the Creative Commons Attribution 4.0 License.
the Creative Commons Attribution 4.0 License.
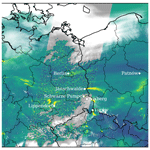
Detectability of CO2 emission plumes of cities and power plants with the Copernicus Anthropogenic CO2 Monitoring (CO2M) mission
Grégoire Broquet
Julia Marshall
Valentin Clément
Armin Löscher
Yasjka Meijer
Dominik Brunner
High-resolution atmospheric transport simulations were used to investigate the potential for detecting carbon dioxide (CO2) plumes of the city of Berlin and neighboring power stations with the Copernicus Anthropogenic Carbon Dioxide Monitoring (CO2M) mission, which is a proposed constellation of CO2 satellites with imaging capabilities. The potential for detecting plumes was studied for satellite images of CO2 alone or in combination with images of nitrogen dioxide (NO2) and carbon monoxide (CO) to investigate the added value of measurements of other gases coemitted with CO2 that have better signal-to-noise ratios. The additional NO2 and CO images were either generated for instruments on the same CO2M satellites (2 km× 2 km resolution) or for the Sentinel-5 instrument (7.5 km× 7.5 km) assumed to fly 2 h earlier than CO2M. Realistic CO2, CO and fields were simulated at 1 km× 1 km horizontal resolution with the Consortium for Small-scale Modeling model extended with a module for the simulation of greenhouse gases (COSMO-GHG) for the year 2015, and they were used as input for an orbit simulator to generate synthetic observations of columns of CO2, CO and NO2 for constellations of up to six satellites. A simple plume detection algorithm was applied to detect coherent structures in the images of CO2, NO2 or CO against instrument noise and variability in background levels. Although six satellites with an assumed swath of 250 km were sufficient to overpass Berlin on a daily basis, only about 50 out of 365 plumes per year could be observed in conditions suitable for emission estimation due to frequent cloud cover. With the CO2 instrument only 6 and 16 of these 50 plumes could be detected assuming a high-noise (σVEG50=1.0 ppm) and low-noise (σVEG50=0.5 ppm) scenario, respectively, because the CO2 signals were often too weak. A CO instrument with specifications similar to the Sentinel-5 mission performed worse than the CO2 instrument, while the number of detectable plumes could be significantly increased to about 35 plumes with an NO2 instrument. CO2 and NO2 plumes were found to overlap to a large extent, although NOX had a limited lifetime (assumed to be 4 h) and although CO2 and NOX were emitted with different NOX:CO2 emission ratios by different source types with different temporal and vertical emission profiles. Using NO2 observations from the Sentinel-5 platform instead resulted in a significant spatial mismatch between NO2 and CO2 plumes due to the 2 h time difference between Sentinel-5 and CO2M. The plumes of the coal-fired power plant Jänschwalde were easier to detect with the CO2 instrument (about 40–45 plumes per year), but, again, an NO2 instrument could detect significantly more plumes (about 70). Auxiliary measurements of NO2 were thus found to greatly enhance the capability of detecting the location of CO2 plumes, which will be invaluable for the quantification of CO2 emissions from large point sources.
- Article
(16051 KB) - Full-text XML
- Companion paper 1
- Companion paper 3
-
Supplement
(285 KB) - BibTeX
- EndNote
The signatory countries of the Paris climate agreement have set ambitious goals to reduce CO2 emissions and limit global warming to below 2 ∘C above preindustrial levels (UNFCCC, 2015). The efficient implementation and management of long-term policies will require consistent, reliable and timely information on CO2 emissions (Ciais et al., 2015; Pinty et al., 2018). The majority of these emissions are concentrated on a small fraction of the globe, primarily on cities and power plants. Acknowledging their important role, cities have started to devise policies for cutting CO2 emissions often surpassing the reduction targets of the respective countries (e.g., C40 cities, 2018). However, many cities are currently lacking detailed CO2 emission inventories and monitoring systems to evaluate their policies.
The European Space Agency (ESA) and the European Commission (EC) therefore propose the Copernicus Anthropogenic Carbon Dioxide Monitoring (CO2M) mission, a constellation of CO2 satellites with imaging capability, to support the quantification of anthropogenic CO2 fluxes and to assist greenhouse gas mitigation policies at the national, city and facility level (Sierk et al., 2019). The satellites are envisioned as an essential component of a CO2 emission monitoring and verification support system to be established under Europe's Earth observation program Copernicus (Ciais et al., 2015; Pinty et al., 2018). The system would allow for observing CO2 plumes of individual point sources such as large cities and power plants and for quantifying the respective emissions during single satellite overpasses (Bovensmann et al., 2010; Pillai et al., 2016; Velazco et al., 2011). A CO2 plume is defined here as an enhancement of CO2 concentrations above the background in the satellite image caused by the emissions of a given source. The emissions of the source can be estimated from the CO2 enhancement inside the plume, which requires that the plume location is identified in the satellite observations and assigned to the source. An atmospheric transport model may be used for simulating the plume location and for estimating the emissions with an inversion framework (e.g., Pillai et al., 2016; Broquet et al., 2018). However, the simulated plume might be significantly displaced due to uncertainties in wind fields and emission heights, which would result in systematic errors in the estimated emissions (Broquet et al., 2018; Brunner et al., 2019). It is therefore desirable to detect the plume directly in the satellite observations, which would make it possible to correct transport-related errors in the simulations but also to estimate the emissions directly from the CO2 enhancements in the plume using plume fitting or mass balance approaches, which only require an estimate of the mean wind speed within the plume (Fioletov et al., 2015; Krings et al., 2013; Varon et al., 2018). While some potential for detecting and estimating emissions from CO2 fluxes has been demonstrated for strong CO2 plumes of megacities and large point sources using the Orbiting Carbon Observatory 2 (OCO-2, Crisp et al., 2017) (Nassar et al., 2017; Reuter et al., 2019), it remains a major challenge to accurately determine the location of CO2 plumes, especially of weaker plumes with signal-to-noise ratios near or below the detection limit for single pixels. The detection of CO2 plumes is additionally challenged by the interference with signals from biospheric CO2 fluxes and other anthropogenic sources in the vicinity of the target. Therefore, measurements of auxiliary trace gases coemitted with CO2 but little affected by biospheric processes such as carbon monoxide (CO) and nitrogen dioxide (NO2) were proposed to help separate anthropogenic from biospheric CO2 signals (Reuter et al., 2014; Ciais et al., 2015).
This study presents results from the SMARTCARB project (use of satellite measurements of auxiliary reactive trace gases for fossil fuel carbon dioxide emission estimation, Kuhlmann et al., 2019), which aimed to assess the potential synergies of measurements of CO and NO2 for observing and quantifying CO2 emissions and to help define the required satellite specifications for the CO2M mission. To address these questions, Observing System Simulation Experiments (OSSEs) were conducted, for which synthetic satellite observations were generated from high-resolution atmospheric transport simulations. The model domain was centered on the city of Berlin and also covered several nearby power plants. Similar simulations were already performed in previous OSSEs (Pillai et al., 2016; Broquet et al., 2018), but they did not have a comparable spatial resolution or temporal extent or did not cover the additional species NO2 and CO as investigated here.
Since the detection of the CO2 plume is a first and important step of a CO2 emission monitoring system, the aim of this paper is to investigate whether and how often CO2 plumes are expected to be detected in the satellite images during a year depending on the size of the CO2M satellite constellation and on instrument error scenarios. The detectability is studied for satellite images of CO2 alone or in combination with images of NO2 and CO to investigate the added value of additional measurements either on the same CO2M satellite (2 km× 2 km, overpass: 11:30 local time) or with the Sentinel-5 instrument (7.5 km× 7.5 km, overpass: 09:30 local time). In this paper, we analyze the signal-to-noise ratios of a city plume and of different point sources for the different instruments. Furthermore, based on a newly developed simple plume detection algorithm, we identify statistically significant plume signals against instrument noise and background variability. The results are used to provide recommendations for the dimensioning of the CO2M mission, which will be a key component of the Copernicus CO2 emission monitoring and verification support system. In a companion paper Brunner et al. (2019) presented the overall model setup and emphasized the importance of properly accounting for the vertical placement of CO2 emissions from large point sources in atmospheric CO2 simulations. In a follow-up study, we will quantify the emissions from Berlin and a few power plants in the model domain from the synthetic satellite observations using both inverse and mass-balance approaches, building on the plume detection presented here.
In a satellite image, a plume may be defined as a collection of spatially connected pixels with elevated signals starting at a source. Whether and how frequently the plume of a given source can be detected depends on several, partly interdependent factors:
-
The number of satellites and the instrument's swath width, as they determine the number of overpasses over the plume and how much of the plume is visible in the satellite image.
-
The intensity of the emission source, which affects the amplitude of the enhancement above background.
-
The meteorological conditions, notably wind speed and turbulence, which determine the dilution and dispersion of the emissions.
-
The single sounding precision of the instrument, which determines if the enhancement within the plume can be detected.
-
The variability of the background, which is caused by anthropogenic emissions and biospheric fluxes in the vicinity of the source and which is additionally affected by meteorology.
-
The presence of clouds partially or fully obscuring the plume.
Since most of these factors vary with season, the detectability also depends on the time of the year. Therefore, long simulations covering a full year were conducted.
Because the detection of weak anthropogenic CO2 plumes is affected by interference with biospheric CO2 signals, auxiliary trace gases coemitted with CO2 could be used for locating the CO2 plume in the satellite image. However, this requires that the plumes of CO2 and of the auxiliary trace gas are spatially congruent. This is usually the case when they are emitted from the same source, for example, a power plant. The shape of the NO2 plumes might deviate from the CO2 plume for two reasons. First, NO2 is emitted mainly as nitrogen monoxide (NO), which is converted to NO2 over time, resulting in lower NO2 concentrations near the source. Second, NO2 decays slowly with time, reducing NO2 concentrations downstream. To account for these two effects, we simulated nitrogen oxides () that slowly decay with time and calculated NO2 from NOX concentrations offline by applying a formula frequently used to represent NO2:NOX ratios downstream of emission sources (Düring et al., 2011). The situation is more complex for cities where the emissions originate from different sectors (industry, heating, transport, etc.) that emit with different temporal profiles and at different altitude levels, and which have different emission ratios (Brunner et al., 2019). In this study, we therefore carefully consider the vertical and temporal profiles of emissions from different sectors, which makes it possible to test for congruence.
2.1 Synthetic satellite observations
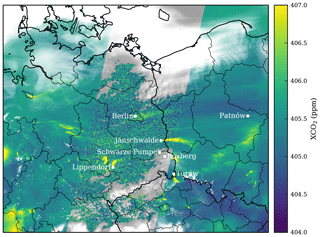
Figure 1Simulated XCO2 field on 23 April 2015 in the SMARTCARB model domain overlaid with an example of a 250 km wide swath of the planned Sentinel CO2 instrument (low-noise scenario). Missing CO2 measurements are shown in gray. Cloud cover is overlayed in white, with transparency corresponding to total cloud fraction.
2.1.1 Model simulations
The synthetic satellite observations were generated from high-resolution simulations conducted with the COSMO-GHG model. COSMO is a hydrostatic, limited-area model developed by the Consortium for Small-scale Modeling (Baldauf et al., 2011), for which an extension has been developed for the simulation of greenhouse gases (COSMO-GHG)(Oney et al., 2015; Liu et al., 2017).
COSMO-GHG was set up to simulate CO2, CO and NOx concentration fields for nearly the complete year 2015 (1 January–25 December). The model domain extended about 750 km in the east–west and 650 km in the south–north direction. It was centered over the city of Berlin and also covered numerous power plants in Germany and neighboring countries. The spatial resolution was 1.1 km × 1.1 km horizontally with 60 vertical levels up to an altitude of 24 km. Figure 1 presents the model domain and marks the location of Berlin and the six largest coal-fired power plants. The detailed model setup is described by Brunner et al. (2019).
Initial and lateral boundary conditions (ICBCs) for meteorological variables were provided by the operational European COSMO-7 analyses of MeteoSwiss with hourly temporal and 7 km horizontal resolution. For the tracers, ICBCs were obtained from the European Centre for Medium-Range Weather Forecasts (ECMWF) through the European Earth observation program Copernicus. CO2 and CO boundary conditions were taken from a global free-running CO2 simulation with 137 levels and about 15 km horizontal resolution (T1279 spectral resolution, experiment gf39, class rd) (Agustí-Panareda et al., 2014). For NO and NO2, boundary conditions were taken from ECMWF's operational global forecasts for aerosol and chemical species with 60 vertical levels and a horizontal resolution of about 60 km (T255 resolution, experiment 0001, class mc) (Flemming et al., 2015).
