Processor), available at: https://earth.esa.int/pi/esa?type=file&table=aotarget&cmd=image&alias=Aeolus_L2B_Algorithm_TBD, last access: 8 May 2020. a, b, c, d, e, f, g
Rittmeister, F., Ansmann, A., Engelmann, R., Skupin, A., Baars, H., Kanitz, T., and Kinne, S.: Profiling of Saharan dust from the Caribbean to western Africa – Part 1: Layering structures and optical properties from shipborne polarization/Raman lidar observations, Atmos. Chem. Phys., 17, 12963–12983, https://doi.org/10.5194/acp-17-12963-2017, 2017 a
Santillan, D., Huber, D., Meringer, M., Reitebuch, O., Schindler, F., and Weiler, F.: VirES for Aeolus – Online visual analysis of Aeolus data, in: ESA Living Planet Symposium 2019, 13–17 May 2019, Milan, Italy, available at: https://elib.dlr.de/130561/ (last access: 8 May 2020), 2019. a
Schmithüsen, H.: Radiosonde measurements during POLARSTERN cruise PS116, Alfred Wegener Institute, Helmholtz Centre for Polar and Marine Research, Bremerhaven, PANGAEA, https://doi.org/10.1594/PANGAEA.903888, 2019. a, b, c
Stoffelen, A., Pailleux, J., Källén, E., Vaughan, J. M., Isaksen, L., Flamant, P., Wergen, W., Andersson, E., Schyberg, H., Culoma, A., Meynart, R., Endemann, M., and Ingmann, P.: The Atmospheric Dynamics Mission for Global Wind Field Measurment, B. Am. Meteorol. Soc., 86, 73–88, https://doi.org/10.1175/BAMS-86-1-73, 2005. a
Stoffelen, A., Marseille, G. J., Bouttier, F., Vasiljevic, D., de Haan, S., and Cardinali, C.: ADM-Aeolus Doppler wind lidar Observing System Simulation Experiment, Q. J. Roy. Meteor. Soc., 132, 1927–1947, https://doi.org/10.1256/qj.05.83, 2006. a, b
Stoffelen, A., Reitebuch, O., Isaksen, L., v. Bismarck, J., Marksteiner, U., Weiler, F., Huber, D., Rennie, M., Dabas, A., Flament, T., Straume-Lindner, A. G., Kanitz, T., de Kloe, J., Marseille, G.-J., and van Zadelhoff, G.-J.: Aeolus First Results, in: Joint EUMETSAT/AMS/NOAA Conference 2019, 30 September–4 October 2019, Boston, USA, available at: https://elib.dlr.de/127413/ (last access: 8 May 2020), 2019. a
Straume, A. G., Rennie, M., Isaksen, L., de Kloe, J., Marseille, G.-J., Stoffelen, A., Flament, T., Stieglitz, H., Dabas, A., Huber, D., Reitebuch, O., Lemmerz, C., Lux, O., Marksteiner, U., Weiler, F., Witschas, B., Meringer, M., Schmidt, K., Nikolaus, I., Geiß, A., Flamant, P., Kanitz, T., Wernham, D., von Bismarck, J., Bley, S., Fehr, T., Floberghagen, R., and Parrinello, T.: ESA's space-based Doppler wind lidar mission Aeolus – First wind and aerosol product assessment results, EPJ Web Conf., 237, 01007, https://doi.org/10.1051/epjconf/202023701007, 2020. a, b, c
Sun, X. J., Zhang, R. W., Marseille, G. J., Stoffelen, A., Donovan, D., Liu, L., and Zhao, J.: The performance of Aeolus in heterogeneous atmospheric conditions using high-resolution radiosonde data, Atmos. Meas. Tech., 7, 2695–2717, https://doi.org/10.5194/amt-7-2695-2014, 2014. a
Tan, D. G. H., Andersson, E., De Kloe, J., Marseille, G.-J., Stoffelen, A., Poli, P., Denneulin, M.-L., Dabas, A., Huber, D., Reitebuch, O., Flamant, P., Le Rille, O., and Nett, H.: The ADM-Aeolus wind retrieval algorithms, Tellus A, 60, 191–205, https://doi.org/10.1111/j.1600-0870.2007.00285.x, 2008. a, b
von Bismarck, J., Straume-Lindner, A. G., de Bruin, F., Buscaglione, F., Elfving, A., Fehr, T., Fischer, P., Floberghagen, R., Kanitz, T., Perrinello, T., Piñero, J., Vogel, P., and Wernham, D.: Aeolus – Status of ESA's Doppler Wind Lidar Mission, in: ESA Living Planet Symposium 2019, 13–17 May 2019, Milan, Italy, available at: https://lps19.esa.int/NikalWebsitePortal/living-planet-symposium-2019/lps19/Agenda/AgendaItemDetail?id=1a3d272c-f7d1-4847-b1c4-08c452f9405f (last access: 8 May 2020), 2019. a
Wandinger, U.: Multiple-scattering influence on extinction- and backscatter-coefficient measurements with Raman and high-spectral-resolution lidars, Appl. Optics, 37, 417–427, https://doi.org/10.1364/AO.37.000417, 1998. a
Witschas, B., Lemmerz, C., Geiß, A., Lux, O., Marksteiner, U., Rahm, S., Reitebuch, O., and Weiler, F.: First validation of Aeolus wind observations by airborne Doppler wind lidar measurements, Atmos. Meas. Tech., 13, 2381–2396, https://doi.org/10.5194/amt-13-2381-2020, 2020. a, b, c