Table 1Emissions of largest power plants in the model domain according to the TNO/MACC-3 inventory for the year 2011 as used in this study.
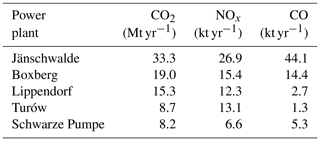
Anthropogenic emissions were obtained by combining the third version of the TNO/MACC inventory (Kuenen et al., 2014, for version 2), which was generated by the Netherlands Organisation for Applied Scientific Research (TNO) for the Monitoring Atmospheric Composition and Climate – Phase III (MACC-3) project, with a detailed inventory provided by the city of Berlin (AVISO GmbH and IE Leipzig, 2016). The inventories provide point and area sources separately for different sectors (e.g., industry, heating and road transport) using Selected Nomenclature for Air Pollution (SNAP) categories. The temporal variability of emissions was accounted for by applying diurnal, weekly and seasonal cycles according to SNAP categories. Furthermore, emissions were vertically distributed using specific vertical profiles for the different emissions categories and plume rise calculations for the six largest power plants and the major point sources in Berlin (Brunner et al., 2019). Hourly biospheric fluxes of both photosynthesis and respiration were generated with the Vegetation Photosynthesis and Respiration Model (VPRM) at the resolution of the COSMO model (Mahadevan et al., 2008).
According to the official inventory of the city of Berlin, total annual CO2 emissions of Berlin were 16.9 Mt CO2 yr−1 (in the reference year 2012 of the inventory). This is about a factor of 2 smaller than in previous studies, e.g., in the LOGOFLUX project (Chimot et al., 2013; Bacour et al., 2015; Pillai et al., 2016), which relied on unrealistically high emissions as provided by the global EDGAR inventory (version 4.1). Due to the diurnal cycle of emissions, emissions were somewhat larger (about 20.0 Mt CO2 yr−1) around the time of the satellite overpasses (10:00–11:00 UTC). Table 1 summarizes the CO2, NOx and CO emissions of the five largest power plants in the domain.
The simulations included a total of 50 different passively transported tracers representing the three different gases further divided into different sources, release times or release altitudes. This also included background tracers constrained at the lateral boundaries by the global-scale models and two tracers for biospheric respiration and photosynthesis for CO2. Due to the reactivity of NOx, five different NOx tracers with e-folding lifetimes of 2, 4, 12, and 24 h and infinity were included, considering that the lifetime of NOx varies between about 2 and 24 h (Schaub et al., 2007). The full list of tracers is provided in the SMARTCARB final report (Kuhlmann et al., 2019, p. 15f).
In this study we use the following seven tracers that have been computed from the 50 tracers in the simulations.
-
X_BER: concentrations from time-varying emissions of Berlin;
-
X_PP: concentrations from time-varying emissions from the six largest power plants in the model domain;
-
X_ANTH: concentrations from other anthropogenic sources in the domain excluding emissions of Berlin (X_BER) and the six largest power plants (X_PP);
-
X_BIO: concentrations from local biospheric fluxes, i.e., respiration and photosynthesis within the domain (only for CO2);
-
X_TOT: concentrations from all emissions and biospheric fluxes as well as inflow from lateral boundaries;
-
X_BER_BG: concentrations from emissions, fluxes and lateral boundaries excluding emissions from Berlin (= X_TOT–X_BER); and
-
X_PP_BG: concentrations from emissions, fluxes and lateral boundaries excluding emissions from the six major power plants (= X_TOT–X_PP),
where X is CO2, CO or NO2. NO2 concentrations were calculated from NOx concentrations using an empirical formula used frequently for representing NO2:NOx ratios downstream of emission sources (Düring et al., 2011). For NO2 only the tracers with a lifetime of 4 h were used. Note that only the sum of the emissions from the six power plants was simulated but not the power plants individually, which often complicated the analysis due to overlapping plumes. For the analysis, the three-dimensional model fields were vertically integrated to compute column-averaged dry air mole fractions of CO2 (XCO2). Likewise, tropospheric CO and NO2 vertical column densities (VCDs) were generated by considering only the model fields below 10 km altitude.
2.1.2 Satellite instrument scenarios
Table 2Satellite platforms and their orbit parameters used in this study. Note that some parameters slightly deviate from the real satellites (see text for details).
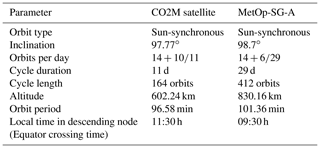
Table 3Observation geometries for instruments on the CO2M satellite and Sentinel-5 used in this study. Note that some parameters can differ from the real satellites (see text for details).
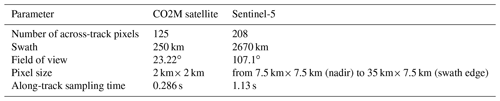
The CO2M mission is a proposed constellation of satellites flying in a sun-synchronous low-Earth orbit with Equator crossing times around 11:30 local time. Each satellite will carry an imaging spectrometer measuring in the near-infrared (NIR) and in two shortwave infrared spectral bands (SWIR 1 and SWIR 2) for retrieving CO2 as the main payload. The NIR band is used to retrieve information on the dry air column, on surface pressure, and on aerosols and clouds. The SWIR-1 and SWIR-2 bands contain weak and strong absorption features of CO2 and provide additional information on aerosols and clouds, especially on thin cirrus clouds. A CO2 retrieval using these three bands is described for example by O'Dell et al. (2012). CO2M is planned to carry also additional instruments for measuring NO2, aerosols and clouds. In an earlier phase, also an instrument measuring CO was considered. The preliminary system concept envisages a pixel size of 4 km2 and a swath width of 250 km or more.
For the CO2, CO and NO2 satellite observations, different instrument scenarios were prescribed by ESA for this study in terms of orbit, spatial resolution, and spatial and temporal coverage of the CO2M instrument. In addition, the Sentinel-5 instrument on board the Meteorological Operational Satellite – Second Generation A (MetOp-SG-A) was studied as an alternative platform for CO and NO2 measurements. Sentinel-5 will be an imaging spectrometer measuring, among others, NO2 and CO columns with a spatial resolution of 7 km× 7 km and a 2650 km swath. MetOp-SG-A will be also on a sun-synchronous orbit but with different Equator crossing times and repeat cycles than the CO2M mission.
In addition to a single satellite, the potential of a constellation of multiple CO2M satellites was also studied. The basic assumption for a constellation is that the individual satellites are spaced with equal angular distance in the same orbit with the same orbit parameters, for instance separated by 180, 120 and 90∘ on a full circle in the case of 2, 3 and 4 satellites. The individual satellites can be distinguished by their starting longitude at the Equator of the first orbit in the repeat cycle. Here, we analyze constellations between one and six satellites.
For the computation of orbits, we adopted the orbit simulator of the Netherlands Institute for Space Research (SRON). Since this simulator makes a few simplifying assumptions, such as circular orbits and tiled ground pixels, satellite and instrument parameters were slightly modified to preserve essential parameters. In particular, orbit periods were calculated to match a given cycle duration and length. The period then determines the altitude and inclination of a circular, sun-synchronous orbit. The altitude of the circular orbits is slightly larger than the typically used mean altitude for elliptic orbits. Since the altitude affects the size of the ground pixels and the width of the swath, field of view and along-track sampling time were set to match exactly the prescribed pixel size at subsatellite point as well as the prescribed swath width. As a result, the number of across-track pixels for the simulated Sentinel-5 did not match exactly the number of pixels for the real Sentinel-5 instrument.
Tables 2 and 3 summarize the orbits and viewing geometries of the two satellites. The CO2M satellite is assumed to have a 250 km wide swath and an 11 d repeat cycle. Within the 11 d cycle, the instrument provides nearly global spatial coverage (Fig. 2). For locations in the SMARTCARB model domain, either one or two overpasses occur during the 11 d repeat cycle depending on the Equator starting longitude of the satellite. The wider swath of Sentinel-5 results in near-daily global coverage (not shown).
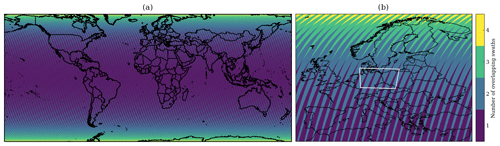
Figure 2Spatial coverage of one CO2M satellite within its 11 d repeat cycle (a) globally and (b) over Europe. The white square marks the COSMO-GHG model domain in which the number of overpasses is either one or two. The exact locations of the stripes are arbitrary and depend on the Equator starting longitude (here: 0∘ E) of the satellite.
XCO2, CO and NO2 column densities were sampled along the satellite swath for 1 year using the tracers from the COSMO-GHG simulations. For CO2M, XCO2, CO and NO2 columns were mapped onto the 2 km × 2 km size pixels along the 250 km wide swath.
For Sentinel-5, CO and NO2 columns were sampled with up to 7.5 km × 7.5 km resolution along the 2670 km wide swath of the Sentinel-5 instrument. Due to the wide swath, the pixel sizes grow towards the edge of the swath. In this study, only the spatial overlap between Sentinel-5 and CO2M were of interest because Sentinel-5 was used for detecting the CO2 emission plumes inside the swath of CO2M.
2.1.3 Instrument error characteristics
The error characteristics of the CO2, NO2 and CO instruments were specified in collaboration with ESA based on previous studies for CarbonSat (Buchwitz et al., 2013) and on performance requirements for Sentinel-5 (Ingmann et al., 2012). For the instruments on the CO2M satellites, two or three scenarios were included in order to cover a realistic range between more or less demanding instruments. Table 4 summarizes the single sounding precision of the different instruments.
Table 4Instrument uncertainty scenarios. VEG50 refers to a reference scene with a surface albedo of a vegetated surface and a solar zenith angle (SZA) of 50∘.
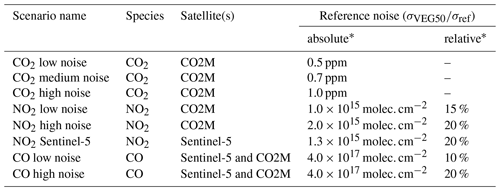
* Whichever is larger.
For XCO2, three different uncertainty scenarios were considered which relate back to the performance estimates derived for the CarbonSat mission concept. CarbonSat was a CO2 imaging spectrometer proposed for ESA's eighth Earth Explorer mission, with specifications similar to those of the CO2M mission. A detailed error budget for CarbonSat was presented in the CarbonSat report for mission selection (ESA, 2015). In the LOGOFLUX study, error parameterization formulas (EPFs) for random and systematic errors were developed, which account for errors introduced by solar zenith angle (SZA), surface reflectance in the near-infrared (NIR) and shortwave infrared (SWIR 1), cirrus clouds and aerosol optical depth (Buchwitz et al., 2013).
Here, the same EPFs were adopted but only applied to compute random errors. These were calculated based on SZA and surface reflectance in the NIR and SWIR-1 band. Surface reflectances were taken from the MODIS MCD43A3 product (version 006) at 1 km spatial resolution (Schaaf and Wang, 2015). A detailed consideration of cirrus clouds and aerosols and their impact on systematic errors was outside the scope of the study as it would have required the collection and processing of a large amount of additional data. The possible impact of not considering systematic errors will briefly be discussed in Sect. 4.
The random error calculated with the EPFs for the so-called vegetation-50 scenario (VEG50, i.e., vegetation albedo and SZA of 50∘) is about 1.5 ppm. In the model domain, mean random errors are slightly smaller at 1.3 ppm. To obtain random errors for the three instrument scenarios with σVEG50 of 0.5, 0.7 and 1.0 ppm, the computed errors were divided by 3.0, 2.14 and 1.5, respectively.
For NO2 VCDs, the overall uncertainties are due to (a) measurement noise and spectral fitting affecting the slant column densities; (b) uncertainties related to the separation of the stratospheric and tropospheric column; and (c) uncertainties in the auxiliary parameters used for air mass factor (AMF) calculations such as clouds, surface reflectance, a priori profile shapes and aerosols (Boersma et al., 2004). The total uncertainties are dominated by uncertainties from spectral fitting for background pixels and by uncertainties in AMF calculations for polluted pixels, respectively. Typical spectral fitting uncertainties of previous instruments such as the Ozone Monitoring Instrument (OMI) were of the order of 1–2 × 1015 molecules cm−2 and AMF uncertainties of the order of 15 %–20 %. These ranges were used to define two different scenarios for a possible CO2M NO2 instrument (Table 4). For the Sentinel-5 UVNS instrument, we assumed a relative uncertainty of 20 % and a minimum uncertainty of 1.3 × 1015 molecules cm−2. In the presence of clouds, the reference noise was increased using the empirical formula developed by Wenig et al. (2008). For a cloud fraction of 30 %, random noise is approximately doubled.
For CO VCDs, the total uncertainty depends on the (a) fitting noise; (b) a priori CO and CH4 profiles; and (c) surface reflectance, aerosols and clouds. We assumed a single sounding precision of 4.0 × 1017 molecules cm−2 and a relative precision of 10 % and 20 % for both Sentinel-5 and the CO2M mission.
2.1.4 Cloud filtering
Satellite observations require filtering for clouds, which significantly reduces the number of observations available for plume detection. For the CO2 product, we removed all CO2 pixels with cloud fractions larger than 1 % because the CO2 requires rigorous cloud filtering (Taylor et al., 2016). The NO2 retrieval can tolerate larger errors and is therefore less sensitive to clouds. For the NO2 product, we used a cloud threshold of 30 % as often applied in satellite NO2 studies (e.g., Boersma et al., 2011). For CO, a cloud threshold of 5 % was used, which is motivated by the cloud threshold used for the MOPITT CO product (Deeter et al., 2017; MOPITT Algorithm Development Team, 2017).
Previous studies used the MODIS cloud mask product available at 1 km resolution (Ackerman et al., 2017) for masking cloudy CO2 observations (Buchwitz et al., 2013; Pillai et al., 2016). Since CO and NO2 observations can tolerate larger cloud fractions, a cloud fraction product would be needed for masking pixels with different thresholds, but the MODIS cloud product is only available at 5 km resolution (Platnick et al., 2017). Therefore, we used total cloud fractions computed by COSMO-GHG, i.e., the same model as used for the tracer transport simulations, that are available at model resolution. COSMO-GHG computes total cloud fraction from layer cloud fractions assuming minimum overlap. The differences between cloud masks derived from COSMO-GHG and MODIS products and their effects on data yield are discussed in Sect. 4.1.
2.2 Plume detection algorithm
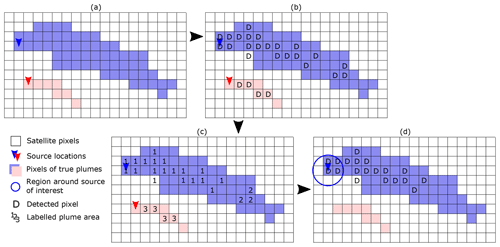
Figure 3Schematic of the processing steps of the plume detection algorithm. (a) A large (blue) and a small plume (red) with sources marked by arrows are located within the satellite overpass. (b) Pixels detected based on the Z test are marked with “D”. (c) Connected pixels are given a unique number each denoting a different plume. (d) All plumes not connected with the source of interest (blue circle) are rejected.
2.2.1 Algorithm
We developed a new but simple plume detection algorithm that uses a statistical test to detect signal enhancements which are significant with respect to instrument noise and variability in background levels. The plume is then identified as a coherent structure of significant pixels. The algorithm involves three processing steps as laid out in Fig. 3.
The first step of the plume detection algorithm finds satellite pixels with CO2, CO or NO2 values significantly larger than the background field using a statistical Z test, for which the distribution of the test statistics can be approximated by a normal distribution (e.g., v. Storch and Zwiers, 2003). The Z test computes a z value given by
where x is an observation from a population with mean value μ and standard deviation σ. The value x is considered significantly larger than μ when the z value is greater that or equal to a critical value. The critical value is calculated from the inverse cumulative distribution function of the normal distribution for a probability q. The probability that x is not significantly larger than μ is the p value ().
A key feature of the algorithm is that the trace gas observation of a single pixel is replaced by a spatial average of pixels in a defined neighborhood. This allows identifying weak plumes with signals of individual pixels well below instrument noise but also bares the risk of diluting the signal at the plume edges. Figure 4 shows examples of neighborhoods with different sizes ns that have been used for testing the algorithm. A large neighborhood results in a stronger smoothing and may therefore produce a larger number of false positives, i.e., pixels outside of the plume that are wrongly assigned to the plume. An ideal neighborhood size balances the need for sensitive plume detection with the requirement of a low fraction of false positives.
Whether a signal enhancement is detectable is primarily determined by the z value (Eq. 1), which can also be interpreted as the signal-to-noise ratio (SNR) at a spatially smoothed satellite pixel. Thereby, the signal is the enhancement above background due to the plume, and the noise is composed of both instrument noise and spatial variability in the background. Since the background, i.e., the trace gas field in the absence of the plume, cannot be directly observed, it needs to be estimated either from the observable trace gas field surrounding the plume or from a climatology or a model. Instrument noise and background variability can have both random and systematic components. The random component would reduce with the inverse square root of the number of valid pixels n in the neighborhood, whereas the systematic error would be approximately independent of n. The number of valid pixels n can be smaller than the size of the neighborhood ns when pixels are missing, e.g., due to clouds or at the boundary of the satellite swath. Therefore, the z value or the SNR can be calculated as follows:
where Xobs represents the spatially averaged satellite observations, Xbg is the estimated background value, and σrand and σsys are the random and systematic errors, respectively. Equation (2) can be calculated for each satellite pixel and compared with the critical value to determine which Xobs's are significantly larger than Xbg.
To compute the SNRs from the satellite image, we computed observed values Xobs as the local means of a neighborhood of size ns (Fig. 4). For sake of simplicity, the background Xbg in this study was estimated from the X_BER_BG and X_PP_BG model tracers for a 200 km× 200 km square centered on the city of Berlin and a 100 km× 100 km square centered on each power plants. The implications of this assumption are discussed in Sect. 4.
For random and systematic errors we assumed that the instrument uncertainty is purely random and that the background variability is purely systematic. For the instrument uncertainty, random errors as listed in Table 4 were used, which reduce with the number of valid pixels in the local neighborhood n due to the inverse scaling by the number of pixels. The background uncertainty was computed from the spatial variance of the background model tracer in a fixed domain surrounding the source as described above. Since it is assumed to be systematic, it does not decrease with the size of the neighborhood.
The result of the Z test is a binary image with “true” values where pixels are significantly enhanced above the background and “false” values where they are not (Fig. 3b). Since the local mean can still be computed for missing center pixels – using neighboring pixels – missing pixels can also be detected as enhanced above the background.
In the second step, pixels that are enhanced (true) and connected are assumed to belong to the same plume. We label regions of connected pixels using a standard labeling algorithm. Neighboring pixels are identified using a Moore neighborhood, where each pixel has eight potential neighbors. Each region is assigned a unique integer value (Fig. 3c).
Finally, in the third step, all connected regions that do not intersect with the source region are removed, leaving only regions that overlap with the source of the plume (Fig. 3d). For cities, the source region is defined by a circle with a radius of 15 km and for point sources by a circle with a radius of 5 km. The last step may remove regions that are part of the real plume but separated from the source by weak signals or missing values (e.g., the region labeled “2” in Fig. 3c).
2.2.2 Performance evaluation
The plume detection algorithm was applied to the CO2 plumes of Berlin and Jänschwalde. The detectability of CO2 plumes was evaluated for the different instrument scenarios by comparing the detected plume with the “true plume” defined by the field of the CO2 tracer released by the respective source (CO2_BER in the case of Berlin, CO2_PP for Jänschwalde) above a low threshold. For Jänschwalde, the performance had to be additionally evaluated by visual inspection due to frequent overlaps with the plumes of other power plants also contained in the CO2_PP tracer.
To evaluate the performance of Sentinel-5 with respect to detecting plumes as observed by CO2M, detected pixels had to be projected onto the pixels of the CO2M instrument. Sentinel-5 pixels were thus only used over the swath of the CO2M satellite rather than over the whole swath of Sentinel-5.
When using NO2 or CO for plume detection, the performance was assessed by comparing the detected pixels with the true CO2 plume rather than the true plume of the auxiliary gas. In this way, the degree of congruence between the CO2 and auxiliary trace gas plumes was considered as well.
For the evaluation, we computed true positives (TPs), false positives (FPs) and the positive predictive value () (Ting, 2010). A good algorithm should have a much smaller number of FPs than TPs and, therefore, a PPV close to 1. To remove the impact of different cloud thresholds, TPs, FPs and PPVs were only computed for cloud-free pixels using a cloud threshold of 1 % because we are primarily interested in valid CO2 observations that can be used for estimating CO2 emissions.
3.1 Coverage and potential for plume detection
In this section, the potential for plume detection is analyzed based on the simulated tracers emitted by the source of interest. These true plumes will be used in the following sections as reference to evaluate the performance of the plume detection algorithm. They can be interpreted as the maximum number of plumes detectable by a perfect, noise-free instrument.
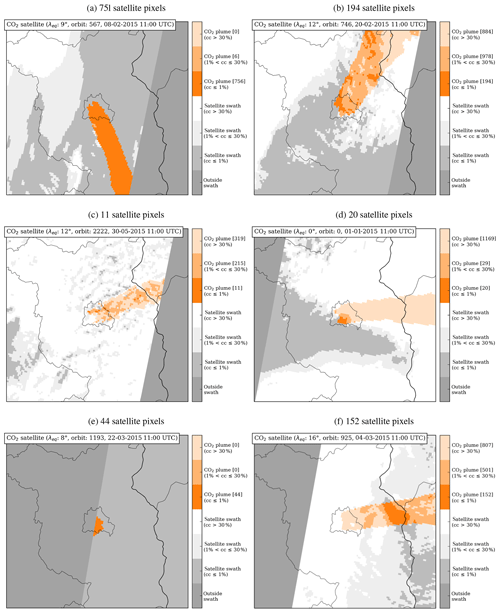
Figure 5Examples of true XCO2 plumes of Berlin (XCO2 signal >0.05 ppm) with different cloud cover fractions (cc). The numbers of XCO2 pixels are shown for cloud fractions ≤1 %. (a–d) Plumes with increasing cloud fraction; (e) plume close to the edge of the swath; (f) plume without cloud-free CO2 observations connected to Berlin.
The frequency with which the CO2 plumes of a given source can be observed depends on how often a satellite passes over the source, how often the CO2 signal is larger than the threshold and how often cloud-free conditions dominate during the overpass. We define an overpass as an intersection between the satellite swath and the source region as specified above. The number of overpasses scales with the number of satellites and the swath width of the instrument. The scaling with the number of satellites is not trivial, however, since individual satellites may pass over the source either once or twice during the 11 d repeat cycle depending on the satellite's Equator starting longitude (see Fig. 2).
The total number of overpasses per satellite is either 34 or 66 per year, depending on whether the satellite has one or two overpasses per 11 d repeat cycle. Since one out of four satellites has only one overpass per 11 d, the number of overpasses per year roughly scales with a factor 1.75 times the number of satellites times the number of 11 d periods per year (about 33). A constellation of six satellites covers the model domain nearly daily.
To define the extent of a plume in the satellite image, we have to set a signal threshold for the tracer field (XCO2_BER for Berlin) above which a pixel is considered as belonging to the plume. A possible threshold is the value at which the signal would become larger than the variability of the background, i.e., where the signal is larger than the standard deviation of the background. Based on the time series of standard deviations of the model background tracer (XCO2_BER_BG for CO2) computed for a 200 km× 200 km square centered on the city of Berlin (Fig. 7c, f and i), we defined a threshold of 0.05 ppm for XCO2, 0.2 × 1015 molec. cm−2 for NO2 and 0.06 × 1017 molec. cm−2 for CO approximately corresponding to the minimum of the standard deviations. Note that these thresholds are significantly smaller than the noise level of the instruments.
A plume was defined as the collection of pixels for which the signal is larger than the threshold. However, we also required that Berlin is inside the swath of the instrument to be able to unambiguously assign a plume to the city. Furthermore, we removed parts of plumes that reentered the swath after leaving it because it is often not possible to correctly assign these parts to their source.
Since a satellite image can be obscured by clouds, we need to define how many pixels are needed to make up a useful plume. This number depends on the application. For example, to estimate emissions of cities, we require that the plume must extend beyond the city limits to contain emissions from the whole city area. The crosswind diameter of Berlin's CO2 plume is typically about 20 km or 10 satellite pixels, which is roughly the diameter of the part of the city with the highest emissions. To cover at least the whole city area, we only consider CO2 plumes with at least 100 cloud-free CO2 pixels to be useful. For the power plants, the crosswind diameter is less than 5 pixels near the source. Therefore, 10 pixels were used to define the minimum number for a useful plume in this case. It should be noted that this number of pixels is not necessarily sufficient for estimating the emissions of a source with certain accuracy, which depends, among others, on instrument precision, meteorology and source strength. Nonetheless, detecting the full crosswind diameter is the minimum requirement, for example, for flux-based inversion methods (e.g., Krings et al., 2013; Reuter et al., 2019). The number of detected pixels is a useful measure for comparing the detectability of CO2 plumes with CO2, CO and NO2 observations because source strength and meteorology are the same for a given source.
For a signal threshold of 0.05 ppm, Berlin's CO2 plume always has more than 100 pixels above the signal threshold. However, many plumes are partly or fully covered by clouds significantly reducing the number of useful plumes. Figure 5a–d present examples of CO2 plumes under different cloud conditions with an increasing fraction of cloudy pixels. Figure 5c and d show examples with plumes of only 11 and 20 pixels, which is much smaller than the area of the city. On the other hand, the 100-pixel threshold does not necessarily remove swaths with plumes in broken clouds (e.g., Fig. 5b), for which it will also be challenging to estimate emissions, because adjacent cloudy pixels increase the XCO2 uncertainty (Taylor et al., 2016). The number of plumes with at least 100 pixels is also reduced when the source is close to the edge of the swath and winds are pushing the plume out of the view of the satellite (e.g., Fig. 5e). These overpasses occur every 11 d due to the repeat cycle of the satellite. As a result, orbits with plumes near the edge of the swath can have up to 20 % less useful plumes.
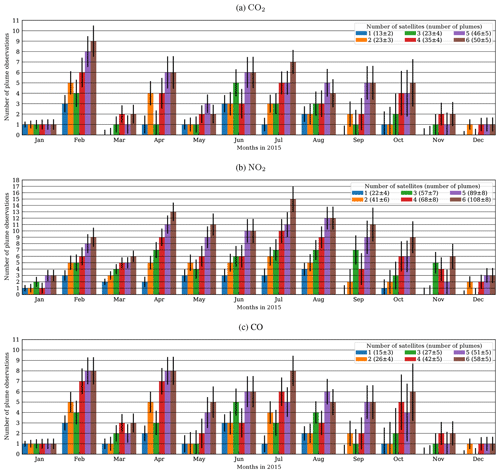
Figure 6Number of cloud-free plumes of the city of Berlin with at least 100 pixels per month for (a) CO2, (b) NO2 and (c) CO. The cloud threshold is 1 % for CO2, 30 % for NO2 and 5 % for CO observations. Error bars are obtained by comparing all available satellites. The number of expected plumes per satellite is 8, 17 and 9 for the CO2, NO2 and CO instrument, respectively.
Figure 6 presents the number of useful city plumes (>100 pixels) per month for CO2, NO2 and CO for constellations of one to six satellites. Plumes without cloud-free observations over the source region (e.g., Fig. 5f) were removed because they cannot be detected by the algorithm used in this study. A constellation of six satellites observes only 50 ± 5 CO2 plumes within 1 year despite almost daily overpasses due to the small number of days with low cloud fractions. Except for February, which was an unusually sunny month in 2015, there is a clear tendency of higher cloud fractions and correspondingly fewer plume observations in winter than in summer. The standard deviations shown in the figures as vertical black bars were estimated from the scatter of observable plumes using satellites with different Equator starting longitudes. The presence of clouds thus reduces the opportunity for plume detection by a factor as a large as 5 to 6 over the city of Berlin. The number of observable NO2 plumes per year (108 ± 8) is about twice as large as for CO2, which is primarily due to the larger cloud threshold of 30 %. For CO the number of observable plumes per year is 58 ± 5. The average number of plumes per satellite and year is thus about 8 (range: 3–13), 9 (4–15) and 17 (7–23) for CO2, CO and NO2, respectively.
The number of observable plumes varies strongly between the individual satellites of a constellation because the number of cloud-free days per year is quite small and the overpass days are different for different Equator starting longitudes. Since satellites are equally spaced in orbit, changing the number of satellites changes the starting longitudes and overpass days of the satellites. As a consequence, the number of observable plumes per constellation can also fluctuate strongly. According to Fig. 6, for example, a constellation of two satellites seems almost equivalent to a constellation of three, but this result is merely a consequence of the fact that cloud cover was often large during these overpasses and Berlin was at the edge of the swath for the satellite with a starting longitude of 8∘. The result would be different for another starting longitude of the first satellite, another city, or another year.
3.2 Signal-to-noise ratios
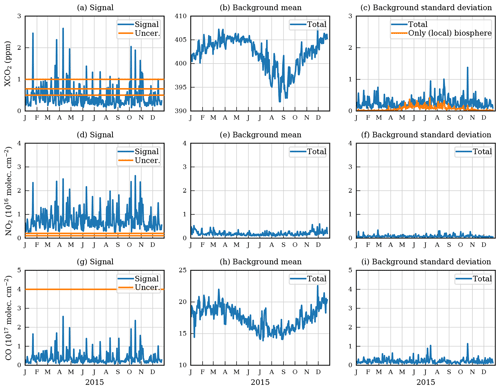
Figure 7Time series of CO2, NO2 and CO plume signals (a, d, g); mean backgrounds (b, e, h); and standard deviations of backgrounds (c, f, i) for Berlin. Signals are the largest local mean values of the X_BER model tracer using a 37-pixel neighborhood. Background means and standard deviations were obtained from the X_BER_BG tracer for a 200 km× 200 km square centered over Berlin. Reference uncertainties (σVEG50∕ref) corresponding to the different instrument scenarios are shown as horizontal lines for comparison.
Table 5The 5th, 50th and 95th percentile of CO2, NO2 and CO signals of Berlin as well as Jänschwalde and Lippendorf power stations.
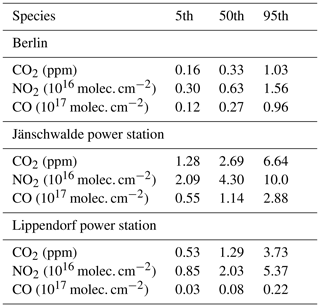
Table 6Median signal-to-noise ratios for signals of Berlin, Jänschwalde and Lippendorf using different uncertainty scenarios. The signals were computed as largest local mean values using a local neighborhood size ns of 37 and 5 for cities and power stations, respectively.
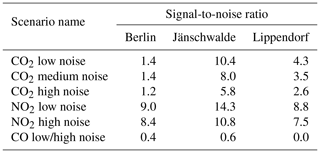
The key measure that determines the detectability of a CO2 plume is the SNR (Eq. 2), which compares the amplitude of the plume signal to the instrument noise and the variability of the background. SNRs provide a first indication of an instrument's suitability for detecting a plume.
Time series of the CO2, NO2 and CO plume signals were computed from the X_BER and X_PP tracers for Berlin and the power stations Jänschwalde and Lippendorf at the overpass time of CO2M, i.e., about 11:00 UTC. The signals were computed as maximum values of the local means within the source region, i.e., a circle with 15 or 5 km radius. Thereby, the local means were computed with a neighborhood ns of size 37 for Berlin and 5 for the power stations (Fig. 4). A large neighborhood reduces the random noise of the measurements and therefore allows detecting smaller signals. On the other hand, a large neighborhood will include background values in the computation of the averages at the plume edges and reduce the signal. The sizes used here roughly correspond to the typical diameters of the CO2 plumes from Berlin (about 15 km) and the power stations (about 6 km), respectively, and were also found most suitable for the plume detection algorithm because they maximize TPs without reducing PPVs too much (see also Kuhlmann et al., 2019). The results for Berlin are presented in Fig. 7 for the three trace gases. The figure compares the daily plume signals (left panels) to the daily mean background values (middle) and their spatial variability (right). The 5th, 50th and 95th percentiles of the time series are summarized in Table 5 for Berlin as well as for the power stations. The signals have a large range due to the variability of emissions (e.g., lower during weekends) and meteorology. The CO2 and NO2 signals of the power stations are between 5 and 10 times larger than those of Berlin. The CO signal of Lippendorf, on the other hand, is smaller than the signal of Berlin. The power plants produce strong local enhancements easily detectable by the CO2 satellite, but the corresponding plumes are much narrower than those of Berlin.
Figure 7b and c present the spatial means and standard deviations of the background around Berlin. Background XCO2 has a strong annual cycle with an amplitude of about 16 ppm. Since the XCO2 plume signal of Berlin is typically only about 0.2 to 1.0 ppm, it is critical to accurately estimate the background XCO2 value in Eq. (2). The spatial variability σbg of the background, on the other hand, is typically only of the order of a few tenths of a part per million. Despite higher XCO2 in winter than in summer, the variability is somewhat larger in summer due to stronger biospheric activity in combination with lower average wind speeds, especially in July and August. Large peaks in the background variability are often caused by plumes from other anthropogenic sources such as the power stations in the southeast of Berlin (Fig. 1).
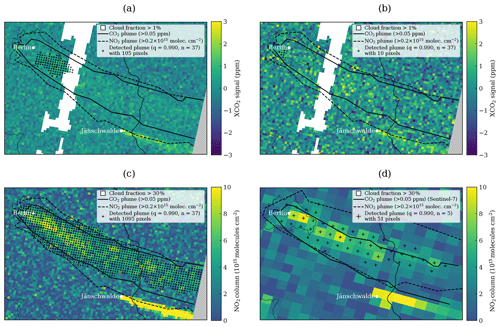
Figure 8Example of plume detection with CO2M's CO2 and NO2 instrument and Sentinel-5's NO2 instrument on 21 April 2015. Significant pixels detected by the algorithm are highlighted as black crosses. The outlines of the true CO2 and NO2 plumes based on the XBER tracers are overlaid as solid and dashed lines, respectively. (a) Low-noise CO2 instrument. (b) High-noise CO2 instrument. (c) High-noise NO2 instrument on the CO2M satellite. (d) NO2 instrument on Sentinel-5.
For NO2, the annual cycle of the background is relatively constant for our idealized NO2 tracer with a constant lifetime of 4 h (Fig. 7e and f). In reality, the lifetime will likely be longer and the variability correspondingly higher in winter. The NO2 signal of Berlin is significantly larger than the background and its variability. Similar to CO2, the CO time series has a strong annual cycle with an amplitude of about 5×1017 molec. cm−2 (Fig. 7h and i) requiring again an accurate estimation of the background. The standard deviation of the background is about half of the CO signal.
Table 6 summarizes the median of all SNRs of Berlin and the two power stations for the different satellite instrument scenarios that have been computed from the time series of highest signals. To understand the numbers, it should be noted that a plume pixel would be detectable when the SNR is larger than 2.3; i.e., z(q)=2.3 for q=99 %. For Berlin, the CO2 SNRs are below this detection limit for all noise levels while NO2 SNRs are above the limit. For the two power stations, SNRs are above the detection limit both for the CO2 and NO2 instrument scenarios, but SNRs for the NO2 instrument scenarios are always larger.
Based on the SNRs, the NO2 plumes should be well detectable. For Berlin, the detection of the CO2 plume with the CO2 instrument will often be challenging due to low SNRs. The CO SNRs are always much smaller than those for CO2, making a CO instrument with the given specifications less suitable for the purpose of plume detection. In the following, we therefore only investigate the potential benefit of auxiliary NO2 observations.
3.3 Plume detection algorithm
The plume detection algorithm was applied to the CO2 plumes of Berlin and Jänschwalde for different instrument scenarios. The probability q was set to 99 % and neighborhood sizes of 37 and 5 were selected for Berlin and the power stations, respectively. In the case of Sentinel-5, the corresponding neighborhood sizes were set to 5 and 1 due the larger pixels of this satellite. Based on an analysis of the positive predictive values, these neighborhood sizes were found most suitable for detecting the city and power plant plumes (Kuhlmann et al., 2019). For Berlin, 20 synthetic satellite images were created for each single overpass with different patterns of random noise. The plume detection algorithm was subsequently applied to each image, and the results were averaged to obtain more robust results independent of the selected noise pattern. For Jänschwalde, only one synthetic satellite image was created for each overpass because no model tracer was available to compare the results with a true plume.
3.3.1 Examples of detected plumes from Berlin
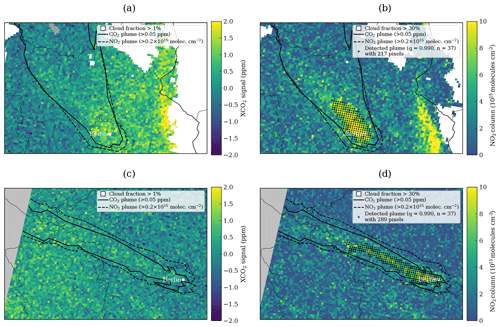
Figure 9(a) Example of 27 February 2015 where plume detection with a CO2 instrument fails because of pronounced horizontal gradients in the CO2 background field. (c) Example of 2 July 2015 where the plume detection fails due to small CO2 signals as a result of high wind speeds. In both cases, the plume can readily be detected with a high-noise NO2 instrument (panels b and d).
Figure 8 shows the CO2 and NO2 plumes of Berlin on 21 April 2015 observed by CO2M and Sentinel-5 for different instrument scenarios. The outlines of the real plumes are overlaid as solid and dashed lines for CO2 and NO2, respectively. Since the CO2 instruments have a lower cloud threshold, a band of cirrus clouds is obscuring the plume in the CO2 observations but not in NO2. Successfully detected pixels are shown as black crosses, and the number of detected pixels (median of all 20 noise realizations) are presented in the legend. On average, a CO2 instrument detects 116±46, 48±40 and 24±30 pixels with noise scenarios σVEG50 of 0.5, 0.7 and 1.0 ppm, respectively (Fig. 8a and b). The number of true positives is slightly smaller, having on average 2 false-positive pixels. Consequently, the PPV is high, ranging between 0.85 and 0.99 for high- and low-noise, respectively.
For the NO2 measurement the band of thin cirrus clouds is not an issue. The NO2 instrument can therefore detect a much larger number of pixels, i.e., 1242±99 and 1203±155 in the case of the low-noise and the high-noise scenarios, respectively (Fig. 8c). On average, the fraction of FPs is relatively large, and the PPV is only 0.80±0.05 and 0.77±0.10 for the low- and high-noise scenarios, respectively. The small PPV is caused by interference with the plume of Jänschwalde, which is just south of the plume of Berlin. For cases where no neighboring plumes have been detected falsely with the NO2 instrument, the spatial match between CO2 and NO2 plumes is generally high, suggesting a high degree of spatial overlap between the CO2 and NO2 plumes.
The Sentinel-5 NO2 instrument is also able to detect the CO2 plume with 879±114 CO2M pixels, but since it is measured 2 h earlier, the NO2 plume seen by Sentinel-5 (dashed line in Fig. 8d) is slightly shifted with respect to the CO2 plume (solid line). As a consequence, the PPVs is low (0.60±0.04).
Figure 9 presents two examples where the CO2 instrument fails to detect the CO2 plume. In Fig. 9a, the CO2 field has a pronounced spatial gradient resulting in a high variance of the background. This gradient is not present in the much-shorter-lived trace gas NO2, making it possible to detect the plume using an NO2 instrument (Fig. 9b). Similar situations occur in roughly 20 % of cloud-free swaths. Figure 9c and d show a second example where the CO2 instrument cannot detect the plume because the signal is very weak due to strong winds. Owing to its better SNR, the NO2 instrument is able to detect the plume also in this situation.
Figure 10 presents two examples comparing the NO2 plume observed by Sentinel-5 to the CO2 plume observed 2 h later by the CO2M satellite. In the first example (panels a and b), Sentinel-5 fails to detect any plume due to clouds, which have largely disappeared by the time of the CO2M overpass. In the second example, both Sentinel-5 and the CO2M satellite detect a plume of similar size, but the Sentinel-5 plume is significantly displaced due to changes in the prevailing winds between the two overpasses.
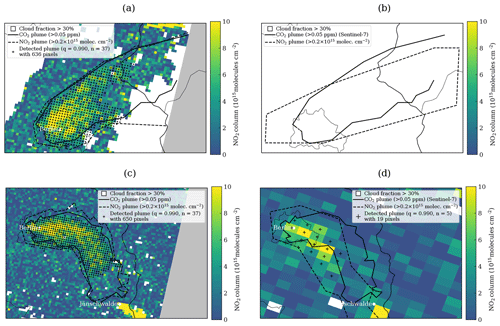
Figure 10Examples of comparing plume detection between the (a, c) CO2M's and (b, d) Sentinel-5's NO2 instruments. (a, b) Due to a time lag of 2 h, the scene is cloudy during the Sentinel-5 overpass but not during the CO2M overpass (3 December 2015). (c, d) The plume position clearly changed between the two overpasses (17 June 2015).
3.3.2 Number and sizes of detected Berlin plumes
To count the number of plumes detectable under the different instrument scenarios, we analyze the 50 plumes observed by a constellation of six satellites, which we had classified in Sect. 3.1 as being potentially useful based on the idealized tracer XBER having more than 100 pixels above a threshold of 0.05 ppm. Table 7 summarizes the results in terms of number and size of the detected plumes. A plume was only counted as detected when at least 100 CO2 pixels were correctly detected (true positives) and when at least 80 % of the detected pixels were true positives (PPV ≥ 0.80). The PPV threshold was found useful for removing plumes interfering with others or plumes shifted due to the earlier overpass time of Sentinel-5.
Table 7 shows that the CO2 instruments detect significantly fewer plumes than the NO2 instruments. Depending on the instrument noise scenario, the CO2 instruments detect plumes with more than 100 pixels with a success rate of only 12 % to 32 %, while for the NO2 instruments the success rates are 68 % to 70 %. Surprisingly, the NO2 instrument with low noise performs slightly worse than the high-noise instrument. This is an artifact of the algorithm often detecting small plumes not related to emissions from Berlin in the case of a low-noise instrument. The Sentinel-5 NO2 instrument detects 20 % of the plumes, thus only half the success rate of the NO2 instrument on the CO2M satellite. The main reason for this low success rate is the spatial mismatch of the plumes due to the 2 h difference in overpass times.
Table 7Number of CO2 plumes from Berlin and the 5th, 50th and 95th percentiles of the number of detected CO2 pixels (TP if PPV ≥ 0.8 and cloud fraction <1 %) for a constellation of six CO2M satellites or one Sentinel-5 satellite. The maximum number of detectable plumes would be 50, corresponding to all potentially useful plumes with at least 100 CO2 pixels with values of the tracer XBER above a threshold of 0.05 ppm.
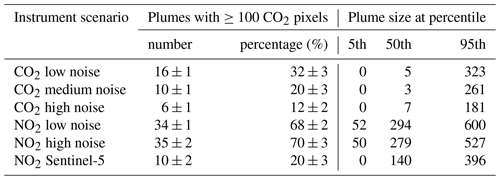
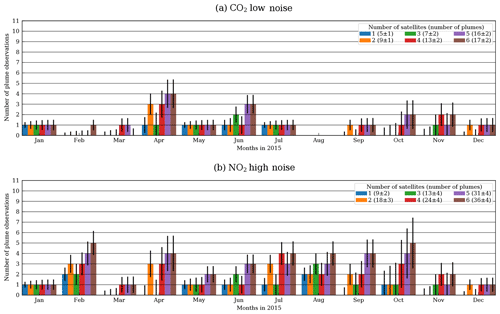
Figure 11Number of detected plumes with at least 100 pixels (TP ≥ 100 and PPV ≥ 0.80) for one to six satellites with the (a) CO2 low-noise and (b) NO2 high-noise scenario. Error bars were estimated from all available satellites with different Equator starting longitudes.
Figure 11 shows the number of plumes per month with at least 100 detected pixels for different constellations between one and six satellites for the CO2 low-noise and the NO2 high-noise scenario. The number of plume detections per month is small and therefore highly sensitive to the specific orbit configuration. For example, two satellites seem to detect more plumes than three, but this result is caused by an unfavorable orbit for observing Berlin for the constellation with three satellites and unfavorable cloud cover as already discussed earlier. The standard deviation was estimated from the number of detectable plumes using satellites with different starting longitudes (i.e., east–west displacements of all orbits). The figure shows that the number of observed plumes generally increases with the number of satellites as expected, but statistical noise can mask the increase from one constellation to the next. The figure confirms the much lower success rates of the CO2 instruments as compared to the NO2 instruments as expected from the computed signal-to-noise ratios.
3.3.3 Detection of plumes from power stations
There are six major power plants in the model domain: Jänschwalde, Boxberg, Schwarze Pumpe, Lippendorf, Turów and Pątnów. Because no model tracer was defined for individual power plants but instead only for the sum of all of them, the true plume of an individual power plant is not known. Therefore, we applied a visual inspection to identify those plume detections which erroneously included neighboring plumes. Furthermore, we limit the analysis to Jänschwalde.
Table 8Number of plumes detected for Jänschwalde with six satellites. The number of plumes are provided for plumes with at least 10 detected CO2 or NO2 pixels and in addition with at least 10 CO2 pixels (cloud cover <1 %). In both cases, plumes were included where neighboring plumes were detected in addition to Jänschwalde, i.e., detection with a large number of false positives (e.g., Fig. 13c). These plumes are also shown separately. The classification uncertainty is about ±5 plumes. The neighborhood size was set to ns=5.
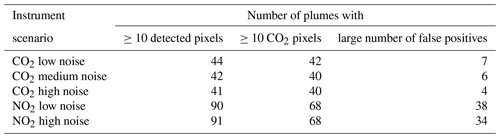
As an example, Fig. 12 shows the successful detection of the CO2 plume of Jänschwalde on 2 November 2015 by different instruments. Since CO2 emissions of Jänschwalde are high with 33.3 Mt CO2 yr−1, the XCO2 signal is very strong and can be detected well even with a high-noise instrument (σVEG50=1.0 ppm). With low noise (σVEG50=0.5 ppm) the weaker plumes of Schwarze Pumpe and Boxberg are visible as well. The NO2 instrument detects the four plumes in the region well. On this day also the Sentinel-5 NO2 instrument successfully detects the plume of Jänschwalde and other point sources. Figure 13 presents a second, more challenging example for 17 February 2015. The CO2 instrument successfully detects the plume with 0.5 ppm uncertainty, but with 1.0 ppm uncertainty, the number of detected pixels is likely too small to be useful for emission estimation. The reason for the low number of detected pixels in this case is the strong horizontal gradient in the CO2 background. The NO2 instrument detects the plume, but because the NO2 plume of Jänschwalde overlaps with neighboring plumes, these plumes are erroneously assigned to Jänschwalde as well. At the coarser resolution of Sentinel-5 the plumes of the individual power plants can hardly be separated and, moreover, the time difference of 2 h results in a plume location that is shifted with respect to the plume seen by the CO2M satellite.
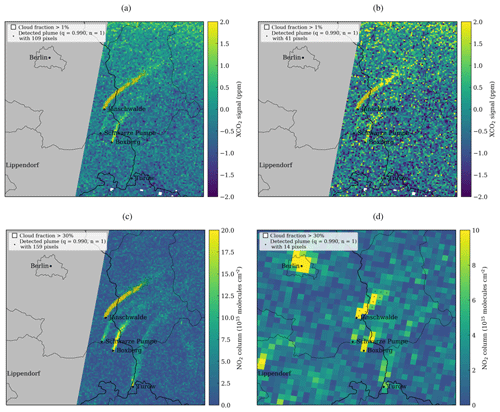
Figure 12Example of plume detection for the Jänschwalde power plant on 2 November 2015 using (a, b) the CO2 instrument with σVEG50 of 0.5 and 1.0 ppm, (c) the NO2 instrument with the high-noise scenario and (d) the NO2 instrument on Sentinel-5.
Table 8 summarizes the results of the plume detection for Jänschwalde under the different instrument scenarios. It shows the number of detected plumes with at least 10 pixels and, in addition, the number of plumes with at least 10 valid CO2 pixels (cloud cover <1 %). Note that, in the case of the much narrower plumes from power plants, fewer pixels are required to form a useful plume. We classified detections that include large parts of the background as failed but still counted detections that include neighboring plumes as successful (e.g., Fig. 13c) because they successfully identified the location of the plume. Since the classification is not always unambiguous, we assigned an uncertainty of about ±5 plumes at most.
In the year 2015, the number of detectable plumes with more than 10 pixels for a constellation of six satellites was between 40 and 45 for a CO2 instrument with σVEG50 of 0.5, 0.7 and 1.0 ppm. At the same time, the NO2 instrument detected about 90 plumes for the low- and high-noise scenario. When only plumes with more than 10 cloud-free CO2 observations were considered, the number was reduced to about 70 plumes. For a smaller number of satellites, the number of detectable plumes would be correspondingly smaller. The NO2 instrument detects more plumes because of its lower sensitivity to clouds, which makes it possible to trace the plume to the source even for partly cloudy scenes. On the other hand, the NO2 instrument often detects overlapping plumes (e.g., Boxberg and Schwarze Pumpe) because the instrument is much more sensitive to small signals further away from the origin than the CO2 instrument. The mean plume size was about 100 pixels for the low-noise CO2 instrument. The plumes detected with the high-noise CO2 instrument were about half the size. The NO2 instruments detected a similar number of CO2 pixels to the low-noise CO2 instrument, but when all detected pixels are counted the number of pixels doubles.
4.1 Comparison with previous studies
In this study we investigated whether and how frequently the CO2 plume of Berlin and power stations can be detected by different constellations of satellites using either CO2 observations alone or in combination with observations of the coemitted trace gases CO and NO2. To address the question, high-resolution CO2, CO and NO2 fields were simulated with the COSMO-GHG model for the year 2015 and used to generate synthetic XCO2, CO and NO2 satellite observations for Sentinel-5 and a constellation of CO2M satellites. Similar OSSEs studies were conducted by Pillai et al. (2016) and Broquet et al. (2018) for Berlin and Paris, respectively, as part of the LOGOFLUX study (Bacour et al., 2015). However, their simulations did not include NO2 and CO fields. A fundamental difference of our study compared to previous studies is the realistic, i.e., not as a random noise, account for transport model errors in the present study, where the location of the plume is not taken from the model but detected in the satellite image using either CO2 or NO2 observations. For this reason, the focus of this paper is on the detectability of the plume, while Pillai et al. (2016) and Broquet et al. (2018) focused on the inversion, which we will describe in a follow-up publication.
Pillai et al. (2016) simulated CO2 fields with the WRF-GHG model with 10 km× 10 km spatial resolution for the year 2008. The resolution was relatively low compared to the 1.1 km× 1.1 km resolution used in our study. They used CO2 emissions from the EDGAR inventory (version 4.1), which are more than twice as high as the emissions reported in the inventory of the city of Berlin as mentioned earlier. A consequence of the unrealistic high emissions is higher CO2 signals (0.80–1.35 ppm) for Berlin than in our study (0.16–1.03 ppm; see Table 5). Note that the signal strength also depends on the spatial resolution, but our XCO2 signals were computed for a local mean (ns=37, i.e., 148 km2 spatial resolution) that is comparable to the model resolution used by Pillai et al. (2016). For Paris, Broquet et al. (2018) conducted simulations with the CHIMERE atmospheric transport model with 2 km spatial resolution. CO2 emissions for the greater urban area were 40–50 Mt CO2 yr−1, which resulted in a XCO2 signal of ∼1 ppm, quite consistent with the plume signals reported here.
For Berlin, we estimated that 3 to 13 potentially useful CO2 plumes (defined as plumes with at least 100 cloud-free pixels above a threshold of 0.05 ppm) would be observable, but not necessarily detectable, during 1 year by a single CO2M satellite with a 250 km wide swath. Pillai et al. (2016) identified 41 potentially useful orbits for estimating emissions with a 500 km wide swath. Although a direct comparison of these two numbers is difficult because of the different swath widths and the different definitions of “usefulness”, we can still conclude that our study identified significantly fewer plumes than Pillai et al. (2016) even after halving their number to account for their wider swath.
Clouds have a strong impact on the number of cloud-free observations. A major difference between Pillai et al. (2016) and our study is the different approaches for masking cloudy observations. Pillai et al. (2016) used the MODIS cloud mask product (MOD35_L2) while we used cloud masks derived from cloud fractions simulated with the COSMO-GHG model. To compare these two approaches, we computed the number of cloud-free pixels using the MODIS cloud mask product (MOD35_L2), the MODIS cloud product (MOD06_L2) and COSMO-GHG simulations. The COSMO-GHG cloud fractions were spatially averaged over the MODIS pixels. The comparison shows that monthly fractions of cloud-free pixels agree well between masks computed from COSMO-GHG and MODIS cloud fractions for both a 1 % and 30 % cloud fraction, while the cloud-free pixels based on the MODIS cloud mask product are about twice as high (Fig. S1 in the Supplement). The larger fraction of cloud-free observations obtained from the MODIS cloud mask product is also consistent with a validation study showing that the product is not very sensitive to optically thin clouds (Ackerman et al., 2008). The differences likely explain the different number of potentially useful orbits between Pillai et al. (2016) and our study. It also suggests that Pillai et al. (2016) overestimate the number of potentially useful orbits while our results are likely more accurate.
Further differences are to be expected because small plumes with less than 100 pixels were excluded in our case and because of different meteorology, especially cloud cover and wind speed, for the different simulation periods. In addition, Pillai et al. (2016) used higher emissions and did not consider vertical profiles of emissions, which results in stronger and correspondingly larger plumes, which are less likely to be fully covered by clouds (Brunner et al., 2019).
Since the number of useful plumes observable by a satellite critically depends on its orbit (with one or two overpasses over Berlin per 11 d repeat cycle), the number of useful plumes per satellite may easily be overestimated if only an optimal orbit is considered. Comparing different Equator starting longitudes as applied in this study reduces the sensitivity to a specific orbit selection.
4.2 Benefits of CO and NO2 measurements
Out of 50 potentially useful plumes, many plumes were too weak to be easily seen by the CO2 instrument. Plumes of Berlin with more than 100 detectable pixels could only be detected in about 12 % of cloud-free cases with a high-noise instrument (σVEG50=1.0 ppm) and about 32 % with a low-noise instrument (σVEG50=0.5 ppm). The success rate of 32 % for an imperfect but still precise instrument (σVEG50=0.5 ppm) would only allow for two to three favorable plume observations per year and satellite. These numbers illustrate the challenge and call for a larger constellation or a wider swath to increase the opportunities for plume detection and emission quantification, and for a CO2 instrument with as low noise as possible.
Adding an NO2 instrument greatly enhanced the opportunities for detecting the CO2 plumes (68 %–70 % of cloud-free cases), since the NO2 plumes largely overlap with the CO2 plumes and since the signal-to-noise ratio is better for the NO2 instrument. Furthermore, variability in the background is less important than for CO2, and the NO2 observations are less sensitive to clouds. Nevertheless, the number of detectable plumes with an NO2 instrument remained small with five to six per satellite and year. A CO instrument with specifications similar to the CO instrument on Sentinel-5 had a smaller signal-to-noise ratio than the CO2 instrument. Such an instrument would add little useful information over a developed region like Germany where combustion processes are well controlled and CO:CO2 emission ratios are correspondingly small. The question of whether CO signals would be sufficiently high in other regions of the globe was outside the scope of this study.
The Sentinel-5 NO2 instrument is well suited to detect the NO2 plume of Berlin. However, the different overpass times of the CO2M satellite (11:30 local time) and Sentinel-5 (09:30 local time) frequently resulted in a significant spatial mismatch between the plumes, which reduced the number of matching plumes to 20 % of the cloud-free cases. This is similar to the CO2 instrument with medium noise but 3 to 4 times lower than in the case of an NO2 instrument placed directly on the CO2M satellite.
The detection of plumes from strong point sources like the Jänschwalde power plant was easier than the detection of city plumes because point sources tend to have stronger and more confined CO2 plumes for the same amount of emitted CO2. In addition, the number of pixels required to map out such a plume was smaller, with only 10 detectable pixels being typically sufficient for identifying the main part of the plume. With a constellation of six satellites, about 40–45 plumes from Jänschwalde (33.3 Mt CO2 yr−1 emissions) with more than 10 detectable pixels could be observed per year even with a high-noise CO2 instrument. This corresponds to six to eight plumes per satellite and year, which is significantly better than for Berlin with the best instrument. The number of detectable plumes further increased by about 50 % with the NO2 instrument (about 70 plumes). Smaller point sources with emissions of about 10 Mt CO2 yr−1 (e.g., Lippendorf, Schwarze Pumpe and Turow) were sometimes detectable with a low-noise CO2 instrument but could also be detected with an NO2 instrument.
Our study did not include systematic errors in the satellite observations from aerosols, clouds and surface reflectance, which can result in spatial patterns resembling plume structures and therefore complicate plume detection. We therefore might overestimate the number of detectable plumes. Although systematic errors affect all satellite products, the effect would be more severe for XCO2 than for NO2 due to the much smaller signal-to-noise ratios. How much aerosols enhance measurement uncertainties and correspondingly reduce the ability to detect plumes cannot be quantified here but will be studied, for example, in a study on the use of aerosol information for estimating fossil fuel CO2 emissions (AEROCARB) performed by a consortium led by SRON.
Our study could not simulate detailed NOX chemistry due to the high computational costs. We can therefore not rule out a larger mismatch between some CO2 and NO2 plumes than simulated here, for example, because of different NO2 decay rates at different altitudes. However, Reuter et al. (2019) found no obvious mismatch when comparing colocated CO2 and NO2 plumes from OCO-2 and TROPOMI observations. A follow-up study for quantifying the effect of NOx chemistry would certainly be desirable.
Since the shape and extent of the plume can be imaged more accurately with an NO2 instrument on the CO2M satellites, the NO2 instrument can also be used to assess and correct transport simulations and improve these simulations through data assimilation.
4.3 Limitations of the current plume detection algorithm
The main objective of this study was to compare the basic detectability of CO2 plumes with CO2, NO2 and CO observations. Our plume detection algorithm was able to detect weak signals well below the single sounding precision but tended to fail when the CO2 or NO2 field was complex, for example when several plumes from adjacent sources overlapped or when the background had a spatial gradient. These cases can be easily identified by a trained human observer as done in this study, but will have to be automatized for application at the global scale, for example by applying machine-learning methods.
In our study area, the attribution of detected enhancements to sources was relatively simple because the locations of the sources were known and plumes were rarely overlapping with plumes from other sources. Source attribution can be more challenging when source locations are not precisely known or when several sources are close to each other. For such cases, the algorithm will have to be extended to be operationally applicable.
The algorithm assumed accurate knowledge of the mean and variance of the background, which were estimated directly from a simulated background tracer. It is possible that due to this optimistic assumption the number of detectable plumes was overestimated. On the other hand, mean and variance were computed in a rather simple way from a large window centered on the source (200 km× 200 km for Berlin). In the present algorithm, spatial gradients in the background field contributed to the variance of the background and thus reduced the ability for plume detection. However, such gradients, if sufficiently smooth, could potentially be accounted for in a more advanced algorithm through spatial interpolation of the background surrounding the plume.
When applied to real satellite observations the background and its variance could either be taken from a model or estimated directly from the satellite observations. Although atmospheric transport models have large uncertainties at the level of individual plumes, they could provide reasonable estimates of the CO2 and NO2 background. Likely the best option is to derive the background directly from the satellite data, which requires further development of the plume detection algorithm presented here. An improved algorithm could start with an a priori estimate of plume location and background and would then be updated iteratively to improve both plume location and background. A model could be used here to determine a suitable a priori plume location and background. An improved version of the algorithm presented in this paper has the potential for increasing the number of detectable plumes per satellite as well as the number of CO2 pixels per plume.
In this paper the potential for detecting CO2 plumes of the city of Berlin and neighboring power stations was investigated for the Copernicus anthropogenic CO2 monitoring mission (CO2M), which is a proposed constellation of CO2 satellites of the European Copernicus program. Since the interference of biospheric CO2 makes the identification of weak anthropogenic CO2 plumes challenging, plumes were detected either from CO2 observations or from observations of the coemitted trace gases CO and NO2. The study used high-resolution atmospheric transport simulations to create realistic CO2, CO and NO2 fields at 1 km× 1 km horizontal resolution to generate synthetic observations of XCO2, CO and NO2 for constellations of up to six CO2M satellites and one Sentinel-5 satellite.
For the city of Berlin about 50 ± 5 potentially useful CO2 plumes were identified for the year 2015 for a constellation of six satellites, i.e., about eight plumes could be observed by a single CO2M satellite per year. This number is somewhat smaller than reported in earlier studies (Bacour et al., 2015; Pillai et al., 2016), mainly because masking cloudy pixels based on the simulated cloud fields leads to less cloud-free observations than using the MODIS cloud mask product. Many of these 50 potentially observable plumes were too weak to be easily detectable by the CO2 instrument. Plumes with more than 100 detectable pixels could only be identified in 12 % and 32 % of cloud-free cases with a high-noise (σVEG50=1.0 ppm) and low-noise CO2 instrument (σVEG50=0.5 ppm), respectively. A CO instrument with the uncertainty scenario used in this study had a signal-to-noise ratio that was lower than for the CO2 instrument and was therefore not suitable for detecting CO2 plumes. On the other hand, adding an NO2 instrument significantly increased the number of detectable plumes (68 %–70 % of cloud-free cases) because CO2 and NO2 plumes generally overlapped well. The better performance of the NO2 instrument was partly due to the higher signal-to-noise ratio and partly due to the lower sensitivity to clouds. The Sentinel-5 instrument was also well suited to detect the NO2 plumes, but the different overpass times of the CO2M satellites (11:30 local time) and Sentinel-5 (09:30 local time) often resulted in a large spatial mismatch.
Strong point sources like the Jänschwalde power plant could be detected more easily with the CO2 instrument (40–45 plumes) because the plumes were spatially more confined and the signals were stronger. The number of detectable plumes increased further with the NO2 instrument by about 50 % (about 70 plumes). The Sentinel-5 instrument could also detect the CO2 plume but could not always distinguish the plumes from neighboring power plants due to the lower spatial resolution. In addition, the spatial mismatch between CO2M and Sentinel-5 was large due to the 2 h time difference between overpasses. Smaller point sources with emissions of about 10 Mt CO2 yr−1 were only detectable with a low-noise CO2 instrument but were in most cases readily detectable with an NO2 instrument.
In this study, power plant plumes could be detected even with an NO2 instrument with high noise. The power plants were equipped with wet scrubber technology for reducing SO2 and NOx emissions but not with the latest available technology. Future updates using selective catalytic or noncatalytic reduction have the potential to further reduce NOx emissions by 20 % to 50 % (Lecomte et al., 2017), which would place higher requirements on the NO2 instrument and would make the low-noise scenario more beneficial.
This study demonstrates the huge benefit of adding an NO2 instrument to a constellation of CO2M satellites for detecting city plumes and weaker point sources. The major advantages of the NO2 instruments are the higher signal-to-noise ratio and the lower sensitivity to clouds. Therefore, adding an NO2 instrument is highly recommended and the low-noise instrument is preferable for detecting also weaker and cleaner plumes in terms of NO2 emissions. Furthermore, development of an advanced plume detection algorithm that can detect CO2 plumes reliably will be essential for the application on an operational satellite.
Column-averaged dry air mole fractions of all simulated tracers are available both as 2-D fields and as synthetic satellite products through ESA. The total three-dimensional model output amounts to 7.5 TB and is archived on Empa servers. Selected fields or time periods can be made available upon request. The TNO/MACC-3 inventory can be requested for research purposes from Hugo Denier van der Gon (TNO, the Netherlands). The TNO-CAMS CO2 emission inventory is publicly available at https://doi.org/10.5281/zenodo.112889 (Kuenen et al., 2017). The emissions inventory of Berlin was kindly provided by Andreas Kerschbaumer, Senatsverwaltung Berlin, and is available for research upon request. The global CO2 simulation, which provided the lateral boundary conditions, was conducted in the framework of the European Earth observation program Copernicus and can be retrieved from ECMWF's MARS archive as experiment gvri, stream lwda, class rd.
The supplement related to this article is available online at: https://doi.org/10.5194/amt-12-6695-2019-supplement.
GK conducted the simulations, generated the satellite observations, developed the plume detection algorithm and wrote the manuscript with input from all coauthors. GB followed the project as external advisor and contributed critical input to the manuscript. JM contributed the VPRM flux data required for the simulations. VC ported the COSMO-GHG extension to GPUs. YM and AL accompanied the study as ESA project and technical officers, respectively, and provided critical inputs and reviews during all phases of the project. DB led the SMARTCARB project and contributed critical input to the manuscript.
The authors declare that they have no conflict of interest.
This study was conducted in the context of the project SMARTCARB funded by the European Space Agency (ESA). The views expressed here can in no way be taken to reflect the official opinion of ESA. The work was supported by a grant from the Swiss National Supercomputing Centre (CSCS) under project ID d73. We acknowledge the contributions of the Federal Office for Meteorology and Climatology (MeteoSwiss), the Swiss National Supercomputing Centre (CSCS) and ETH Zurich to the development of the GPU-accelerated version of COSMO. We would like to thank Oliver Fuhrer (MeteoSwiss), who supported the porting of COSMO-GHG to GPUs and the setup of the model on the supercomputer at CSCS. We would like to acknowledge Richard Engelen and Anna Agusti-Pannareda (ECMWF) as well as the European Earth Observation Program Copernicus and the EU project CHE for providing support and access to global CO2 model simulation fields. The TNO/MACC-3 emissions inventory and temporal emission profiles were kindly provided by Hugo Denier van der Gon (TNO, the Netherlands). We would also like to thank Jochen Landgraf and Joost aan de Brugh (SRON) for providing the orbit simulator. We are also very grateful to Andreas Kerschbaumer, Senatsverwaltung Berlin, for providing the emission inventory of Berlin and additional material as well as for being available for discussions on its proper usage.
This research has been supported by the European Space Agency (contract no. 4000119599/16/NL/FF/mg).
This paper was edited by Ilse Aben and reviewed by two anonymous referees.
Ackerman, S., Holz, R., Frey, R., Eloranta, E., Maddux, B., and McGill, M.: Cloud detection with MODIS. Part II: validation, J. Atmos. Ocean. Tech., 25, 1073–1086, https://doi.org/10.1175/2007JTECHA1053.1, 2008. a
Ackerman, S., Menzel, P., Frey R., and Baum, B.: MODIS Atmosphere L2 Cloud Mask Product. NASA MODIS Adaptive Processing System, Goddard Space Flight Center, https://doi.org/10.5067/MODIS/MYD35_L2.061, 2017. a
Agustí-Panareda, A., Massart, S., Chevallier, F., Boussetta, S., Balsamo, G., Beljaars, A., Ciais, P., Deutscher, N. M., Engelen, R., Jones, L., Kivi, R., Paris, J.-D., Peuch, V.-H., Sherlock, V., Vermeulen, A. T., Wennberg, P. O., and Wunch, D.: Forecasting global atmospheric CO2, Atmos. Chem. Phys., 14, 11959–11983, https://doi.org/10.5194/acp-14-11959-2014, 2014. a
AVISO GmbH and IE Leipzig: Erstellung der Berliner Emissionskataster Industrie, Gebäudeheizung, sonstiger Verkehr, Kleingewerbe, sonstige Quellen, Baustellen – Schlussbericht Juni 2016, Tech. rep., available at: https://www.berlin.de/senuvk/umwelt/luftqualitaet/de/emissionen/download/Endbericht_Emissionkataster_2015.pdf (last access: 25 November 2019), 2016. a
Bacour, C., Boesch, H., Bovensmann, H., Breon, F.-M., Broquet, G., Buchwitz, M., Houweling, S., Klonecki, A., Krings, T., and Santaren, D.: LOGOFLUX 2 – CarbonSat Earth Explorer 8 Candidate Mission – Inverse Modelling and Mission Performance Study, Final report of ESA study contract n°4000109818/14/NL/FF/lf, project led by NOVELTIS (France), Report, 2015. a, b, c
Baldauf, M., Seifert, A., Forstner, J., Majewski, D., Raschendorfer, M., and Reinhardt, T.: Operational convective-scale numerical weather prediction with the COSMO model: description and sensitivities, Mon. Weather Rev., 139, 3887–3905, https://doi.org/10.1175/Mwr-D-10-05013.1, 2011. a
Boersma, K. F., Eskes, H. J., and Brinksma, E. J.: Error analysis for tropospheric NO2 retrieval from space, J. Geophys. Res., 109, D04311, https://doi.org/10.1029/2003JD003962, 2004. a
Boersma, K. F., Eskes, H. J., Dirksen, R. J., van der A, R. J., Veefkind, J. P., Stammes, P., Huijnen, V., Kleipool, Q. L., Sneep, M., Claas, J., Leitão, J., Richter, A., Zhou, Y., and Brunner, D.: An improved tropospheric NO2 column retrieval algorithm for the Ozone Monitoring Instrument, Atmos. Meas. Tech., 4, 1905–1928, https://doi.org/10.5194/amt-4-1905-2011, 2011. a
Bovensmann, H., Buchwitz, M., Burrows, J. P., Reuter, M., Krings, T., Gerilowski, K., Schneising, O., Heymann, J., Tretner, A., and Erzinger, J.: A remote sensing technique for global monitoring of power plant CO2 emissions from space and related applications, Atmos. Meas. Tech., 3, 781–811, https://doi.org/10.5194/amt-3-781-2010, 2010. a
Broquet, G., Bréon, F.-M., Renault, E., Buchwitz, M., Reuter, M., Bovensmann, H., Chevallier, F., Wu, L., and Ciais, P.: The potential of satellite spectro-imagery for monitoring CO2 emissions from large cities, Atmos. Meas. Tech., 11, 681–708, https://doi.org/10.5194/amt-11-681-2018, 2018. a, b, c, d, e, f
Brunner, D., Kuhlmann, G., Marshall, J., Clément, V., Fuhrer, O., Broquet, G., Löscher, A., and Meijer, Y.: Accounting for the vertical distribution of emissions in atmospheric CO2 simulations, Atmos. Chem. Phys., 19, 4541–4559, https://doi.org/10.5194/acp-19-4541-2019, 2019. a, b, c, d, e, f
Buchwitz, M., Reuter, M., Bovensmann, H., Pillai, D., Heymann, J., Schneising, O., Rozanov, V., Krings, T., Burrows, J. P., Boesch, H., Gerbig, C., Meijer, Y., and Löscher, A.: Carbon Monitoring Satellite (CarbonSat): assessment of atmospheric CO2 and CH4 retrieval errors by error parameterization, Atmos. Meas. Tech., 6, 3477–3500, https://doi.org/10.5194/amt-6-3477-2013, 2013. a, b, c
C40 cities: 27 C40 cities have peaked their greenhouse gas emissions, available at: https://c40-production-images.s3.amazonaws.com/other_uploads/images/1923_Peaking_emissions_Media_Pack_Extended_version.original.pdf?1536847923 (last access: 25 November 2019), 2018. a
Chimot, J., Breon, F.-M., Prunet, P., Vinuesa, J.-F., Camy-Peyret, C., Broquet, G., Chevallier, F., Renault, E., Houweling, S., Buchwitz, M., Bovensmann, H., Pillai, D., Reuter, M., Marshall, J., Brunner, D., Bergamaschi, P., Ciais, P., and Klonecki, A.: LOGOFLUX – CarbonSat Earth Explorer 8 Candidate Mission – Inverse Modelling and Mission Performance Study, Final report of ESA study contract n°40010537/12/NL/CO, project led by NOVELTIS (France), Report, 2013. a
Ciais, P., Crisp, D., Gon, H. v. d., Engelen, R., Heimann, M., Janssens-Maenhout, G., Rayner, P., and Scholze, M.: Towards a European Operational Observing System to Monitor Fossil CO2 emissions – Final Report from the expert group, European Commission, Copernicus Climate Change Service, Report, 2015. a, b, c
Crisp, D., Pollock, H. R., Rosenberg, R., Chapsky, L., Lee, R. A. M., Oyafuso, F. A., Frankenberg, C., O'Dell, C. W., Bruegge, C. J., Doran, G. B., Eldering, A., Fisher, B. M., Fu, D., Gunson, M. R., Mandrake, L., Osterman, G. B., Schwandner, F. M., Sun, K., Taylor, T. E., Wennberg, P. O., and Wunch, D.: The on-orbit performance of the Orbiting Carbon Observatory-2 (OCO-2) instrument and its radiometrically calibrated products, Atmos. Meas. Tech., 10, 59–81, https://doi.org/10.5194/amt-10-59-2017, 2017. a
Deeter, M. N., Edwards, D. P., Francis, G. L., Gille, J. C., Martínez-Alonso, S., Worden, H. M., and Sweeney, C.: A climate-scale satellite record for carbon monoxide: the MOPITT Version 7 product, Atmos. Meas. Tech., 10, 2533–2555, https://doi.org/10.5194/amt-10-2533-2017, 2017. a
Düring, I., Bächlin, W., Ketzel, M., Baum, A., Friedrich, U., and Wurzler, S.: A new simplified NO∕NO2 conversion model under consideration of direct NO2-emissions, Meteorol. Z., 20, 67–73, https://doi.org/10.1127/0941-2948/2011/0491, 2011. a, b
ESA: Report for mission selection: CarbonSat, ESA SP-1330/1 (2 volume series), Report, 2015. a
Fioletov, V. E., McLinden, C. A., Krotkov, N., and Li, C.: Lifetimes and emissions of SO2 from point sources estimated from OMI, Geophys. Res. Lett., 42, 1969–1976, https://doi.org/10.1002/2015GL063148, 2015. a
Flemming, J., Huijnen, V., Arteta, J., Bechtold, P., Beljaars, A., Blechschmidt, A.-M., Diamantakis, M., Engelen, R. J., Gaudel, A., Inness, A., Jones, L., Josse, B., Katragkou, E., Marecal, V., Peuch, V.-H., Richter, A., Schultz, M. G., Stein, O., and Tsikerdekis, A.: Tropospheric chemistry in the Integrated Forecasting System of ECMWF, Geosci. Model Dev., 8, 975–1003, https://doi.org/10.5194/gmd-8-975-2015, 2015. a
Ingmann, P., Veihelmann, B., Langen, J., Lamarre, D., Stark, H., and Courrǵes-Lacoste, G. B.: Requirements for the GMES Atmosphere Service and ESA's implementation concept: Sentinels-4/-5 and -5p, Remote Sens. Environ., 120, 58–69, https://doi.org/10.1016/j.rse.2012.01.023, 2012. a
Krings, T., Gerilowski, K., Buchwitz, M., Hartmann, J., Sachs, T., Erzinger, J., Burrows, J. P., and Bovensmann, H.: Quantification of methane emission rates from coal mine ventilation shafts using airborne remote sensing data, Atmos. Meas. Tech., 6, 151–166, https://doi.org/10.5194/amt-6-151-2013, 2013. a, b
Kuenen, J. J. P., Visschedijk, A. J. H., Jozwicka, M., and Denier van der Gon, H. A. C.: TNO-MACC_II emission inventory; a multi-year (2003–2009) consistent high-resolution European emission inventory for air quality modelling, Atmos. Chem. Phys., 14, 10963–10976, https://doi.org/10.5194/acp-14-10963-2014, 2014. a
Kuenen, J. J. P., Visschedijk, A. J. H., Denier van der Gon, H. A. C., Jonkers, S., and Janssens-Maenhout, G.: TNO-CAMS European CO2 emissions 2000–2014 v1, TNO, https://doi.org/10.5281/zenodo.112889, 2017. a
Kuhlmann, G., Clément, V., Marschall, J., Fuhrer, O., Broquet, G., Schnadt-Poberaj, C., Löscher, A., Meijer, Y., and Brunner, D.: SMARTCARB – Use of Satellite Measurements of Auxiliary Reactive Trace Gases for Fossil Fuel Carbon Dioxide Emission Estimation, Final report of ESA study contract n°4000119599/16/NL/FF/mg, Tech. rep., Empa, Swiss Federal Laboratories for Materials Science and Technology, Dübendorf, Switzerland, available at: https://www.empa.ch/documents/56101/617885/FR_Smartcarb_final_Jan2019.pdf (last access: 25 November 2019), 2019. a, b, c, d
Lecomte, T., de la Fuente, J. F. F., Neuwahl, F., Canova, M., Pinasseau, A., Jankov, I., Brinkmann, T., Roudier, S., and Sancho, L. D.: Best Available Techniques (BAT) Reference Document for Large Combustion Plants – Industrial Emissions Directive 2010/75/EU Integrated Pollution Prevention and control, Tech. rep., 2017. a
Liu, Y., Gruber, N., and Brunner, D.: Spatiotemporal patterns of the fossil-fuel CO2 signal in central Europe: results from a high-resolution atmospheric transport model, Atmos. Chem. Phys., 17, 14145–14169, https://doi.org/10.5194/acp-17-14145-2017, 2017. a
Mahadevan, P., Wofsy, S. C., Matross, D. M., Xiao, X., Dunn, A. L., Lin, J. C., Gerbig, C., Munger, J. W., Chow, V. Y., and Gottlieb, E. W.: A satellite-based biosphere parameterization for net ecosystem CO2 exchange: Vegetation Photosynthesis and Respiration Model (VPRM), Global Biogeochem. Cy., 22, 2, https://doi.org/10.1029/2006GB002735, 2008. a
MOPITT Algorithm Development Team: Measurements of Pollution in the Troposphere (MOPITT) Version 7 Product User's Guide, available at: https://www2.acom.ucar.edu/sites/default/files/mopitt/v7_users_guide_201707.pdf (last access: 25 November 2019), 2017. a
Nassar, R., Hill, T. G., McLinden, C. A., Wunch, D., Jones, D. B. A., and Crisp, D.: Quantifying CO2 Emissions From Individual Power Plants From Space, Geophys. Res., 44, 10045–10053, https://doi.org/10.1002/2017GL074702, 2017. a
O'Dell, C. W., Connor, B., Bösch, H., O'Brien, D., Frankenberg, C., Castano, R., Christi, M., Eldering, D., Fisher, B., Gunson, M., McDuffie, J., Miller, C. E., Natraj, V., Oyafuso, F., Polonsky, I., Smyth, M., Taylor, T., Toon, G. C., Wennberg, P. O., and Wunch, D.: The ACOS CO2 retrieval algorithm – Part 1: Description and validation against synthetic observations, Atmos. Meas. Tech., 5, 99–121, https://doi.org/10.5194/amt-5-99-2012, 2012. a
Oney, B., Henne, S., Gruber, N., Leuenberger, M., Bamberger, I., Eugster, W., and Brunner, D.: The CarboCount CH sites: characterization of a dense greenhouse gas observation network, Atmos. Chem. Phys., 15, 11147–11164, https://doi.org/10.5194/acp-15-11147-2015, 2015. a
Pillai, D., Buchwitz, M., Gerbig, C., Koch, T., Reuter, M., Bovensmann, H., Marshall, J., and Burrows, J. P.: Tracking city CO2 emissions from space using a high-resolution inverse modelling approach: a case study for Berlin, Germany, Atmos. Chem. Phys., 16, 9591–9610, https://doi.org/10.5194/acp-16-9591-2016, 2016. a, b, c, d, e, f, g, h, i, j, k, l, m, n, o, p, q
Pinty, B., Janssens-Maenhout, G., Dowell, M., Zunker, H., Brunhe, T., Ciais, P., Dee, D., van der Gon, H. D., Dolman, H., Drinkwater, M., Engelen, R., Heimann, M., Holmlund, K., Husband, R., Kentarchos, A., Meijer, Y., Palmer, P., and Scholz, M.: An Operational Anthropogenic CO2 Emissions Monitoring & Verification Support capacity – Baseline Requirements, Model Components and Functional Architecture, Report, https://doi.org/10.2760/39384, 2018. a, b
Platnick, S., Ackerman, S., King, M., Wind, G., Meyer, K., Menzel, P., Frey, R., Holz, R., Baum, B., and Yang, P.: MODIS atmosphere L2 cloud product (06_L2), NASA MODIS Adaptive Processing System, Goddard Space Flight Center, https://doi.org/10.5067/MODIS/MYD06_L2.061, 2017. a
Reuter, M., Buchwitz, M., Hilboll, A., Richter, A., Schneising, O., Hilker, M., Heymann, J., Bovensmann, H., and Burrows, J.: Decreasing emissions of NOx relative to CO2 in East Asia inferred from satellite observations, Nat. Geosci, 7, 792–795, https://doi.org/10.1038/ngeo2257, 2014. a
Reuter, M., Buchwitz, M., Schneising, O., Krautwurst, S., O'Dell, C. W., Richter, A., Bovensmann, H., and Burrows, J. P.: Towards monitoring localized CO2 emissions from space: co-located regional CO2 and NO2 enhancements observed by the OCO-2 and S5P satellites, Atmos. Chem. Phys., 19, 9371–9383, https://doi.org/10.5194/acp-19-9371-2019, 2019. a, b, c
Schaaf, C. and Wang, Z.: MCD43A3 MODIS/Terra+Aqua BRDF/Albedo Daily L3 Global – 500m V006 [Data set], NASA EOSDIS Land Processes DAAC, https://doi.org/10.5067/MODIS/MCD43A3.006, 2015. a
Schaub, D., Brunner, D., Boersma, K. F., Keller, J., Folini, D., Buchmann, B., Berresheim, H., and Staehelin, J.: SCIAMACHY tropospheric NO2 over Switzerland: estimates of NOx lifetimes and impact of the complex Alpine topography on the retrieval, Atmos. Chem. Phys., 7, 5971–5987, https://doi.org/10.5194/acp-7-5971-2007, 2007. a
Sierk, B., Bézy, J.-L., Löscher, A., and Meijer, Y.: The European CO2 Monitoring Mission: observing anthropogenic greenhouse gas emissions from space, https://doi.org/10.1117/12.2535941, 2019. a
Taylor, T. E., O'Dell, C. W., Frankenberg, C., Partain, P. T., Cronk, H. Q., Savtchenko, A., Nelson, R. R., Rosenthal, E. J., Chang, A. Y., Fisher, B., Osterman, G. B., Pollock, R. H., Crisp, D., Eldering, A., and Gunson, M. R.: Orbiting Carbon Observatory-2 (OCO-2) cloud screening algorithms: validation against collocated MODIS and CALIOP data, Atmos. Meas. Tech., 9, 973–989, https://doi.org/10.5194/amt-9-973-2016, 2016. a, b
Ting, K. M.: Confusion Matrix, 209–209, Springer US, Boston, MA, https://doi.org/10.1007/978-0-387-30164-8_157, 2010. a
UNFCCC: Paris Agreement, FCCC/CP/2015/L.9/Rev1, available at: http://unfccc.int/resource/docs/2015/cop21/eng/l09r01.pdf (last access: 25 November 2019), 2015. a
Varon, D. J., Jacob, D. J., McKeever, J., Jervis, D., Durak, B. O. A., Xia, Y., and Huang, Y.: Quantifying methane point sources from fine-scale satellite observations of atmospheric methane plumes, Atmos. Meas. Tech., 11, 5673–5686, https://doi.org/10.5194/amt-11-5673-2018, 2018. a
Velazco, V. A., Buchwitz, M., Bovensmann, H., Reuter, M., Schneising, O., Heymann, J., Krings, T., Gerilowski, K., and Burrows, J. P.: Towards space based verification of CO2 emissions from strong localized sources: fossil fuel power plant emissions as seen by a CarbonSat constellation, Atmos. Meas. Tech., 4, 2809–2822, https://doi.org/10.5194/amt-4-2809-2011, 2011. a
v. Storch, H. and Zwiers, F. W.: Statistical Analysis in Climate Research, Cambride University Press, Cambridge, https://doi.org/10.1017/CBO9780511612336, 2003. a
Wenig, M. O., Cede, A. M., Bucsela, E. J., Celarier, E. A., Boersma, K. F., Veefkind, J. P., Brinksma, E. J., Gleason, J. F., and Herman, J. R.: Validation of OMI tropospheric NO2 column densities using direct-Sun mode Brewer measurements at NASA Goddard Space Flight Center, J. Geophys. Res., 113, D16S45, https://doi.org/10.1029/2007JD008988, 2008. a
- Article
(16051 KB) - Full-text XML